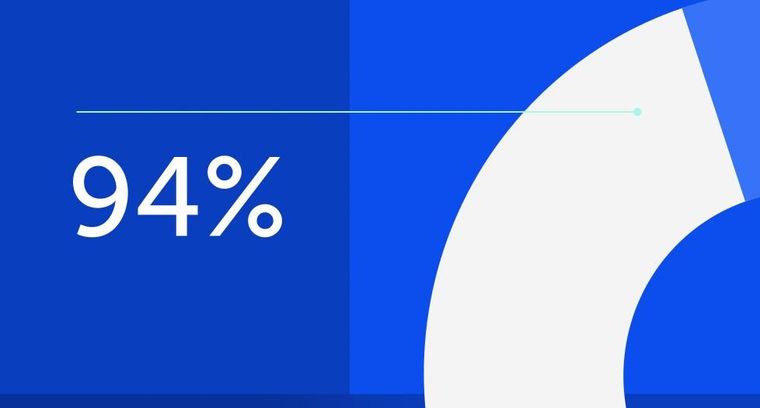
94% of researchers rate our articles as excellent or good
Learn more about the work of our research integrity team to safeguard the quality of each article we publish.
Find out more
REVIEW article
Front. Phys., 18 October 2022
Sec. Physical Acoustics and Ultrasonics
Volume 10 - 2022 | https://doi.org/10.3389/fphy.2022.1044890
This article is part of the Research TopicAdvances in Noise Reduction and Feature Extraction of Acoustic SignalView all 13 articles
Future naval warfare has placed high demands on underwater targets’ target detection, target recognition, and opposition resistance, among other things. However, the ocean’s complex underwater acoustic environment and the evolving “stealth” technology of underwater targets pose significant challenges to target detection systems, which has become a hot topic in the field of underwater acoustic signal processing in various countries. This study introduced the mechanism of underwater target radiation noise generation, analyzed the research progress and development of underwater target radiation noise recognition by applying machine learning from three perspectives: signal acquisition, feature extraction, and signal recognition at home and abroad, and elaborated on the challenges of underwater target-radiated noise recognition technology against the backdrop of rapid computing science development, and finally, an integrated signal processing method based on the fusion of traditional feature extraction methods and deep learning is proposed for underwater target radiation noise recognition, which improves the low recognition rate of traditional methods and also circumvents the problem of deep learning requiring high computational cost, and is an important direction for future hydroacoustic signal processing.
The ocean will be the primary battlefield of future high-tech warfare, and future naval weaponry will inevitably include information and intelligence. With the advancement of equipment countermeasure and counter-countermeasure technology, the complete application of underwater acoustic countermeasure and counter-countermeasure technology in recent battles has demonstrated its value in naval combat. Target detection and target recognition refer to the use of signal processing technology for underwater acoustic signal processing in order to complete target detection, parameter estimation, and target recognition, as well as other military tasks, in the complex marine battlefield environment of underwater acoustic countermeasure and counter-countermeasure. Target recognition is also the foundation of underwater acoustic countermeasure and counter-countermeasure, and many subsequent tasks can only be completed by precisely recognizing hostile targets.
The future of naval warfare places great demands on underwater targets’ target detection, target recognition, and anti-resistance capabilities, among other things. However, the ocean’s complex underwater acoustic environment and evolving “stealth” technology of underwater targets pose significant challenges to target detection systems, particularly for counter-countermeasure equipment, under strong noise and small aperture conditions, to detect different types of underwater target radiation noise and accurate positioning from the complex navigation noise and ocean environment noise, which has been a difficult problem of underwater target detection [1]. The reverberant background in the underwater acoustic complex environment increases the complexity of underwater acoustic active target detection, and remote detection is difficult to achieve, especially with the constant advancement of underwater acoustic countermeasure technology. At the same time, due to the complexity of the underwater target radiation noise creation and radiation mechanism, its composition is different, as is the water acoustic channel [2]. The detection system’s original good performance will not be satisfactory, and detection performance will be severely deteriorated.
This study analyzes the mechanism of underwater target radiation noise generation, then reviews the progress and challenges in underwater target radiation noise feature extraction and feature recognition, introduces the shortcomings of traditional underwater target radiation noise signal processing methods, and proposes a method combining traditional underwater target radiation noise feature extraction and deep learning, which not only improves the recognition rate but also circumvents the high computational cost.
The rest of this article is organized as follows: the mechanism of underwater target radiation noise generation is introduced in Section 2. The development trend of underwater target radiation noise recognition is introduced in Section 3. Future research directions and future prospects are introduced in Section 4. Section 5 concludes this article.
In navigation and operation, propellers and other machinery produce vibration through the shell to the water radiation acoustic waves, resulting in radiation noise. Underwater targets have a variety of distinct characteristics, and radiation noise sources are classified into three types: mechanical noise, propeller noise, and hydrodynamic noise. There are two types of radiation noise spectrum: continuous spectrum and line spectrum. Mechanical noise should be regarded as a superposition of a strong line spectrum and weak continuous spectrum, which is the main component of radiation noise in the low-frequency band. Propeller noise is made up of two components: propeller cavitation noise and propeller rotation noise. Cavitation noise is a continuous spectrum component, while propeller rotation noise is primarily expressed as a line spectrum superimposed on the continuous spectrum, and it is the main component of noise in the low-frequency band. In terms of intensity, hydrodynamic noise is frequently masked by mechanical noise and propeller noise. However, in some cases, hydrodynamic noise may become the primary noise source in the range in which the line spectrum appears. Due to high mechanical speed, the line spectrum shifts to high frequencies, and with these radiation noise differential features, underwater targets provide a theoretical basis for target recognition and estimation [3, 4].
The electric power system was once the main source of power for underwater targets in order to improve the quietness and delay the target warning time, and its main shortcoming was its range. The Swedish TP2000, the United States MK50, and the British “sailfish” have all adopted quiet thermodynamic systems that are 80 percent quieter than electric systems at the same speed, thanks to the development of closed cycle thermodynamic systems.
Underwater target radiation noise is typically generated using an excitation or vibration source, transmitted through the underwater target shell, and radiated outward through the water medium, as illustrated in Figure 1, for two types of underwater target radiation noise generation models.
The thermodynamic underwater target excitation source consists of a high-speed rotating body, a reciprocating motion body, a high-speed gear reducer, a high-speed pump, and other motion mechanisms, but its radiation noise is dependent on the vibration of the shell; as long as the thermodynamic underwater target vibration isolation method is appropriate, its radiation noise is less than that of an electric power underwater target; thus, the detection of underwater target radiation noise discrepancy is possible.
Underwater target detection and recognition technology evolves in tandem with military requirements, economic development, and the advancement of other disciplines. It is to use underwater target radiation noise as the research object and advanced signal processing technology to extract multi-directional and multi-level high-quality target difference features and then build an artificial intelligence target recognition model based on the features to achieve accurate underwater target recognition. It primarily consists of the links depicted in Figure 2.
1) Underwater target radiation noise information analysis and preferential difference feature extraction: using underwater acoustic signal processing methods, eliminate noise interference, retain information reflecting the target’s features, and then obtain the underwater target’s key information using feature extraction and preferential selection.
2) Accurate recognition of underwater target radiation noise: establish an underwater target detection and recognition method, based on an artificial intelligence model, and then determine the type of underwater targets and motion parameter information to evaluate the advantages and disadvantages of the different feature extraction methods.
Underwater target radiation noise feature extraction is an important way to realize underwater target radiation noise information representation, which lays an important foundation to realize effective underwater target radiation noise recognition, and good “extractable” features are both good separability and can maintain good similarity between the same kind, so feature extraction is important for underwater target radiation noise recognition.
In 1996, A. Passamante proposed time series characterization using the repeatability of similar sequences [6], and Chen Xiangdong et al. [7] applied the repeatability of similar sequences to underwater acoustic signal processing and then realized the feature extraction of ship-radiated noise. Meng et al. [8, 9] constructed a nine-dimensional feature vector of ship-radiated noise using statistical features, including over-zero wavelength, tachistoscopic amplitude, over-zero wavelength difference, wave train region, and wave column region. Tian et al. [10] used underwater acoustic target-radiated noise signal waveforms as features to achieve effective recognition of underwater acoustic targets. Wang et al. [11] proposed a new statistical complexity measure to achieve underwater target radiation noise recognition.
Xu Yuanchao et al. [12] rearranged the logarithmic spectrum frequency points and proposed a double logarithmic spectrum feature for ship radiation noise classification. Nan Lin et al. [13] analyzed the cepstrum features of the periodic impulse signal and ship-radiated noise and improved the non-cooperative detection performance of ship-radiated noise using a cepstrum-wavelet. Bing-Yang Wang [14] proposed a control algorithm to simulate the spectrum of ship radiation noise based on the study of the ship radiation noise characteristics, combined with the power spectrum feature. Bo-Bin Rao [15] used the Detection of Envelope Modulation on Noise (DEMON) method to obtain broadband modulation features of ship radiation noise. Shen et al. [16] used the poles of the AR model to extract the short-time frequency peak of the signal and proposed a new multiple frequency shift keying (MFSK) signal modulation recognition method using clustering features.
Chen et al. [17] implemented the signal detection of ship noise using the wavelet packet transform method. Wu Chengxi et al. [18] extracted multiple features of ship-radiated noise in frequency bands using the wavelet packet decomposition technique and improved the ship recognition accuracy. Li et al. [19] and Hong et al. [20] achieved effective recognition of underwater target-radiated noise using complete ensemble empirical mode decomposition with adaptive noise (CEEMDAN). Fang et al. [21] proposed a selective noise-assisted empirical mode decomposition method for the classification and recognition of underwater targets. Xie et al. [22] proposed a new feature extraction method in which the hybrid features of underwater target-radiated noise are extracted based on an improved variational mode decomposition method for underwater target-radiated noise recognition.
Guo Zheng et al, [23] proposed a generalized multiscale pattern erosion spectrum entropy (GMPESE) nonlinear underwater target-radiated noise feature extraction method based on the mathematical morphology method. Gaunaurd et al. [24] discussed a method for time–frequency image detection of underwater radar and sonar target echoes. Hui Junying et al. [25] combined pressure and particle velocity to propose an acoustic composite sensor for low-intensity radar noise measurement. Li et al. [26–28] carried out research on complexity analysis of time series signals, discrimination of bearing fault diagnosis, and identification of hydroacoustic target radiation noise using dispersion entropy, fractional slope entropy, and VMD, respectively, with good results, demonstrating the feasibility of entropy in the field of signal identification.
In summary, scholars have conducted a lot of research on underwater target radiation noise feature extraction and achieved gratifying results, which provide a basis for underwater target radiation noise recognition under strong background environmental noise interference and weak information extraction conditions. However, the existing feature extraction methods are closely associated with recognition methods which restricts their engineering progress, and few feature extraction methods that can be applied to actual underwater target radiation noise recognition have been reported.
Using artificial intelligence methods to input the extracted underwater target radiation noise features to achieve underwater target classification recognition is the goal of conducting underwater target radiation noise classification. A good classification model is one that does not depend on a certain feature and is applicable to many types of underwater targets, that is, robustness and generalization.
Meng et al. [29], Li et al. [30], and Ke et al. [31] achieved the recognition of underwater targets using a support vector machine model with kernel functions as radial basis functions as classifiers. Hui Jiang [32] used the Gaussian mixture model (GMM) for ship and island bird acoustic signal recognition. Wang et al. [33] used a decision tree to achieve the recognition of underwater target-radiated noise. Yang et al. [34] used an underwater acoustic signal processing model based on the gray wolf optimized kernel extreme learning machine model to achieve classification recognition of underwater target-radiated noise. Li et al. [35] calculated the recognition rate of ship-radiated noise using the K-nearest neighbor algorithm and validated the ship-radiated noise feature extraction method. Zhou et al. [36] proposed a wavelet domain-hidden Markov tree model to achieve underwater target radiation noise classification.
Luo et al. [37–39] used the normalized spectrum of the signal as input and completed the space–frequency joint detection of the line spectrum of underwater acoustic signals using a restricted Boltzmann machine. Yang et al. [40] proposed a new cooperative deep learning method for underwater acoustic target recognition by combining deep long- and short-term memory networks and deep self-coding neural networks. Satheesh et al. [41] constructed a data-efficient underwater target classifier using the generative modeling capability of an auxiliary classifier generative adversarial network to achieve underwater target radiation noise classification. Cao et al. [42] used sparse self-encoders to learn invariant features from underwater target spectra, combined with stacked autoencoders and softmax to achieve underwater target classification. Cai et al. [43–46] introduced multi-perspective light field reconstruction into the field of underwater target recognition and implemented multi-target recognition based on a generative adversarial network. Feng et al. [47] proposed a fusion feature and 18-layer residual network method to achieve the classification of underwater targets. Liu et al. [48] used a 1D-CNN model to identify the envelope-modulated line spectrum on the DEMON spectrum of underwater target radiation noise with good generalization capability. Yang et al. [49] proposed a deep neural network classification system, which can automatically learn more discriminative advanced features in the wavelet packet component energy and then realized the classification and recognition of underwater targets. Wu et al. [50] addressed the problem of small samples in underwater target recognition, a matching model with multiple target feature extraction methods, and a deep neural network model is constructed to achieve automatic, efficient, and accurate underwater target recognition.
In conclusion, researchers both at home and abroad have conducted useful investigations and achieved significant results in underwater target radiation noise recognition using artificial intelligence models. Traditional machine learning methods rely more on the signal acquisition and signal feature extraction, whereas deep learning methods have greater robustness and generalization ability, but the problems of poor physical interpretation and higher computational cost remain.
At the moment, China is aggressively implementing marine strategy, underwater target recognition technology in both military and civilian domains will be a big show, and a large number of demands will undoubtedly give rise to a large number of scientific and technological achievements output. The traditional method of underwater target radiation noise feature extraction recognition can no longer meet China’s economic and social development. Artificial intelligence has emerged as one of the strategic technologies driving China’s future scientific and technological development. Combining artificial intelligence and underwater target radiation noise recognition to provide a reliable foundation and means for underwater target radiation noise recognition will be a significant future development direction.
1) Develop more efficient deep learning models
The introduction of deep learning methods into the field of underwater acoustic signal processing has greatly improved underwater target radiation noise recognition methods. In the short term, deep learning does not appear to require the use of features such as line spectra and frequency spectra that are used in traditional underwater target radiation noise processing, because deep learning methods are a completely data-based processing method, but the characterization of its features is a serious problem; however, the excessive computational cost and poor physical interpretability severely limit the engineering applications of deep learning methods, thus, the development of deep learning frameworks with more concise computational frameworks and lower computational costs and the improvement of their physical interpretability is the next work to focus on.
2) Combining traditional machine learning methods and deep learning methods
Deep learning methods rely on a large amount of raw data, but due to the confidentiality policy, it is difficult or even impossible to obtain a large amount of underwater target radiation noise, and the data collected from semi-physical simulation tests cannot truly restore the underwater target radiation noise, although some deep learning methods can generate some feature information, but the authenticity of these feature information cannot be verified; therefore, combining traditional machine learning methods and deep learning methods, integrating and extracting a large amount of feature information under small sample conditions, and then using deep learning to improve the recognition rate of underwater target radiation noise will be an important direction for future research. The process of combining deep learning methods and traditional machine learning methods for underwater target radiation noise recognition is shown in Figure 3.
FIGURE 3. Flow chart of deep learning methods and traditional machine learning methods for underwater target radiation noise recognition.
With the continuous development of the national economy, the recognition of underwater target radiation noise has become one of the hot topics that scholars are currently concerned about the recognition of underwater target radiation noise. This study first introduced the mechanism of underwater target radiation noise generation, then analyzed the necessity of underwater target radiation noise recognition, then composed the underwater target radiation noise feature extraction methods and underwater target radiation noise recognition methods commonly used at present, and clarified the main problems and challenges of existing methods by reviewing the research progress at home and abroad, and finally, the future development direction in the field of underwater target radiation noise recognition was also analyzed to meet the demand for underwater target radiation noise recognition in the rapid development of the national economy.
Writing—original draft preparation, LZ; investigation, LX; and reviewed and edited the manuscript, WN and ZQ.
This work was supported in part by the National Natural Science Foundation of China (Nos. 52105068 and 11902236), China Postdoctoral Science Foundation (No. 2021M692509), Natural Science Basic Research Program of Shaanxi Province (No. 2021JQ-860), and Science Foundation of Xi’an Aeronautical Institute (Nos. 2020KY0223 and 2020KY1228).
LX was employed by China National Heavy Machinery Research Institute Co., Ltd.
The remaining authors declare that the research was conducted in the absence of any commercial or financial relationships that could be construed as a potential conflict of interest.
All claims expressed in this article are solely those of the authors and do not necessarily represent those of their affiliated organizations, or those of the publisher, the editors, and the reviewers. Any product that may be evaluated in this article, or claim that may be made by its manufacturer, is not guaranteed or endorsed by the publisher.
1. Yang H, Lee K-H, Choo Y, Kookhyun K. Underwater acoustic research trends with machine learning: General background. J Ocean Eng Technol (2020) 34(2):147–54. Cited in: Pubmed; PMID KJD:ART002580627. doi:10.26748/ksoe.2020.015
2. Huang T, Wang T. Research on analyzing and processing methods of ocean sonar signals. J Coastal Res (2019) 94:208–12. Cited in: Pubmed; PMID WOS:000485711600045. doi:10.2112/si94-044.1
3.Naval Weapons Engineering (2020). Introduction to naval weapons engineering. Available from: https://fas.org/man/dod-101/navy/docs/es310/asw_sys/asw_sys.htm [accessed on 4 May 2020].
4. Yang H, Lee K-H, Choo Y, Kookhyun K. Underwater acoustic research trends with machine learning: Passive SONAR applications. J Ocean Eng Technol (2020) 34(3):227–36. Cited in: Pubmed; PMID KJD:ART002596748. doi:10.26748/ksoe.2020.017
5. Yang H, Byun S, Lee K-H, Choo Y, Kookhyun K. Underwater acoustic research trends with machine learning: Active SONAR applications. J Ocean Eng Technol (2020) 34(4):277–84. Cited in: Pubmed; PMID KJD:ART002615997. doi:10.26748/ksoe.2020.018
6. Passamante A, Bromely D, Farrell ME. Time series characterization using the repeatability of similar sequences. Physica D: Nonlinear Phenomena (1996) 96(1-4):100–9. Cited in: Pubmed; PMID WOS:A1996VJ20400009. doi:10.1016/0167-2789(96)00015-2
7. Chen X, Gao X, Lu J. Research on time domain characteristics of ship radiated noise based on repeatability of similar sequences. J Southeast Univ (1998) 1998:17–23.
8. Meng Q, Yang S, Piao S. The classification of underwater acoustic target signals based on wave structure and support vector machine. The J Acoust Soc America (2014) 136(4):2265. doi:10.1121/1.4900181
9. Meng Q, Yang S. A wave structure based method for recognition of marine acoustic target signals. J Acoust Soc America (2015) 137(4):2242. doi:10.1121/1.4920186
10. Tian S, Chen D, Wang H, Liu J. Deep convolution stack for waveform in underwater acoustic target recognition. Sci Rep (2021) 11(1):9614. Cited in: Pubmed; PMID WOS:000656457900011. doi:10.1038/s41598-021-88799-z
11. Wang J, Chen Z. Feature extraction of ship-radiated noise based on intrinsic time-scale decomposition and a statistical complexity measure. Entropy (2019) 21(11):1079. Cited in: Pubmed; PMID WOS:000502145000052. doi:10.3390/e21111079
12. Xu Y, Cai Z, Kong X. Classification of ship radiated noise based on Bi-logarithmic scale spectrum and convolutional network. J Electro Inf Tech (2022) 44:1947–55. doi:10.11999/JEIT211407
13. Nan L, Han S, Liu W, Xu J. Non cooperative detection method of underwater acoustic DSSS signal under disturbed condition. Ship Electron Eng (2017) 37:46–8+58. doi:10.3969/j.issn.1672-9730.2017.09.010
14. Wang B. Spectrum control algorithm in acoustic field. Mine Warfare & Ship Self-Defence (2015) 23:54–7.
15. Rao B. Feature extraction method for weak modulation of ship radiated noise. (China: Southeast University) (2020). Thesis.
16. Shen L, Gong K, Fan L, Pan Y. MFSK signal recognition method based on the feature of local density and distance. J Signal Process (2016) 32(12):1478–88. Cited in: Pubmed; PMID CSCD:5885455. doi:10.16798/j.issn.1003-0530.2016.12.013
17. Sheng C, HongHua Z. Detection of underwater acoustic signal from ship noise based on WPT method. In: Proceedings of the Fourth International Workshop on Chaos-Fractals Theories and Applications; 2011 19-21 Oct; IWCFTA (2011). p. 324–7. Citedin: Pubmed; PMID INSPEC:12407766. doi:10.1109/iwcfta.2011.27
18. Wu C, Wang B, Xu Q, Zhu Y. Ship radiated noise recognition technology based on wavelet packet decomposition and PCA-Attention-LSTM. Tech Acoust (2022) 41:264–73. doi:10.16300/j.cnki.1000-3630
19. Li Y, Chen X, Yu J. A hybrid energy feature extraction approach for ship-radiated noise based on CEEMDAN combined with energy difference and energy entropy. Processes (2019) 7(2):69. Cited in: Pubmed; PMID WOS:000460800900015. doi:10.3390/pr7020069
20. Yang H, Li L-l, Li G-h, Guan Q-r. A novel feature extraction method for ship-radiated noise. Defence Tech (2022) 18(4):604–17. Cited in: Pubmed; PMID WOS:000793693500007. doi:10.1016/j.dt.2021.03.012
21. Niu F, Hui J, Zhao A, Cheng Y, Chen Y. Application of SN-emd in mode feature extraction of ship radiated noise. Math Probl Eng (2018) 2018:1–16. in: Pubmed; PMID WOS:000453782500001. doi:10.1155/2018/2184612
22. Xie D, Sun H, Qi J. A new feature extraction method based on improved variational mode decomposition, normalized maximal information coefficient and permutation entropy for ship-radiated noise. Entropy (2020) 22(6):620. Cited in: Pubmed; PMID WOS:000551064200001. doi:10.3390/e22060620
23. Guo Z, Zhao M, Hu C, Ni J. A study on feature extraction and application for ship radiated noise's generalized multiscale mathematical morphology feature. J Vibration Shock (2022) 41:21–8+100. doi:10.13465/j.cnki.jvs.2022.04.004
24. Gaunaurd GC, Brill D, Huang H, Moore PWB, Strifors HC. Signal processing of the echo signatures returned by submerged shells insonified by dolphin "clicks": Active classification. J Acoust Soc Am (1998) 103(3):1547–57. in: Pubmed; PMID WOS:000072522900036. doi:10.1121/1.421302
25. Hui J, Wang Y, Liang G, Li C. Research on measurement and simulation of low noise pass-through characteristics. Appl Acoust (2000) 2000:29–34.
26. Li Y, Geng B, Jiao S. Dispersion entropy-based lempel-ziv complexity: A new metric for signal analysis. Chaos, Solitons & Fractals (2022) 161:112400. Cited in: Pubmed; PMID INSPEC:21959212. doi:10.1016/j.chaos.2022.112400
27. Li Y, Mu L, Gao P. Particle swarm optimization fractional slope entropy: A new time series complexity indicator for bearing fault diagnosis. Fractal Fract (2022) 6(7):345. in: Pubmed; PMID WOS:000832093000001. doi:10.3390/fractalfract6070345
28. Li Y, Tang B, Yi Y. A novel complexity-based mode feature representation for feature extraction of ship-radiated noise using VMD and slope entropy. Appl Acoust (2022) 196:108899. Cited in: Pubmed; PMID WOS:000853020600014. doi:10.1016/j.apacoust.2022.108899
29. Meng Q, Yang S, Yu S. Recognition of marine acoustic target signals based on wave structure and support vector machine. J Electro Inf Tech (2015) 37(9):2117–23. Cited in: Pubmed; PMID CSCD:5505564.
30. Li W. Support vector machine and ship radiation noise classification. Ship Sci Tech (2017) 39:19–21. doi:10.3404/j.issn.1672–7619.2017.6A.006
31. Ke X, Yuan F, Cheng E. Integrated optimization of underwater acoustic ship-radiated noise recognition based on two-dimensional feature fusion. Appl Acoust (2020) 159:107057. Cited in: Pubmed; PMID WOS:000502894900008. doi:10.1016/j.apacoust.2019.107057
32. Jiang H. Research on target recognition method based on spectrum analysis. China: Zhejiang Ocean University (2020). Thesis.
33. Wang B, Wu C, Zhu Y, Zhang M, Li H, Zhang W. Ship radiated noise recognition technology based on ML-DS decision fusion. Comput Intelligence Neurosci (2021) 2021:2021–14. in: Pubmed; PMID WOS:000756171100004. doi:10.1155/2021/8901565
34. Yang H, Gao L, Li G. Underwater acoustic signal prediction based on MVMD and optimized kernel Extreme learning machine. Complexity (2020) 2020:1–17. in: Pubmed; PMID WOS:000533315200005. doi:10.1155/2020/6947059
35. Li Y, Jiao S, Geng B, Zhou Y. Research on feature extraction of ship-radiated noise based on multi-scale reverse dispersion entropy. Appl Acoust (2021) 173:107737. Cited in: Pubmed; PMID WOS:000595628100043. doi:10.1016/j.apacoust.2020.107737
36. Yue Z, Wei K, Qing X. A novel modeling and recognition method for underwater sound based on HMT in wavelet domain. Ai 2004: Adv Artif IntelligenceProceedings (2004) 3339:332–43. Cited in: Pubmed; PMID WOS:000226133600030. doi:10.1007/978-3-540-30549-1_30
37. Luo X, Feng Y. An underwater acoustic target recognition method based on restricted Boltzmann machine. Sensors (2020) 20(18):5399. Cited in: Pubmed; PMID WOS:000580193400001. doi:10.3390/s20185399
38. Luo X, Feng Y, Zhang M. An underwater acoustic target recognition method based on combined feature with automatic coding and reconstruction. Ieee Access (2021) 9:63841–54. . Cited in: Pubmed; PMID WOS:000645842000001. doi:10.1109/access.2021.3075344
39. Luo X, Shen Z. A space-frequency joint detection and tracking method for line-spectrum components of underwater acoustic signals. Appl Acoust (2021) 172:107609. Cited in: Pubmed; PMID WOS:000590401800007. doi:10.1016/j.apacoust.2020.107609
40. Yang H, Xu G, Yi S, Li Y. Ieee. A new cooperative deep learning method for underwater acoustic target recognition. In: Oceans 2019 - Marseille; 17-20 June 2019; Marseille, France (2019). Cited in: Pubmed; PMID WOS:000591652100423.
41. Chandran SC, Kamal S, Mujeeb A, Supriya MH. Generative adversarial learning for improved data efficiency in underwater target classification. Eng Sci Tech Int J (2022) 30:101043. Cited in: Pubmed; PMID WOS:000807389200005. doi:10.1016/j.jestch.2021.07.006
42. Cao X, Zhang X, Yu Y, Niu L. Deep learning-based recognition of underwater target. In: 2016 IEEE International Conference on Digital Signal Processing (DSP); 16-18 Oct. 2016; China (2016). p. 89–93.
43. Cai L, Luo P, Zhou G. Multistage analysis of abnormal human behavior in complex scenes. J Sensors (2019) 2019:1–10. in: Pubmed; PMID WOS:000499158000002. doi:10.1155/2019/1276438
44. Cai L, Luo P, Zhou G, Chen Z. Maneuvering target recognition method based on multi-perspective light field reconstruction. Int J Distrib Sens Netw (2019) 15(8):155014771987065–12. in: Pubmed; PMID WOS:000482086100001. doi:10.1177/1550147719870657
45. Cai L, Luo P, Zhou G, Xu T, Chen Z. Multiperspective light field reconstruction method via transfer reinforcement learning. Comput Intelligence Neurosci (2020) 2020:1–14. in: Pubmed; PMID WOS:000514358700001. doi:10.1155/2020/8989752
46. Cai L, Sun Q. Multiautonomous underwater vehicle consistent collaborative hunting method based on generative adversarial network. Int J Adv Robotic Syst (2020) 17(3):172988142092523. Cited in: Pubmed; PMID WOS:000546901500001. doi:10.1177/1729881420925233
47. Feng H, Chengwei L, Lijuan G, Feng C, Haihong F. Underwater acoustic target recognition with ResNet18 on ShipsEar dataset. In: 2021 IEEE 4th International Conference on Electronics Technology (ICET); 7th to 10th May 2021; Chengdu, China (2021). p. 1240–4. doi:10.1109/icet51757.2021.9451099in: Pubmed; PMID INSPEC:20843152
48. Liu D, Zhao X, Cao W, Wang W, Lu Y. Design and performance evaluation of a deep neural network for spectrum recognition of underwater targets. Comput Intelligence Neurosci (2020) 2020:1–11. in: Pubmed; PMID WOS:000561340500001. doi:10.1155/2020/8848507
49. Yu Y, Cao X, Zhang X. Ieee. Underwater target classification using deep neural network. In: 2018 Oceans - Mts/Ieee Kobe Techno-Oceans (Oto); 28-31 May 2018; Kobe, Japan (2018). . Cited in: Pubmed; PMID WOS:000465206800134.
Keywords: underwater acoustic target, radiated noise recognition, feature extraction, feature selection, machine learning
Citation: Zhufeng L, Xiaofang L, Na W and Qingyang Z (2022) Present status and challenges of underwater acoustic target recognition technology: A review. Front. Phys. 10:1044890. doi: 10.3389/fphy.2022.1044890
Received: 15 September 2022; Accepted: 27 September 2022;
Published: 18 October 2022.
Edited by:
Yuxing Li, Xi’an University of Technology, ChinaReviewed by:
Bo Geng, Xi’an University of Technology, ChinaCopyright © 2022 Zhufeng, Xiaofang, Na and Qingyang. This is an open-access article distributed under the terms of the Creative Commons Attribution License (CC BY). The use, distribution or reproduction in other forums is permitted, provided the original author(s) and the copyright owner(s) are credited and that the original publication in this journal is cited, in accordance with accepted academic practice. No use, distribution or reproduction is permitted which does not comply with these terms.
*Correspondence: Lei Zhufeng, bGVpemh1ZmVuZ0B4YWF1LmVkdS5jbg==
Disclaimer: All claims expressed in this article are solely those of the authors and do not necessarily represent those of their affiliated organizations, or those of the publisher, the editors and the reviewers. Any product that may be evaluated in this article or claim that may be made by its manufacturer is not guaranteed or endorsed by the publisher.
Research integrity at Frontiers
Learn more about the work of our research integrity team to safeguard the quality of each article we publish.