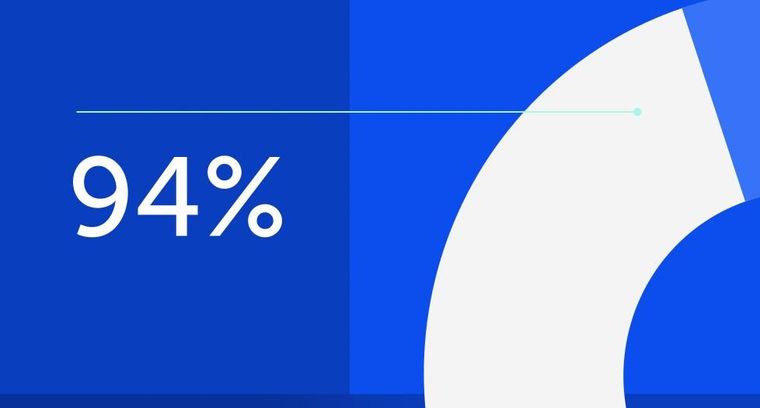
94% of researchers rate our articles as excellent or good
Learn more about the work of our research integrity team to safeguard the quality of each article we publish.
Find out more
ORIGINAL RESEARCH article
Front. Phys., 12 December 2022
Sec. Social Physics
Volume 10 - 2022 | https://doi.org/10.3389/fphy.2022.1047835
This article is part of the Research TopicIntegrating Physical and Social Sciences towards the Sustainable Development GoalsView all 7 articles
Despite successful results of using complex networks to model and characterize the spread of dengue cases, works to date have mainly used data from primarily reported cases, without further consideration whether they were later confirmed or not. On the other hand, a study of the interdependence of confirmed and discarded cases of arboviruses have emphasized that the co-circulation of three arboviruses—dengue, Zika and chikungunya—may have led to false diagnoses due to several similarities in the early symptoms of the three diseases on acute phase. This implies that case notifications of one disease could be confirmed cases of others, and that discarded cases must be taken into account to avoid misinterpretations of the phenomenon. In this work we investigated the consequences of including information from discarded and confirmed cases in the analysis of arbovirus networks. This is done by firstly evaluating the possible changes in the networks after removing the discarded cases from the database of each arbovirus, and secondly by verifying the cross-relationship of the indices of the networks of confirmed and discarded cases of arboviruses. As will be detailed later on, our results reveal changes in the network indices when compared to when only confirmed cases are considered. The magnitudes of the changes are directly proportional to the amount of discarded cases. The results also reveal a strong correlation between the average degree of the networks of discarded cases of dengue and confirmed cases of Zika, but only a moderate correlation between that for networks of discarded cases of dengue and confirmed cases of chikungunya. This finding is compatible with the fact that dengue and Zika diseases are caused by closely related flaviviruses, what is not the case of the chikungunya caused by a togavirus.
Urban arboviruses are a public health problem affecting millions of people worldwide [1]. Brazil, for example, has experienced successive dengue epidemics over the years [2] and, more recently, outbreaks of Zika and chikungunya became new problems leading to an increase in cases of congenital microcephaly and Guillain-Barré syndrome in the case of Zika [3–6], and intense arthralgia that can last for months or even years and lead to chronic and disabling forms, in the case of chikungunya [6–8]. These three arboviruses are transmitted by vectors Aedes genus mosquitos. Several factors favor the increase of that mosquito population, as generation of deforested regions, the accelerated and disorderly increase of the human population in urban areas, lack of adequate sanitation and infrastructure in these cities [9, 10], concomitantly with enhanced predisposition to water accumulation in such places. The understanding of how the spread of these arboviruses happens is extremely important to find better control strategies. Complex networks have been used in different studies focused on identifying and characterizing aspects of the dengue dissemination dynamics [11–19]. As for analyzes of recent large scale Zika outbreaks, a number of similar works are already available [20, 21].
The simultaneous co-circulation of these three arboviruses in the same places causes a complex problem for public health. Indeed, several similarities of their clinical symptoms difficult their precise diagnosis on acute phase as well as monitoring their spread between different municipalities, impairing the design of more efficient strategies for their control [6, 22–25].
Recent studies have focused on aspects related to the co-circulation of dengue, Zika and chikungunya and consequent misdiagnosis. An estimation of the interdependence in the time series of notified, confirmed and discarded cases of the three arbovirus has been presented in [23]. Similarly, the consequences of misdiagnosis in underestimating the Zika epidemics in the Americas was discussed in [25]. On the other hand, in previous studies on dengue spread using network approaches [11–16], as well as on an on-going work analysing the simultaneous co-circulation of three arboviruses [26], databases of primarily identified cases have been used. Such early classification does not account to whether each case has been later subject of a subsequent and a more precise analysis, being reclassified as confirmed, discarded, or inconclusive. Consequently, the use of primarily reported cases to carry fundamental studies in conditions where similar viruses co-circulate can cause misinterpretations about the phenomenon, due to the possible differences between the networks obtained with data from reported cases and confirmed cases.
The objective of this work is to extract insights about the spread of arbovirus based on a detailed analysis of networks built from time series of notified, confirmed, and discarded cases in the state of Bahia, Brazil. The collected data indicate that dengue, the arbovirus that is circulating for a largest time in Brazil, is responsible for the largest number of primarily notified cases. Besides that, since dengue has similar symptoms to those following Zika and chikungunya infections, both the number of notified and discarded cases has substantially increased in the years 2015 and 2016, suggesting the possibility of misdiagnosis when the two other arbovirus were not yet present in large parcels of the territory. Therefore, it is expected that, after comparing pairs of networks of notified and confirmed cases, those built from dengue data will present the largest differences as compared to their counterparts from Zika and chikungunya. Finally, by comparing networks of discarded cases of one disease with those of notified and/or confirmed cases of another disease, we may collect evidence that the misdiagnosis of arbovirus can be related to the phenomenon of multiple co-circulation. Such an output will support the claim that building networks based on data of confirmed cases is crucial to developing efficient the strategies of combat and control of these arboviruses.
The data obtained on dengue, Zika and chikungunya in this study were from the Notifiable Diseases Information System (Sistema de Informação de Agravos de Notificação—SINAN) from Brazilian Ministry of Health (MoH), available for public access online [27]. Each arbovirus database contains information on the reported cases of each patient for the 417 municipalities of the state of Bahia in the period 2014—2019 and the final classification (confirmed, discarded and inconclusive), based on laboratory or clinical and epidemiological diagnosis. The area of Bahia is 564,760 km2, and the largest part of person and load transportation is made through land roads [28]. As such, Bahia is larger than countries like Spain (504,030 km2), but with a much smaller population (15,000,000). Bahia has very different climatic conditions, including areas with very different rainfall patterns, but with smaller temperature variations. This climatic heterogeneity can locally affect the vector’s life cycle and the number of arbovirus infections [29]. From these databases we extract time series of weekly number of cases (notified, confirmed or discarded for each arboviruses) in each municipality.
The Motif-synchronization (MS) between time series starts by mapping a time series into a series of motifs as illustrated in Figure 1. Motifs are patterns defined only by the relative positions of the subsequent set of points of the original series and resulting slopes represented by straight lines between them (see Figure 1A). Here we consider only a set of six motifs, labeled as
FIGURE 1. (A) Time series being converted into motifs series by mapping a set of three consecutive points of the time series into one of the 6 motifs. (B) Patterns of the 6 different adopted motifs, which depend only on the relative positions of the points in the time series. (C) A set of 5 motifs serieswhich will undergo the pairwise synchronization procedure. (D) extention of the motif series synchronization with no time lag by allowing for different delay times. The synchronization score is chosen at the delay time at which synchronization was maximum. Fonte: Adapted from [26].
Here we consider the usual point of view that, while general graph theory studies relationships between objects of any arbitrary set, networks mostly refer to a graph G(V,E) based on a set of elements of a given system. Its elements are often called nodes (or vertices) represented by
In this study, the network nodes represent the municipalities present the databases, while the edges between nodes are included whenever the correlation between the corresponding time series of weekly cases exceeds an adopted threshold. The construction of the disease networks was based on the information of the patient residence municipality and date of onset of symptoms. For the purpose of avoiding strong fluctuations, we summed the daily number of cases over 1 week and worked with number of cases per epidemiological week (EW). In addition, the final classification available in all databases was used to cast the cases into confirmed, discarded or inconclusive. In the data set used in this study, the inconclusive cases were assumed to be confirmed and added to the corresponding database. With such assumption, for each of the three diseases, three different networks were built based on the number of notified, confirmed and discarded cases, according to the following characteristics:
Notification network—constructed from the time series of reported cases of arboviruses. All patients who arrive at a healthcare unit and found suspicious to have one of the three diseases are registered in the respective database.
Confirmed network—constructed from the time series of confirmed cases. Confirmed cases identify patients who were suspicious of arbovirus that did not become discarded cases. The criteria for this classification may be clinical/epidemiological or clinical/laboratory [23].
Discarded network—constructed from the time series of discarded cases, i.e., patients who had the suspicion of that specific arbovirus discarded by specific laboratory or clinical criteria, e.g., compatibility with another disease [23].
To model the disease spreading based on the indicated database, networks were constructed based on two independent procedures: the MS between time series discussed in the previous subsection and the Time-Varying Graphs (TVG) framework. A TVG is defined [34, 35] as a set of M graphs
The methodology resulting from the combination of these two concepts has successfully been employed to build large networks based on time series related to any actual system as diverse as the brain [30, 36], economic activity [37] and disease spreading [15, 20, 38]. In this last case, as was done in the dengue networks first studied by Araújo et al [15], it may allow to the evaluation and characterization of the time necessary for the disease to disseminate in a geographical region taking into account the distance between the municipalities. In the TVG networks we work with, municipalities represent the networks nodes, while the edges between two nodes, say i and j, are introduced according to whether a previously chosen threshold value is smaller than the largest correlation value
For the sake of definitiveness, the construction of the networks proceeds along the following steps:
(i) Based on the date of first symptoms and municipality of residence, build the time series of number of cases (reported, confirmed or discarded) for each municipality i, in which each point of the time series corresponds to the number of cases of certain arbovirus on each epidemiologic week
(ii) Map each case time series into a corresponding case-motif time series, each of them with T-2 entries since motifs are defined by three successive data points and calculate within a sliding window of size LM.
(iii) For each time t, evaluate the synchronization between two case-motif series i and j over a time window of length LM =5, corresponding to five epidemiological weeks, resulting in a maximum of M = T-6 values.
(iv) Calculates the synchronization degree
(v) Insert directed edges into the M TVG networks according to whether, for each vertex pairs
The threshold
Note that, according to these requirements,
For each obtained TVG, a corresponding aggregate static network (ASN) AR can be evaluated by summing the adjacency matrices over t, i.e.:
where its elements
In order to compare typical features of spreading events caused by different arboviruses in a same time interval, or how the use of notified or confirmed databases impact the aspects drawn from the applied formalism, it is important to measure how similar the corresponding TVGs are. To this purpose we consider the definition of topological network distance [40] as a measure of the dissimilarity
where
In this section we present and discuss the most important results from our analyses which, for the sake of convenience, are casted into two main groups. The first one is related to differences in the TVG networks obtained by working with notified and confirmed cases. The second other one is related to findings from the cross-relationship among TVGs based on confirmed and discarded cases of the three arboviruses.
We start by presenting a descriptive statistic of the yearly number of confirmed and discarded cases in the databases for the three arboviruses as one expects that, the larger the number of discarded cases, the larger will be the dissimilarity between the confirmed and notified case networks for the same arbovirus. Figure 2 shows the percentages of confirmed and discarded cases with respect to the number of reported cases of each arbovirus database. They indicate that, from 2014 to 2017, the largest percentage of discarded cases occurred in the dengue database, while in 2018 and 2019 this occurred in the Zika database. So, according to the above indicated hypothesis, the largest differences are to be expected between the networks of notified and confirmed dengue cases, as we will discuss in the following subsections.
FIGURE 2. Percentage of cases discarded in the databases of each of the arboviruses for each analysed year. (A) Chikungunya. (B) Dengue. (C) Zika.
In accordance with previous works, we first consider the relationship between the average node degree of the resulting TVG network, defined as the total number of networks edges divided by the number of network nodes, and the 7-week averages of number of cases in the corresponding epidemiological week, which are displayed in Figure 4. This way it is possible to verify whether the average degree index, also referred to as connectivity, is able to detect evidences of number of cases between the municipalities. As illustrated by Figure 3 and Table 1, when working with confirmed and discarded cases for the three arboviruses we found strong correlations between the two variables, similarly to what was observed when one considers notified number of cases and corresponding average network degree [26]. This supports that the generated networks provide a good modeling of arboviruses cases for these specific databases, which is also valid for notified cases TVG. However, note that the reduction in the amount of discarded cases relative to the confirmed cases in the databases leads to a much sparser network and consequently smaller value of its connectivity, which is reflected by the slope of the least squares linear adjustment.
FIGURE 3. Graphs of the average network node degree vs. average of weekly number of cases of each arbovirus network in the corresponding TVGs. (A) Confirmed cases networks; (B) Discarded cases networks.
TABLE 1. Pearson correlation coefficient (R-Pearson) values for all panels in Figure 3.
Figure 5 shows the time evolution of the weekly number of notified, confirmed and discarded cases in the chikungunya, dengue and Zika databases. It can be directly compared with Figure 5, which displays the time evolution of the average network node degree in the corresponding TVG’s. A visual analysis reveals that the corresponding series have overall similar patterns, especially in some specific time intervals, as observed for the first chikungunya outbreak.
This can be confirmed by the Pearson correlation coefficient (R-Pearson) measured between the time series of the connectivity of notified and confirmed networks. Figure 5 shows the obtained values for the two largest outbreaks observed in 2015 and 2016, henceforth respectively identified as Outbreak 1 and Outbreak 2, indicating a strong correlation between the average degrees of these two networks for all three arboviruses.
In the case of chikungunya, it is possible to verify that the correlation in the first outbreak is larger than the in second, as suggested by the visual analysis of Figure 5. There we also note that the connectivity of confirmed case networks is smaller than that of the notified cases, which stays in accordance with the smaller number of cases in Figure 4A. The same feature is observed when we compare the very small number of discarded chikungunya cases with the corresponding very sparse network in Figures 4A, 5A. As of dengue results, the comparison of the connectivity of notified and confirmed networks in Figure 5B shows that they also follow a similar pattern in both outbreaks, which is confirmed by Figure 6. Furthermore, this can be visually identified by comparing the number of notified, confirmed and discarded cases in Figure 4B. Finally, results for Zika indicate that the series of connectivity of notified and confirmed networks have very similar patterns at both outbreaks, just as occurred with dengue networks. This can be confirmed by Figure 6, which shows that the correlation of networks of notified and confirmed of Zika is high for both peaks. As observed with chikungunya, the connectivity of the discarded network is quite small, becoming almost negligible during the entire period.
FIGURE 4. Time evolution of the 7-week average number of notified, confirmed and discarded cases. (A) Chikungunya. (B) Dengue. (C) Zika. The vertical grid lines highlight the beginning of a new year.
FIGURE 5. Time evolution of the average degree of TVG to networks of notified, confirmed and discarded cases. (A) Chikungunya. (B) Dengue. (C) Zika. The vertical grid lines highlight the beginning of a new year.
FIGURE 6. Pearson correlation coefficient (R-Pearson) of the temporal evolution of the average degree of the network of notified and confirmed. (A) Outbreak 1. (B) Outbreak 2.
As detailed discussed in previous works (see [15, 26]) another important analysis to disclose aspects of how the spread of arboviruses occurs in a given region amounts to identifying the relationship of delay time with the distance between municipalities. Indeed, the time delay obtained by the MS procedure (see [30]) indicates how fast the process occurs and whether it follows a diffusion like process or not. As in the previous analyses, the results strongly depend on the data accuracy. In Figure 7 we show the graphs of delay time vs. distance for the notified and confirmed networks of the three arboviruses for outbreaks 1 and 2. This way it is possible to verify whether there was a change in the resulting patterns.
FIGURE 7. Dependence of delay time vs. distance for notified and confirmed networks. (A) Chikungunya. (B) Dengue. (C) Zika.
In spite of some large differences among the notified and confirmed networks verified in the previous subsection, the corresponding comparison of the dependence between delay time and distance based on Figure 7 indicates that, despite the small quantitative changes between the results for confirmed and notified networks, the main features remain almost the same.
To obtain further insights on disease spread provided by the adopted framework, we evaluated the notified and confirmed ASN networks for the three arboviruses, in the periods corresponding to Outbreak 1 and 2. As discussed in Section 2.3, the ASN defined by Eq. 1. Condenses the information of the TVG during the selected time period.
A geographical display of the resulting ASN’s is shown in Figure 8, where the size of the nodes (municipalities) corresponds to the reported number of cases, and the width of the corrections is proportional to the matrix elements (??). The visual analysis of the networks in Figures 8A,C reveal, for chikungunya and Zika, small changes between the notified and confirmed ASN’s. On the other hand, the two dengue ASN’s in Figure 8B present more differences, with a notifiable smaller number of connections in the confirmed ASN. This stays in accordance with the results in Figures 4B, 5B.
FIGURE 8. Notified and Confirmed Aggregate static networks (ASN’s) corresponding to the period of the Outbreak 1 for chikungunya (A), dengue (B), and Zika (C).
A quantitative measure of the dissimilarity between the topological structures of the notified and confirmed ASN networks was evaluated using Eq. 2. In Section 2.3. The dissimilarity
In Figure 9 we show a greater dissimilarity between the dengue ASN’s of notified and confirmed for the period of Outbreaks 1 and 2. Although both the number of discarded cases and average network node degree during the Outbreak 2 are larger than those during Outbreak 1, the same is valid for the corresponding quantities of notified cases. In fact, the proportion of discarded cases and resulting average node degree with respect to those of notified cases are larger during Outbreak 1, leading to a greater dissimilarity during the first outbreak. The dissimilarities between the notified and confirmed ASN’s for chikungunya and Zika are considerably smaller than those for dengue in both periods. However, the dissimilarities in Outbreak 1 are smaller than those in Outbreak 2.
FIGURE 9. Dissimilarity between the networks of notified and confirmed of Chikungunya, Zika and Dengue.
We investigated the association between the discarded cases of dengue with those confirmed of Zika and chikungunya based on the correlation between the corresponding series of average degree of networks. The time periods including Outbreaks 1 and 2 were analyzed separately. Figure 10 highlights the used series and the considered periods.
FIGURE 10. Time series of the average degree of the networks of discarded cases of dengue and confirmed cases of Zika and chikungunya. The vertical grid lines highlight the beginning of a new year.
The obtained results are illustrated by the graphs Figure 11. In panel A we see that, for the period corresponding to Outbreak 1, the correlations are very weak, both for the networks of discarded dengue and confirmed Zika (0.22) as for those of discarded dengue and confirmed chikungunya (0.12). However, in Outbreak 2 the correlation between the series increases, becoming strong for dengue and confirmed Zika (0.79) and moderate for discarded dengue and confirmed chikungunya (0.62). The higher correlation values between dengue and Zika as compared to those between dengue and chikungunya, stay in accordance with the results found by Oliveira et al [23] based on different analyzes using the number of cases in the databases. It is possible to observe that the correlations based on a network property reproduce results from the case time series.
FIGURE 11. Pearson correlation coefficient (R-Pearson) of the temporal evolution of the networks of discarded dengue and confirmed Zika and chikungunya (A) Outbreak 1. (B) Outbreak 2
This work aimed at using a network formalism to investigate the implications of false diagnoses in understanding the spread of the three co-circulating arboviruses in a given region. We used databases of notified, confirmed and discarded cases in the state of Bahia, Brazil, to setup networks and establish comparisons between different networks for one same arbovirus and/or for distinct arbovirus. We presented quantitative results both for disease time evolution based on TVG network sets and for ASN networks obtained from the former. They are expressed in terms of their network connectivity, connectivity correlations between two different series, and the dissimilarity between ASN networks. More detailed analyses highlight two periods in 2015 and 2016 when two large outbreaks occurred. The used databases indicate that dengue, arbovirus that circulates for a much longer time in Bahia, usually has the largest number of notified, confirmed and discarded cases.
Being the arbovirus with largest number of discarded cases, dengue also has the corresponding network with largest connectivity. This is easy to identify during both outbreaks, particularly during Outbreak 1. For the same networks of the other cases, the connectivity is negligible during Outbreak 1, but has a substantial increase in Outbreak 2. Consistently, the same pattern is observed for the difference in the connectivity between the notified and confirmed networks, namely: large differences for dengue in both periods and, for Zika and chikungunya, much smaller and negligible differences in Outbreaks 2 and 1.
We emphasize that the comparison between networks of different arbovirus found in this work indicates that, for discarded dengue cases, the main connectivity peak in Outbreak 1 is shifted backwards in relation to those of confirmed cases of Zika and chikungunya. This time lag explains the weak correlation between the corresponding series for the mean network node degree, in opposition to what could be expected. Nevertheless, it should be reminded that the Zika virus was identified only in May 2015 [42], when the discarded dengue peak was about to end. There were less wrong dengue diagnoses during Outbreak 2 but stronger correlations among the connectivity of discarded dengue networks with those of confirmed Zika and chikungunya.
By taking into account all TVG networks in a properly chosen interval, the dissimilarity values are quite useful to provide information for the average behavior. Here the values found for the dissimilarity between the ASN’s of notified and confirmed cases are in accordance with the commented results from correlation analysis. The greater the number of discarded cases, the greater the dissimilarity between the corresponding networks. In spite of that, our results for the dependence between time delay and distance between municipalities for notified and confirmed cases are very similar, indicating that the network dissimilarity has a relatively small influence on the phenomenon arboviruses in the state as they were made. This important finding provided by comparison between two network based approaches does not eliminate the concern with wrong diagnoses, to ensure adequate therapeutic approach to patients and obtain more accurate incidence estimates of each arbovirus.
Our correlation results based on network connectivity are in accordance with previous findings of correlation analyses between notified, confirmed and discarded cases of chikungunya, dengue, and Zika in the whole Brazilian territory [23]. In particular, the higher correlation between the connectivity of discarded dengue networks with Zika as compared to that between dengue and chikungunya. Although our results cannot claim causality, this correlation shows that discarded cases of dengue can actually correspond to cases of Zika and, in a smaller number, to cases of chikungunya. But above all, we show that the used network approach is consistent with analyses performed on actual series of cases.
Such association between discarded and confirmed is supported by similarity and differences in clinical symptoms that may lead to wrong diagnosis, as discussed by Vu et al [8]. They suggest that, in the case of chikungunya, the very frequent presence of intense arthralgia usually causes the first suspicion to prove correct. However, in the absence of such symptom and the lack of knowledge of this disease, chikungunya may be wrongly diagnosed as dengue, as was specially the case just after the introduction of this virus in 2014 [2, 43]. Thus, this may actually be the case corresponding to a small part of the discarded cases of dengue, as indicated in [23]. Since the first laboratory confirmed cases of Zika in Bahia occurred only by the end of the first half of 2015 [44], its early symptoms were confused with those dengue and the serodiagnosis may present a result of cross-reaction, since both are flaviruses [45], these aspects support the previous findings based on a vector autoregressive models (VAR) [23] that discarded cases of dengue corresponded actually to Zika cases.
Publicly available datasets were analyzed in this study. This data can be found here: https://datasus.saude.gov.br/transferencia-de-arquivos/.
Conceptualization, RA, JM, and ES; Study design and methodology: RA, JM, ES, and MT; Provided data: MT, MC, LS, and LC; Implemented the study and carried statistical analyses: ES and RA; Discussed and interpreted the analyses: ES, RA, JM, and HB; First draft of the manuscript: ES, RA, JM, and HS. All authors read and contributed with the manuscript. All authors reviewed the final manuscript and agreed to the published version of the manuscript.
This work is supported by the Center of Data and Knowledge Integration for Health (CIDACS) through the Zika Platform- a long-term surveillance platform for the Zika virus and microcephaly, Unified Health System (SUS)—Brazilian Ministry of Health. ESP is funded by the Wellcome Trust (Grant Number 213589/Z/18/Z). The work was partially supported by the National Council of Technological and Scientific Development, CNPq, Brazil (JGVM: Grant number 307828/2018-2; HS: Grant numbers 431990/2018-2 and 313423/2019-9; RFSA: Grant numbers 422561/2018-5 and 304257/2019-2). The funders had no role in study design, data collection and analysis, decision to publish, or preparation of the manuscript.
The authors declare that the research was conducted in the absence of any commercial or financial relationships that could be construed as a potential conflict of interest.
All claims expressed in this article are solely those of the authors and do not necessarily represent those of their affiliated organizations, or those of the publisher, the editors and the reviewers. Any product that may be evaluated in this article, or claim that may be made by its manufacturer, is not guaranteed or endorsed by the publisher.
MS, Motif-Synchronization; ASN, Aggregate Static Network.
1.World Health Organization. Dengue guidelines for diagnosis, treatment, prevention and control. New edition. Geneva, Switzerland: World Health Organization (2009). p. 160. Psychiatric News.
2. De Souza LJ. Dengue, Zika e Chikungunya – diagnóstico, Tratamento e Prevenção. Rio De Janeiro: Editora Rubio (2016). p. 204.
3. Galán-Huerta KA, Rivas-Estilla AM, Martinez-Landeros EA, Arellanos-Soto D, Ramos-Jiménez J. The Zika virus disease: An overview. Medicina Universitaria (2016) 18(71):115–24. doi:10.1016/j.rmu.2016.05.003
4. Krauer F, Riesen M, Reveiz L, Oladapo OT, Porgo V, Haefliger A, et al. Zika virus infection as a cause of congenital brain abnormalities and Guillain – barre syndrome : Systematic review. Plos Med (2017) 14:e1002203.
5. Luz KG, dos Santos GIV, de Magalhães Vieira R. Febre pelo vírus Zika. Epidemiol Serv Saude (2015) 24(4):785–8. doi:10.5123/s1679-49742015000400021
6. Paixão ES, Teixeira MG, Rodrigues LC. Zika, chikungunya and dengue: The causes and threats of new and reemerging arboviral diseases. BMJ Glob Health (2018) 3:e000530. doi:10.1136/bmjgh-2017-000530
7. Pialoux G, Gaüzère B-A, Jauréguiberry S, Strobel M. Chikungunya, an epidemic arbovirosis. Lancet Infect Dis (2007) 7(5):319–27. doi:10.1016/s1473-3099(07)70107-x
8. Vu DM, Jungkind D, LaBeaud AD. Chikungunya virus. Clin Lab Med (2017) 37(2):371–82. doi:10.1016/j.cll.2017.01.008
9. Almeida LS, Cota ALS, Rodrigues DF. Saneamento, arboviroses e determinantes ambientais: Impactos na saúde urbana. Cienc saude coletiva (2020) 25(10):3857–68. doi:10.1590/1413-812320202510.30712018
10. de Assis Mendonça F, e Souza AV, de Almeida Dutra D. Saúde pública, urbanização e dengue no Brasil. Soc Nat (2009) 21(3):257–69. doi:10.1590/s1982-45132009000300003
11. Malik HAM, Abid F, Wahiddin MR, Waqas A. Modeling of internal and external factors affecting a complex dengue network. Chaos Solitons & Fractals (2021) 144:110694. doi:10.1016/j.chaos.2021.110694
12. Malik HAM, Abid F, Mahmood N, Wahiddin MR, Malik A. Nature of complex network of dengue epidemic as a scale-free network. Healthc Inform Res (2019) 25(3):182. doi:10.4258/hir.2019.25.3.182
13. Saba H, Vale VC, Moret MA, Miranda JGV. Spatio-temporal correlation networks of dengue in the state of Bahia. BMC Public Health (2014) 14:1085–9. doi:10.1186/1471-2458-14-1085
14. Saba H, Moret MA, Barreto FR, Araújo MLV, Jorge EMF, Nascimento Filho AS, et al. Relevance of transportation to correlations among criticality, physical means of propagation, and distribution of dengue fever cases in the state of Bahia. Sci Total Environ (2018) 618:971–6. doi:10.1016/j.scitotenv.2017.09.047
15. Araújo MLV, Miranda JGV, Sampaio R, Moret MA, Rosário RS, Saba H. Nonlocal dispersal of dengue in the state of Bahia. Sci Total Environ (2018) 631:40–6. doi:10.1016/j.scitotenv.2018.02.198
16. Malik HAM, Mahesar AW, Abid F, Waqas A, Wahiddin MR. Two-mode network modeling and analysis of dengue epidemic behavior in Gombak, Malaysia. Appl Math Model (2017) 43:207–20. doi:10.1016/j.apm.2016.10.060
17. Malik HAM, Abid F, Wahiddin MR, Bhatti Z. Robustness of dengue complex network under targeted versus random attack. Complexity (2017) 2017:1–12. doi:10.1155/2017/2515928
18. Massad E, Ma S, Chen M, Struchiner CJ, Stollenwerk N, Aguiar M. Scale-free network of a dengue epidemic. Appl Maths Comput (2008) 195(2):376–81. doi:10.1016/j.amc.2007.04.102
19. Li Q, Cao W, Ren H, Ji Z, Jiang H. Spatiotemporal responses of dengue fever transmission to the road network in an urban area. Acta Tropica (2018) 183(2017):8–13. doi:10.1016/j.actatropica.2018.03.026
20. Prabodanie RAR, Stone L, Schreider S. Spatiotemporal patterns of dengue outbreaks in Sri Lanka. Infect Dis (2020) 52(5):350–60. doi:10.1080/23744235.2020.1725108
21. Srivastav AK, Yang J, Luo XF, Ghosh M. Spread of zika virus disease on complex network—a mathematical study. Math Comput Simul (2019) 157:15–38. doi:10.1016/j.matcom.2018.09.014
22. Donalisio MR, Freitas ARR, Von Zuben APB. Arboviruses emerging in Brazil: Challenges for clinic and implications for public health. Rev Saude Publica (2017) 51:30–5. doi:10.1590/s1518-8787.2017051006889
23. Oliveira JF, Rodrigues MS, Skalinski LM, Santos AES, Costa LC, Cardim LL, et al. Interdependence between confirmed and discarded cases of dengue, chikungunya and zika viruses in Brazil: A multivariate time-series analysis. PLoS One (2020) 15(2):e0228347–13. doi:10.1371/journal.pone.0228347
24. Ioos S, Mallet HP, Leparc Goffart I, Gauthier V, Cardoso T, Herida M. Current Zika virus epidemiology and recent epidemics. Medecine et Maladies Infectieuses (2014) 44(7):302–7. doi:10.1016/j.medmal.2014.04.008
25. Oidtman RJ, España G, Perkins TA. Co-circulation and misdiagnosis led to underestimation of the 2015–2017 Zika epidemic in the Americas. Plos Negl Trop Dis (2021) 15(3):e0009208. doi:10.1371/journal.pntd.0009208
26. Santos ES, Miranda JGV, Saba H, Skalinski LM, Araújo MLV, Veiga RV, et al. Complex network analysis of arboviruses in the same geographic domain: Differences and similarities (2022). Submitt to Chaos, Solitons Fractals.
27.Governo do Brasil. Ministério da Saúde. Departamento de Informática do SUS. Transferência de Arquivos. (2022). Available from: https://datasus.saude.gov.br/transferencia-de-arquivos/ (Accessed January 10, 2022).
28.Instituto Brasileiro de Geografia e Estatística (IBGE). Território e ambiente (2021). Available from: https://cidades.ibge.gov.br/brasil/ba/panorama (Accessed January 10, 2022).
29. Filho ASN, Murari TB, Ferreira P, Saba H, Moret MA. A spatio-temporal analysis of dengue spread in a Brazilian dry climate region. Sci Rep (2021) 11(1):11892–8. doi:10.1038/s41598-021-91306-z
30. Rosário RS, Cardoso PT, Muñoz MA, Montoya P, Miranda JGV. Motif-synchronization: A new method for analysis of dynamic brain networks with eeg. Physica A: Stat Mech its Appl (2015) 439:7–19. doi:10.1016/j.physa.2015.07.018
31. Barabási AL. Network science. Available at: http://barabasi.com/networksciencebook/ (Accessed January 10, 2022).
32. Newman MEJEJ. The structure and function of complex networks. SIAM Rev (2003) 45(2):167–256. doi:10.1137/s003614450342480
33. Watts DJ, Strogatz SH. Collective dynamics of “small-world” networks. Nature (1998) 393(6684):440–2. doi:10.1038/30918
34. Tang J, Scellato S, Musolesi M, Mascolo C, Latora V. Small-world behavior in time-varying graphs. Phys Rev E (2010) 81(5):055101–4. doi:10.1103/physreve.81.055101
35. Nicosia V, Tang J, Musolesi M, Russo G, Mascolo C, Latora V. Components in time-varying graphs. Chaos (2012) 22(2):023101.
36. Stefano Filho CA, Attux R, Castellano G. Can graph metrics be used for EEG-BCIs based on hand motor imagery? Biomed Signal Process Control (2018) 40:359–65. doi:10.1016/j.bspc.2017.09.026
37. de Barros Pereira HB, do Rosário RS, de Area Leão Pereira EJ, Moreira DM, Ferreira P, Miranda JGV. Network dynamic and stability on European Union. Phys A Stat Mech Its Appl (2022) 587:126532. doi:10.1016/j.physa.2021.126532
38. Saba H, Nascimento Filho AS, Miranda JGV, Rosário RS, Murari TB, Jorge EMF, et al. Synchronized spread of COVID-19 in the cities of Bahia, Brazil. Epidemics (2022) 39(2021):100587. doi:10.1016/j.epidem.2022.100587
39. Netto M. MoSyn. GitHub Repos (2022). Available from: https://github.com/mpnetto/MoSyn.git (Accessed January 10, 2022).
40. Andrade RFS, Miranda JGV, Pinho STR, Lobão TP. Measuring distances between complex networks. Phys Lett A (2008) 372(32):5265–9. doi:10.1016/j.physleta.2008.06.044
41. Andrade RFS, Miranda JGV, Lobão TP. Neighborhood properties of complex networks. Phys Rev E (2006) 73(4):046101–5. doi:10.1103/physreve.73.046101
42. Campos GS, Bandeira AC, Sardi SI. Zika virus outbreak, bahia, Brazil. Emerg Infect Dis (2015) 21(10):1885–6. doi:10.3201/eid2110.150847
43. de Andrade Zanotto PM, de Cerqueira Leite LC. The challenges imposed by dengue, zika, and chikungunya to Brazil. Front Immunol (2018) 9(8):1964. doi:10.3389/fimmu.2018.01964
44. Fantinato FFST, Araújo ELL, Ribeiro IG, de Andrade MR, de Medeiros Dantas AL, Rios JMT, et al. Description of the first cases of Zika virus fever investigated in municipalities of the Brazilian Northeastern Region, 2015. Epidemiol Serv Saude (2016) 25(4):683–90. doi:10.5123/s1679-49742016000400002
Keywords: complex networks (CNs), disease spread analysis, dengue, Zika, chikungunya
Citation: Santos ES, Miranda JGV, Saba H, Skalinski LM, V. Veiga R, Costa MdCN, Cardim LL, Paixão ES, Barreto ML, Teixeira MG and Andrade RFS (2022) Network analysis of spreading of dengue, Zika and chikungunya in the state of Bahia based on notified, confirmed and discarded cases. Front. Phys. 10:1047835. doi: 10.3389/fphy.2022.1047835
Received: 18 September 2022; Accepted: 28 November 2022;
Published: 12 December 2022.
Edited by:
Leonardo B. L. Santos, Cemaden, BrazilReviewed by:
Sabrina Camargo, Center for Complex Systems and Brain Sciences (CEMSC3), ArgentinaCopyright © 2022 Santos, Miranda, Saba, Skalinski, V. Veiga, Costa, Cardim, Paixão, Barreto, Teixeira and Andrade. This is an open-access article distributed under the terms of the Creative Commons Attribution License (CC BY). The use, distribution or reproduction in other forums is permitted, provided the original author(s) and the copyright owner(s) are credited and that the original publication in this journal is cited, in accordance with accepted academic practice. No use, distribution or reproduction is permitted which does not comply with these terms.
*Correspondence: Roberto F. S. Andrade, cmFuZHJhZGVAdWZiYS5icg==
†These authors have contributed equally to this work
Disclaimer: All claims expressed in this article are solely those of the authors and do not necessarily represent those of their affiliated organizations, or those of the publisher, the editors and the reviewers. Any product that may be evaluated in this article or claim that may be made by its manufacturer is not guaranteed or endorsed by the publisher.
Research integrity at Frontiers
Learn more about the work of our research integrity team to safeguard the quality of each article we publish.