Multiclass classification using quantum convolutional neural networks with hybrid quantum-classical learning
- 1Laboratory of Molecular Beams, Physical Chemistry Division, Department of Chemistry, Lomonosov Moscow State University, Moscow, Russia
- 2Russian Quantum Center, Moscow, Russia
- 3National University of Science and Technology “MISIS”, Moscow, Russia
A Corrigendum on
Multiclass classification using quantum convolutional neural networks with hybrid quantum-classical learning
by Bokhan D, Mastiukova AS, Boev AS, Trubnikov DN and Fedorov AK (2022). Front. Phys. 10:1069985. doi: 10.3389/fphy.2022.1069985
In the original article, there were errors in the Abstract section, page 1. The sentences “Our results show that accuracies of our solution are similar to classical convolutional neural networks with comparable numbers of trainable parameters. We expect that our finding provide a new step towards the use of quantum neural networks for solving relevant problems in the NISQ era and beyond” have been copyedited for clarity. The corrected sentences appears below:
“Our results show that the accuracy of our solution is similar to classical convolutional neural networks with comparable numbers of trainable parameters. We expect that our findings will provide a new step toward the use of quantum neural networks for solving relevant problems in the NISQ era and beyond.”
In the original article, there was a spelling mistake in one of the keywords. Instead of “paramaterized,” it should be “parameterized.”
In the original article, there was an error in the Introduction section, page 1, first paragraph. The sentence “Specifically, in the quantum gate-based model, quantum algorithms are implemented as a sequence of logical operations under the qubits (quantum analogs of classical bits), which compose the corresponded quantum circuits terminating by qubit-selective measurements [3]” has been copyedited for clarity. The corrected sentence appears below:
“Specifically, in the quantum gate-based model, quantum algorithms are implemented as a sequence of logical operations under the qubits (quantum analogs of classical bits), which comprise the corresponding quantum circuits terminated by qubit-selective measurements [3].”
In the original article, there was an error in the Introduction section, page 2, first paragraph. The sentence “The amount of computing power for such applications is, however, greatly exceeds resources of currently available quantum computing devices” has been copyedited for clarity. The corrected sentence appears below:
“The amount of computing power for such applications, however, significantly exceeds the resources of currently available quantum computing devices.”
In the original article, there was an error in the Introduction section, page 2, second paragraph. The sentence “An interesting link between quantum-classical (variational) model and architectures of artificial neural networks opens up prospects for the use of such an approach for machine learning problems [15–22]” has been copyedited for clarity. The corrected sentence appears below:
“An interesting link between the quantum-classical (variational) model and architectures of artificial neural networks opens up prospects for the use of such an approach for machine learning problems [15–22].”
In the original article, there was an error in the Introduction section, page 2, third paragraph. The sentence “Very recently, an approach based on quantum convolutional neural network (QCNN) [32] have been used for binary classification, albeit, a way to its extension to the multiclass classification case has been discussed” has been copyedited for clarity. The corrected sentence appears below:
“Very recently, an approach based on quantum convolutional neural network (QCNN) [32] has been used for binary classification and a method to extend it to the multiclass classification case has been discussed.”
In the original article, there was an error in the Figure 2 caption, page 3. The sentence “Quantum circuits for preliminary scanning: In (A) the 4-qubit filter with 4-qubit entanglement shown; in (B) the stack of 3-qubit filters with 4-qubit entanglement is presented; in (C) the 2-qubit filters with 4-qubit entanglement demonstrated” has been copyedited for clarity. The corrected sentence appears below:
In the original article, there was an error in the Introduction section, page 3, second paragraph. The sentence “The developed approach use a traditional utilization of convolutional neural networks, in which few fully connected layers are placed after several convolutional layers” has been copyedited for clarity. The corrected sentence appears below:
“The developed approach uses traditional convolutional neural networks, in which few fully connected layers are placed after several convolutional layers.”
In the original article, there was an error in the Introduction section, page 3, second paragraph. The sentence “Then we discuss the modification of a quantum peceptron, which enables us to obtain highly accurate results using quantum circuits with relatively small number of parameters” has a spelling mistake. The corrected sentence appears below:
“Then we discuss the modification of a quantum perceptron, which enables us to obtain highly accurate results using quantum circuits with a relatively small number of parameters.”
In the original article, there was an error in the Introduction section, page 3, second paragraph. The sentence “The obtained results demonstrate successful solving the classification problem for the 4-classes of MNIST images” has been copyedited for clarity. The corrected sentence appears below:
“The obtained results demonstrate successful solving of the classification problem for the 4-classes of MNIST images.”
In the original article, there were errors in the General scheme section, page 3, fourth paragraph. The sentences “This approach use parametrized (variational) quantum circuits, where the exact parameters of quantum gates within the circuit can be changed. The general structure of our variational circuit is represented on Figure 1” have been copyedited for clarity. The corrected sentence appears below:
“This approach uses parametrized (variational) quantum circuits, where the exact parameters of quantum gates within the circuit can be changed. The general structure of our variational circuit is shown in Figure 1.”
In the original article, there was an error in the General scheme section, page 3, fifth paragraph. The sentence “In fact, due to the high cost of this step [11], we generate a set of encoding circuits, and store their parameters and structure in a memory, thus, hereby making the quantum version of dataset” was copyedited for clarity. The corrected sentence appears below:
“In fact, due to the high cost of this step [11], we generate a set of encoding circuits, and store their parameters and structures in a memory, thus generating a quantum dataset.”
In the original article, there was an error in the General scheme section, page 4, first paragraph. The sentence “It should be noted however that existing algorithms for amplitude encoding scales exponentialy with N; further study is needed to overcome this problem” has a spelling mistake. The corrected sentence appears below:
“It should be noted however that existing algorithms for amplitude encoding scale exponentially with N; further study is needed to overcome this problem.”
In the original article, there were errors in the General scheme section, page 4, second paragraph. The sentences “Each such layer consists of 2 sublayers that are needed to maintain translational invariance (at least, partially), and all the filters of the same size contain identical trainable parameters as it takes place the case for classical convolutional neural networks (CCNN). We note that for filters with the size of 3 we need a virtual qubit, which is always set to zero; such a trick is needed to fit the filter into 8 qubits in the translationally invariant manner” have been copyedited for clarity. The corrected sentences appears below:
“Each such layer consists of 2 sublayers that are needed to maintain translational invariance (at least, partially), and all the filters of the same size contain identical trainable parameters as is the case for classical convolutional neural networks (CCNN). We note that for filters with the size of 3 we need a virtual qubit, which is always set to zero; such a method is needed to fit the filter into 8 qubits in the translationally invariant manner.”
In the original article, there was an error in the Structures of layers section heading, page 4. The section heading “Structures of layers” has been corrected to “Structure of layers” to remain consistent throughout the article.
In the original article, there was an error in the Structure of layers section, sub-section 3.1 Preliminary scanning using n-qubit filters, page 4, sixth paragraph. The sentence “The structure of 4-qubit filters is presented in Figure 2A. First of all, RY(Θ1), RY(Θ2), RY(Θ3) and RY(Θ4) rotations are added in order to rotate each of four qubit separately” has been copyedited for clarity. The corrected sentence appears below:
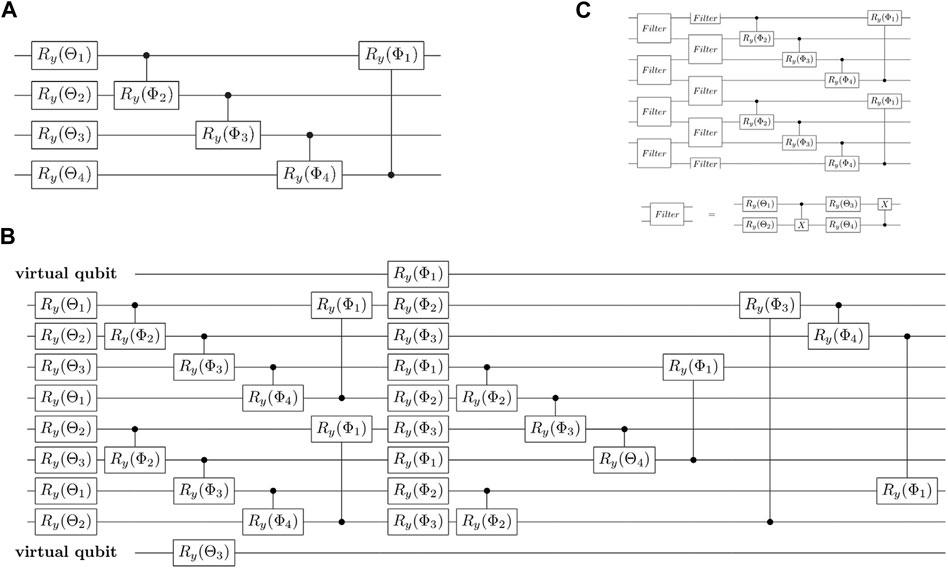
FIGURE 2. Quantum circuits for preliminary scanning: In (A) the 4-qubit filter with 4-qubit entanglement is shown; in (B) the stack of 3-qubit filters with 4-qubit entanglement is presented; in (C) the 2-qubit filters with 4-qubit entanglement is demonstrated.
“The structure of 4-qubit filters is presented in Figure 2A. First of all, RY(Θ1), RY(Θ2), RY(Θ3) and RY(Θ4) rotations are added in order to rotate each of the four qubits separately.”
In the original article, there was an error in the Structure of layers section, sub-section 3.1 Preliminary scanning using n-qubit filters, page 4, sixth paragraph. The sentence “Here, as we demonstrate, the parameterized entanglement scheme provide higher accuracy of image classification due to the more flexible learning algorithm” has been copyedited for clarity. The corrected sentence appears below:
“Here, as we demonstrate, the parameterized entanglement scheme provides higher accuracy of image classification due to the more flexible learning algorithm.”
In the original article, there was an error in the Structure of layers section, sub-section 3.1 Preliminary scanning using n-qubit filters, page 4, seventh paragraph. The sentence “The degree of entanglement is controlled by parameters Φ, which makes learning process more flexible, and, thus, classification procedure may become more accurate” has been copyedited for clarity. The corrected sentence appears below:
“The degree of entanglement is controlled by the parameters Φ, which makes the learning process more flexible, and, thus, the classification procedure may become more accurate.”
In the original article, there was an error in the Structure of layers section, sub-section 3.1 Preliminary scanning using n-qubit filters, page 4, seventh paragraph. The sentence “In quantum domain, we can switch from separable (non-entangled) to entangled state, what could play the role of non-linearities in classical learning” has been copyedited for clarity. The corrected sentence appears below:
“In the quantum domain, we can switch from separable (non-entangled) to entangled states, that play the role of non-linearities in classical learning.”
In the original article, there was an error in the Structure of layers section, sub-section 3.1 Preliminary scanning using n-qubit filters, page 4, eighth paragraph. The sentence “In order to rotate 3 indiviadual qubits RY(Θ1), RY (Θ2) and RY (Θ3) gates are added” has a spelling mistake. The corrected sentence appears below:
“In order to rotate 3 individual qubits RY(Θ1), RY (Θ2) and RY (Θ3) gates are added.”
In the original article, there was an error in the Structure of layers section, sub-section 3.1 Preliminary scanning using n-qubit filters, page 4, eighth paragraph. The sentence “Even though, the entanglement of 3 qubits looks more intuitive in this case, as we show below, the 4-qubit one provide more accurate results on image recognition” has been copyedited for clarity. The corrected sentence appears below:
“Even though the entanglement of 3 qubits looks more intuitive in this case, as we show below, the 4-qubit entanglement provides more accurate results on image recognition.”
In the original article, there were errors in the Structure of layers section, sub-section 3.1 Preliminary scanning using n-qubit filters, page 4, eighth paragraph. The sentences “As in all previous cases, we use 4-qubit entanglement and the filter consist of 4 individual rotations with additional entanglement by CNOT gates. The idea of using 4-qubit entanglement is inspired by classical CNN, where generation of new feature maps is done by summation of contracted with weights previous feature maps and subsequent application of non-linearity” have been copyedited for clarity. The corrected sentences appear below:
“As in all previous cases, we use 4-qubit entanglement and the filter consists of 4 individual rotations with additional entanglement by CNOT gates. The idea of using 4-qubit entanglement is inspired by classical CNN, where generation of new feature maps is done by summation of the shared weights of previous feature maps and subsequent application of non-linearity.”
In the original article, there was an error in the Structure of layers section, sub-section 3.2 Quantum convolutional neural network layer with pooling, page 4, ninth paragraph. The sentence “We note that in the pooling circuit, controlled RZ rotation is activated if the first qubit is at state 1, while the controlled RX gate is used when upper qubit at state 0” has been copyedited for clarity. The corrected sentence appears below:
“We note that in the pooling circuit, controlled RZ rotation is activated if the first qubit is at state 1, while the controlled RX gate is used when the upper qubit is at state 0.”
In the original article, there was an error in the Structure of layers section, sub-section 3.3 Regular layers, page 5, first paragraph. The sentence “The second reduction of qubit number in circuit is done by two pooling procedure as in the case of convolution layers” has been copyedited for clarity. The corrected sentence appears below:
“The second reduction of qubit number in the circuit is done by two pooling procedures as in the case of convolution layers.”
In the original article, there was an error in the Structure of layers section, sub-section 3.4 Toffoli and controlled rotation gates, page 5, second paragraph. The sentence “The situation is typically more difficult with for three-qubit gates, such as Toffoli gate” has been copyedited for clarity. The corrected sentence appears below:
“The situation is typically more difficult for three-qubit gates, such as a Toffoli gate.”
In the original article, there was an error in the Structure of layers section, sub-section 3.4 Toffoli and controlled rotation gates, page 5, second paragraph. The sentence “In order to implement the Toffoli gate, we consider the qubit inversion as a rotation operation around X or Y axes and in our case doubly-controlled RY(Θ) gate is used with the value of Θ = 2π” has been copyedited for clarity. The corrected sentence appears below:
“In order to implement the Toffoli gate, we consider the qubit inversion as a rotation operation around X or Y axes and in our case a doubly-controlled RY(Θ) gate is used with the value of Θ = 2π.”
In the original article, there were errors in the Structure of layers section, sub-section 3.4 Toffoli and controlled rotation gates, page 6, first paragraph. The sentences “The circuit is presented in Figure 4B and it corresponds to the representation of sum of parameterized n-controlled rotations, which are considered in Ref. [40]. Toffoli gate, in fact, can be considered as a sum of such rotations with n = 2, where Θ angles of all rotations, except the one that is controlled by 11th combination, are set to zero. The definition of α angles is realized along the lines of the procedure of Ref. [40]; they are obtained from Θ angles by simple matrix transformation” have been copyedited for clarity. The corrected sentences appear below:
“The circuit is presented in Figure 4B and it corresponds to the representation of the sum of parameterized n-controlled rotations, which are considered in Ref. [40]. A Toffoli gate, in fact, can be considered as a sum of such rotations with n = 2, where Θ angles of all rotations, except the one that is controlled by the 11th combination, are set to zero. The definition of α angles is realized along the lines of the previously described procedure [40]; they are obtained from Θ angles by simple matrix transformation.”
In the original article, there were errors in the Classification results section, page 6, fourth paragraph. The sentences “All the simulations are performed using Cirq python library for the constructions of quantum circuits; TensorFlowQuantum library [39] is used for the implementation of machine learning algorithm with parameterized quantum circuits. We use the Adam version of gradient descent with learning rate equal to 0.00005, the overall number of trainable parameters in the QCNN circuit is equal to 149” have been copyedited for clarity. The corrected sentences appear below:
“All the simulations are performed using Cirq python library for the constructions of quantum circuits; TensorFlowQuantum library [39] is used for the implementation of the machine learning algorithm with parameterized quantum circuits. We use the Adam version of gradient descent, with a learning rate equal to 0.00005, and the overall number of trainable parameters in the QCNN circuit is equal to 149.”
In the original article, there was an error in the Classification results section, page 6, fifth paragraph. The sentence “Within the first set training and classification is done for the case, when dataset consists of images, which has certain similarity and, thus, classification problem become more difficult” has been copyedited for clarity. The corrected sentence appears below:
“Within the first set, training and classification are completed for the case, when the dataset consists of images which have certain similarities; thus, the classification problem becomes more difficult.”
In the original article, there were errors in the Classification results section, page 6, seventh paragraph. The sentences “The second experimental set is focused on images, which are strongly differs from each other, thus, making recognition process easier; MNIST digits 0, 1, 2, and 3; fashion MNIST images with labels 1, 2, 8, and 9 are considered. Total number of considered images of each type is given in Table 1” have been copyedited for clarity. The corrected sentences appear below:
“The second experimental set is focused on images, which strongly differ from one another thus making the recognition process easier; MNIST images of digits 0, 1, 2, and 3 and fashion MNIST images with labels 1, 2, 8, and 9 are used here. The total number of considered images of each type is given in Table 1.”
In the original article, there were errors in the Classification results section, page 6, eighth paragraph. The sentences “Each image vector is normalized to one since only such kind of vectors can be used by amplitude encoding algorithm. Results of image classification are given in Table 2” have been copyedited for clarity. The corrected sentences appear below:
“Each image vector is normalized to one since only that type of vector can be used by the amplitude encoding algorithm. The results of image classification are given in Table 2.”
In the original article, there was an error in the Classification results section, page 6, eighth paragraph. The sentence “QCNN examples, provided within documentation of TensorFlowQuantum [39] also can be relatively simply generalized for the case of multiclass classification tasks” has been copyedited for clarity. The corrected sentence appears below:
“QCNN examples, provided within the documentation of TensorFlowQuantum [39] also can be relatively simply generalized for the case of multiclass classification tasks.”
In the original article, there was an error in the Classification results section, page 7, first paragraph. The sentence “In the second column of Table 2 we provide results of experiments with circuits, similar to those of Ref. [31]” has been copyedited for clarity. The corrected sentence appears below:
“In the second column of Table 2 we provide the results of experiments with circuits, similar to previous results [31].”
In the original article, there were errors in the Classification results section, page 7, first paragraph. The sentences “Also, we remove all the parts of circuit of Figure 1, which are placed after regular layers, i.e., pooling layers, final layers and the part with Toffoli gates. Entanglement of ancilla qubits with regular layers is done via CNOT gates according to Figure 2 of reg [31]. Third column of Table 2 contain results, obtained with full circuit of Figure 1. Significant improvement of the accuracy of classification results caused by two facts. First one—is the usage of parameterized entanglemet in our circuit. Secondly, increase of the performance may be connected with the fact, that our circuit constructed in a similar way to classical neural networks—we use qubit reduction procedure in analogy with the reduction of number of layers outputs in classical case until the number of outputs become equal to the number of target classes. Note that in Figure 1 ancilla qubits are used only at read-out step and no entanglement is needed between ancillas and other qubits during computational procedure, what can significantly simplify requirements to the corresponding quantum hardware. We also compare obtained quantum results with results of the CCNN with similar number of parameters, which is 188 in our case. The structure of the CCNN is presented in Table 3. Clearly, classical results are more accurate, what indicate on the fact that with similar number of parameters classical model is still more expressive. An analysis of possible quantum advantage in ML tasks is presented in Ref. [46]. In their study authors analyze ML models based on kernel functions and show that with enough data provided classical methods become more powerfull then corresponding quantum algoritms. Thus, additional study is still needed to find ML tasks where quantum algorithms will outperform thier classical analogs” have been copyedited for clarity and to correct spelling mistakes. The corrected sentences appear below:
“Also, we remove all the parts of the circuit of Figure 1, which are placed after regular layers, i.e., pooling layers, final layers, and the part with Toffoli gates. Entanglement of ancilla qubits with regular layers is done via CNOT gates according to Figure 2 of Ref. [31]. The third column in Table 2 contains the results obtained with the full circuit shown in Figure 1. The significant improvement in the accuracy of the classification results is caused by two facts. Firstly, the usage of parameterized entanglement in our circuit. Secondly, the increased performance may be connected with the fact that our circuit is constructed in a similar way to classical neural networks—we use a qubit reduction procedure analogous to the reduction of the number of layer outputs in the classical case, i.e., reduction until the number of outputs equals the number of target classes. Note that in Figure 1 ancilla qubits are used only at the read-out step and no entanglement is needed between ancillas and other qubits during the computational procedure, significantly simplifying the corresponding quantum hardware requirements. We also compare obtained quantum results with results of the CCNN with a similar number of parameters, which is 188 in our case. The structure of the CCNN is presented in Table 3. Clearly, the classical results are more accurate, indicating that, with a similar number of parameters, the classical model is still more expressive. An analysis of possible quantum advantage in ML tasks is presented in Ref. [46]. In their study, the authors analyze ML models based on kernel functions and show that with enough data provided, classical methods become more powerful than the corresponding quantum algorithms. Thus, additional study is still needed to find ML tasks where quantum algorithms will outperform their classical analogs.”
In the original article, there were errors in the Classification results section, page 7, second paragraph. The sentences “In overall, the QCNN can produce accuracy of multiclass classification that are qualitatively similar to the classical model if the number of parameters are comparable. We would like to mention that the similar level of the accuracy has been achieved in Ref. [31] for the case of the 3-class classification problem. Here we have demonstrated this level of the accuracy for the 4-class classification tasks, which to the best of our knowledge is the first such demonstration” have been copyedited for clarity. The corrected sentences appear below:
“Overall, the QCNN can produce accurate multiclass classifications that are qualitatively similar to the classical model if the number of parameters is comparable. A similar level of accuracy has been achieved previously [31] for the 3-class classification problem. Here, we have demonstrated this level of the accuracy for the 4-class classification tasks, which to the best of our knowledge is the first such demonstration.”
In the original article, there were errors in the Conclusion section, page 7, third paragraph. The sentences “The main conceptual improvements that we have realized are the new model for quantum perceptron and the optimized structure of the quantum circuit. We have shown the use of the proposed approach for 4-class classification for the case of four MNIST” have been copyedited for clarity. The corrected sentences appear below:
“The main conceptual improvements that we have realized are the new model for quantum perceptron and an optimized structure of the quantum circuit. We have shown the use of the proposed approach for 4-class classification for the case of four MNISTs.”
In the original article, there was an error in the Conclusion section, page 7, third paragraph. The sentence “Moreover, since the scheme require the use of multiqubit gates, the qudit processors, where multiqubit gate decompositions can be implemented in a more efficient manner, can be of interest for the realization of such algorithms” has been copyedited for clarity. The corrected sentence appears below:
“Moreover, since the scheme requires the use of multiqubit gates, the qudit processors, where multiqubit gate decompositions can be implemented in a more efficient manner, can be of interest for the realization of such algorithms.”
In the published article, there was an error in the Conflict of interest statement. The statement “The authors declare that the research was conducted in the absence of any commercial or financial relationships that could be construed as a potential conflict of interest” has been updated. The correct Conflict of Interest statement appears below.
“Authors DB, AM, AB, and AF were employed at the Russian Quantum Center which provides consulting services. Owing to the employments and consulting activities of authors, the authors have financial interests in the commercial applications of quantum computing.”
The authors apologize for these errors and state that this does not change the scientific conclusions of the article in any way. The original article has been updated.
Publisher’s note
All claims expressed in this article are solely those of the authors and do not necessarily represent those of their affiliated organizations, or those of the publisher, the editors and the reviewers. Any product that may be evaluated in this article, or claim that may be made by its manufacturer, is not guaranteed or endorsed by the publisher.
Keywords: quantum learning, multinomial classification, parameterized quantum circuit, variational circuits, amplitude encoding
Citation: Bokhan D, Mastiukova AS, Boev AS, Trubnikov DN and Fedorov AK (2023) Corrigendum: Multiclass classification using quantum convolutional neural networks with hybrid quantum-classical learning. Front. Phys. 10:1112211. doi: 10.3389/fphy.2022.1112211
Received: 30 November 2022; Accepted: 21 December 2022;
Published: 18 April 2023.
Approved by:
Frontiers Editorial Office, Frontiers Media SA, SwitzerlandCopyright © 2023 Bokhan, Mastiukova, Boev, Trubnikov and Fedorov. This is an open-access article distributed under the terms of the Creative Commons Attribution License (CC BY). The use, distribution or reproduction in other forums is permitted, provided the original author(s) and the copyright owner(s) are credited and that the original publication in this journal is cited, in accordance with accepted academic practice. No use, distribution or reproduction is permitted which does not comply with these terms.
*Correspondence: Denis Bokhan, denisbokhan@mail.ru; Aleksey K. Fedorov, akf@rqc.ru