- School of Management, Harbin Institute of Technology, Harbin, China
Fake news spreads rapidly on social networks; the aim of this study is to compare the characteristics of the social relationship networks (SRNs) of refuters and non-refuters to provide a scientific basis for developing effective strategies for debunking fake news. First, based on six types of fake news published on Sina Weibo (a Chinese microblogging website) during 2015–2019 in China, a deep learning method was used to build text classifiers for identifying debunked posts (DPs) and non-debunked posts (NDPs). Refuters and non-refuters were filtered out, and their follower–followee relationships on social media were obtained. Second, the differences between DPs and NDPs were compared in terms of the volume and growth rate of the posts across various types of fake news. The SRNs of refuters and non-refuters and the
Introduction
Since the 2016 U.S. presidential election, “fake news” has become a common term in the mainstream vernacular [1, 2]. Similar to Western countries, fake news is also prevalent in China [3], and it largely involves online rumors (yao yan in Chinese [4]). Furthermore, with the rapid development of social networking services (SNSs), each user in a social network has become both a spreader and receiver of information, and millions of people present, comment on, or share various topics on social media every day [5]. In addition, the emergence of social media as an information dissemination channel has reduced the gap between content producers and consumers and profoundly changed the way users obtain information, debate, and shape their attitudes [6, 7]. Although authoritative organizations such as the government and news media have made considerable efforts to debunk fake news, social media enables individuals to rapidly distribute fake news through social networks owing to its abundance of users and complex network structure, causing considerable panic in society [8, 9].
Effective debunking can be considered as a competition between debunked and non-debunked information. Thus, if we need to reduce and combat the proliferation of fake news on social media, we must improve the identification of the spread of the differences between debunked and non-debunked information on social media, make debunked information an effective hedge against non-debunked information, and understand the structure and functions of these technologically advanced social networks [10].
A better understanding of debunking strategies associated with different types of fake news can help address fake news more specifically on social media. As most researchers have concentrated on politically sensitive or field-specific fake news on social media, it has been difficult to derive common rules applicable to countering all types of fake news generated within a certain field [11]. Although in previous studies, the differences between the spread of facts and that of rumors on Twitter across various topics [12] or between the typical features of rumor and anti-rumor accounts on Sina Weibo [9] have been comprehensively evaluated, the differences between different types of debunked and non-debunked fake news based on real data on Chinese social media have been thoroughly assessed in only a few studies.
Social media (e.g., Sina Weibo) are information dissemination platforms based on social relationships (follower-followee relationships), wherein information dissemination is closely related to people’s social relationships [13], especially in networks that are large, complex, heterogeneous, and scalable [14]. Thus, people’s social relationships and information dissemination networks are interrelated and mutually reinforcing. The breadth and depth of people’s social relationship networks (SRNs) determine the breadth and depth of the information they obtain and how far the dissemination of this information can spread. Although the methods used for the study of fake news, such as fake news debunking detection (e.g., using features extracted from news articles and their social contexts, such as textual features and users’ profiles [15]) and diffusion network structure analysis (e.g., reposting or commenting networks [16]), have been identified, the ways in which users’ social relationships on social networks influence the spread of debunking messages have not been widely investigated. Additionally, these problems are important to address because, if a debunking methodology for fake news is not shared quickly and widely on social networks, people will fail to combat fake news in a timely and effective manner. Consequently, false information will continue to misguide public opinion on social media [17].
To meet the aforementioned objectives, first, we used a dataset containing 49,278 posts from 176 fake news events published on Sina Weibo from July 2015 to September 2019 and divided the fake news into six topics. Second, we developed a text classifier using deep learning by applying a long short-term memory (LSTM) algorithm to identify debunked posts (DPs) and non-debunked posts (NDPs), filtered the corresponding refuters and non-refuters, and obtained 74,987 follower-followee relationships between these refuters and non-refuters. Third, we analyzed the differences in the volume and growth rates between the DPs and NDPs for each type of fake news by comparing them in terms of the number and cumulative probability distribution of the posts. Fourth, for each type of fake news, we constructed SRNs involving refuters and non-refuters and the
The contributions of this study are significant both in theory and practice. First, unlike previous research that focused on the sharing of fake news [6, 18], in this study, by using deep learning method and social network analysis method, we focus on the comparison of differences between the debunking and non-debunking of fake news across various topics and systematically construct, compare, and analyze the SRNs of refuters or non-refuters to provide strategies for combating various categories of fake news on Chinese social media at the macro level. Thus, we shift the scholarly focus from the dominant area of fake news information sharing to the emerging area of employing users and the social relationships among them to combat various types of fake news on Chinese social media platforms. Second, on a practical level, the “fake news” literature in China is expanded by focusing on various types of day-to-day online rumors on social media available from a large volume of fake news datasets. This study provides insight to practitioners such as social media managers, government staff, news authorities, and media staff on ways to debunk different types of fake news using targeted and personalized governance strategies.
The remainder of this paper is structured as follows. Related Work introduces work related to this study. Materials and Methods describes the data and methods. Results details the results of the experiments. Discussion presents a discussion on this study, the limitations of this study, and a scope for future work. Conclusion provides the conclusions of the study.
Related Work
Before introducing the study problem, we provide brief remarks on the terminology used. Researchers have provided different interpretations of the definitions and connotations of fake news, misinformation, and rumors; these terms are often used interchangeably in academic research [19]. First, we disregarded the politicized nature that the term “fake news” has indicated, especially since the 2016 U.S. presidential elections. Second, we adopted a broader definition of news on social media: it includes any information (e.g., text, emoticons, and links) posted on social media [12]. Third, we did not consider the intentions behind those who posted the online information [2] and the differences between automated social robots and humans [12]. For these reasons and owing to its useful scientific meaning and construction, we retained the term “fake news,” which is used by most researchers, to represent our research objective [1, 8]; this term refers to false news or false rumors stemming from authorities’ (e.g., government agencies, state media, and other authoritative organizations) statements that were determined to be false.
Social media platforms employ different approaches to combat online fake news. The first key strategy is to undermine economic incentives and shift focus to developing technical solutions to help users make more informed decisions [20], for example, by showing warning messages and relying on fact-checking units [2, 8, 17, 21–24]. In this context, Hoaxy is a platform used for the collection, detection, analysis, and fact-checking of fraudulent online content from various viewpoints [22]. It was tested by collecting approximately 1,442,295 tweets and articles from 249,659 different users [16]. Content from news websites and social media was fed into a database that was updated on a regular basis, and it was analyzed to extract different hidden patterns [22]. Pennycook et al. selected fake news headlines from Snopes.com, a third-party website that fact-checks news stories, to investigate whether warning tags would effectively reduce belief in fake news by a prominent intervention that involved attaching warnings to the headlines of news stories that have been disputed by third-party fact-checkers [24]. Although researchers and online fact-checking organizations are continuously improving their fact-checking measures against the spread of fake news, most fact-checking processes depend on human labor, which requires considerable time and money [25, 26]. Despite recent advancements in automatic detection, identification models for fact checking lack the required adaptive and systematic applications [2]. Table 1 lists popular fact-checking analysis tools that are used to check the authenticity of online content.
Social network analysis has become a widely accepted tool [27]. Thus, the second key strategy is broadcasting denials to the public to prevent the exposure of individuals to fake news on social media [8], which can reduce the possibility of fake news spreading [28]. Fake news often spreads over social media through interpersonal communication [23], and personal involvement remains a salient construct of the spread of fake news on social media [29]. Thus, the key approach to combat the spread of fake news is to use the power of social relationships on social networks to spread messages from one individual to another [30]. Some researchers preferred to examine interventions on social networks that might be effective in debunking fake news by using source detection, i.e., finding out the person or location from whom or where the false information in the social network or web started spreading [16, 31–35] (see Table 1). For example, Shelke and Attar provided a state-of-the-art survey of different source detection methodologies along with different available datasets and experimental setups in case of existing single and multiple misinformation sources [35]. Whereas other researchers preferred to investigate the propagation dynamics of fake news [16]. As shown in Table 1, the prominent methods of fake news diffusion models available in the literature can be classified into three major categories: soft computing [36], epidemiological [37, 38], and mathematical approaches [39–41]. It is hard to execute these approaches because most of these studies are based on complex mathematical, physical, and epidemiological models; furthermore, in the real world, users may go beyond just controlling the simulation settings in the diffusion models [17].
The third key strategy is fake news detection. Different artificial intelligence algorithms along with cognitive psychology and mathematical models are used to identify false content [16]. As the assessment of the veracity of a news story is complex from an engineering point of view, the research community is approaching this task from different perspectives; Table 1 presents some prominent research on datasets, experimental settings, training, validation, and testing methods used in various machine learning and deep learning technologies, and other methods to address the issue [16, 42] (see Table 1).
As presented in Table 1, the first research aspect is the study of datasets and experimental settings. Different formats of datasets are used for content and behavioral analyses such as text tweets, images, headlines, news articles, URLs, users’ comments, suggestions, and discussions on particular events [16]. Most researchers used Twitter, Sina Weibo, and Facebook’s Application Programming Interface (API) for collecting and analyzing rumors and fake news as data sources [12, 43, 44], whereas other researchers preferred using a data repository such as FakeNewsNet that contains two comprehensive datasets PolitiFact and GossipCop to facilitate research in the field of fake news analysis [45]. These datasets collect multi-dimensional information from news content and social contexts and spatiotemporal data from diverse news domains [16]. In addition, some researchers compared the details of some of the widely used datasets and experimental setups in detail [46].
The second research aspect is the study of handcrafted feature extraction. Machine and deep learning are prominent techniques for designing models for detecting false information. The effectiveness of these algorithms mainly depends on pattern analysis and feature extraction of text [12, 47, 48], images [47, 49], users [50] messages [50, 51], propagation [52], structural [48], temporal [53], and linguistic specific features [16, 54] (see Table 1).
The third research aspect is the study of network structures. Network structures are innovative methods of assessing the credibility of a target article [55]. For example, Ishida and Kuraya proposed a bottom-up approach with relative, mutual, and dynamic credibility evaluation using a dynamic relational network (or mutual evaluation model) of related news articles, wherin each node can evaluate and in turn be evaluated by other nodes for credibility based on the consistency of the content of the node [56]. In addition, to model network structures and the user connectivity of online social networks, scalable synthetic graph generators were used by researchers. These generators provided a wide variety of generative graph models that could be used by researchers to generate graphs based on the extraction of different features such as propagation, temporal, connectivity, follower-followee relationship [16]. For example, Edunov et al. proposed Darwini, a graph generator that captures several core characteristics of real graphs and can be used efficiently to study the propagation and detection of false content by generating different social connections in the form of a graph [57]. To accomplish this, Darwini produces local clustering coefficients, degree distributions, node page ranks, eigenvalues, and many other matrices [57].
The fourth research aspect is the study of machine learning and deep learning classifiers. Some scholars have investigated real-time data in social media sites using stance detection methods to identify people who are supportive, neutral, or opposed to fake news [58]. These methods are widely based on the computation of machine or deep learning algorithms to achieve open or target-specific classification [59–61]. For example, Pérez-Rosas et al. focused on the linguistic differences between fake news and legitimate news content by using machine learning techniques, including a linear support vector machine (SVM) classifier, and obtained 78% accuracy in detecting fake news on two novel datasets [62]. The major disadvantage of machine-learning-based models is that they are dependent on hand-crafted features that require exhaustive, meticulous, detailed, and biased human efforts; thus, recent technologies are shifting the trend towards deep learning-based models [16]. For example, Zarrella and Marsh applied a recurrent neural network (RNN) initialized with features learned via distant supervision to tackle the SemEval-2016 task 6 (Detecting Stance in Tweets, Subtask A: Supervised Frameworks) [63]. In parallel, they trained embeddings of words and phrases with the word2vec Skip-Gram method. This effort achieved the top score among 19 systems with an F1 score of 67.8%, and one of 71.1% in a non-official test. Poddar et al. developed a novel neural architecture for detecting the veracity of a rumor using the stances of people engaging in a conversation about it on Twitter [64]. Taking into consideration the conversation tree structure, the proposed CT-Stance model (stance predictor model) achieved the best performance with an accuracy of 79.86% when considering all three realistically available signals (target a tweet, conversation sequence, time). In addition, there are other fake news detection methods, such as the use of cognitive psychology to analyze human perceptions. For example, Kumar and Geethakumari explored the use of cognitive psychology concepts to evaluate the spread of misinformation, disinformation, and propaganda in online social networks by examining four main ingredients: the coherency of the message, credibility of the source, consistency of the message, and general acceptability of the message; they used the collaborative filtering property of social networks to detect any existing misinformation, disinformation, and propaganda [65].
Based on the analysis of the aforementioned related work, some previous studies on fake news debunking are summarized in Table 1. First, the differences between different types of debunked and non-debunked fake news based on real data on Chinese social media have not been comprehensively assessed; second, the ways in which users’ social relationships on social networks influence the spread of debunking messages have also not been fully investigated. Unlike previous studies, we used a deep learning method to build text classifiers for identifying debunked posts (DPs) and non-debunked posts (NDPs), and filtered out refuters and non-refuters. Then based on social analysis method, we focused on the empirical analysis of real data on Chinese social media platforms by comparing the characteristics of the SRNs of refuters and non-refuters, and we tried to discover useful strategies for debunking different categories of fake news effectively.
Materials and Methods
Study Context and Data Collection
Sina Weibo, often referred to as “Chinese Twitter,” is one of the most influential social network platforms in China [68]. Twitter is a widely used microblogging platform worldwide, and its content is mainly written in English, while Sina Weibo is the most famous microblogging platform in China, where all posts are written in Chinese [69]. Social media are known as interactive computer-mediated technologies that allow people to create, share or exchange information [69]. The increasing popularity of social media websites and Web 2.0 has led to an exponential growth of user-generated content, especially text content on the Internet [69], which generates a large amount of unstructured text data and provides data support for our research. Thus, to avoid selection bias in the data, our data were collected from Zhiwei Data Sharing Platform’s (hereinafter referred to as Zhiwei Data) database that contains fake news of public events posted on Sina Weibo from July 2015 to September 2019, among which all the fake news events have been verified as “false.” We then obtained 176 widely spread fake news events, for a total of 49,278 posts. We also cooperated with Zhiwei Data to obtain user profiles through the business API of Sina Weibo, including the demographic characteristics of the users, such as their names, genders, types of accounts, locations, the number of their posts, followees, and followers, the source of their posts, and their posting times.
As publicly available data were used in this study, we only referred to the summarized results and did not derive any sensitive data. Information on the individuals studied in this research has not been published elsewhere.
Methodology
Identification of Debunked Fake News Posts
Fake news contains partly true, false, and mixed information; thus, researchers need to know what kind of opinions are expressed by people communicating on social media; this can be determined through stance detection [58]. A stance is a person’s opinion on or attitude toward some target entity, idea, or event determined from a posting, e.g., “in favor of,” “neutral,” “against,” [70], “support,” “deny,” “comment,” or “query” [71]. According to the content of 176 fake news events, we considered both subjective expressions and their corresponding targets, which might not be explicitly mentioned, and labeled each stance with respect to a specific target of each event; each stance was divided into two relative standpoints, “against” and “other,” corresponding to DPs from refuters and NDPs from non-refuters, respectively. Additionally, a text classifier was developed to detect DPs, which indicated that people who made these posts were against fake news. Although we acknowledge that binary labeling has certain limitations, for our current research, we highlighted the main research objectives as well as the overwhelming advantages of this straightforward and simple classification when compared to its weaknesses [72].
Stance detection is an area with closely related subjects [58]. Thus, it is modeled as a supervised learning process to achieve better results when a training dataset is required. Therefore, to obtain a fully available dataset, we asked three members of our team to label posts using an “against” or “other” stance. Two members with a detailed understanding of fake news labeled 10,000 posts, which were randomly selected from 49,278 posts. Furthermore, we tackled this labeling analysis based on the conversations stemming from direct and nested replies to the posts originating from fake news [71]. Next, these two members discussed all the annotation results and reannotated the posts to agree on the differences. Finally, a third member randomly selected 1,000 posts from 10,000 posts for an annotation to calculate the intercoder reliability. In addition, Cohen’s kappa is popular descriptive statistics for summarizing the cross-classification of two nominal variables with
According to the external references in a stop word dictionary, we used Python’s (Version 3.6.2) regular expressions to clean the sample data (including the compile and sub methods in Python’s RE package), including removing the relevant stop words (based on the list of stop words produced by the Harbin Institute of Technology), URLs, and punctuation and correcting any misspelt words. Then, we created our user dictionary, which includes some terms related to the 176 fake news events, and we used the Jieba Chinese text segmentation module in Python, one of the most widely used word segmentation tools for the Chinese, to segment the sample data. We then used word2vec from Google for word embedding [76]. The vector representations of words learned by word2vec can extract the deep semantic relationships between words, contributing more to text classification [77].
A long short-term memory (LSTM [78]) network was developed on the basis of recurrent neural networks (RNNs), which are capable of processing serialized information through their recurrent structures, to solve problems related to gradient vanishing or exploding [79]. LSTM does show a remarkable ability in processing natural language. Particularly, on Chinese social media posts where the words have complex context-dependent relationships, LSTM models perform well in applications with text classification tasks [79, 80]. Therefore, a deep neural network with an LSTM algorithm was employed to build the text classifier. In the LSTM model training process, the hyperparameter is a parameter that sets the value before the model is trained. Generally, the hyperparameters need to be optimized, and a set of optimal hyperparameters is selected for the model to improve the quality of the learning [81]. To prevent the overfitting problem of the model in the training process, we selected some appropriate combinations of parameters to train the model, and the hyperparameter configuration of the model was as follows: batch size = 512; maximum length of the sentence = 70; dropout = 0.5; activation = sigmoid; loss function = binary cross-entropy.
Then, we tested the vector dimensions of 50, 100, 150, … , 450, and 500 to obtain the best word vector dimension. We chose accuracy and F1 score as metrics to measure the performance of the classifiers [82]. A 10-fold cross-validation technique was also adopted to train our classifier and evaluate the performance of our classification algorithm. The experimental results of the classification are presented in Table 2. Compared with other dimensions, the experimental results had a relatively high accuracy of 90.98% and an F1 score of 92.05% with a word vector dimension of 350. Thus, we used a word vector dimension of 350 to build our classification. Finally, we obtained 25,856 DPs from 6,114 refuters and 23,422 NDPs from 8,285 non-refuters using our classifier. A flowchart for identifying debunked fake news posts is shown in Figure 1 [see procedures (1–5) in Figure 1].
Qualitative Classification of Account Types and Fake News Topics
First, to analyze and examine how various accounts (refuters and non-refuters) were involved in DPs and NDPs, we referred to account classification standards based on the Sina Weibo certification on their homepages, which includes ten types of accounts: ordinary, media, government, celebrity, Weibo’s got talent, enterprise, campus, organization, website, and Weibo girl.
Second, we referenced the fake news classification standards of Twitter [12] and previous studies regarding the rumor classification standards of Sina Weibo in China [83]. We divided the fake news into six categories using the Zhiwei Data’s classification criteria: society, health, business, science and technology (hereinafter referred to as science), disaster, and politics and finance (hereinafter referred to as politics) (Table 3). We also asked three annotators from Zhiwei Data to label 176 events according to these six categories. Additionally, the Cohen’s kappa (
For each type of fake news, we used the number of posts to indicate the spreading volumes of the DPs and NDPs. We also sorted each event in ascending order according to the time of the post and calculated the relative hours of any post relative to the first post in each news event. For the six types of fake news, we aggregated all their corresponding events. Here, the epoch
where
Social Relationship Network Construction and Analysis
On Sina Weibo, users (followers) may choose to follow any other users (followees); thus, they can automatically receive all the posts published by their followees, such as on Twitter [85]. Here, an SRN with weak ties is formed; users can easily follow many people without talking to them directly. Thus, we filtered out all the follower-followee relationships (hereinafter referred to as following relationships) of 6,114 refuters and 8,285 non-refuters involved in spreading fake news. We also filtered the source nodes (followers) and target nodes (followees) in the following relationship of users involved in fake news propagation and finally obtained 74,987 following relationships.
A set of following relationships and the set of Sina Weibo users connected by these relationships formed an SRN [85]. Relationships between people can be captured as graphs where vertices represent entities and edges represent connections among them [86]. A
We computed the following set of basic network properties, which allowed us to encode each network according to a tuple of features: (1) the average degree (
Note that our analysis is based on the fact that fake news propagation has already ended; we did not construct a reposting network. Some of the network indicators (1–4) in the aforementioned network analysis are only descriptions and portrayals of the basic properties of the network and cannot be used to explain the reasons for the differences in the propagation of DPs and NDPs. Therefore, to compare SRNs more effectively, we considered defining measurement indices based on the following three aspects. First, we considered that some accounts exhibited different characteristics in the spread of fake news [18] and that there might be some individual differences, that is, different types of nodes might play different roles in the spread. Second, in interpersonal communication, fake news tends to initially flow to neighbors, friends, and colleagues of the spreaders and then circulate in certain regions and groups; thus, we used the size of the nodes with social relationships to symbolize the magnitude of this spreading effect. Furthermore, if a shortcut existed between two local cliques, was more conducive to the spread of information, and the number of weakly connected components on social networks indicated the magnitude of this influence. Therefore, we defined the following three measure indices:
(1) Proportion of account types (
To avoid differences in the sizes of the SRNs of different types of fake news, we considered the relative index of the proportion of account types participating in DPs and NDPs as follows:
where
(2) Network size of the
To avoid the differences in the scales of the SRNs of different types of fake news, we considered analyzing the ratio of the number of nodes in the SRNs before and after
where
(3) Number of weakly connected components (
A weakly connected component of a directed graph is a maximal (sub)graph, where there exists a path u
Additionally, we used the open-source software Gephi 0.9.2 to visualize networks and calculate the network properties [90, 91]. In the network, nodes were colored according to ten types of accounts, and the sizes of the nodes corresponded to their total degree (including the in-degree and out-degree), that is, the number of followees and followers of this account, which is a measure of the influence of an account. The Fruchterman-Reingold layout algorithm was used to calculate the graph layout and draw a graph by force-directed placement [92].
Statistical Analyses
Pearson chi-square (
Research Procedures
The process followed in this study is illustrated in Figure 1. First, we collected 49,278 posts of 176 fake news events on Sina Weibo from July 2015 to September 2019. Second, we used the LSTM algorithm to build a stance classifier, which divided posts into DPs and NDPs and filtered out refuters and non-refuters for further analysis. Third, we divided the account types into ten categories and fake news into six topics. Fourth, for each type of fake news, we obtained 74,987 following relationships of refuters and non-refuters and constructed SRNs and their
Results
Development of Debunked and Non-Debunked Posts Over Time
Distribution of Debunked and Non-Debunked Posts
To achieve a better comparison between DPs and NDPs, we used graphs for clearer data representation. The quarterly numbers of all the DPs and NDPs diffused on Sina Weibo from July 2015 to September 2019 are shown in Figure 2A. Owing to the scarcity and imbalance of the dataset at certain periods and for better comparison with the research on Twitter [12], we used quarterly data for the representation in Figure 2A. Note that the spread of fake news has increased yearly, particularly in 2018 and 2019. Similarly, the spread of DPs has gradually but significantly increased, especially in the third and fourth quarters of 2018; furthermore, the number of DPs is much higher than that of the NDPs, and the gap between the DPs and NDPs is gradually increasing (
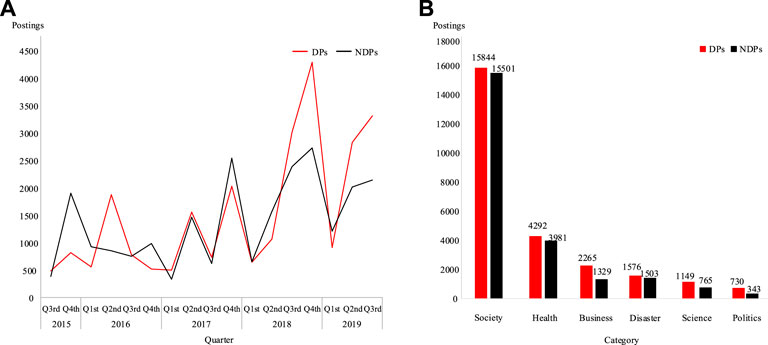
FIGURE 2. Descriptive analysis of DPs and NDPs: (A) quarterly DPs and NDPs and (B) the number of DPs and NDPs under the six fake news topics. Notes: Science represents science and technology and Politics denotes politics and finance.
The total number of DPs and NDPs for various types of fake news are shown in Figure 2B. The result of the Pearson chi-square test was
Spreading Growth Rates of Debunked and Non-Debunked Posts
For the spread of fake news, early debunking is important to minimize harmful effects. Therefore, we considered further examining the differences in the propagation growth rates of DPs and NDPs. Using Eq. 1, we calculated the
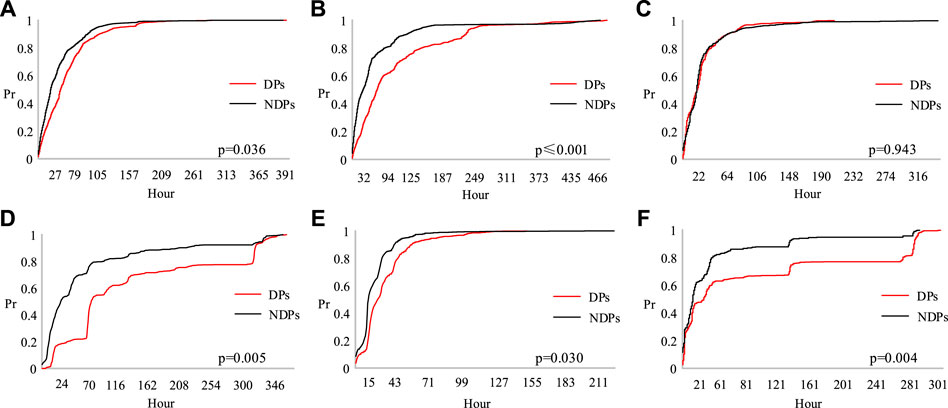
FIGURE 3. Growth rate of debunked posts (DPs) and non-debunked posts (NDPs) in six categories of fake news over time: (A) Society, (B) Health, (C) Business, (D) Science, (E) Disaster, and (F) Politics. Notes: Science represents science and technology and Politics represents politics and finance.
Figure 3 shows that for all types of fake news, in the first 1/3 propagation stage, the NDPs and DPs spread rapidly; however, the details of the spread patterns are different. Except for business-related fake news (as shown in Figure 3C, the
Social Relationship Networks of Refuter–Refuter and Non-Refuter–Non-Refuter
Distribution of Account Types
To investigate the different roles played by the various types of accounts in the growth rate of DPs and NDPs, we first examined the proportion of accounts in all types of fake news; the results are shown in Figure 4. For DPs and NDPs, the Pearson chi-square tests indicated that the results had a very high degree of statistical significance in the account distribution under different types of fake news [Figure 4A:
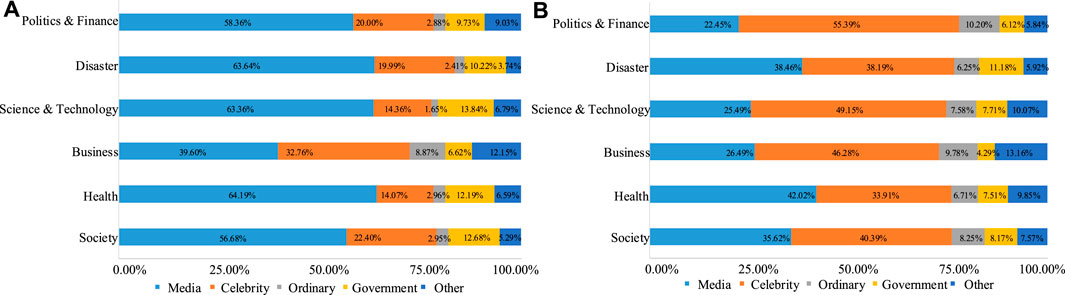
FIGURE 4. Proportion of account types in (A) debunked posts (DPs) and (B) non-debunked posts (NDPs) under six types of fake news. Notes: Other types of accounts include Weibo got talent, enterprise, campus, organization, website, and Weibo girl accounts.
Celebrity and media accounts played an important role in the spreading growth rates of DPs and NDPs. Therefore, combining the “80/20” distributions [95], we mainly focused on the role played by media and celebrity accounts in the propagation of DPs and NDPs, referring to Eq. 2. The results are shown in Table 4. We found that, for business-related fake news that achieved a better debunking effect on the spread of DPs, the relative proportion of the celebrity accounts in DPs to those in NDPs was the highest (
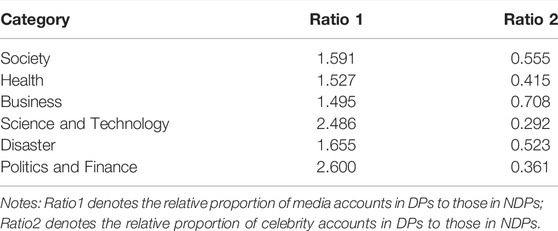
TABLE 4. Relative proportion of account types in DPs and those in NDPs under six types of fake news.
Comparison of Differences in Networks of Refuters and Non-Refuters
To analyze the factors affecting the growth rate of DPs and NDPs for the six types of fake news, we constructed SRNs of refuters and non-refuters and the corresponding
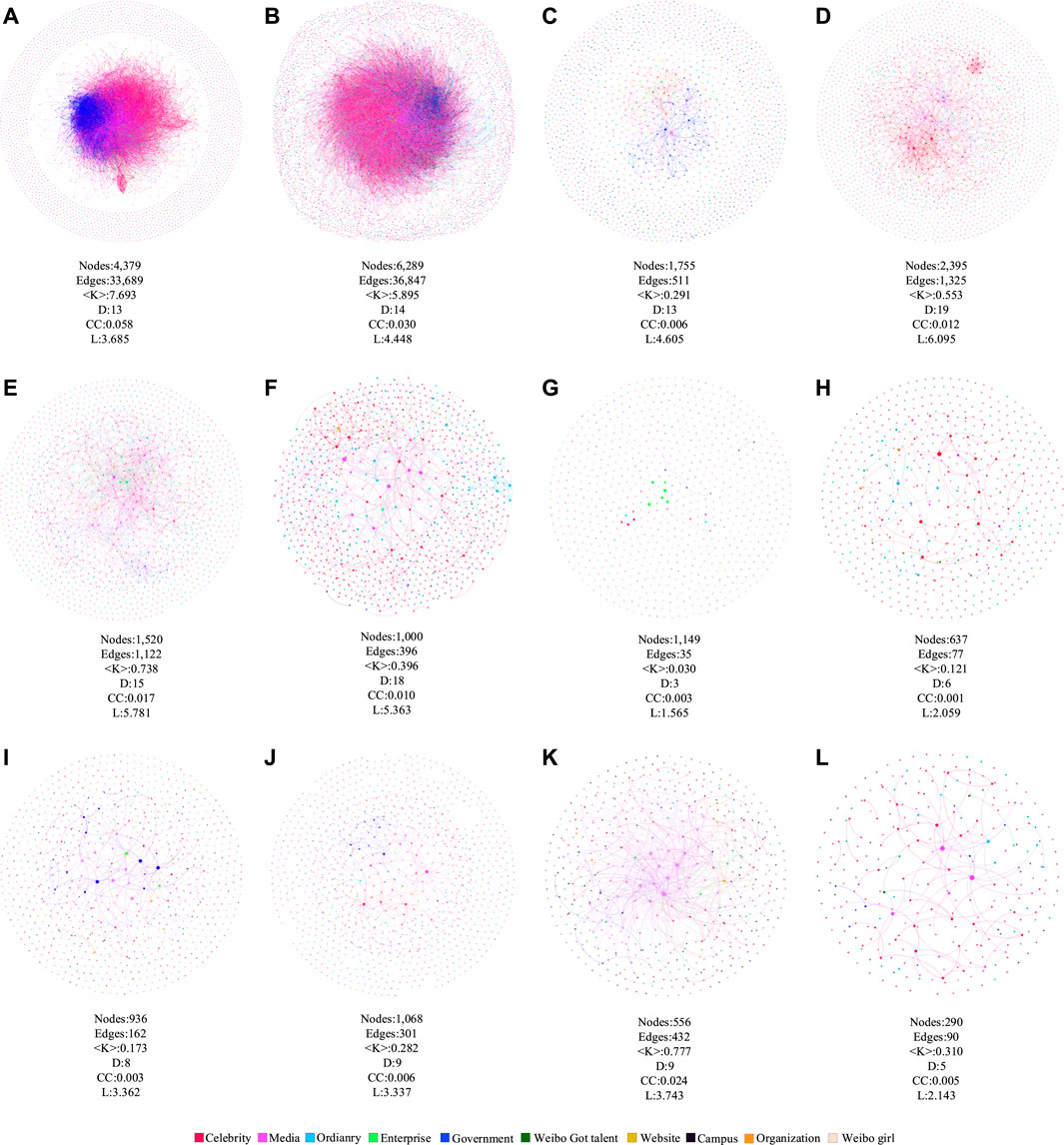
FIGURE 5. SRNs under six types of fake news: (A) of refuters (R–R) in society-related fake news, (B) of non-refuters (NR–NR) in society-related fake news, (C) R–R in health-related fake news, (D) NR–NR in health-related fake news, (E) R–R in business-related fake news, (F) NR–NR in business-related fake news, (G) R–R in science-related fake news, (H) NR–NR in science-related fake news, (I) R–R in disaster-related fake news, (J) NR–NR in disaster-related fake news, (K) R–R in politics-related fake news, and (L) NR–NR in politics-related fake news.
As shown in Figure 5, the fake news types with better debunking effects (business) and those with worse debunking effects (society, health, disaster, politics, and science) do not show significant differences in the properties of
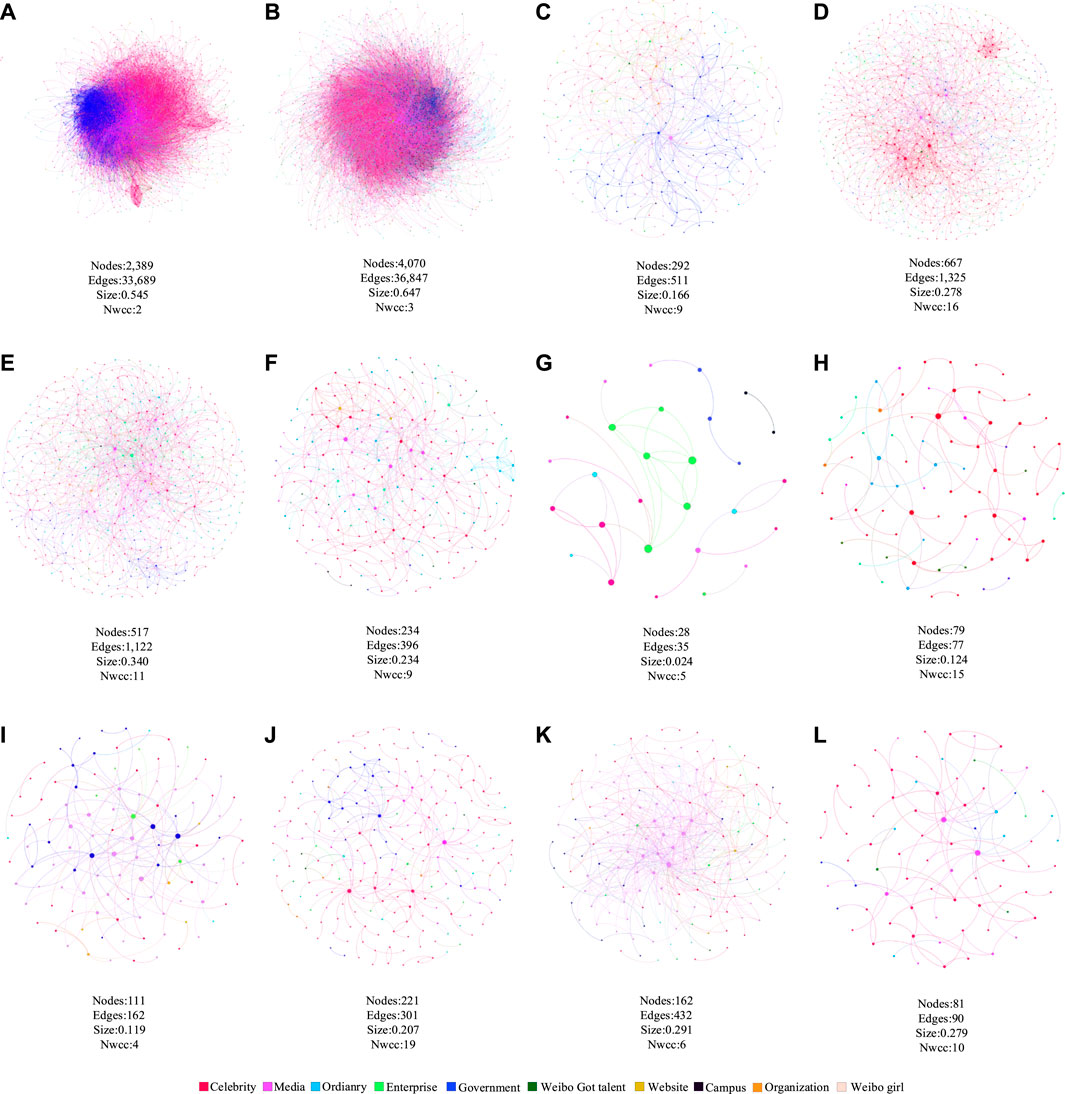
FIGURE 6.
First, to analyze the impact of the size of nodes with following relationships in the SRN on the spread of information, the network size of the
Second, we also found that the number of weakly connected components in the
Discussion
Our results are intended to highlight the differences in the growth rates between DPs and NDPs under different fake news topics from the perspective of SRNs on social media in China, as well as to deeply explore the reasons for the differences, and provide the following four key insights.
First, as shown in Figure 2, our results indicated that the spread of fake news is increasing yearly, similar to the findings reported by Vosoughi et al. [12]. We found that in Chinese social media, people have unique preferences for fake news on different topics. To be specific, our results showed that society- and health-related fake news were highly important to Chinese society [4], society-related fake news with 15,844 DPs and 15,501 NDPs, followed by health with 4,292 DPs and 3,981 NDPs, which differed from the distribution on Twitter (corresponding to politics, urban legends, business, terrorism, and war, respectively [12]). Thus, on the one hand, our results confirmed that the information-spreading patterns were different between categories of fake news events [96] and that such a pattern was determined by the event attributes [84]. On the other hand, our results confirmed that the differences in their spread in different countries can be attributed to cultural differences, the news media environment, and other environmental factors [97]. Furthermore, as shown in Figure 3, our results also showed that the debunking effect of business-related fake news was better than that of the other types of fake news. This finding is not consistent with those in previous research on fake news on Twitter, which found that falsehood diffused significantly farther, faster, deeper, and more broadly than the truth in all categories of information, and the effects were more pronounced for false political news than for false news about terrorism, natural disasters, science, urban legends, or financial information [12]. In contrast, in our findings, business news had a high
Second, for fake news on different topics, the results in Table 4 showed that regardless of whether the posts were DPs or NDPs, celebrity accounts (
Third, as shown in Figure 6, for fake news on different topics, our results showed that messages were more likely to spread in networks with following relationships, that is, the larger the size of the nodes (
However, we acknowledge that our research has certain limitations, which give way to promising topics for further research. First, we only analyzed posts on Sina Weibo, which has limitations in terms of data size and multi-platform sources. Thus, we will consider adding the data of other social media platforms, such as those of Baidu Tieba and WeChat. Second, most of our research results are the experimental results of a comparative analysis of users’ SRNs. Therefore, in future research, we will consider using simulation methods to enhance the robustness of our research results, such as applying some physical models for further analysis [109]. We will also consider the internal mechanism of the time delays of DPs among different categories of fake news and analyze the reasons why people opt for refuting or accepting the DPs. Thirdly, to understand the large-scale fake news on Chinese social media platforms, although we focused on the comparison of differences between the debunking and non-debunking of fake news across various topics and obtained some general and reliable findings based on a large-scale multi-category fake news dataset, some of the findings in our results were validation of some intuitive results or previous studies. In the future, by investigating different types of fake news on Chinese social media platforms, we hope to find more interesting and surprising results based on social relationship networks. For example, previous research indicated that structural positions stratified by such variables as gender, age, ethnicity, paid employment, educational attainment, income, and family responsibilities may shape the formation of social relationships and one’s ability to gain valuable information [110]. Therefore, in the future, we hope to do more insightful investigations on how the attributes of social relationship networks might affect the spread of debunking information. Finally, to highlight the research objective, we detected only two stances. In a future study, we will consider a more detailed refutation method based on multiple classifications using deep learning algorithms, not only by evaluating the true and false labels of fake news but also by combining concepts such as sociology, psychology, and communication theory to focus on controversy detection, degree of divergence, and group polarization. We will also consider using fake news data on the COVID-19 epidemic for an in-depth study.
Conclusion
The growth in social networking has made social media platforms such as Sina Weibo a breeding ground for fake news. Based on the six types of fake news spread on Sina Weibo, first, in this study, we investigated the differences in the volume and growth rates of posts between DPs and NDPs by comparing them in terms of their number and cumulative probability distribution. Second, we used three indices to explore the network characteristics of the following relationships of refuters and non-refuters, namely the proportion of account types, the network size of
Data Availability Statement
The raw data supporting the conclusion of this article will be made available by the authors, further inquiries can be directed to the corresponding author.
Author Contributions
XW: Conceptualization, Methodology, Software, Visualization, Formal analysis, Data Curation, Writing. FC: Methodology, Formal analysis. NM: Methodology. GY: Conceptualization, Writing, Funding acquisition.
Funding
This research was funded by the National Natural Science Foundation of China (Grant No. 72074060).
Conflict of Interest
The authors declare that the research was conducted in the absence of any commercial or financial relationships that could be construed as a potential conflict of interest.
Publisher’s Note
All claims expressed in this article are solely those of the authors and do not necessarily represent those of their affiliated organizations, or those of the publisher, the editors and the reviewers. Any product that may be evaluated in this article, or claim that may be made by its manufacturer, is not guaranteed or endorsed by the publisher.
Acknowledgments
The authors would like to acknowledge the editor’s contribution and show appreciation to the reviewers for their helpful comments and recommendations. The authors would like to acknowledge all the authors cited in the references. The authors would like to thank Zhiwei Data Sharing Platform (http://university.zhiweidata.com/) for our data support.
References
1. Grinberg N, Joseph K, Friedland L, Swire-Thompson B, Lazer D. Fake News on Twitter during the 2016 U.S. Presidential Election. Science (2019) 363(6425):374–8. doi:10.1126/science.aau2706
2. Zhang X, Ghorbani AA. An Overview of Online Fake News: Characterization, Detection, and Discussion. Inf Process Management (2020) 57(2):102025. doi:10.1016/j.ipm.2019.03.004
3. Cheng Y, Lee C-J. Online Crisis Communication in a post-truth Chinese Society: Evidence from Interdisciplinary Literature. Public Relations Rev (2019) 45(4):101826. doi:10.1016/j.pubrev.2019.101826
4. Guo L. China's "Fake News" Problem: Exploring the Spread of Online Rumors in the Government-Controlled News Media. Digital Journalism (2020) 8(8):992–1010. doi:10.1080/21670811.2020.1766986
5. Chen X, Wang N. Rumor Spreading Model Considering Rumor Credibility, Correlation and Crowd Classification Based on Personality. Sci Rep (2020) 10(1):5887–15. doi:10.1038/s41598-020-62585-9
6. Del Vicario M, Bessi A, Zollo F, Petroni F, Scala A, Caldarelli G, et al. The Spreading of Misinformation Online. Proc Natl Acad Sci USA (2016) 113(3):554–9. doi:10.1073/pnas.1517441113
7. Wang D, Qian Y. Echo Chamber Effect in the Discussions of Rumor Rebuttal about COVID-19 in China: Existence and Impact. J Med Internet Res (2021).
8. Lazer DMJ, Baum MA, Benkler Y, Berinsky AJ, Greenhill KM, Menczer F, et al. The Science of Fake News. Science (2018) 359(6380):1094–6. doi:10.1126/science.aao2998
9. Wu Y, Deng M, Wen X, Wang M, Xiong X. Statistical Analysis of Dispelling Rumors on Sina Weibo. Complexity (2020) 2020. doi:10.1155/2020/3176593
10. Gradoń KT, Hołyst JA, Moy WR, Sienkiewicz J, Suchecki K. Countering Misinformation: A Multidisciplinary Approach. Big Data Soc (2021) 8(1):20539517211013848.
11. Liu Y, Jin X, Shen H, Bao P, Cheng X. A Survey on Rumor Identification over Social media. Chin J Comput (2018) 40(1):1–23.
12. Vosoughi S, Roy D, Aral S. The Spread of True and False News Online. Science (2018) 359(6380):1146–51. doi:10.1126/science.aap9559
13. Xu K, Zheng X, Cai Y, Min H, Gao Z, Zhu B, et al. Improving User Recommendation by Extracting Social Topics and Interest Topics of Users in Uni-Directional Social Networks. Knowledge-Based Syst (2018) 140:120–33. doi:10.1016/j.knosys.2017.10.031
14.Ahn Y-Y, Han S, Kwak H, Moon S, and Jeong H, editors. Analysis of Topological Characteristics of Huge Online Social Networking Services. Proceedings of the 16th international conference on World Wide Web (2007).
15. Pierri F, Piccardi C, Ceri S. Topology Comparison of Twitter Diffusion Networks Effectively Reveals Misleading Information. Sci Rep (2020) 10(1):1372–9. doi:10.1038/s41598-020-58166-5
16. Meel P, Vishwakarma DK. Fake News, Rumor, Information Pollution in Social media and Web: A Contemporary Survey of State-Of-The-Arts, Challenges and Opportunities. Expert Syst Appl (2020) 153:112986. doi:10.1016/j.eswa.2019.112986
17. Pal A, Chua AYK, Hoe-Lian Goh D. Debunking Rumors on Social media: The Use of Denials. Comput Hum Behav (2019) 96:110–22. doi:10.1016/j.chb.2019.02.022
18. Oehmichen A, Hua K, Amador Diaz Lopez J, Molina-Solana M, Gomez-Romero J, Guo Y-k. Not All Lies Are Equal. A Study into the Engineering of Political Misinformation in the 2016 US Presidential Election. IEEE Access (2019) 7:126305–14. doi:10.1109/access.2019.2938389
19. Wang Q, Yang X, Xi W. Effects of Group Arguments on Rumor Belief and Transmission in Online Communities: An Information cascade and Group Polarization Perspective. Inf Management (2018) 55(4):441–9. doi:10.1016/j.im.2017.10.004
20. Jung A-K, Ross B, Stieglitz S. Caution: Rumors Ahead—A Case Study on the Debunking of False Information on Twitter. Big Data Soc (2020) 7(2):2053951720980127. doi:10.1177/2053951720980127
21.AlRubaian M, Al-Qurishi M, Al-Rakhami M, Hassan MM, and Alamri A, editors. CredFinder: A Real-Time Tweets Credibility Assessing System. 2016 IEEE/ACM International Conference on Advances in Social Networks Analysis and Mining (ASONAM. IEEE (2016).
22. Shao C, Hui PM, Wang L, Jiang X, Flammini A, Menczer F, et al. Anatomy of an Online Misinformation Network. PloS one (2018) 13:e0196087. doi:10.1371/journal.pone.0196087
23. J-w J, Lee E-J, Shin SY. What Debunking of Misinformation Does and Doesn't. Cyberpsychology, Behav Soc Networking (2019) 22(6):423–7.
24. Pennycook G, Bear A, Collins ET, Rand DG. The Implied Truth Effect: Attaching Warnings to a Subset of Fake News Headlines Increases Perceived Accuracy of Headlines without Warnings. Management Sci (2020) 66(11):4944–57. doi:10.1287/mnsc.2019.3478
25. Dale R. NLP in a post-truth World. Nat Lang Eng (2017) 23(2):319–24. doi:10.1017/s1351324917000018
26. Shu K, Sliva A, Wang S, Tang J, Liu H. Fake News Detection on Social Media. SIGKDD Explor Newsl (2017) 19(1):22–36. doi:10.1145/3137597.3137600
27. Stieglitz S, Mirbabaie M, Ross B, Neuberger C. Social media Analytics - Challenges in Topic Discovery, Data Collection, and Data Preparation. Int J Inf Manag (2018) 39:156–68. doi:10.1016/j.ijinfomgt.2017.12.002
28. Zhang N, Huang H, Su B, Zhao J, Zhang B. Dynamic 8-state ICSAR Rumor Propagation Model Considering Official Rumor Refutation. Physica A: Stat Mech Its Appl (2014) 415:333–46. doi:10.1016/j.physa.2014.07.023
29. Chua AYK, Banerjee S. Intentions to Trust and Share Online Health Rumors: An experiment with Medical Professionals. Comput Hum Behav (2018) 87:1–9. doi:10.1016/j.chb.2018.05.021
30. Tripathy RM, Bagchi A, Mehta S. Towards Combating Rumors in Social Networks: Models and Metrics. Ida (2013) 17(1):149–75. doi:10.3233/ida-120571
31. Zhu K, Ying L. Information Source Detection in the SIR Model: A Sample-Path-Based Approach. IEEE/ACM Trans Networking (2014) 24(1):408–21.
32.Liu Y, Gao C, She X, and Zhang Z, editors. A Bio-Inspired Method for Locating the Diffusion Source with Limited Observers. 2016 IEEE Congress on Evolutionary Computation (CEC). IEEE (2016).
33.Choi J, Moon S, Woo J, Son K, Shin J, and Yi Y, editors. Rumor Source Detection under Querying with Untruthful Answers. IEEE INFOCOM 2017-IEEE Conference on Computer Communications. IEEE (2017).
34. Louni A, Subbalakshmi KP. Who Spread that Rumor: Finding the Source of Information in Large Online Social Networks with Probabilistically Varying Internode Relationship Strengths. IEEE Trans Comput Soc Syst (2018) 5(2):335–43. doi:10.1109/tcss.2018.2801310
35. Shelke S, Attar V. Source Detection of Rumor in Social Network - A Review. Online Soc Networks Media (2019) 9:30–42. doi:10.1016/j.osnem.2018.12.001
36. Han S, Zhuang F, He Q, Shi Z, Ao X. Energy Model for Rumor Propagation on Social Networks. Physica A: Stat Mech its Appl (2014) 394:99–109. doi:10.1016/j.physa.2013.10.003
38. Wang X, Li Y, Li J, Liu Y, Qiu C. A Rumor Reversal Model of Online Health Information during the Covid-19 Epidemic. Inf Process Management (2021) 58(6):102731. doi:10.1016/j.ipm.2021.102731
39. Cheng J-J, Liu Y, Shen B, Yuan W-G. An Epidemic Model of Rumor Diffusion in Online Social Networks. The Eur Phys J B (2013) 86(1):1–7. doi:10.1140/epjb/e2012-30483-5
40. Tong G, Wu W, Du D-Z. Distributed Rumor Blocking with Multiple Positive Cascades. IEEE Trans Comput Soc Syst (2018) 5(2):468–80. doi:10.1109/tcss.2018.2818661
41. Pham DV, Nguyen GL, Nguyen TN, Pham CV, Nguyen AV. Multi-topic Misinformation Blocking with Budget Constraint on Online Social Networks. IEEE Access (2020) 8:78879–89. doi:10.1109/access.2020.2989140
42. Saquete E, Tomás D, Moreda P, Martínez-Barco P, Palomar M. Fighting post-truth Using Natural Language Processing: A Review and Open Challenges. Expert Syst Appl (2020) 141:112943. doi:10.1016/j.eswa.2019.112943
43. Zubiaga A, Aker A, Bontcheva K, Liakata M, Procter R. Detection and Resolution of Rumours in Social media: A Survey. ACM Comput Surv (Csur) (2018) 51(2):1–36.
44. Li Z, Zhang Q, Du X, Ma Y, Wang S. Social media Rumor Refutation Effectiveness: Evaluation, Modelling and Enhancement. Inf Process Management (2021) 58(1):102420. doi:10.1016/j.ipm.2020.102420
45. Shu K, Mahudeswaran D, Liu H. FakeNewsTracker: a Tool for Fake News Collection, Detection, and Visualization. Comput Math Organ Theor (2019) 25(1):60–71. doi:10.1007/s10588-018-09280-3
46.Al-Qurishi M, Al-Rakhami M, Alrubaian M, Alarifi A, Rahman SMM, and Alamri A, editors. Selecting the Best Open Source Tools for Collecting and Visualzing Social media Content. 2015 2nd world symposium on web applications and networking (WSWAN). IEEE (2015).
47. Yang Y, Zheng L, Zhang J, Cui Q, Li Z, Yu PS. TI-CNN: Convolutional Neural Networks for Fake News Detection. arXiv preprint arXiv (2018) 180600749.
48. Vicario MD, Quattrociocchi W, Scala A, Zollo F. Polarization and Fake News. ACM Trans Web (2019) 13(2):1–22. doi:10.1145/3316809
49. Jin Z, Cao J, Zhang Y, Zhou J, Tian Q. Novel Visual and Statistical Image Features for Microblogs News Verification. IEEE Trans multimedia (2016) 19(3):598–608.
50.Yang F, Liu Y, Yu X, and Yang M, editors. Automatic Detection of Rumor on Sina Weibo. Proceedings of the ACM SIGKDD workshop on mining data semantics (2012).
51.Lin D, Lv Y, and Cao D, editors. Rumor Diffusion Purpose Analysis from Social Attribute to Social Content. 2015 International Conference on Asian Language Processing (IALP). IEEE (2015).
52.Castillo C, Mendoza M, and Poblete B, editors. Information Credibility on Twitter. Proceedings of the 20th international conference on World wide web (2011).
53. Shu K, Mahudeswaran D, Wang S, Lee D, Liu H. Fakenewsnet: A Data Repository with News Content, Social Context, and Spatiotemporal Information for Studying Fake News on Social media. Big data (2020) 8(3):171–88. doi:10.1089/big.2020.0062
54. Vosoughi S, Mohsenvand MN, Roy D. Rumor Gauge. ACM Trans Knowl Discov Data (2017) 11(4):1–36. doi:10.1145/3070644
55. Zhou S, Ng ST, Lee SH, Xu FJ, Yang Y. A Domain Knowledge Incorporated Text Mining Approach for Capturing User Needs on BIM Applications. Eng Construction Architectural Management (2019).
56. Ishida Y, Kuraya S. Fake News and its Credibility Evaluation by Dynamic Relational Networks: A Bottom up Approach. Proced Computer Sci (2018) 126:2228–37. doi:10.1016/j.procs.2018.07.226
57. Edunov S, Logothetis D, Wang C, Ching A, Kabiljo M. Darwini: Generating Realistic Large-Scale Social Graphs. arXiv preprint arXiv:161000664 (2016).
58. Lillie AE, Middelboe ER. Fake News Detection Using Stance Classification: A Survey. arXiv preprint arXiv (2019) 190700181:907–16. doi:10.1007/978-3-319-50496–485
59. Xu R, Zhou Y, Wu D, Gui L, Du J, Xue Y. Overview of NLPCC Shared Task 4: Stance Detection in Chinese Microblogs. Nat Lang understanding Intell Appl Springer (2016). p. 907–16. doi:10.1007/978-3-319-50496-4_85
60. Aker A, Derczynski L, Bontcheva K. Simple Open Stance Classification for Rumour Analysis. arXiv preprint arXiv (2017) 170805286. doi:10.26615/978-954-452-049-6_005
61.Dungs S, Aker A, Fuhr N, and Bontcheva K, editors. Can Rumour Stance Alone Predict Veracity? Proceedings of the 27th International Conference on Computational Linguistics (2018).
62. Pérez-Rosas V, Kleinberg B, Lefevre A, Mihalcea R. Automatic Detection of Fake News. arXiv preprint arXiv (2017) 170807104.
63. Zarrella G, Marsh A. Mitre at Semeval-2016 Task 6: Transfer Learning for Stance Detection. arXiv preprint arXiv:160603784 (2016). doi:10.18653/v1/s16-1074
64.Poddar L, Hsu W, Lee ML, and Subramaniyam S, editors. Predicting Stances in Twitter Conversations for Detecting Veracity of Rumors: A Neural Approach. 2018 IEEE 30th International Conference on Tools with Artificial Intelligence (ICTAI. IEEE (2018).
65. Kumar KK, Geethakumari G. Detecting Misinformation in Online Social Networks Using Cognitive Psychology. Human-centric Comput Inf Sci (2014) 4(1):1–22. doi:10.1186/s13673-014-0014-x
66. Wang Z, Sui J. Multi-level Attention Residuals Neural Network for Multimodal Online Social Network Rumor Detection. FrPhy (2021) 9:466. doi:10.3389/fphy.2021.711221
67.Conforti C, Pilehvar MT, and Collier N, editors. Towards Automatic Fake News Detection: Cross-Level Stance Detection in News Articles. Proceedings of the First Workshop on Fact Extraction and VERification (FEVER) (2018).
68. Rodríguez CP, Carballido BV, Redondo-Sama G, Guo M, Ramis M, Flecha R. False News Around COVID-19 Circulated Less on Sina Weibo Than on Twitter. How to Overcome False Information? Int Multidisciplinary J Soc Sci (2020) 9(2):107–28.
69. Chen W, Lai KK, Cai Y. Exploring Public Mood toward Commodity Markets: A Comparative Study of User Behavior on Sina Weibo and Twitter. Intr (2020) 31(3):1102–19. doi:10.1108/intr-02-2020-0055
70. Mohammad SM, Sobhani P, Kiritchenko S. Stance and Sentiment in Tweets. ACM Trans Internet Technol (2017) 17(3):1–23. doi:10.1145/3003433
71. Derczynski L, Bontcheva K, Liakata M, Procter R, Hoi GWS, Zubiaga A. SemEval-2017 Task 8: RumourEval: Determining Rumour Veracity and Support for Rumours. arXiv preprint arXiv (2017) 170405972.
72. Yu Y, Duan W, Cao Q. The Impact of Social and Conventional media on Firm Equity Value: A Sentiment Analysis Approach. Decis support Syst (2013) 55(4):919–26. doi:10.1016/j.dss.2012.12.028
73. Warrens MJ. Weighted Kappa Is Higher Than Cohen's Kappa for Tridiagonal Agreement Tables. Stat Methodol (2011) 8(2):268–72. doi:10.1016/j.stamet.2010.09.004
74. Fleiss JL, Cohen J, Everitt BS. Large Sample Standard Errors of Kappa and Weighted Kappa. Psychol Bull (1969) 72(5):323–7. doi:10.1037/h0028106
75. Cohen J. A Coefficient of Agreement for Nominal Scales. Educ Psychol Meas (1960) 20(1):37–46. doi:10.1177/001316446002000104
76. Mikolov T, Chen K, Corrado G, Dean J. Efficient Estimation of Word Representations in Vector Space. arXiv preprint arXiv:13013781 (2013).
77. Zhang D, Xu H, Su Z, Xu Y. Chinese Comments Sentiment Classification Based on Word2vec and SVMperf. Expert Syst Appl (2015) 42(4):1857–63. doi:10.1016/j.eswa.2014.09.011
78. Hochreiter S, Schmidhuber J. Long Short-Term Memory. Neural Comput (1997) 9(8):1735–80. doi:10.1162/neco.1997.9.8.1735
79. Li Y, Wang X, Xu P. Chinese Text Classification Model Based on Deep Learning. Future Internet (2018) 10(11):113. doi:10.3390/fi10110113
80. Chang C, Masterson M. Using Word Order in Political Text Classification with Long Short-Term Memory Models. Polit Anal (2020) 28(3):395–411. doi:10.1017/pan.2019.46
81. Fei R, Yao Q, Zhu Y, Xu Q, Li A, Wu H, et al. Deep Learning Structure for Cross-Domain Sentiment Classification Based on Improved Cross Entropy and Weight. Scientific Programming (2020) 2020. doi:10.1155/2020/3810261
82. Yu B, Chen W, Zhong Q, Zhang H. Specular Highlight Detection Based on Color Distribution for Endoscopic Images. FrPhy (2021) 8:575. doi:10.3389/fphy.2020.616930
83. Lin D, Ma B, Jiang M, Xiong N, Lin K, Cao D. Social Network Rumor Diffusion Predication Based on Equal Responsibility Game Model. IEEE Access (2018) 7:4478–86.
84. Liu C, Zhan X-X, Zhang Z-K, Sun G-Q, Hui PM. How Events Determine Spreading Patterns: Information Transmission via Internal and External Influences on Social Networks. New J Phys (2015) 17(11):113045. doi:10.1088/1367-2630/17/11/113045
85. Huang R, Sun X. Weibo Network, Information Diffusion and Implications for Collective Action in China. Inf Commun Soc (2014) 17(1):86–104. doi:10.1080/1369118x.2013.853817
86. Sarıyüce AE, Gedik B, Jacques-Silva G, Wu K-L, Çatalyürek ÜV. Incremental K-Core Decomposition: Algorithms and Evaluation. VLDB J (2016) 25(3):425–47. doi:10.1007/s00778-016-0423-8
87. Dorogovtsev SN, Goltsev AV, Mendes JF. K-core Organization of Complex Networks. Phys Rev Lett (2006) 96:040601. doi:10.1103/PhysRevLett.96.040601
88. Alvarez-Hamelin JI, Dall'Asta L, Barrat A, Vespignani A. K-core Decomposition: A Tool for the Visualization of Large Scale Networks. arXiv preprint cs/0504107 (2005).
89. Newman MEJ. The Structure and Function of Complex Networks. SIAM Rev (2003) 45(2):167–256. doi:10.1137/s003614450342480
90.Bastian M, Heymann S, and Jacomy M, editors. Gephi: An Open Source Software for Exploring and Manipulating Networks. Third international AAAI conference on weblogs and social media (2009).
92. Fruchterman TMJ, Reingold EM. Graph Drawing by Force-Directed Placement. Softw Pract Exper (1991) 21(11):1129–64. doi:10.1002/spe.4380211102
93. Sharpe D. Chi-square Test Is Statistically Significant: Now what? Pract Assess Res Eval (2015) 20(1):8.
94. Kerby DS. The Simple Difference Formula: An Approach to Teaching Nonparametric Correlation. Compr Psychol (2014) 3:11. doi:10.2466/11.it.3.1
95. Nelson JL, Taneja H. The Small, Disloyal Fake News Audience: The Role of Audience Availability in Fake News Consumption. New Media Soc (2018) 20(10):3720–37. doi:10.1177/1461444818758715
96. Crane R, Sornette D. Robust Dynamic Classes Revealed by Measuring the Response Function of a Social System. Proc Natl Acad Sci (2008) 105(41):15649–53. doi:10.1073/pnas.0803685105
97. DiFonzo N, Bordia P. Rumor Psychology: Social and Organizational Approaches. American Psychological Association (2007). doi:10.1037/11503-000
98. Fath BP, Fiedler A, Li Z, Whittaker DH. Collective Destination Marketing in China: Leveraging Social media Celebrity Endorsement. Tourism Anal (2017) 22(3):377–87. doi:10.3727/108354217x14955605216113
99. Djafarova E, Rushworth C. Exploring the Credibility of Online Celebrities' Instagram Profiles in Influencing the Purchase Decisions of Young Female Users. Comput Hum Behav (2017) 68:1–7. doi:10.1016/j.chb.2016.11.009
100. Malik A, Khan ML, Quan-Haase A. Public Health Agencies Outreach through Instagram during the Covid-19 Pandemic: Crisis and Emergency Risk Communication Perspective. Int J Disaster Risk Reduction (2021) 61:102346. doi:10.1016/j.ijdrr.2021.102346
101. George J, Gerhart N, Torres R. Uncovering the Truth about Fake News: A Research Model Grounded in Multi-Disciplinary Literature. J Management Inf Syst (2021) 38(4):1067–94. doi:10.1080/07421222.2021.1990608
102. Zajonc RB. Social Facilitation. Science (1965) 149(3681):269–74. doi:10.1126/science.149.3681.269
103. Luqiu LR, Schmierbach M, Ng Y-L. Willingness to Follow Opinion Leaders: A Case Study of Chinese Weibo. Comput Hum Behav (2019) 101:42–50. doi:10.1016/j.chb.2019.07.005
104. Zhao Y, Kou G, Peng Y, Chen Y. Understanding Influence Power of Opinion Leaders in E-Commerce Networks: An Opinion Dynamics Theory Perspective. Inf Sci (2018) 426:131–47. doi:10.1016/j.ins.2017.10.031
105. Wang Z, Liu H, Liu W, Wang S. Understanding the Power of Opinion Leaders' Influence on the Diffusion Process of Popular mobile Games: Travel Frog on Sina Weibo. Comput Hum Behav (2020) 109:106354. doi:10.1016/j.chb.2020.106354
106. Granovetter MS. The Strength of Weak Ties. Am J Sociol (1973) 78(6):1360–80. doi:10.1086/225469
107. Wei J, Bu B, Guo X, Gollagher M. The Process of Crisis Information Dissemination: Impacts of the Strength of Ties in Social Networks. Kyb (2014). doi:10.1108/k-03-2013-0043
108. Petróczi A, Nepusz T, Bazsó F. Measuring Tie-Strength in Virtual Social Networks. Connections (2007) 27(2):39–52.
Keywords: fake news, stance detection, deep learning, debunking, refuter, social relationship network
Citation: Wang X, Chao F, Ma N and Yu G (2022) Exploring the Effect of Spreading Fake News Debunking Based on Social Relationship Networks. Front. Phys. 10:833385. doi: 10.3389/fphy.2022.833385
Received: 11 December 2021; Accepted: 18 February 2022;
Published: 26 April 2022.
Edited by:
Matjaž Perc, University of Maribor, SloveniaReviewed by:
Marija Mitrovic Dankulov, University of Belgrade, SerbiaValerio Restocchi, University of Edinburgh, United Kingdom
Copyright © 2022 Wang, Chao, Ma and Yu. This is an open-access article distributed under the terms of the Creative Commons Attribution License (CC BY). The use, distribution or reproduction in other forums is permitted, provided the original author(s) and the copyright owner(s) are credited and that the original publication in this journal is cited, in accordance with accepted academic practice. No use, distribution or reproduction is permitted which does not comply with these terms.
*Correspondence: Guang Yu, yug@hit.edu.cn