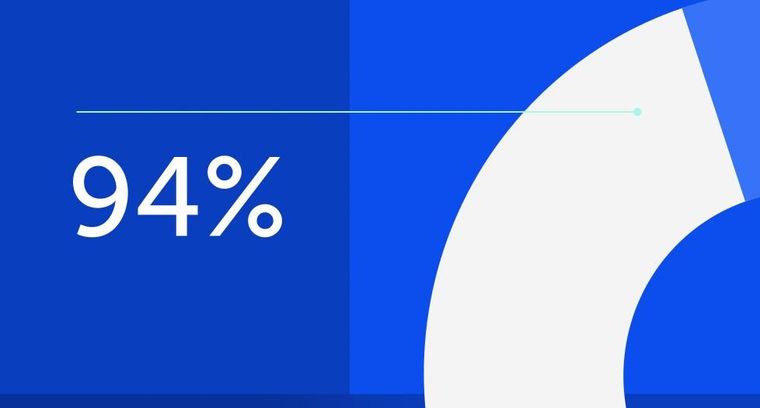
94% of researchers rate our articles as excellent or good
Learn more about the work of our research integrity team to safeguard the quality of each article we publish.
Find out more
EDITORIAL article
Front. Phys., 12 January 2023
Sec. Statistical and Computational Physics
Volume 10 - 2022 | https://doi.org/10.3389/fphy.2022.1101756
This article is part of the Research TopicDynamical Systems, PDEs and Networks for Biomedical Applications: Mathematical Modeling, Analysis and SimulationsView all 18 articles
Editorial on the Research Topic
Dynamical systems, PDEs and networks for biomedical applications: Mathematical modeling, analysis and simulations
In recent decades, mathematics for healthcare [1] and quantitative biomedicine have become increasingly important. This research area includes various aspects such as modeling, analysis and simulation of numerous highly relevant processes in clinical practice and combines different scientific disciplines like biology, bioengineering, physics, mathematics and medicine. Important biomedical applications are for instance cellular, biochemical and biomolecular processes, tumour growth, electrophysiology of the heart or the brain, tissue regeneration and biomedical engineering. In this line of research, mathematical and computational modeling, cf. e.g. [2–4], have become essential components in interdisciplinary and multidisciplinary research projects and contributes significantly to a better understanding of real-world phenomena. The great importance of this research field also results in the establishment of numerous research centres and research groups in the field of mathematics for healthcare and quantitative biomedicine.
New interdisciplinary research fields like computational cardiology [5], computational neurosciences [6] and computational oncology [7] arise and can be used to develop new therapies and help in improving clinical decision [8–12]. There are plenty of important examples of mathematical and numerical approaches which are applied to drug (safety) testing or drug development, the analysis and simulation of complex dynamics in the heart or the brain such as cardiac arrhythmias [13] or seizures [14], and are becoming more and more relevant these days. Computer modeling of complex dynamic processes like wound and bone healing [15] gives new insights into how such complex biological processes work and helps to make better predictions.
The origins for these developments lie in the great opportunities for developing not only complex (and detailed) models that can be tested against experimental data, which is becoming available in increasing amount and quality, but also computational methods to solve these models. Together, this opens new challenges and prospects for a systematical investigation of both biological and mathematical issues from life science. Thus, this Research Topic is focused on advanced methods in mathematical and computational modeling of biomedical phenomena using (experimental) data, their analysis and numerical simulations that contribute to a significantly improved comprehension of these phenomena. The main focus of this Research Topic is three-fold: 1) modeling approaches using differential equations or networks describing the behavior of single cells, cell groups, tissue or organs. 2) The analysis of the dynamics and behavior of certain biomedical models improving the state-of-art understanding of certain phenomena like cardiovascular and neurological diseases and disorders. 3) Improved numerical methods to simulate and analyze complex biomedical models.
In computational cardiology, the electrophysiological behavior of cardiac tissue is usually modelled either by the monodomain or bidomain models, which mathematically represent coupled systems of partial and ordinary differential equations, and give insights into the conduction properties of the electrochemical wave traversing the cardiac muscle. The bidomain model is considered to be the gold standard for numerical simulations and the monodomain model is a special case assuming equal anisotropy rates. Jæger et al. studied and compared the bidomain model, which represents the average over a large number of cardiomyocytes, and the EMI model, which is considered to be more accurate since it includes explicitly the extracellular space (E), the cell membrane (M) and the intracellular space (I). The authors showed the limitation of the bidomain model and that it can be derived directly from the cell-based EMI model as well as their similarities. Both models provide remarkably similar results when the parameters of importance for the conduction velocity lie in the normal range. The authors concluded that the bidomain model represents normal cardiac conduction very well if the scale of interest contains many cells, but cannot be used to study conduction in the vicinity of individual cells. Therefore, depending on the biomedical application one has three accurate models of different complexity and different numerical efforts. In this line of research, Mulimani et al. and Zykov et al. studied different types of spiral waves occurring in monodomain models. Monodomain models have been proved to be good approximations of cardiac tissue for wave propagation. Mulimani et al. focused their investigations on the effects of cellular parameters on the properties of the action potential of a myocyte. For instance, this study provides an understanding of how changes in the action potential at single-cell level can be related to changes in spiral-wave frequency at tissue level, which may also result in life-threatening cardiac arrhythmias. Furthermore, Zykov et al. investigated spiral wave instability in a modified Barkley model and showed the existence of two quite different parameter regions of this instability. The first region can be linked to the presence of a Hopf bifurcation and is a well known scenario, while the second region cannot be explained by a Hopf bifurcation and a hysteresis phenomenon was detected on its boundary. Besides the efficient modelling of a certain problem, the development of advanced numerical algorithms are important. Xiao et al. introduced a Fourier filter-based physics-informed convolutional recurrent network that can solve partial differential equations without labeled data. This approach was applied to several problems such as the two-dimensional viscous Burger’s equation and FitzHugh–Nagumo reaction-diffusion equations. On single-cell level Hustad et al. pointed out that ODE computations can use a substantial fraction of the total computer time of a heart simulator and they focused on systematically studying how to efficiently use modern multicore CPUs for this costly computational task. Furthermore, they concluded that the largest performance improvement of the ODE solvers arises from using SIMD vectorisation. Molinari et al. established an experimentally calibrated computational model of ex-vivo cardiac radiofrequency catheter ablation featuring the inclusion of three-dimensional myocardial anisotropy, the implementation of a multi-scale time lag thermal ablation model, and the adoption of a model of cell death for the estimation of tissue damage and lesion sizing. The investigation gave new insights into the anisotropic thermal behavior of cardiac tissue and proved that the intrinsic anisotropic microstructure plays a crucial role in the thermo-electrical response of the tissue undergoing hyperthermic treatments.
In the realm of neuroscience, Daversin-Catty et al. introduced a new mathematical and numerical formalism of pulsatile viscous fluid flow in networks based on topological and geometrical model reduction, which is a robust and effective computational approach for large scale in silico studies. Moreover, different computational scenarios were studied, e.g. perivascular space flow induced by cardiac pulse wave-induced movement of the inner vascular wall. Furthermore, the framework established a foundation for future computational studies of perivascular flow to improve our understanding of brain transport. Yamakou et al. investigated coherence resonance and self-induced stochastic resonance in multiplex neural networks, which consist of electrically connected FitzHugh–Nagumo model neurons, in the presence of spike-timing-dependent plasticity. Here, several important questions leading to interesting future research directions were addressed, such as: Which of these noise-induced resonance phenomena is more robust to parametric perturbations? In order to better understand burst generation in inhibitory half-center central pattern generators, Olenik et al. studied a minimal network of two neurons coupled through depressing synapses. They have shown that synaptic depression of inhibition can enable the two-cell (Morris–Lecar model) network to produce burst solutions of different periods, when the strength of the maximum synaptic conductance is varied. Furthermore, Liu et al. investigated BAM neural networks with delays. By using generalized Halanay inequalities and constructing appropriate Lyapunov functionals, some novelty criteria are obtained for the asymptotic stability of BAM neural systems with time delays. Spiliotis et al. analyzed network dynamics under healthy, Parkinsonian and deep brain stimulation conditions with the aim to improve deep brain stimulation treatment. For this purpose the authors built a large scale computational model that consists of certain elements of the basal ganglia network. This new approach is based on the detection of network communities or modules central to activity distribution. The aim of the paper was two-fold: 1) to identify positions of nodes of high functional impact using network analysis and 2) to explore the relationship between anatomical structure and neural activity using modified Hodgkin–Huxley models. The analysis showed that in all modular areas, Parkinsonian conditions alter power spectrogams.
The last area of this Research Topic concerns modeling and analysis to inform healthcare decision-making in terms of therapy/drug delivery (Zlobina et al.), managing infections (Kuttler et al.), informing new drugs’ discovery (Bondar et al.) and managing pandemics (Chattopadhyay et al.). Zlobina et al. proposed that a qualitative model with a proper level of abstraction can be used to predict the response of a wound to dynamic therapy and investigated mathematically the most effective spatio-temporal regimes for drug delivery to accelerate wound closure. This study provides a possible optimal regime of therapy that focuses on macrophage activity and a hypothesis of treatment outcome to be tested in future experiments. Kuttler et al. introduced a mathematical model for bacterial growth and control by antibiotics treatment, including quorum sensing as a special kind of communication. The system is formalized by a system of semi-linear parabolic partial differential equations and can be used for in silico studies of bacterial cooperative behavior in the special case of quorum sensing. The obtained data suggest that even a small bacterial population maintains an essential quorum. Bondar et al. discussed general principles of protonation-coupled protein conformational dynamics and how these apply to G-Protein Coupled Receptors. The authors found that the internal H-bond networks contain sites where structural rearrangements upon protonation change could be transmitted throughout the protein. In Chattopadhyay et al. a novel method of approximating a closed form solution of the ensemble averaged density profiles and correlation statistics of coupled dynamical systems, drawing from a technique used in mathematical biology to calculate a quantity called the basic reproduction number R0, was presented. It was shown that the R0 formulation can be used to calculate the correlation between diffusivity paths, agreeing closely with the exact numerical solution of the double-diffusion model.
The topics covered in this Research Topic are far from exhaustive. Rather, they point to a broader range of problems that can not only be addressed by available mathematical and computational approaches, but also stimulate the development of novel tools and techniques.
AE, KT-A, GL, and EM conceived and wrote the editorial.
We would like to thank all reviewers for their constructive comments in an attempt to initiate a dialogue between theoreticians and practitioners in the articles of this Research Topic. KT-A gratefully acknowledges the financial support of the EPSRC via grant EP/T017856/1. KT-A also acknowledges the support of the Technical University of Munich—Institute for Advanced Study, funded by the German Excellence Initiative.
The authors declare that the research was conducted in the absence of any commercial or financial relationships that could be construed as a potential conflict of interest.
All claims expressed in this article are solely those of the authors and do not necessarily represent those of their affiliated organizations, or those of the publisher, the editors and the reviewers. Any product that may be evaluated in this article, or claim that may be made by its manufacturer, is not guaranteed or endorsed by the publisher.
1. Tsaneva-Atanasova K, Diaz-Zuccarini V. Editorial: Mathematics for healthcare as part of computational medicine. Front Physiol (2018) 9:985. doi:10.3389/fphys.2018.00985
2.L Geris, editor. Computational modeling in tissue engineering. Berlin, Germany: Springer (2013). doi:10.1007/978-3-642-32563-2
3.K-A Mardal, ME Rognes, TB Thompson, and LM Valnes, editors. Mathematical modeling of the human brain. Berlin, Germany: Springer (2022). doi:10.1007/978-3-030-95136-8
4.J Sundnes, G Lines, B Nielsen, KA Mardal, and A Tveito, editors. Computing the electrical activity in the heart. Berlin, Germany: Springer (2006). doi:10.1007/3-540-33437-8
5. Niederer S, Lumens J, Trayanova N. Computational models in cardiology. Nat Rev Cardiol (2019) 16:100–11. doi:10.1038/s41569-018-0104-y
6. Kass RE, Amari SI, Arai K, Brown EN, Diekman CO, Diesmann M, et al. Computational neuroscience: Mathematical and statistical perspectives. Annu Rev Stat Its Appl (2018) 5:183–214. doi:10.1146/annurev-statistics-041715-033733
7. Karolak A, Markov DA, McCawley LJ, Rejniak KA. Towards personalized computational oncology: From spatial models of tumour spheroids, to organoids, to tissues. J R Soc Interf (2018) 15:20170703. doi:10.1098/rsif.2017.0703
8. Barbolosi D, Ciccolini J, Lacarelle B, Barlési F, André N. Computational oncology — Mathematical modelling of drug regimens for precision medicine. Nat Rev Clin Oncol (2016) 13:242–54. doi:10.1038/nrclinonc.2015.204
9. Kringelbach ML, Deco G. Brain states and transitions: Insights from computational neuroscience. Cel Rep (2020) 32:108128. doi:10.1016/j.celrep.2020.108128
10. Owens AP, Allen M, Ondobaka S, Friston KJ. Interoceptive inference: From computational neuroscience to clinic. Neurosci Biobehavioral Rev (2018) 90:174–83. doi:10.1016/j.neubiorev.2018.04.017
11. Peirlinck M, Costabal F, Yao J, Guccione JM, Tripathy S, Wang Y, et al. Precision medicine in human heart modeling. Biomech Model Mechanobiol (2021) 20:803–31. doi:10.1007/s10237-021-01421-z
12. Trayanova NA, O’Hara T, Bayer JD, Boyle PM, McDowell KS, Constantino J, et al. Computational cardiology: How computer simulations could be used to develop new therapies and advance existing ones. EP Europace (2012) 14:v82–9. doi:10.1093/europace/eus277
13. Trayanova NA, Doshi AN, Prakosa A. How personalized heart modeling can help treatment of lethal arrhythmias: A focus on ventricular tachycardia ablation strategies in post-infarction patients. WIREs Syst Biol Med (2020) 12:e1477. doi:10.1002/wsbm.1477
14. Depannemaecker D, Destexhe A, Jirsa V, Bernard C. Modeling seizures: From single neurons to networks. Seizure (2021) 90:4–8. doi:10.1016/j.seizure.2021.06.015
Keywords: mathematical and computational modeling, biomedical applications, applied analysis, complex dynamic processes, computational medicine, differential equations, network
Citation: Erhardt AH, Tsaneva-Atanasova K, Lines GT and Martens EA (2023) Editorial: Dynamical systems, PDEs and networks for biomedical applications: Mathematical modeling, analysis and simulations. Front. Phys. 10:1101756. doi: 10.3389/fphy.2022.1101756
Received: 18 November 2022; Accepted: 30 December 2022;
Published: 12 January 2023.
Edited by:
Marcel Filoche, École Supérieure de Physique et de Chimie Industrielles de la Ville de Paris, FranceReviewed by:
Alfonso Bueno-Orovio, University of Oxford, United KingdomCopyright © 2023 Erhardt, Tsaneva-Atanasova, Lines and Martens. This is an open-access article distributed under the terms of the Creative Commons Attribution License (CC BY). The use, distribution or reproduction in other forums is permitted, provided the original author(s) and the copyright owner(s) are credited and that the original publication in this journal is cited, in accordance with accepted academic practice. No use, distribution or reproduction is permitted which does not comply with these terms.
*Correspondence: André H. Erhardt, YW5kcmUuZXJoYXJkdEB3aWFzLWJlcmxpbi5kZQ==
Disclaimer: All claims expressed in this article are solely those of the authors and do not necessarily represent those of their affiliated organizations, or those of the publisher, the editors and the reviewers. Any product that may be evaluated in this article or claim that may be made by its manufacturer is not guaranteed or endorsed by the publisher.
Research integrity at Frontiers
Learn more about the work of our research integrity team to safeguard the quality of each article we publish.