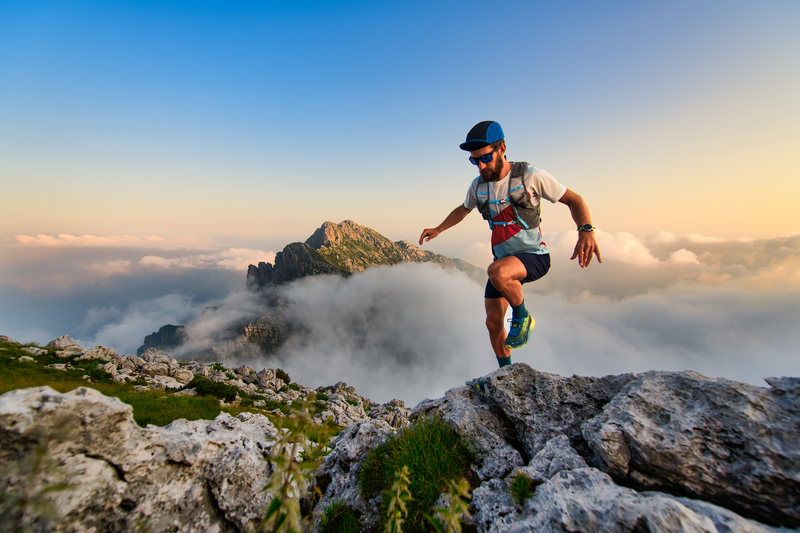
95% of researchers rate our articles as excellent or good
Learn more about the work of our research integrity team to safeguard the quality of each article we publish.
Find out more
ORIGINAL RESEARCH article
Front. Phys. , 31 March 2022
Sec. Optics and Photonics
Volume 10 - 2022 | https://doi.org/10.3389/fphy.2022.836523
This article is part of the Research Topic Advances in Polarimetry and Ellipsometry: Fundamentals and Applications View all 14 articles
In this work, we propose a high-throughput online identification method of bioaerosols based on multi-angle polarization index system (MAPIS). In the study, four categories and 10 subclasses of aerosol samples from biological and non-biological sources are detected under three incident polarization mode. Then their measured MAPIS shows that bioaerosols like pollen can be easily distinguished from other types of aerosols. Not only that, experimental results also indicate the feasibility of fine identification between different kinds of bioaerosols based on MAPIS in P and R modes. To further extract simple and optimized polarization characterization parameters suitable for bioaerosols, we analyze the multidimensional data of MAPIS by PCA then validate the aerosol recognition accuracy using the first two principal components by multiple groups of randomly mixed aerosol datasets. The comparison with PCA components based on only scattering intensity demonstrate that MAPIS can be not only applied in the specific identification of bioaerosols but also suitable for the distinction between different kinds of bioaerosols.
Bioaerosols are highly associated with a wide range of health effects with major public health impact [1]. It is important to develop some monitoring system that could offer the capability of real-time monitoring of biological aerosols [2]. Pollen is a major fraction of bioaerosols and is receiving increasing attention due to its high allergenic potential and the associated impacts on personal life quality and economy [3]. Pollens have various effects on human health and the environment. Plant pollens are similarly IgE binding allergens that may cause allergic reactions [4]. Airborne pollens are often considered major agents of allergy-related diseases [5] such as asthma, rhinitis, and atopic eczema [6, 7]. The allergenicity of some pollen is further enhanced by particulate pollution in the atmosphere [8]. Due to the effects of climate change on biota, the negative effects of airborne pollen on humans are increasing [9–11]. The number of people suffering from allergies due to pollen inhalation is increasing every year [12]. Also, for environment, pollen can also act as an environmental pollutant by acting as a nucleus for cloud droplets and ice crystals, affecting the solar radiation reaching earth and the optical properties of clouds, thereby reducing visibility [13].
In the area of public health and allergies, the monitoring and predicting of pollens is challenging, partly due to the lack of standardized and widely applicable offline laboratory analysis or online continuous monitoring methods [14]. Traditional pollen monitoring employs fluorescence microscopy, such as extractive staining fluorescence microscopy [15] and direct staining fluorescence microscopy [16]. Moreover, various imaging techniques have been used for pollen detection, such as scanning electron microscopy (SEM) [17], transmission electron microscopy (TEM) [18], x-ray imaging [19], etc. These techniques allow for single particle analysis but provide data at a relatively low time resolution due to time-consuming preparation steps or complicated setups [20–23].
Also, some other methods have been developed based on light scattering, ultraviolet laser-induced fluorescence, and holography combined with deep learning [24]. Wu developed a label-free bioaerosol sensor based on holographic microscopy and deep-learning, which is designed to get rid of transferring to laboratory and manual inspection [25]. Mitsumoto proposed a novel flow particle analyzer based on the design of flow cytometer [26]. The device classifies pollen species by simultaneously detecting both scattered light and the characteristic fluorescence excited by ultraviolet light in the flow cell. Kawashima developed a device which measures the sideward and forward scattering intensities of laser light from each particle to quantify a specific pollen type (Japanese cedar) in Japan [27].
Currently, there are very few related literatures on the polarization characteristics of pollens in ambient air, and the corresponding polarization measurement is only limited to the depolarization rate of pollens [28]. The optical properties of pollen particles can be described by the depolarization rate obtained in the lidar detection [22, 29]. Here non-spherical pollens can produce a strong depolarization rate, which can be distinguished from the background backscattering of other aerosols [22]. In addition, according to the depolarization rate [30–33], many studies have shown the potential to distinguish different kinds of pollens in the atmosphere. There is research work on the Muller matrix of ragweed pollen in the visible spectral range [28], which provides a feasible way to identify pollens by using precise polarimetric fingerprints.
Our previous studies have shown that multi-angle polarization index system (MAPIS) could be used for characterizing non-biologically derived aerosols such as dust [34], soot [35] or irregular particle samples [36]. In this study, focusing on bioaerosols, we detect the multi-angle polarization scattering signals of individual biological aerosols and then show their characteristic MAPIS different from other abiotic aerosol particles under different incident polarization states. The measured data of various types of aerosol samples are given and compared in this paper, including two dust type samples, two water-soluble type samples, two carbonaceous aerosol samples, and several kinds of bioaerosols (including three kinds of pollens and pearl powder). Each sub-category is measured independently. By principal component analysis (PCA), an unsupervised learning method, we extract some specific indicators based on MAPIS. The results show that, even without the assistance of fluorescence, only by MAPIS, we can accurately distinguish bioaerosols such as pollens from non-biologically derived particles and can also subdivide the subclasses of pollens. The technology used in this study has the advantages of non-invasive, online real-time and high-throughput analysis. These preliminary studies confirm the potential of MAPIS applied in a fine identification and characterization of bioaerosols.
Figure 1 shows the schematic diagram of our experimental setup, which has been presented in [37]. The light source is a solid laser (532 nm, 100 mW, MSL-III-532, Changchun New Industries Optoelectronics Technology Co., Ltd.). The incident light can be modulated into three polarization states (horizontal linear, 45° linear and right-handed circular polarization) by PSG (polarization state generator). We define these three measurement modes as H mode, P mode, and R mode. The polarized light is then focused at the center of the air flow by a cylindrical lens. The width and height of the laser spot is 1 and 0.04 mm. In the actual measurement, in order to judge whether any suspended particle is passing through the detection area, we use the intensity at 10° scattering angle as the trigger basis of polarization signal acquisition. When the forward 10° scattering signal exceeds the preset discrimination threshold, the scattered signals at four angles (30°, 60°, 85°, 115°) are then synchronously recorded. For each angle, a spatial filter module composed of a lens and an aperture at fixed location is used to eliminate the influence of stray light. Also, there is an optical trap at the end of laser beam to eliminate the forward stray light.
FIGURE 1. (A) Overview of structure of measuring instrument; (B) schematic diagram of experiment setup. PSG, polarization state generator (composed of a polarizing prism with a quarter wave plate for R mode and a half wave plate for H and P mode); C, cylindrical lens; L, spatial filter module (composed of a lens and an aperture); P1-P4, scattered light polarization signal channel at four angles (30°, 60°, 85°, 115°); I, scattered light intensity signal channel at 10°; PSA, polarization state analyzer; FP, film polarizer; PC, personal computer.
A four-quadrant polarization state analyzer (0°, 90°, 45°, 135° linear polarizer) is applied at each angle. We also test every four-quadrant polarization module using polarimeter to ensure the orientation deviation of polarizers no more than 2°. The scattered light is spatially divided into four parts and transmitted respectively to four SiPMT detectors via an optical fiber bundle. The light intensity is converted and recorded by data acquisition device (FCFR-USB2068, Fcctec Technology, China) at a sampling rate of 1 MHz. The Stokes parameter elements S0, S1, and S2 could be easily calculated as described in Eq. 1. Currently we only use linear polarizing films due to the restrictions of the manufacture process, so this study does not involve the circular polarization items. Even so, S1 and S2 of Stokes vector at multi-angles already shows ability to characterize bioaerosols such as pollen.
An optimally designed sheath nozzle is used to make sure particles passing through the center of detection area one by one. Sample flow carries sample particles passing through the laser beam within the protection of sheath flow. The effectiveness of the instrument is verified by experiments of standard PSL particles, which has been mentioned in our previous work [38]. The agreement between the measured results and Mie theoretical calculation results based on a single scattering assumption can further confirm that the multiple scattering is hardly involved in our measurements. The velocity of air flow is controlled by two-flow controller and a gas pump. A particle flies through the detection area within 50 μs and we sample one point of signal every 1 µs. Thus, our current instrument can obtain signals of up to around 20,000 particles in about 1 s.
We choose four types of typical aerosol samples with different properties: dust, water-soluble salts, carbon, and biologically derived particles. Arizona dust and fly ash are measured as representation of dust. Sodium sulfate and sodium chloride are measured as representation of water-soluble salts. Disordered mesoporous carbon and hollow carbon spheres are measured as representation of carbon aerosols. Chamomile pollen, rose pollen, Osmanthus pollen, and pearl powder are measured as representation of biologically derived aerosols. Each subclass above is measured independently. The Stokes vector elements S0, S1, and S2 are measured at four angles for each measurement mode. Pollen is a common and easily accessible class of biological aerosols. It should be noted that these pollen samples were provided by the drug supplier (Yiqi Herbs), and the pollen went through the grinding process which caused their size to be smaller, but its composition unchanged. According to Ref. [39–42], the untreated pollen size will be larger than 10 um. For example, the diameter of Chamomile Pollen is around 16.6 um [39]. As for pearl powder, it is a mixture of protein (β-chitin, silk-like proteins, and acidic glycoproteins) and calcium carbonate [43, 44], which can also belong to biomass source in composition.
Before measurements, aerosol particles from dust type, carbon type and biological type are screened through a 500-mesh sieve to ensure a relatively uniform particle size and then generated and diffused into uniformly dispersed suspended particles by the TSI-3400A aerosol generator. Salt aerosols of water-soluble type are atomized by a Met One 255 atomizer and then pass through a drying tube. All the detailed morphology information of samples can be found in Table 1. The particle size after screening in our experiments is less than 10 microns and was monitored synchronously by an optical particle sizer. In our experiments, we used optical particle sizer (OPS-3330, TSI) for particle size measurement. The measurement process and the accuracy of the OPS can be referenced in [45].
The Stokes parameters S1 and S2 at four angles in each measurement mode for different types of aerosol samples are shown in Figure 2. In Figure 2, non-biological samples are represented by dots of different colors, while pollen samples are represented by green series cross-symbols. For each sub-category sample, we randomly select 10,000 measured data points to display for convenience. Apparently, compared with the differences within sub-categories of non-biological particles, the difference between non-biological origin samples and bioaerosol samples are significantly larger intuitively in terms of multi-angle polarization index system (MAPIS) regardless of the measurement mode.
FIGURE 2. Pollen and non-biological samples under the multi-angle polarization index system (MAPIS), 10,000 samples for each type.
PCA is defined as an orthogonal linear transformation that transforms the data to a new coordinate system such that the greatest variance by some scalar projection of the data comes to lie on the first coordinate (called the first principal component), the second greatest variance on the second coordinate, and so on [46]. The first principal component can be considered as a projection direction that can best explain the data difference. Then, the ability of the second and third principal components to explain the data difference decreases in turn. Therefore, when we distinguish between biological and non-biological aerosols, the first principal component from the measured data of all kinds of aerosols provides a possible optimal expression for the distinction between these two categories. Similarly, when we further want to accurately identify different subclasses under the category of biological aerosols, the first principal component from the measured data of only various bioaerosols can used as a classification parameter to identify which kind of biological aerosol is detected.
Here we define X as a measured multidimensional data matrix, and w as a weight coefficient matrix of each principal component. Then the weight coefficient vector of the first principal component, w1, can be obtained by optimizing Rayleigh quote.
To further extract some specific indicator to distinguish between bioaerosols and abiotic particles, we employ the PCA (Principal Component Analysis) method to analyze the measured MAPIS. The PCA results under different measurement modes are shown in Figures 3A–C. PCA is an unsupervised learning method, which means that the input data of PCA does not contain the type information of each particle point. Based on the data distribution along the horizontal axis direction of Figures 3A–C, the first principal component extracted by PCA, that is, the direction that shows the overall maximum variance of data, can perfectly separate pollen samples and non-biological samples. Next, the intra-class differences of pollen samples and non-biological samples are roughly along the vertical axis direction of Figures 3A–C, implying that the second principal component probably is suitable for the subdivision of different bioaerosols. The PCA coefficients and interpretation coefficients of the first two principal components for different measurement modes are shown in Table 2. Regardless of the measurement mode, the contribution by the extracted first principal component is significantly greater than the contribution of the second principal component.
FIGURE 3. Pollen and non-biological samples on PCA first and second principal component. (A) MAPIS under H mode; (B) MAPIS under P mode; (C) MAPIS under R mode. (D) S0 at four angles under H mode; (E) S0 at four angles under P mode; (F) S0 at four angles under R mode.
TABLE 2. Principal component coefficients and interpretation coefficients under different mode for pollen and non-biological samples MAPIS data.
As a reference, we also use S0 at four scattering angles in three measurement modes as input for PCA operation. The results are shown in Figures 3D–F, which is similar with the sideward and forward scattering intensities measured in [27]. The PCA results using only multi-angle scattering intensity are quite similar under different incident polarization modes. There is not much difference in the relative positions of different samples, and the difference is likely due to the rotation of the coordinate system. So, the intensity of scattered light from multiple angles alone is not enough to distinguish bioaerosols and non-biological origin particles. However, with the help of MAPIS based on linear polarization vector analysis of the detected light, the high discrimination and specific recognition of bioaerosols can be easily realized. By PCA, we can further extract the first principal components as a good indicator specifically for bioaerosols like pollen.
Concretely, for MAPIS under H mode, we can set the position where the first principal component is equal to −0.5 as the discrimination line, and then determine that the measured data whose value range is on the left of this line comes from biological particles. Similarly, for P mode, the discrimination line can be set at the position of the first principal component equal to 0.4, and for R mode, the line can be set at the position of the first principal component equal to 0.25.
The above discrimination basis can be evaluated on 15 measured datasets which is randomly generated. Each dataset contains measured MAPIS data of bioaerosols and non-biological particles mixed with a certain proportion, and the predicted proportions using the above judgment and the comparison with the preset proportion can be shown in Figure 4. We preset five particle number contents of biological aerosols in the mixed dataset, and then randomly extract data three times for each specified proportion to establish total 15 verification datasets.
FIGURE 4. True and predicted proportion of pollen using PC1 from MAPIS. (A) Measurement conducted in H mode; (B) Measurement conducted in P mode; (C) Measurement conducted in R mode.
For all the 15 datasets, Figure 4 indicates minor deviations less than 1% between the predicted and true proportion, which further confirms the feasibility of our method to specifically distinguish between bioaerosols and non-biological particles. By an auxiliary observation using a particle size analyzer, there is little difference in the particle size distribution interval of the measured samples. SEM photos of bioaerosols reveal more complex and regular microstructures compared to non-biological particles. So, the polarization optical difference between non-biological particles and bioaerosols may be due to the microstructures combined with the complex refractive index factor. The relevant detailed microphysical interpretation needs to be further studied.
Next, the measured MAPIS of the sub-categories of bioaerosols are shown in Figure 5. Compared with Figure 2, various Stokes elements at different angles and for different incident polarization states have different recognition abilities. Specifically, the polarization indexes in H mode show a weaker discrimination than those in P mode and R mode. Both the forward (30° and 60°) polarized scattering signals in P mode and the backward (85° and 115°) polarized scattering signals in R mode seem to be suitable for the classification and identification of different kinds of biological aerosols.
Similarly, PCA is used to analyze the measured Stokes element S1 and S2 at four angles in each incident polarization mode and extract the optimized polarization characterization expression. The PCA coefficients and interpretation coefficients of the first two principal components in different measurement modes are shown in Table 3, and the measured data distribution of different biological particles using the first two principal components is shown in Figures 6A–C. It can be seen that four bioaerosols are almost inseparable in H mode but can be clearly distinguished by the principal components in P and R mode. The class separation distances among biological samples using MAPIS in R mode show better discrimination of biological aerosol species than using data in P mode, which implies that particles of biological origin are more sensitive to circularly polarized incident light.
TABLE 3. Principal component coefficients and interpretation coefficients under different mode for biological samples MAPIS data
FIGURE 6. Biological samples on PCA first and second principal component. (A) MAPIS under H mode; (B) MAPIS under P mode; (C) MAPIS under R mode. (D) S0 at four angles under H mode; (E) S0 at four angles under P mode; (F) S0 at four angles under R mode.
Also, we extract principal components from the scattering intensity S0 at four angles in three measurement modes for comparison, which is shown in Figures 6D–F. Similar with the case in Figures 3D–F, the PCA results using only multi-angle scattering intensity cannot distinguish different kinds of bioaerosols. There is not much difference in the relative positions of the measured data.
Then, we used a verification method similar to that in Section 3.1; we constructed five measured datasets of four biological aerosols mixed with different ratios. Based on the statistical distribution on the first principal component for each incident polarization mode, we can predict the proportion of different kinds of bioaerosols and compare them with the preset ratios. The number of aerosol class measured is k; xn is the probability density curve of the nth aerosol sample on the first principal component. y is the measured probability density curve on the first principal component for a mixture of these k kinds of aerosols, where y and xn are vectors. If αn is the estimated proportion of the nth aerosol class, we multiply and sum the probability density curve for polarization parameters of different kinds of bioaerosols by their proportions, then by fitting the probability density curve based on the estimated mixing ratio of different aerosol class with the real y based on randomly sampled measured datasets. By least square method in Eq. 1, the optimal solution of proportions could be found out, as shown in Figure 7.
FIGURE 7. True and predicted proportion of biological samples using MAPIS. (A) Measurement conducted in H mode; (B) Measurement conducted in P mode; (C) Measurement conducted in R mode.
By observing the classification results based on the measured biological aerosol datasets with five different mixture ratios, using MAPIS measured in P and R mode, the identification error of the first principal component is less than 3% for all the datasets. Compared with other abiotic types, the differences of polarization parameters among various bioaerosols are not so big. However, with the help of PCA, different measured Stokes indexes can be combined to form an optimized parameter with a sufficient discrimination suitable for bioaerosol classification.
In this paper, we investigate the characterization ability of the multi-angle polarization index system (MAPIS) for bioaerosols (especially pollen). Stokes vectors S1 and S2 of 10 kinds of aerosol samples are measured at four scattering angles under three incident polarization states. The types of samples can be divided into four major categories, namely, dust, water-soluble salts, carbon, and bioaerosols. Among them, the first three types belong to non-biological particles, and each of them contains two subclass samples. There are four kinds of bioaerosols, mainly pollens.
Experiment results show that, regardless of the polarization state of the incident light, non-biological particles and bioaerosols can be clearly differentiated based on the measured MAPIS. Moreover, when the incident light is 45° linear polarized or circular polarized, we can also subdivide the kind of bioaerosols according to the data distribution of MAPIS. By comparison with the measured data of multi-angle scattering intensity, the scattering signals without polarization analysis are not sufficient to determine whether the particulate matter is of biological origin or distinguish the sub-categories of bioaerosols. To simplify the multidimensional characterization parameters of MAPIS, the first two principal components extracted by a PCA analysis of all 10 kinds of sample data can be used as specific indicators of bioaerosols. Also, another PCA analysis of four kinds of biological sample data can confirm the feasibility of its first principal component to predict the particle proportion of mixed bioaerosol samples.
To fully obtain and understand the polarization scattering response from more types of biological aerosols, we still have a lot of follow-up work to promote. The limitations of biological aerosol samples in this paper will affect the universality of specific indicators of polarization characterization and related errors. However, the research of this paper still shows the potentials of the synchronous polarization analysis at multi scattering angles. Taking pollen as an example, the microphysical differences between real biological aerosols and abiotic aerosols are difficult to be simply attributed to size or composition factors. Based on the measured MAPIS and the information extraction by machine learning, the accurate discrimination and fine classification of biological aerosols like pollen are feasible in on-line high-throughput measurements. The above studies demonstrate the characterization ability of the multi-angle polarization index system (MAPIS) for in situ fast identification of bioaerosols from other non-biological particles. Also, we can subdivide different biological particles based on measured MAPIS of various aerosol samples. PCA analysis can help us extract one or two optimized polarization indexes based on the combination of multiple Stokes vector elements, according to different characterization needs for bioaerosols. Using the first principal component respectively from ten kinds of sample data and four kinds of biological sample data, the specific recognition error of biological type aerosols is no more than 1%, and the discrimination error of different bioaerosols is less than 3%. Our preliminary study lays a solid foundation to further apply polarization technology and method to analyze more important aerosols such as bacteria and virus particles.
The raw data supporting the conclusion of this article will be made available by the authors, without undue reservation.
NZ and QX conceived the idea of the manuscript. QX and WG prepare the samples and performed the experiments. QX wrote the original manuscript and analyzed the results. NZ, JG, YH, and HM performed the language editing. All authors have given approval to the final version of the manuscript.
This work is supported by the National Key Program of Science and Technology Supporting Economy of China (2020YFF01014500ZL); Science and Technology Research Program of Shenzhen Grant (JCYJ20200109142820687).
The authors declare that the research was conducted in the absence of any commercial or financial relationships that could be construed as a potential conflict of interest.
The handling editor declared a past co-authorship with one of the authors HM.
All claims expressed in this article are solely those of the authors and do not necessarily represent those of their affiliated organizations, or those of the publisher, the editors, and the reviewers. Any product that may be evaluated in this article, or claim that may be made by its manufacturer, is not guaranteed or endorsed by the publisher.
1. Douwes J, Thorne P, Pearce N, Heederik D. Bioaerosol Health Effects and Exposure Assessment: Progress and Prospects. Ann Occup Hyg (2003) 47(3):187–200. doi:10.1093/annhyg/meg032
2. Miaomiao T, Fangxia S, Maosheng Y, Tong Z. Development of an Automated Electrostatic Sampler (AES) for Bioaerosol Detection. Aerosol Sci Tech (2011) 45(9):1154–60. doi:10.1080/02786826.2011.582193
3. Pöhlker C, Huffman JA, Förster J-D, Pöschl U. Autofluorescence of Atmospheric Bioaerosols: Spectral Fingerprints and Taxonomic Trends of Pollen. Atmos Meas Tech (2013) 6:3369–92. doi:10.5194/amt-6-3369-2013
4. King MD, Lacey RE, Pak H, Fearing A, Ramos G, Baig T, et al. Assays and Enumeration of Bioaerosols-Traditional Approaches to Modern Practices. Aerosol Sci Tech (2020) 54:611–33. doi:10.1080/02786826.2020.1723789
5. Taketomi EA, Sopelete MC, de Sousa Moreira PF, de Assis Machado Vieira F. Pollen Allergic Disease: Pollens and its Major Allergens. Braz J Otorhinolaryngol (2006) 72:562–7. doi:10.1016/S1808-8694(15)31005-3
6. Weber RW, Adkinson N, Bochner B, Burks A, Busse W, Holgate S, et al. Middleton’s Allergy: Principles and Practice. Elsevier Health Sciences (2013). p. 430.
7. Bousquet J, Khaltaev N, Cruz AA, Denburg J, Fokkens WJ, Togias A, et al. Allergic Rhinitis and its Impact on Asthma (ARIA) 2008 Update (In Collaboration with the World Health Organization, GA(2)LEN and AllerGen). Allergy (2008) 63:8–160. doi:10.1111/j.1398-9995.2007.01620.x
8. Behrendt H, Becker W-M. Localization, Release and Bioavailability of Pollen Allergens: the Influence of Environmental Factors. Curr Opin Immunol (2001) 13:709–15. doi:10.1016/S0952-7915(01)00283-7
9. Beggs PJ. Impacts of Climate Change on Aeroallergens: Past and Future. Clin Exp Allergy (2004) 34:1507–13. doi:10.1111/j.1365-2222.2004.02061.x
10. D'amato G, Cecchi L. Effects of Climate Change on Environmental Factors in Respiratory Allergic Diseases. Clin Exp Allergy (2008) 38:1264–74. doi:10.1111/j.1365-2222.2008.03033.x
11. Shea KM, Truckner RT, Weber RW, Peden DB. Climate Change and Allergic Disease. J Allergy Clin Immunol (2008) 122:443–53. doi:10.1016/j.jaci.2008.06.032
12. Schmidt CW. Pollen Overload: Seasonal Allergies in a Changing Climate. Environ Health Perspect (2016) 124:A70–5. doi:10.1289/ehp.124-A70
13. Steiner AL, Brooks SD, Deng C, Thornton DCO, Pendleton MW, Bryant V. Pollen as Atmospheric Cloud Condensation Nuclei. Geophys Res Lett (2015) 42:3596–602. doi:10.1002/2015GL064060
14. Walser SM, Gerstner DG, Brenner B, Bünger J, Eikmann T, Janssen B, et al. Evaluation of Exposure-Response Relationships for Health Effects of Microbial Bioaerosols - A Systematic Review. Int J Hyg Environ Health (2015) 218:577–89. doi:10.1016/j.ijheh.2015.07.004
15. Dong L, Qi J, Shao C, Zhong X, Gao D, Cao W, et al. Concentration and Size Distribution of Total Airborne Microbes in Hazy and Foggy Weather. Sci Total Environ (2016) 541:1011–8. doi:10.1016/j.scitotenv.2015.10.001
16. Perrino C, Marcovecchio F. A New Method for Assessing the Contribution of Primary Biological Atmospheric Particles to the Mass Concentration of the Atmospheric Aerosol. Environ Int (2016) 87:108–15. doi:10.1016/j.envint.2015.11.015
17. Schrank E. Scanning Electron and Light Microscopic Investigations of Angiosperm Pollen from the Lower Cretaceous of Egypt. Pollen et Spores (1983) 25:213–42.
18. Behnke H-D. Transmission Electron Microscopy and Systematics of Flowering Plants. In: Flowering Plants. Springer (1977). p. 155–78. doi:10.1007/978-3-7091-7076-2_11
19. Wang S, Wang D, Wu Q, Gao K, Wang Z, Wu Z. 3D Imaging of a rice Pollen Grain Using Transmission X-ray Microscopy. J Synchrotron Radiat (2015) 22:1091–5. doi:10.1107/S1600577515009716
20. Chen L-WA, Zhang M, Liu T, Fortier K, Chow JC, Alonzo F, et al. Evaluation of Epifluorescence Methods for Quantifying Bioaerosols in fine and Coarse Particulate Air Pollution. Atmos Environ (2019) 213:620–8. doi:10.1016/j.atmosenv.2019.05.051
21. Park C, Lee S, Kim G, Lee S, Lee J, Heo T, et al. Three-dimensional Refractive-index Distributions of Individual Angiosperm Pollen Grains. Curr Opt Photon (2018) 2:460–7. doi:10.3807/COPP.2018.2.5.460
22. Shang X, Giannakaki E, Bohlmann S, Filioglou M, Saarto A, Ruuskanen A, et al. Optical Characterization of Pure Pollen Types Using a Multi-Wavelength Raman Polarization Lidar. Atmos Chem Phys (2020) 20:15323–39. doi:10.5194/acp-20-15323-2020
23. Swanson BE, Huffman JA. Pollen Clustering Strategies Using a Newly Developed Single-Particle Fluorescence Spectrometer. Aerosol Sci Tech (2020) 54:426–45. doi:10.1080/02786826.2019.1711357
24. Huffman JA, Perring AE, Savage NJ, Clot B, Crouzy B, Tummon F, et al. Real-time Sensing of Bioaerosols: Review and Current Perspectives. Aerosol Sci Tech (2020) 54:465–95. doi:10.1080/02786826.2019.1664724
25. Wu Y, Calis A, Luo Y, Chen C, Lutton M, Rivenson Y, et al. Label-free Bioaerosol Sensing Using mobile Microscopy and Deep Learning. ACS Photon (2018) 5:4617–27. doi:10.1021/acsphotonics.8b01109
26. Mitsumoto K, Yabusaki K, Kobayashi K, Aoyagi H. Development of a Novel Real-Time Pollen-Sorting Counter Using Species-specific Pollen Autofluorescence. Aerobiologia (2010) 26:99–111. doi:10.1007/s10453-009-9147-1
27. Kawashima S, Thibaudon M, Matsuda S, Fujita T, Lemonis N, Clot B, et al. Automated Pollen Monitoring System Using Laser Optics for Observing Seasonal Changes in the Concentration of Total Airborne Pollen. Aerobiologia (2017) 33:351–62. doi:10.1007/s10453-017-9474-6
28. Cholleton D, Bialic E, Dumas A, Kaluzny P, Rairoux P, Miffre A. Laboratory Evaluation of the (VIS, IR) Scattering Matrix of Complex-Shaped Ragweed Pollen Particles. J Quant Spectrosc Radiat Transf (2020) 254:107223–9. doi:10.1016/j.jqsrt.2020.107223
29. Noh YM, Müller D, Lee H, Choi TJ. Influence of Biogenic Pollen on Optical Properties of Atmospheric Aerosols Observed by Lidar over Gwangju, South Korea. Atmos Environ (2013) 69:139–47. doi:10.1016/j.atmosenv.2012.12.018
30. Cao X, Roy GA, Bernier R. Lidar Polarization Discrimination of Bioaerosols. Opt Eng (2010) 49:116201. doi:10.1117/1.350587710.1117/12.849649
31. Noh YM, Müller D, Lee H, Choi TJ. Influence of Biogenic Pollen on Optical Properties of Atmospheric Aerosols Observed by Lidar over Gwangju, South Korea. Atmos Environ (2013) 69:139–47. doi:10.1016/j.atmosenv.2012.12.018
32. Sassen K. Boreal Tree Pollen Sensed by Polarization Lidar: Depolarizing Biogenic Chaff. Geophys Res Lett (2008) 35:1–4. doi:10.1029/2008GL035085
33. Sicard M, Izquierdo R, Alarcón M, Belmonte J, Comerón A, Baldasano JM. Near-surface and Columnar Measurements with a Micro Pulse Lidar of Atmospheric Pollen in Barcelona, Spain. Atmos Chem Phys (2016) 16:6805–21. doi:10.5194/acp-16-6805-2016
34. Li D, Chen F, Zeng N, Qiu Z, He H, He Y, et al. Study on Polarization Scattering Applied in Aerosol Recognition in the Air. Opt Express (2019) 27:A581–A595. doi:10.1364/OE.27.00A581
35. Li D, Zeng N, Zhan D, Chen Y, Zeng M, Ma H. Differentiation of Soot Particulates in Air Using Polarized Light Scattering Method. Appl Opt (2017) 56:4123–9. doi:10.1364/AO.56.004123
36. Chen Y, Zeng N, Chen S, Zhan D, He Y, Ma H. Study on Morphological Analysis of Suspended Particles Using Single Angle Polarization Scattering Measurements. J Quantitative Spectrosc Radiative Transfer (2019) 224:556–65. doi:10.1016/j.jqsrt.2018.12.006
37. Liao R, Zeng N, Zeng M, He Y, Ma H. Estimation and Extraction of the Aerosol Complex Refractive index Based on Stokes Vector Measurements. Opt Lett (2019) 44:4877–80. doi:10.1364/OL.44.004877
38. Liao R, Guo W, Zeng N, Guo J, He Y, Di H, et al. Polarization Measurements and Evaluation Based on Multidimensional Polarization Indices Applied in Analyzing Atmospheric Particulates. Appl Sci (2021) 11:5992. doi:10.3390/app11135992
39. Stanski C, Moro RSS, Nogueira MKF, Kuniyoshi YS, da Luz CFP. Palynology of Species of Anthemideae, Eupatorieae, Inuleae, Mutiseae and Senecianeae Tribes Occurring in the Region of Campos Gerais, Paraná State, Brazil. Iheringia Ser Bot (2018) 73:353–62. doi:10.21826/2446-8231201873312
40. Singh K, Sharma YP, Sharma PR, Gairola S. Pollen Morphology and Variability of the Rosa L. Species of Western Himalaya in India. Genet Resour Crop Evol (2020) 67:2129–48. doi:10.1007/s10722-020-00967-8
41. Duan Y, Li W, Zheng S, Sylvester SP, Li Y, Cai F, et al. Functional Androdioecy in the Ornamental Shrub Osmanthus Delavayi (Oleaceae). Plos One (2019) 14:e0221898. doi:10.1371/journal.pone.0221898
42. Yu ZR, Wang XD, Su BM, Zhang Y. First Evidence of the Use of Freshwater Pearls as a Cosmetic in Ancient China: Analysis of white Makeup Powder from a Northern Song Dynasty Lv Tomb (Lantian, Shaanxi Province, China). Archaeometry (2017) 59:762–74. doi:10.1111/arcm.12268
43. Chen X, Peng L-H, Chee S-S, Shan Y-H, Liang W-Q, Gao J-Q. Nanoscaled Pearl Powder Accelerates Wound Repair and Regeneration In Vitro and In Vivo. Drug Dev Ind Pharm (2019) 45:1009–16. doi:10.1080/03639045.2019.1593436
44. Dai J, Yang S, Jin J, Li G. Electrospinning of PLA/pearl Powder Nanofibrous Scaffold for Bone Tissue Engineering. RSC Adv (2016) 6:106798–805. doi:10.1039/C6RA21796F
45. Guo W, Zeng N, Liao R, Xu Q, Guo J, He Y, et al. Simultaneous Retrieval of Aerosol Size and Composition by Multi-Angle Polarization Scattering Measurements. Opt Lasers Eng (2022) 149:106799. doi:10.1016/j.optlaseng.2021.106799
46. Wold S, Esbensen K, Geladi P. Principal Component Analysis. Chemometrics Intell Lab Syst (1987) 2:37–52. doi:10.1016/0169-7439(87)80084-9
47. Fletcher R, Bright D. Shape Factors of ISO 12103-A3 (Medium Test Dust). Filtration + Sep (2000) 37:48–56. doi:10.1016/S0015-1882(00)80200-1
48. Groundwater H, Twardowski MS, Dierssen HM, Sciandra A, Freeman SA. Determining Size Distributions and Composition of Particles Suspended in Water: A New SEM-EDS Protocol with Validation and Comparison to Other Methods. J Atmos Oceanic Tech (2012) 29:433–49. doi:10.1175/JTECH-D-11-00026.1
49. Keskinen H, Kortelainen A-M, Jaatinen A, Yli-Pirilä P, Joutsensaari J, Romakkaniemi S, et al. Increased Hygroscopicity of Arizona Test Dust Seeds by Secondary Organic Aerosol Coating from α-pinene Ozonolysis. Boreal Environ Res (2014) 19:182–90.
50. Chen H, Laskin A, Baltrusaitis J, Gorski CA, Scherer MM, Grassian VH. Coal Fly Ash as a Source of Iron in Atmospheric Dust. Environ Sci Technol (2012) 46:2112–20. doi:10.1021/es204102f
51. Wang S, Baxter L, Fonseca F. Biomass Fly Ash in concrete: SEM, EDX and ESEM Analysis. Fuel (2008) 87:372–9. doi:10.1016/j.fuel.2007.05.024
52. Chuan Z, Ji-Tong W, Xu L, Dong-Hui L, Wen-Ming Q, Li-Cheng L. Facile Preparation, Structural Control and Spheroidization of Mesoporous Carbons Using Hydrolyzed Water Glass as a Template. J Inorg Mater (2015) 30:848–54. doi:10.15541/jim20150036
53. Li S, Pasc A, Fierro V, Celzard A. Hollow Carbon Spheres, Synthesis and Applications - a Review. J Mater Chem A (2016) 4:12686–713. doi:10.1039/C6TA03802F
54. Yuan C, Liu X, Jia M, Luo Z, Yao J. Facile Preparation of N- and O-Doped Hollow Carbon Spheres Derived from Poly(o-Phenylenediamine) for Supercapacitors. J Mater Chem A (2015) 3:3409–15. doi:10.1039/C4TA06411A
55. Yang Q. Pollen Morphology of Osmanthus Decorus and O.× Burkwoodii. J Hubei Univ Nationalities-Natural Sci Edition (2010) 28:286–8.
56. Kandler K, Benker N, Bundke U, Cuevas E, Ebert M, Knippertz P, et al. Chemical Composition and Complex Refractive index of Saharan Mineral Dust at Izaña, Tenerife (Spain) Derived by Electron Microscopy. Atmos Environ (2007) 41:8058–74. doi:10.1016/j.atmosenv.2007.06.047
57. Wyatt PJ. Some Chemical, Physical, and Optical Properties of Fly Ash Particles. Appl Opt (1980) 19:975–83. doi:10.1364/AO.19.000975
58. Tang IN. Chemical and Size Effects of Hygroscopic Aerosols on Light Scattering Coefficients. J Geophys Res (1996) 101:19245–50. doi:10.1029/96JD03003
Keywords: polarization scattering, bioaerosol, stokes vector, PCA, pollen
Citation: Xu Q, Zeng N, Guo W, Guo J, He Y and Ma H (2022) Multi-Angle Polarization Index System for Pollen Type Bioaerosol Recognition. Front. Phys. 10:836523. doi: 10.3389/fphy.2022.836523
Received: 15 December 2021; Accepted: 17 February 2022;
Published: 31 March 2022.
Edited by:
Ji Qi, Imperial College London, United KingdomReviewed by:
Jean-Baptiste Renard, Laboratoire de Physique et Chimie de l'Environnement et de l'Espace (LPC2E), FranceCopyright © 2022 Xu, Zeng, Guo, Guo, He and Ma. This is an open-access article distributed under the terms of the Creative Commons Attribution License (CC BY). The use, distribution or reproduction in other forums is permitted, provided the original author(s) and the copyright owner(s) are credited and that the original publication in this journal is cited, in accordance with accepted academic practice. No use, distribution or reproduction is permitted which does not comply with these terms.
*Correspondence: Nan Zeng, emVuZ25hbkBzei50c2luZ2h1YS5lZHUuY24=; Hui Ma, bWFodWlAdHNpbmdodWEuZWR1LmNu
Disclaimer: All claims expressed in this article are solely those of the authors and do not necessarily represent those of their affiliated organizations, or those of the publisher, the editors and the reviewers. Any product that may be evaluated in this article or claim that may be made by its manufacturer is not guaranteed or endorsed by the publisher.
Research integrity at Frontiers
Learn more about the work of our research integrity team to safeguard the quality of each article we publish.