- 1College of Artificial Intelligence, Zhejiang College of Security Technology, Wenzhou, China
- 2WenZhou University of Technology, Wenzhou, China
Educational behavioral psychology refers to the fact that college students within campus networks have various psychological cognition toward novel information and behavior. This is hardly ever taken into account or theoretically examined in weighted network research. According to psychological traits and a student’s willingness to adopt fresh behaviors, we categorize students’ behaviors into the active and passive. On this basis, a threshold models is established for the behavior of active and passive students in weighted networks, and the influence behavioral psychology on information propagation is discussed. In order to qualitatively investigate the information propagation mechanism, a partition theory based on edge-weight and behavioral psychology is developed. Active students encourage the acceptance of new behaviors and the spread of information, according to theoretical study and simulation results. However, the phase transition intersected was more significant. When the percentage of enrolled pupils is high, a continuous phase transition is present in the growth pattern of the final adoption size. In contrast, as the proportion of active students declines, the increasing pattern alterss to discontinuous phase transition. In addition, weight distribution heterogeneity facilitates the dissemination of information and does not alter phase transition pattern. Finally, the theoretical analysis is in good agreement with the simulation results.
1 Introduction
The campus socialization of college students has been paid more and more attention as a result of the gradual improvement of higher education. In campus socialization, students’ online information dissemination is becoming more and more crucial. The information propagation theory can be used to describe a variety of behaviors of college students, including social recommendation, online learning, online entertainment, among others.
For information propagation, researchers have investigated numerous potential influences on the information propagation mechanisms in depth studies for information propagation models, including node distribution structures [1, 2], memory effects [3, 4], and heterogeneous adoption thresholds [5, 6].
Numerous research have shown that the spread of knowledge demonstrates social reinforcement or affirmation [7, 8]. One of the traditional models for describing social reinforcement is the threshold model based on non-Markov processes, in which the adoption of individual behavior demonstrates memory effects [9]. Additionally, a number of threshold models that are accurate to the network have been proposed to test their influence on information transmission, including binary adoption probability [10], truncated normal adoption probability [11], gate-like adoption probability [12], and sigmoid adoption probability [13]. It is worth noting that in the course of information propagation, the non-redundant feature, i.e., the prohibition of repetition of information propagation at the same edge, cannot be ignored.
The distribution of information is also greatly impacted by the variability of individual closeness in genuine social networks. People are more likely to obtain information from their friends and family than from complete strangers. The influence of intimacy heterogeneity on information dissemination was confirmed by the researchers, who built the connective links between people as edges with diverse weight distribution [14, 15].
Existing literature has suggested that populations are heterogeneous because different people have different attitudes toward the same action, for example [16–18]. Behavioral psychology is rarely considered in college educational studies. On campus, for example, students’ psychological heterogeneity is common. College students often send and receive messages on social networks, and their adoption behavior can be influenced by personal psychology. Some students are very interested in new information and are willing to adopt and disseminate it when the information around them is not universally accepted. However, some students are more passive. They are willing to adopt information only when there are more adoptors around them. They are always hesitant about popular behavior, verifying information multiple times before adopting it. Therefore, according to the students’ different psychological factors, they can be divided into active and passive individuals. Psychologically active students become interested in their own behavior once they have access to information. They gradually increase their willingness to accept behavior as they learn more information. But students with passive psychology will verify this information several times before taking action. In other words, a student’s psychology has something to do with the likelihood that they would pick up a new behavior on campus.
Taking these factors into account, we investigated the effects of behavioral heterogeneity on weighted network information propagation. Only a small percentage of p students were active, while others were passive. To capture the behavioral variability of students, two adoption threshold probability functions that correspond to active and passive students are presented. To further examine the information propagation mechanism, a partition theory based on edge-weight and population heterogeneity is developed. Finally, the simulation outcomes demonstrate that the predictions of the theory are consistent with the information transmission under behavior heterogeneity. This article’s remaining sections are organized as follows. The second section establishes an information transmission model that makes use of behavior heterogeneity on weighted networks. A partition theory based on edge-weight and behavior heterogeneity is demonstrated in Section 3. Section 4 of the report discusses the experiment’s findings. Finally, Section 5 presents the conclusions.
2 Propagation model with behavioral heterogeneity
We build a weighted social network model with N individuals and a degree distribution of p(k) to investigate the impact of population heterogeneity on the mechanism for information propagation. Our methodology uses the uncorrelated configuration model to prevent intra-degree correlations. A generalized SAR (susceptible-adopted-recovered) model to depict the information propagation mechanism in weighted social network models is shown in Figure 1. Each node in the SAR model is constantly in one of three states: the sensitive state (S-state), the adopted state (A-state), and the restored state. The S-state node is able to communicate with its neighbors and does not adopt this behavior. This behavior has been adopted by A-state nodes, and they are eager to spread information among their neighbors. R-state nodes stop taking an active interest in behavior and stop spreading information.
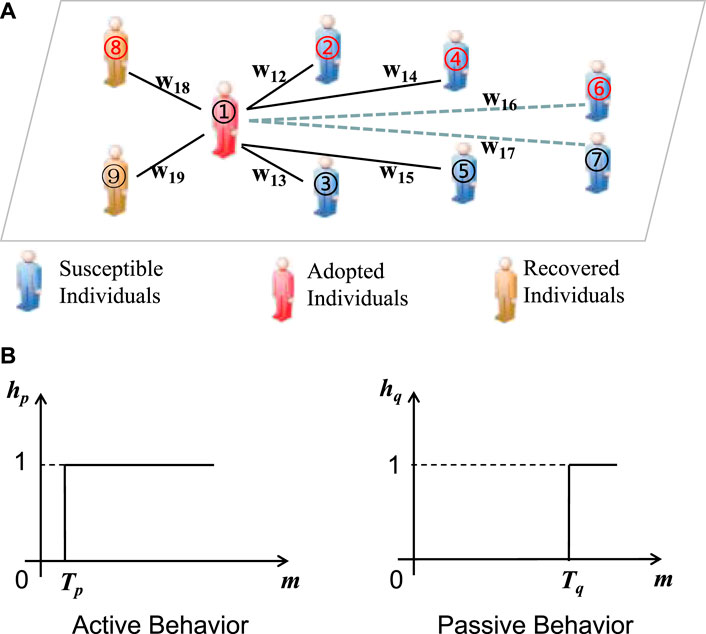
FIGURE 1. (A) SAR model description on weighted networks. Red labels represent active students, such as Nos. 2, 4, 6 and 8. Black labels represent passive students such as students 3, 5, 7, 9. The symbol ω is edge-weight. At t, A-State Student 1 disseminates information to his/her classmates or friends. Information cannot be transferred over the edge, as indicated by the dotted lines. Information is not sent across the corresponding borders of solid lines, as indicated. (B) The left subgraph shows the behavior of active students using a threshold model. The right subgraph is a threshold threshold model passive student behavior adoption. mis the sum of all the information that S-level students have successfully obtained. pand qare the scores of active and passive students, respectively.
Additionally, edges with a weight distribution are used to indicate individual correlations. Then, in order to reflect the heterogeneity of edge, the distribution of edge weights is introduced. The edge-weight distribution is denoted by a function f(ω), and the edge-weight between two neighboring nodes i and j is denoted by the symbol ωij. Indicate the likelihood that an S-state node will learn something from its A-state neighbor node by using the following notation:
where β is the probability of propagation per unit of information. If ωij = 1, it is demonstrated by λω = β that edge weight has no impact on the transmission of information. Additionally, λ(ωij) increases monotonically as ωij increases.
Let m represent the total amount of data that the S-state node has successfully received. In weighted social networks, information does not initially spread because mj = 0 (j for the S-state node). The total number of the node j’s message blocks increases by one at each step, mj right arrow mj + 1, following the successful receipt of a message from the A-state neighbor I I across the relevant edge.
Additionally, two functions are suggested to illustrate the threshold for individual behavior in order to describe the effects of behavior heterogeneity on the dissemination of information, as shown in Figure 1. The function that depicts the behavior adoption threshold for active students is denoted by
The behavior adoption threshold function for conservatives is represented by
only a small percentage of students with p are active, and only a small percentage of students with q are passive. p + q = 1. In fact, for active students, they are a very high willing to adopt the behavior. When small messages are received, their willingness to act this way is very low. Passive students will adopt behavior only if there are more adoptors around them.
The following is a summary of the information propagation details in weighted networks. First, we chose a few of p students at random to operate as active nodes, while the remaining students served as passive nodes. Then, we choose a subset of ρ0 students at random to serve as the A-state node (seed) and all other nodes as the S-state. This information is transmitted from the A-state node to all neighbors through corresponding edge. The likelihood λ(ωij) is matched by a weight of ωij when the S-State node j successfully gets information from the A-State neighbor node i. The collection block of the message is mj → mj + 1 when the S-state node j is successful in receiving it. Information will not be repeated through the same edges due to the non-redundancy of information propagation. Additionally, with a chance of hp (m, Tp), if j is active, it adopts the behavior and changes to the A status. A state changes to A if j is passive with the probability hq (m, Tq). The A-state node loses interest in the behavior after information transmission and changes to R-state with a probability of γ. Information no longer propagates once there are no A-state nodes left in the weighted network.
3 Propagation theory based on edge-weight and behavioral heterogeneity
The study of nonredundant information memory with behavioral heterogeneity on weighted networks is based on citations. On the basis of this, this paper puts forward a theory of information partition based on behavioral heterogeneity and analyzes the mechanism of personal information dissemination. Assume that the node in a cavity state [19] implies that it can receive information from its neighbors and cannot send information externally.
The probability that the node will not get information from the following node is t because of the random distribution of the edge weights.
where the likelihood that the side of the ω weight does not spread information to its S-country neighbors is given by θw(t).
The likelihood that the S-state nodes i and ki will collectively get a m pieces communication from their neighbor at time t may be written as
Think about the threshold functions for behavior adoption and behavioral heterogeneity. If the S-state node i is active, it will still be in S-sate at time t with a likelihood of not adopting the behavior after cumulatively accepting m bits of information.
The likelihood that the quantity of the aggregate information pieces by time t is computed by for the active S-state node i is then
After receiving m bits of information cumulatively, if the S state node i is passive, it has not yet exhibited this behavior and is still in the S-state in terms of time t probability.
Following that, i and t are the probabilities of the total amount of information blocks in a passive S-state node. calculated by
The likelihood that the S-state node i receives m pieces of information and maintains S-state, then, up until time t, is given by
As a result, we can write the proportion of S-state nodes in a weighted network at time t as
We start by taking into account the θω(t) before calculating S(t). Because all nodes in a network have only three states, θω(t) can be broken down to
where the probability that the S-state node i contacts an A-state neighbor j through the appropriate edge of weight ω and has not successfully received information from the A-state node j by time t is denoted by the symbol ψA,ω(t). The chance that the S-state node i contacts a S (or R)-state neighbor j via the matching edge with weight ω is known as ψS,ω(t) (or ψR,ω(t)).
Then, we compute ψS,ω(t) first. The cavity theory’s influence prevents the node i from transmitting data to nearby nodes. Therefore, the S-state node j with kj neighbors can obtain information from those kj − 1 in addition to the node i. Consequently, the likelihood that node j will accumulate to acquire n bits of information from its surrounding nodes by time t is
Think about the threshold functions for behavior adoption and behavioral heterogeneity. If the S-state node j is active, it will still be in S-sate at time t with a likelihood of not adopting the behavior after cumulatively accepting n bits of information.
After cumulatively accepting n pieces of information, if the S-state node j is passive, it has not yet adopted the behavior and will likely still be in S-sate by time t.
Consequently, the likelihood that the node j remains in the S-state after receiving n bits of information cumulatively is
With the corresponding weight margin ω, the likelihood that the node i will link to the S-state node j is given by
where
The evolutionary equation of ψR,ω(t) and ψA,ω(t) is then examined. With a probability of λω, the S-state node i successfully takes the information from its A-state neighbor j via the appropriate edge of weight ω. Thus, θω(t) development can be described by
On the other hand, the R-state node may transition from the A-state with a probability of γ if the A-state node loses interest in information propagation. Therefore, it is possible to determine the evolution of ψR,ω(t) by
When the initial conditions θω(0) = 1 and ψR,ω(0) = 0 are combined with Eqs. 18, 19, we may obtain the evolution of ψR,ω(t)
Substituting Eq.17–20 into Eq. 12, we can obtain
Substituting Eq. 21 into Eq. 18, the evolution of θω(t) can be rewritten as
Throughout the network, the density variation of each state can be expressed as
and
So, by combination and iteration Eq.11 and 23, and Eq.24, S(t), A(t)and R(t), i.e., you can calculate the density of each state at any given time step length.
There are only S-state and R-state nodes in the network when t → ∞. The maximum size of behavior adoption is R (∞). Let
S (∞) and R (∞) can be derived by combining and iterating Eq. 11 and 25, and A (∞) = 0.
4 Results and discussions
We conduct comprehensive numerical simulations and theoretical studies on weighted Scale-Free (SF) [20] and Erdő-Rényi (ER) [21] networks, respectively, to validate the theoretical study presented above. The network size is 104; that is, there are at least 104 dynamically independent persons in the network. The network’s average degree is
In our simulations, we employed the relative variance
where the ensemble average is represented by ⟨⋯ ⟩. The key moment of information global propagation is represented by the highest values of relative variance.
4.1 Information propagation on weighted ER network
We start by investigating how information spreads on a weighted ER network. The nodes of the ER network are subject to Poisson distribution, i.e.,
In a weighted ER network, Figure 2 investigates the impact of a unit propagation probability beta on the final adaptation size of various proportions of active nodes. The initial seed percentage is rho0 = 0.01. The adoption criteria are Tp = 1 and Tq = 5, respectively. Figures 2A,B illustrate how the final adoption size, R (∞), increased to global adoption as β increased. Additionally, as the percentage of students who are actively enrolled rises, so does the eventual adoption size, or R (∞). The adoption of the behavior can be encouraged by p. There is a crossover: the R (∞) growth pattern exhibits a continuous phase transition with a sizable p component (between p = 0.5 and p = 0.8). However, the growth pattern of R (∞) shows that the phase transition is discontinuous when the p dollar is small (i.e., p = 0.2). Figure 2 3) and 4) simultaneously display the crucial propagation probabilities and relative variance of 1) and (b), respectively. Phase transition and the adoption of global behavior take place at this crucial time. Additionally, there was a rise in the weighted weighted distribution exponent earlier outbreak of information propagation outbreak as compared to subparagraphs 1) and (b). However, the phase transition pattern is unaffected by the weight distribution. The findings of the simulation accord well with the theoretical prediction (line) (symbol).
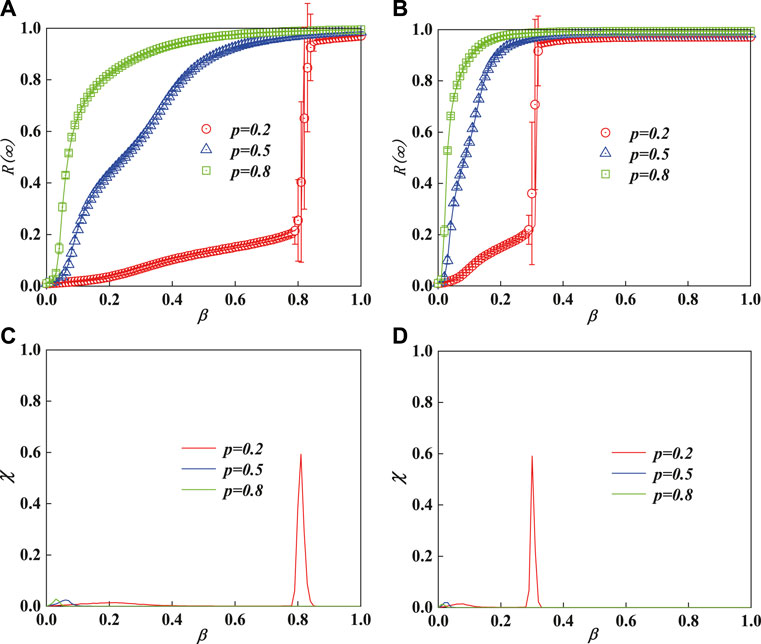
FIGURE 2. Unit propagation probability’s impact on the final adaptive size of nodes in a weighted ER network with various percentages of active nodes, in beta. The phase transition is shown to be impacted by the heterogeneity of the weight distribution in (a1) (αω = 2) and (b1) (αω = 3). The relative variances and thresholds in subparagraphs (A) and (B), respectively, are indicated in subparagraphs (C) and (D). Additionally, the theoretical predictions (dotted lines) in subparagraphs (A,B) are in good agreement with the simulation results (symbols). Other parameters are ρ0 = 0.01, Tp = 1, and Tq = 5.
The co-effect of p per fashionista and beta per unit of propagation probability is examined in Figure 3 for the weighted ER network R (∞). The effects of the weighted heterogeneity of the weight heterogeneity plane (β, p) on R (∞) are shown in Figure 3 1) (αω = 2) and 2) (αω = 3). The initial fraction of seeds ρ0 = 0.01. The adoption thresholds are Tp = 1 and Tq = 5. The crossover phenomena appears when β increases. There are three areas in the parameter plane (β, p). There is no widespread trend of adopting new behaviors in region I, and the percentage of trendy people is quite low (p). The cause of this is that students’ lack of passion to participate in information propagation during the earliest stages of information dissemination prevents information propagation. In area II, the growing pattern of R (∞) illustrates the discontinuous phase transition as the proportion of active students p increases. The growth pattern of R (∞) displays a persistent phase change in area III. In fact, the dissemination of information and the adoption of passive behavior are dominated by active students in the III district. Additionally, the weight distribution’s heterogeneity speeds up information transfer without altering the phase transition pattern.
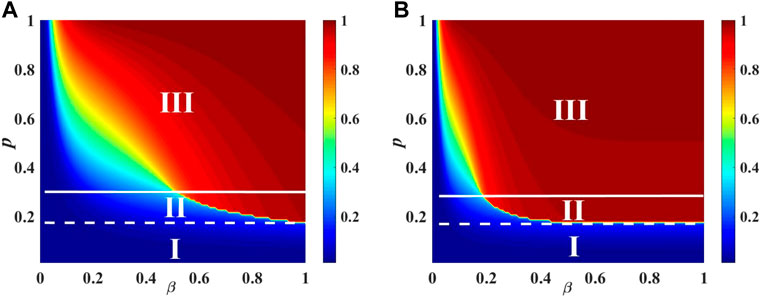
FIGURE 3. The final adoption size of a single weighted ER network is affected by the interaction of the unit propagation probability of beta and the active student part of p. There was no global behavior outbreak, discontinuous phase transition, or continuous phase transition in regions I, II, or III as described in subparagraphs (A) and (B) (αω = 2 and 3, respectively). Other parameters are ρ0 = 0.01, Tp = 1, and Tq = 5.
4.2 Information propagation on weighted SF network
The degree index v and the heterogeneity of node degree distribution in SF networks are negatively associated. Nodes’ degree follows a power-law distribution. The degree exponent of the SF network is presented by equation p(k) = ξk−v, where ξ = 1/∑kk−v. The minimum and maximum degrees are, respectively, kmin = 4 and kmax ∼ 100.
Figure 4 illustrates the effect of unit propagation probability of β and active student portion of p on individual final adoption size in weighted SF networks with degree distribution heterogeneity. the vertical subgraphs utilizes the same degree distribution index, i.e. the subgraph from first column to third column corresponds to v = 2, 4. The initial fraction of seeds ρ0 = 0.01. The weight distribution exponent αω = 2. The adoption criteria are Tp = 1 and Tq = 5, respectively. With the increase of beta, R (∞) becomes more widely used. Additionally, p encourages the adoption of the behavior. The growth pattern of the final adoption size exhibits a continuous and phase transition with the increase in the percentage of active students. Additionally, when subgraphs 3) and 4) are compared, it can be seen that degree distribution heterogeneity facilitates information dissemination without changing the growth patterns that ultimately result in adoption size. Additionally, in Figure 4A, the global adoption size increases with the percentage of enrolled students when the degree distribution index is 2.
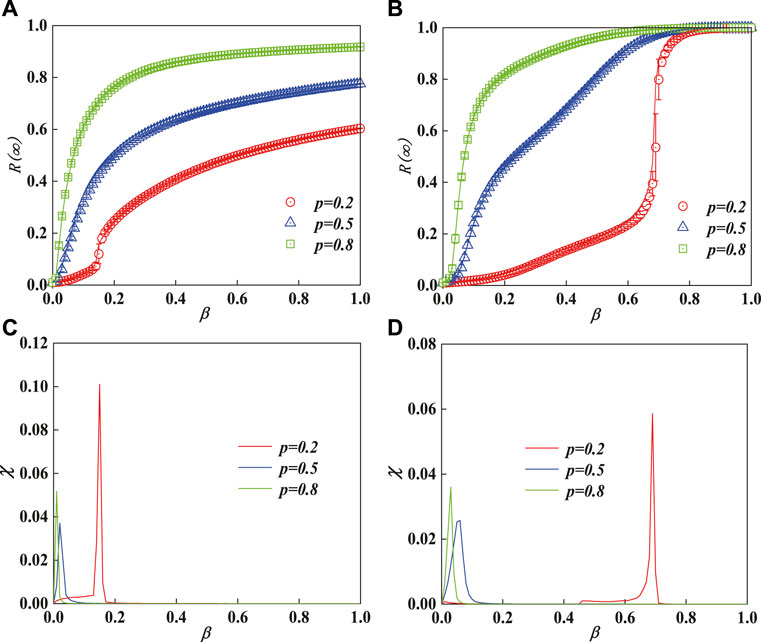
FIGURE 4. Effect of β per unit propagation probability and p active student portion on the final adoption size of weighted SF network individuals. the vertical subgraphs utilizes the same degree distribution index, i.e., the subgraph from first column to third column corresponds to v = 2, 4. Subgraphs (A) and (B) present the impact of β and p on the size of final individual adoptions with degree distribution heterogeneity. Figure (C) and (D) present the relative variances and thresholds for (A,B), respectively. Theoretical analysis (dotted line) is in good agreement with simulation values (symbol). Other parameters are ρ0 = 0.01, αω = 2, Tp = 1, and Tq = 5.
For a weighted SF network with v = 2, 4, respectively, Figures 5A,B examine the fluctuation of R (∞) on the information propagation parameter plane (β, p). The initial seed percentage is ρ0 = 0.01. Alpha αω = 2, which is the exponent of weight heterogeneity. The adoption criteria are Tp = 1 and Tq = 5, respectively. Phase transitions occur as a result of the crossover phenomenon when beta increases. Even more exciting, when the degree distribution heterogeneity is highly heterogeneous, at v = 2, the eventual size of individual adoptions rises slowly as the proportion of students in work increases. But when the degree distribution heterogeneity is less heterogeneous, at v = 4, the eventual size of individual adoptions increases rapidly with the proportion of active students. In Zone I, there has been no global outburst behavior adoption. In region II, the growth model of R (∞) exhibits a sequential phase transition in phase II. In fact, in the II District (Figures 5A,B), active students dominate the dissemination of information and stimulate the adoption of passive behavior.
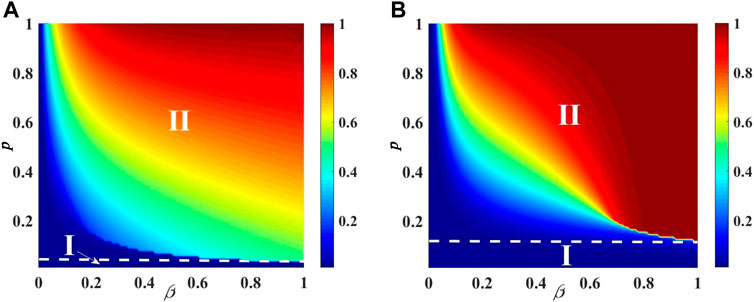
FIGURE 5. The combined impact of active student score and unit propagation probability on the final adoption size of weighted SF network users. The effects of (β, p) on the ultimate adoption size at v = 2.4 are shown in subparagraphs (A) and (B), respectively. In (A,B), two regions appeared: region I experienced a sustained phase shift while region II did not experience a worldwide behavior outbreak. Other parameters are ρ0 = 0.01, αω = 2, Tp = 1, and Tq = 5.
5 Conclusion
This paper discusses the influence of behavioral psychology on the dissemination on campus sociality. We randomly select a small percentage of p students as active, while others were passive. We also take individual intimacy heterogeneity into account, which is depicted in the campus network as the edge-weight. Then, in order to demonstrate the behavioral psychology, we provide two adoption threshold functions. For active students, they are willing to receive and disseminate information if the information around them is not universally accepted. But passives are willing to adopt information if only when there are more adoptors around them. They are always hesitant about popular behavior, verifying information multiple times before adopting it. A threshold model based on edge weight and heterogeneity is suggested to conceptually investigate the impacts of psychology heterogeneity. We discover several fascinating information transmission phenomena through theoretical investigation and simulation findings. First, engaged students encourage the spread of knowledge and the adoption of new behaviors. The phase transition crossover phenomenon also manifests. The growth pattern of R (∞) changes has transitioned from a discontinuous phase to a continuous phase with an increase in p. In addition, the distribution of weights facilitates the dissemination of information without altering the pattern of phase transition pattern. Despite being essential to the spread of information, behavioral psychology lacks thorough theoretical modeling and study. The effects of behavior psychology on weighted work are modeled and analyzed qualitatively and quantitatively. By considering behavior psychology on weighted networks, this paper reveals the intrinsic mechanism of campus social.
Data availability statement
The original contributions presented in the study are included in the article/supplementary material, further inquiries can be directed to the corresponding author.
Author contributions
JY and YC designed and performed the research and wrote the manuscript.
Conflict of interest
The authors declare that the research was conducted in the absence of any commercial or financial relationships that could be construed as a potential conflict of interest.
Publisher’s note
All claims expressed in this article are solely those of the authors and do not necessarily represent those of their affiliated organizations, or those of the publisher, the editors and the reviewers. Any product that may be evaluated in this article, or claim that may be made by its manufacturer, is not guaranteed or endorsed by the publisher.
References
1. Duan B, Chen H, Sun L, Tang Q, Tian S, Xie M. Identifying influential spreaders in complex networks by propagation probability dynamics. Chaos (2019) 29(3):033120. doi:10.1063/1.5055069
2. Pang S, Wen X, Fei H. Controllability limit of edge dynamics in complex networks. Phys Rev E (2019) 100(2):022318. doi:10.1103/physreve.100.022318
3. Williams OE, Lillo F, Latora V. Effects of memory on spreading processes in non-markovian temporal networks. New J Phys (2019) 21(4):043028. doi:10.1088/1367-2630/ab13fb
4. Dodds P, Watts J. Universal behavior in a generalized model of contagion. Phys Rev Lett (2004) 92(21):218701. doi:10.1103/physrevlett.92.218701
5. Peng H, Peng W, Zhao D, Wang W. Impact of the heterogeneity of adoption thresholds on behavior spreading in complex networks. Appl Mathematics Comput (2020) 386:125504. doi:10.1016/j.amc.2020.125504
6. Wang W, Tang M, Shu P, Wang Z. Dynamics of social contagions with heterogeneous adoption thresholds: Crossover phenomena in phase transition. New J Phys (2016) 18(1):013029. doi:10.1088/1367-2630/18/1/013029
7. Wang W, Tang M, Zhang H, Lai Y. Dynamics of social contagions with memory of nonredundant information. Phys Rev E (2015) 92(1):012820. doi:10.1103/physreve.92.012820
8. Han L, Lin Z, Tang M, Zhou J, Zou Y, Guan S. Impact of contact preference on social contagions on complex networks. Phys Rev E (2020) 101(4):042308. doi:10.1103/physreve.101.042308
9. Wang W, Shu P, Zhu YX, Tang M, Zhang YC. Dynamics of social contagions with limited contact capacity. Chaos (2015) 25(10):103102. doi:10.1063/1.4929761
10. Granovetter M. Threshold models of collective behavior. Am J Sociol (1978) 83(6):1420–43. doi:10.1086/226707
11. Yang Q, Zhu X, Tian Y, Wang G, Zhang Y, Chen L. The influence of heterogeneity of adoption thresholds on limited information spreading. Appl Mathematics Comput (2021) 411:126448. doi:10.1016/j.amc.2021.126448
12. Zhu X, Wang W, Cai S, Stanley H. Optimal imitation capacity and crossover phenomenon in the dynamics of social contagions. J Stat Mech (2018)(6) 063405. doi:10.1088/1742-5468/aac914
13. Fink C, Schmidt AC, Barash V, Kelly J, Cameron C, Macy M. Investigating the observability of complex contagion in empirical social networks. In: Tenth International AAAI Conference on Web and Social Media (2016).
14. Zhu X, Yang Q, Tian H, Ma J, Wang W. Contagion of information on two-layered weighted complex network. IEEE Access (2019) 7:155064–74. doi:10.1109/access.2019.2948941
15. Zhu X, Ma J, Su X, Tian H, Wang W, Cai S. Information spreading on weighted multiplex social network. Complexity (2019) 2019:5920187. doi:10.1155/2019/5920187
16. González M, HidalgoCésar A, Barabási A-L. Understanding individual human mobility patterns. Nature (2009) 453:238–782. doi:10.1038/nature07850
17. Perc M. Does strong heterogeneity promote cooperation by group interactions? New J Phys (2011) 13(12):123027. doi:10.1088/1367-2630/13/12/123027
18. Wang W, Tang M, Zhang HF, Gao H, Do Y, Liu ZH. Epidemic spreading on complex networks with general degree and weight distributions. Phys Rev E (2014) 90(4):042803. doi:10.1103/physreve.90.042803
19. Yuan X, Hu Y, Stanley H, Havlin S. Eradicating catastrophic collapse in interdependent networks via reinforced nodes. Proc Natl Acad Sci U S A (2017) 114(13):3311–5. doi:10.1073/pnas.1621369114
20. Catanzaro M, Boguná M, Pastor-Satorras R. Generation of uncorrelated random scale-free networks. Phys Rev E (2005) 71(2):027103. doi:10.1103/physreve.71.027103
21. Paul E, Rényi A. On the evolution of random graphs. Publ Math Inst Hung Acad Sci (1960) 5(1):17–60.
22. Shu P, Wei W, Ming T, Do Y. Numerical identification of epidemic thresholds for susceptible-infected-recovered model on finite-size networks. Chaos (2015) 25(6):063104. doi:10.1063/1.4922153
Keywords: complex network, behavioral propagation, active-passive psychology, phase transition, crossover phenomena
Citation: Ye J and Chen Y (2022) Social contagion influenced by active-passive psychology of college students. Front. Phys. 10:1019118. doi: 10.3389/fphy.2022.1019118
Received: 14 August 2022; Accepted: 29 August 2022;
Published: 04 October 2022.
Edited by:
Xuzhen Zhu, Beijing University of Posts and Telecommunications, ChinaReviewed by:
Yuexia Zhang, Beijing Information Science and Technology University, ChinaRun-Ran Liu, Hangzhou Normal University, China
Shimin Cai, University of Electronic Science and Technology of China, China
Copyright © 2022 Ye and Chen. This is an open-access article distributed under the terms of the Creative Commons Attribution License (CC BY). The use, distribution or reproduction in other forums is permitted, provided the original author(s) and the copyright owner(s) are credited and that the original publication in this journal is cited, in accordance with accepted academic practice. No use, distribution or reproduction is permitted which does not comply with these terms.
*Correspondence: Yi Chen, MDAxOTUwMzVAd3p1dC5lZHUuY24=