- 1Translational Biophotonics Laboratory, Institute of Bioengineering and Bioimaging, Agency for Science, Technology and Research (A*STAR), Singapore, Singapore
- 2Nanyang Technological University, Singapore, Singapore
The recently developed Diffuse Speckle Pulsatile Flowmetry (DSPF) technique offers high measurement rates of around 300 Hz for non-invasive blood flow measurement of blood flow in deep tissue (up to a depth of approximately 15 mm), showing promising potential for the monitoring of various pathologies associated with abnormal blood flow. The effects of various parameters associated with this technique such as speckle size and exposure time on the measured flow readings, however, have yet to be studied. In this work, we examine these relationships experimentally, observing that the number of pixels per speckle (a measure of speckle size) and exposure time have a strong inverse relationship and a positive relationship respectively with the measured DSPF readings in no-flow setups using both static single light scattering and multiple light scattering mediums. We also studied how the sensitivity of DSPF readings to flow is affected by these parameters, finding that the number of pixels per speckle and exposure time have an inverse relationship and strong positive linear relationship respectively with the gradient of the regression line between the actual and measured flow rates in a dynamic setup using a tissue-mimicking flow phantom. It is hoped that these results would enable researchers using the DSPF technique to select and utilize the most optimized settings for their specific use applications.
1 Introduction
Blood flow is a critical metric used for the monitoring of various normal and pathological conditions in the human body; abnormal blood flow patterns may indicate the incidence of myocardial ischemia [1], hemorrhagic and ischemic stroke [2, 3], and diabetic foot [4]. With growing interest towards the continuous monitoring of cardiovascular conditions in inpatient and remote care settings, new sensors utilising novel techniques to measure various cardiovascular parameters have been developed. These include optical fiber-based sensors such as those reported in [5, 6], which have the advantages of being unaffected by electromagnetic interference, being lightweight and chemically stable, among others [6, 7]. Recently, Diffuse Speckle Pulsatile Flowmetry (DSPF) has been developed as a non-invasive, portable, and cost-effective optical fiber-based technique for blood flow measurement in deep tissue (up to approximately 15 mm) [8–10]. DSPF shares similar principles with its predecessor, Diffuse Speckle Contrast Analysis (DSCA), which uses illumination and detection structure similar to Diffuse Correlation Spectroscopy (DCS) and data processing methods similar to Laser Speckle Contrast Imaging (LSCI) [11]. Unlike DSCA, however, DSPF uses a multimode (MM) detection fiber instead of a single mode (SM) detection fiber which enables increased signal throughput and confers it the advantage of being able to reach relatively high measurement rates of up to ∼300 Hz [8], in contrast to the slow blood flow measurement rates of approximately 5 Hz in fiber-based DSCA [8]. This allows it to capture the flow pulsations across the range of heart rates, as well as the intricate flow patterns which occur within each cardiac cycle. More details of DSPF and DSCA can be found in [8] and [12] respectively, and in Supplementary Table 1
Both these modalities are increasingly being employed in research studies for biomedical and clinical applications. Since it was developed in 2013, DSCA has been used in studies to assess tissue perfusion for the foot angiosome concept [13] and cerebral blood flow monitoring during middle cerebral artery occlusion (MCAO) in a rodent model [14]. In the former, it was able to measure the low frequency oscillations of blood flow in the foot, showing promising potential to support the diagnosis and monitoring of foot ulcers which is a complication arising from diabetes [13]. In the latter, it was able to detect the decrease in perfusion in neurological tissue as a result of MCAO, showing potential to observe cerebral hemodynamics associated with transient ischemic stroke [14]. DSPF, while only developed in 2020, has already been shown to be able to measure pulse wave velocity with a temporal resolution of 3 ms, paving the way for its use in the measurement of cardiovascular parameters such as heart rate and blood pressure [15]. Compared to other optical blood flow measurement modalities like LSCI [16], DCS [17] and Laser Doppler Flowmetry (LDF) [18], DSPF is advantageous in being able to measure perfusion in deep tissue with cost-effective instrumentation and simple data processing methods [8], and is thus likely to become more widely adopted in research labs and hospitals.
2 Theoretical background
In the fundamental mathematical model underlying both DSPF and DSCA that has been established and documented, several physical parameters including speckle size, magnification of the imaging system and exposure time have significant impacts on the output (flow index) [8, 12]. The performance of DSPF may be compromised if these parameters are not selected carefully. Several theoretical studies examining some of these parameters have been published based on the mathematical model of DSCA [19, 20], however since fiber-based DSCA systems use a SM fiber which only offers a single transmission mode for signal detection, the speckle size does not substantially affect the output reading. In contrast, because DSPF utilizes a MM detection fiber which transmits multiple light modes and allows a random speckle pattern to form at its tip [21], speckle size is a critical parameter that affects the output flow reading. To date, the effects of speckle size and exposure time on the output flow reading in DSPF systems have not been studied thoroughly through experimental validation, and as such we focus on these in this paper. We study the effects of speckle size (as measured by pixels per speckle) and Charged-Coupled Device (CCD) camera exposure time on the measured DSPF readings under static conditions, as well as the sensitivity of the readings to flow under dynamic conditions.
We envisioned that the results from these experimental characterizations would provide further insight into the theory of laser speckle analysis and facilitate appropriate parameter selection when the DSPF method is used in its various biomedical applications.
As some background, the DSPF technique measures the intensity contrast of a laser speckle pattern captured by a CCD camera through a MM detection fiber [8]. A speckle pattern is formed by the random interference of coherent light, such as that from a laser, when it is reflected off a surface [22]. When this pattern is imaged by a CCD’s pixel array, it is known as an image speckle [23] and its intensity contrast
where
To account for the altered light intensity distribution of the speckle pattern which results from the use of a MM fiber, DSPF measures the background intensity profile and uses it to correct the raw speckle patterns captured in each frame [8].
Like DSCA and LSCI, DSPF uses Eq. 2 to relate
Here,
In this equation
Specifically, Eq. 3 is used in combination with Eq. 2 to relate
Within this model, however, more still remains to be understood about the parameter
Using this relationship, they developed the first speckle model, shown in Eq. 5 [24, 27]:
where
where
While the importance of
This, however, required the use of a single-photon-counting avalanche photodiode (APD), which does not feature in DSPF or DSCA instrumentation, only in DCS instrumentation [22]. More recently, Parthasarathy et al. [27] developed a method to estimate
To determine
In addition, we seek to investigate the sensitivity of
3 Experiment on static phantom
To characterize the effect of the number of pixels per speckle on the measured flow reading (
where
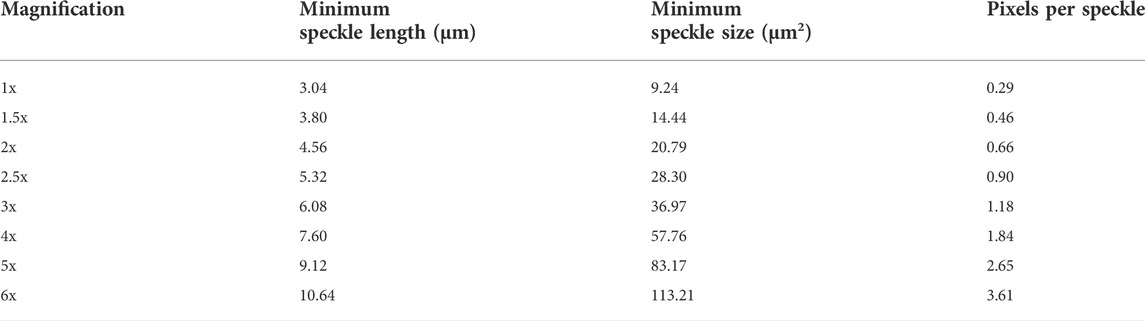
TABLE 1. Minimum speckle length, minimum speckle size and pixels per speckle for each magnification used.
A 200 µm core size MM fiber was used to transmit the laser light to illuminate the static medium. This core size was chosen over the smaller 62.5 µm and the larger 400 µm core sizes because it was deemed to be a size which balanced the tradeoffs between these other sizes - one that was not too small such that the number of observable speckles in the captured image speckle is severely limited and subsequent analysis would be impaired, yet one which was small enough such that it would not impose significant practical limitations on the flexibility of the fiber. Two different static mediums were used – a single-scattering, non-transparent solid iron medium and a multiple-scattering, silica-based tissue-mimicking phantom with
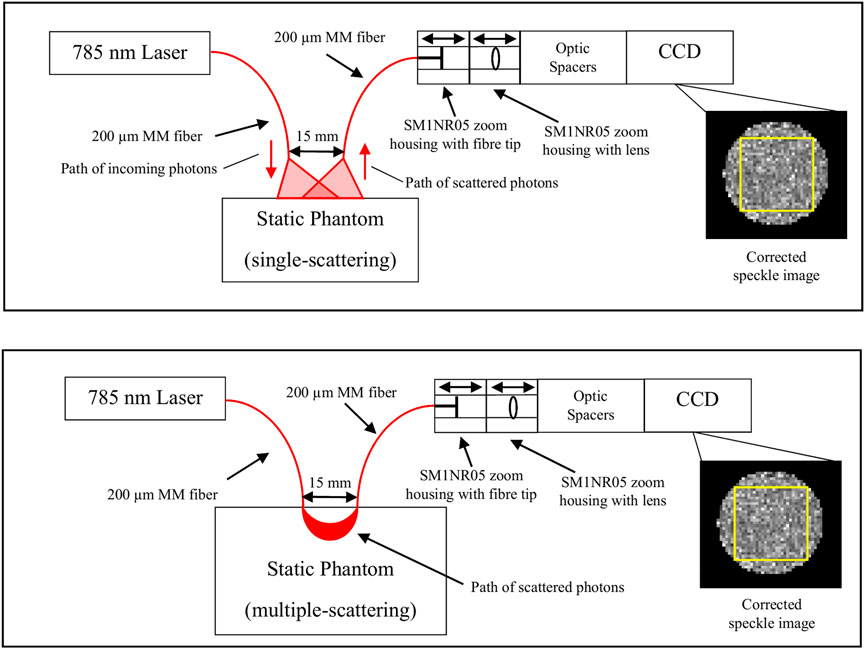
FIGURE 1. Schematic of the setup used in the static experiment. 785 nm laser light was delivered via a 200 μm MM fiber to illuminate a static phantom, and the backscattered light was transmitted via another 200 μm MM fiber placed 15 mm away to a CCD which captured the speckle image. SM1NR05 zoom housings containing the fiber tip and lens, along with optic spacers, were used to adjust the magnification of the system. The raw image captured by the CCD was then corrected against a normalized background pattern to obtain a corrected speckle image. The yellow box in the corrected speckle image shows the area in the speckle pattern used for the calculation of
A representative speckle image was obtained at each magnification, and these are shown in Figures 2A–H.
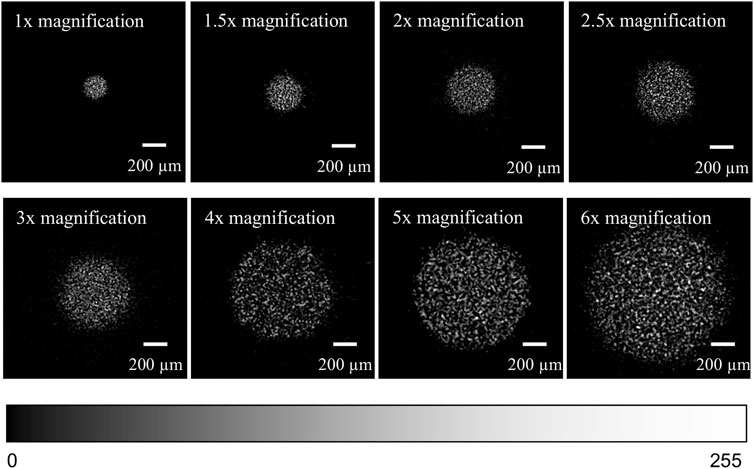
FIGURE 2. (A) (top row, leftmost) Corrected speckle image at 1× magnification. (B) (top row, 2nd from left) Corrected speckle image at 1.5× magnification. (C) (top row, 2nd from right) Corrected speckle image at 2× magnification. (D) (top row, rightmost) Corrected speckle image at 2.5× magnification. (E) (2nd row, leftmost) Corrected speckle image at 3× magnification. (F) (2nd row, 2nd from left) Corrected speckle image at 4× magnification. (G) (2nd row, 2nd from right) Corrected speckle image at 5× magnification. (H) (2nd row, rightmost) Corrected speckle image at 6× magnification. The brightness of each image has been adjusted to highlight the speckle pattern. The colour bar shows the range of pixel intensities from 0 to 255 (8-bit).
At each magnification and exposure time,
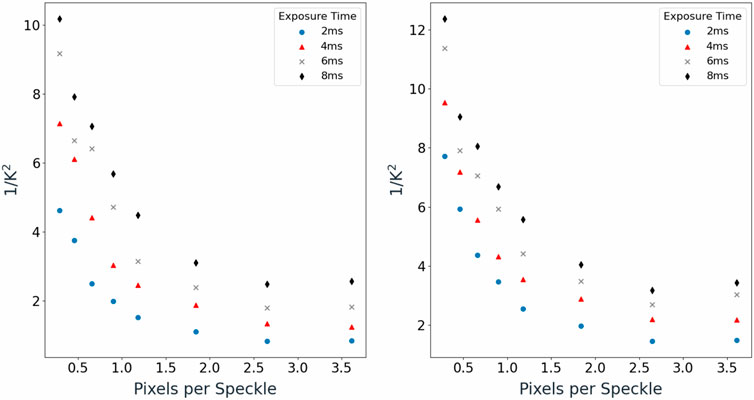
FIGURE 3. (A) (left) Mean
The general trend between
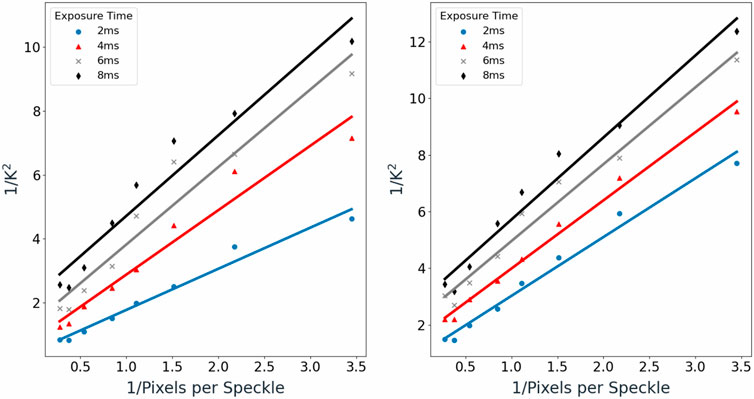
FIGURE 4. (A) (left) Mean
Good linear fitting was observed, with coefficient of determination (R2) values ranging from 0.92 to 0.97 for the single-scattering medium and 0.97 to 0.99 for the multiple-scattering medium.
Knowing that
Based on Figures 3A,B, exposure time was also observed to have a positive relationship with
4 Experiment on dynamic phantom
A separate experiment was performed to determine the effect of the number of pixels per speckle and CCD camera exposure time on the sensitivity of the
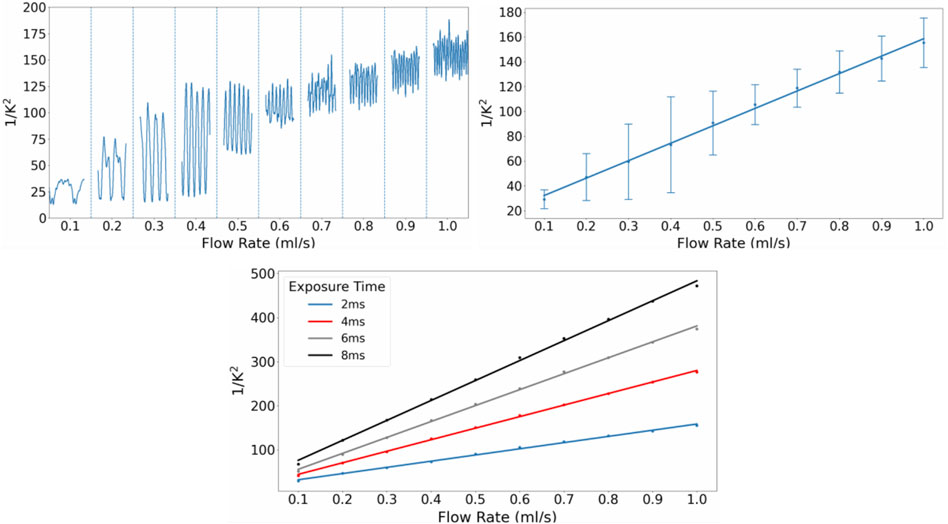
FIGURE 5. (A) (top left) Smoothened
As seen in Figure 5A, the periodic changes in flow rate produced by the peristaltic pump were detected by the CCD camera and reflected in the fluctuating
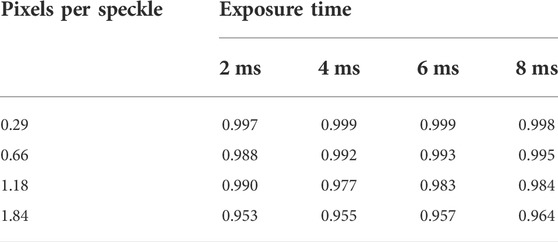
TABLE 2. R2 values for the plots of the obtained mean
Increasing the exposure time of the CCD camera resulted in an increase in the sensitivity of the system, as seen in the increasing gradients of the regression lines of the mean
The magnitudes of the gradients were also calculated for the other configurations of pixels per speckle and exposure time. These are plotted in Figure 6.
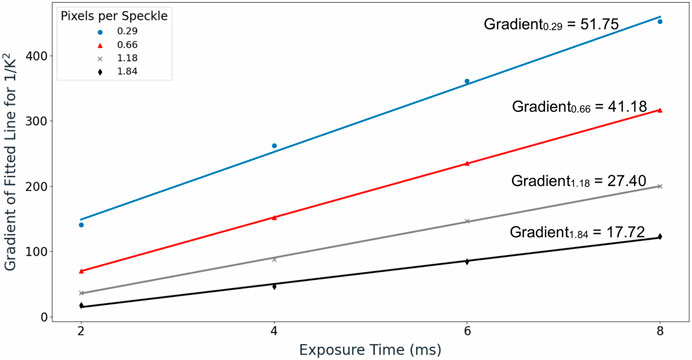
FIGURE 6. Gradient values of the regression lines fitted between
A linear relationship between the magnitude of the gradient and exposure time was observed across all pixels per speckle values, with coefficient of determination (R2) values exceeding 0.99 for all cases. The gradient for
5 Discussion
We believe the results presented herein may offer a better theoretical understanding of diffuse laser speckle analysis. The proposed linear relationship between pixels per speckle and
The parameters used in our study were tailored towards the use of DSPF systems in biomedical applications. The range of exposure times studied was within the normal range used in biomedical applications [16], and the pixels per speckle values used were chosen based on practically feasible magnifications of the imaging system. We deemed magnifications of the imaging system from 1x to 4x for flow setups to be practically feasible as they did not require excessively thick lens or large image distances. Imaging magnifications lower than 1x were not experimented with, although these might be beneficial because they cause the energy from the backscattered photons to be concentrated onto a smaller area on the CCD array, improving the SNR. For the purposes of this study, however, we believed that an imaging magnification of 1x was sufficiently small. The 1x magnification is also arguably the most convenient magnification that can be used, given that it can involve just placing the fiber tip at the CCD array, removing the need for lenses and space to accommodate larger image distances. This may be especially useful for sensors for which compactness is a key requirement.
6 Conclusion
In this study, we present results of experimental characterization tests on the recently developed DSPF technique. Because DSPF uses a MM detection fiber which captures multiple speckles in a single image frame and renders speckle size a parameter which affects the output flow readings, we designed and implemented a lens module that could allow the magnification of the imaging system to be changed easily to study the effect of speckle size. We have been able to determine the relationship between
Data availability statement
The original contributions presented in the study are included in the article/Supplementary Material, further inquiries can be directed to the corresponding authors.
Author contributions
Conceptualization, RB; methodology, RB; software, RB; validation, TC; formal analysis, TC; investigation, TC; resources, MO; data curation, TC and RB; writing—original draft preparation, TC; writing—review and editing, RZ and RB; visualization, TC; supervision, RB; project administration, RB; funding acquisition, RB. All authors have read and agreed to the published version of the manuscript.
Funding
This research was funded by Agency of Science, Technology and Research (A*STAR) under its Industry Alignment Fund prepositioning program (IAF-PP), Award H19/01/a0/0EE9 and BMRC Central Research Fund (UIBR) 2021.
Conflict of interest
The authors declare that the research was conducted in the absence of any commercial or financial relationships that could be construed as a potential conflict of interest.
Publisher’s note
All claims expressed in this article are solely those of the authors and do not necessarily represent those of their affiliated organizations, or those of the publisher, the editors and the reviewers. Any product that may be evaluated in this article, or claim that may be made by its manufacturer, is not guaranteed or endorsed by the publisher.
Supplementary material
The Supplementary Material for this article can be found online at: https://www.frontiersin.org/articles/10.3389/fphy.2022.1006484/full#supplementary-material
References
1. Nakano H, Okazaki K, Ajiro Y, Suzuki T, Oba K. Clinical usefulness of the common carotid artery blood flow velocity ratio as measured by an ultrasonic quantitative flow measurement system: Evaluation with respect to prevalence of ischemic heart disease. J Nippon Med Sch (2001) 68(6):482–9. doi:10.1272/jnms.68.482
2. Xiao M, Li Q, Feng H, Zhang L, Chen Y. Neural vascular mechanism for the cerebral blood flow autoregulation after hemorrhagic stroke. Neural plasticity (2017) 2017:1–12. doi:10.1155/2017/5819514
4. Pendsey SP. Understanding diabetic foot. Int J Diabetes Dev Ctries (2010) 30(2):75–9. doi:10.4103/0973-3930.62596
5. Han P, Li L, Zhang H, Guan L, Marques C, Savović S, et al. Low-cost plastic optical fiber sensor embedded in mattress for sleep performance monitoring. Opt Fiber Tech (2021) 64:102541. doi:10.1016/j.yofte.2021.102541
6. Leal-Junior AG, Díaz CR, Leitão C, Pontes MJ, Marques C, Frizera A. Polymer optical fiber-based sensor for simultaneous measurement of breath and heart rate under dynamic movements. Opt Laser Tech (2019) 109:429–36. doi:10.1016/j.optlastec.2018.08.036
7. Peters K. Polymer optical fiber sensors—a review. Smart materials and structures. 2010;20(1):013002.
8. Bi R, Du Y, Singh G, Ho J-H, Zhang S, Attia ABE, et al. Fast pulsatile blood flow measurement in deep tissue through a multimode detection fiber. J Biomed Opt (2020) 25(5):1–10.
9. Durduran T, Choe R, Baker WB, Yodh AG. Diffuse optics for tissue monitoring and tomography. Rep Prog Phys (2010) 73(7):076701. doi:10.1088/0034-4885/73/7/076701
10. Buckley EM, Parthasarathy AB, Grant PE, Yodh AG, Franceschini MA. Diffuse correlation spectroscopy for measurement of cerebral blood flow: Future prospects. Neurophotonics (2014) 1(1):011009. doi:10.1117/1.nph.1.1.011009
11. Bi R, Dong J, Lee K. Deep tissue flowmetry based on diffuse speckle contrast analysis. Opt Lett (2013) 38(9):1401–3. doi:10.1364/ol.38.001401
12. Bi R, Dong J, Lee K. Multi-channel deep tissue flowmetry based on temporal diffuse speckle contrast analysis. Opt Express (2013) 21(19):22854–61. doi:10.1364/oe.21.022854
13. Yeo C, Jung H, Lee K, Song C. Low frequency oscillations assessed by diffuse speckle contrast analysis for foot angiosome concept. Sci Rep (2020) 10(1):17153–14. doi:10.1038/s41598-020-73604-0
14. Yeo C, Kim H, Song C. Cerebral blood flow monitoring by diffuse speckle contrast analysis during MCAO surgery in the rat. Curr Opt Photon (2017) 1(5):433–9.
15. Teng Z, Gao F, Xia H, Chen W, Li C. In vivo pulse wave measurement through a multimode fiber diffuse speckle analysis system. Front Phys (2020) 8(613342). doi:10.3389/fphy.2020.613342
16. Boas DA, Dunn AK. Laser speckle contrast imaging in biomedical optics. J Biomed Opt (2010) 15(1):011109. doi:10.1117/1.3285504
17. Yu G. Diffuse correlation spectroscopy (DCS): A diagnostic tool for assessing tissue blood flow in vascular-related diseases and therapies. Curr Med Imaging Rev (2012) 8(3):194–210. doi:10.2174/157340512803759875
18. Obeid A, Barnett N, Dougherty G, Ward G. A critical review of laser Doppler flowmetry. J Med Eng Technol (1990) 14(5):178–81. doi:10.3109/03091909009009955
19. Liu J, Zhang H, Lu J, Ni X, Shen Z. Quantitative model of diffuse speckle contrast analysis for flow measurement. J Biomed Opt (2017) 22(7):076016. doi:10.1117/1.jbo.22.7.076016
20. Liu J, Zhang H, Lu J, Ni X, Shen Z. Simultaneously extracting multiple parameters via multi-distance and multi-exposure diffuse speckle contrast analysis. Biomed Opt Express (2017) 8(10):4537–50. doi:10.1364/boe.8.004537
21. Leal-Junior AG, Frizera A, Marques C, Pontes MJ. Optical fiber specklegram sensors for mechanical measurements: A review. IEEE Sens J (2019) 20(2):569–76. doi:10.1109/jsen.2019.2944906
22. Sironi L, D'Alfonso L, Bouzin M, Ceffa NG, Collini M, Chirico G, et al. Optical blood flow measurement in microcirculatory systems (2016).
23. Briers JD. Laser Doppler, speckle and related techniques for blood perfusion mapping and imaging. Physiol Meas (2001) 22(4):R35–66. doi:10.1088/0967-3334/22/4/201
24. Fercher A, Briers JD. Flow visualization by means of single-exposure speckle photography. Opt Commun (1981) 37(5):326–30. doi:10.1016/0030-4018(81)90428-4
25. Briers JD, Webster S. Laser speckle contrast analysis (LASCA): A nonscanning, full-field technique for monitoring capillary blood flow. J Biomed Opt (1996) 1(2):174–9. doi:10.1117/12.231359
26. Bi R, Dong J, Poh CL, Lee K. Optical methods for blood perfusion measurement—Theoretical comparison among four different modalities. J Opt Soc Am A (2015) 32(5):860–6. doi:10.1364/josaa.32.000860
27. Parthasarathy AB, Tom WJ, Gopal A, Zhang X, Dunn AK. Robust flow measurement with multi-exposure speckle imaging. Opt Express (2008) 16(3):1975–89. doi:10.1364/oe.16.001975
28. Lemieux P-A, Durian D. Investigating non-Gaussian scattering processes by using nth-order intensity correlation functions. J Opt Soc Am A (1999) 16(7):1651–64. doi:10.1364/josaa.16.001651
Keywords: laser speckle, speckle size, diffuse speckle contrast analysis, diffuse speckle pulsatile flowmetry, exposure time
Citation: Choo TWJ, Zhang R, Bi R and Olivo M (2022) Experimental characterization of diffuse speckle pulsatile flowmetry system. Front. Phys. 10:1006484. doi: 10.3389/fphy.2022.1006484
Received: 29 July 2022; Accepted: 22 August 2022;
Published: 26 September 2022.
Edited by:
Rahul Kumar Gangwar, University of Delhi, IndiaReviewed by:
Carlos Marques, University of Aveiro, PortugalSantosh Kumar, Liaocheng University, China
Akhilesh Kumar Pathak, Chulalongkorn University, Thailand
Copyright © 2022 Choo, Zhang, Bi and Olivo. This is an open-access article distributed under the terms of the Creative Commons Attribution License (CC BY). The use, distribution or reproduction in other forums is permitted, provided the original author(s) and the copyright owner(s) are credited and that the original publication in this journal is cited, in accordance with accepted academic practice. No use, distribution or reproduction is permitted which does not comply with these terms.
*Correspondence: Renzhe Bi, bi_renzhe@ibb.a-star.edu.sg; Malini Olivo, malini_olivo@ibb.a-star.edu.sg