- 1Research Institute for Smart Cities, School of Architecture and Urban Planning, Shenzhen University, Shenzhen, China
- 2School of Resource and Environmental Sciences, Wuhan University, Wuhan, China
Human daily mobility plays an important role in urban research. Commuting of urban residents is an important part of urban daily mobility, especially in working days. However, the characteristic of the mobility network formed by the commuting of urban residents and its impact on the internal structure of the city are still an important work that needs to be explored further. Aiming to study the living–working interaction pattern of meta-populations over urban divisions within cities, a fine-grained dataset of living–working tracking of Shenzhen is curated and used to construct an urban living–working mobility network, and the living–working interaction pattern is analyzed through the community structures of the network. The results show that human daily mobility plays an important role in understanding the formation of urban structure, the administrative divisions of the city affect human daily mobility, and human daily mobility reacts on the formation of urban structure.
Introduction
Large-scale demographic census enables measurements of human living–working traces, which have become popular and served as essential reasons of motivation for human mobility [1]. The living–working interactions of meta-populations over urban divisions within cities have been extensively studied in a recent work (e.g., urban activities [1–4] and urban balance [5–8]). To study human living–working movements, especially within cities, living–working networks provide a useful way to characterize living–working styles among people in different sites. Although urban transportation patterns between locations change over time, many studies of human mobility assume they are representative [9], neglecting the limitation of transportation. For example, in Shenzhen, on average, people travel by subway for distance longer than 27 km while by bus for 9 km [10]. This is, arguably, due to the lack of fine-grained public datasets that could describe the living–working dynamics within cities. There are some open-access datasets covering small geographical locations considering the time ordering of location tracking, such as networks of mobile-phone users within a city [11] and between cities [12], which can infer people’s living and working locations potentially. However, fine-grained living–working datasets covering large geographical regions within a city with large populations are still missing from the open-access datasets.
In this paper, we curate and amass a fine-grained dataset of living–working mobility to study urban interactions. We capture living and working position tracking of millions of workers from an open-data program in Shenzhen. Each location in our dataset represents a group of workers in an official administrative division. Directed movement of each individual from a source living location to a destination working location denotes a change of location for the corresponding individual. The overall directed mobility network of locations is finally compiled by sequentially processing the directed movements for all individuals. In the network, a node represents a location. A weighted edge represents the total number of workers’ movements from living to working locations.
The dataset contains movements of nearly 6 million anonymized cellular phone users among 71 subdivisions (henceforth locations), covering 10 geographically adjacent districts, investigated during the year 2015. This total geographic area, located in Shenzhen as a major city in the Guangdong Province of China, covers more than 20 square kilometers and, in 2017, had a population of nearly 12.9 million. This city has become one of the four largest and wealthiest cities in China [13]. Thus, it is a fine-grained living–working dataset covering large geographical regions within a city with large populations. However, the characteristic of the mobility network formed by the commuting of urban residents and its impact on the internal structure of the city are still an important work that needs to be explored further. To study the living–working interaction pattern of meta-populations over urban divisions within cities, we process the above raw dataset to extract a dynamic and directed mobility network of the city. Then, based on the constructed urban living–working mobility network, the human living–working mobility pattern and the community structure of the city are analyzed. We found that there is an interaction between the human daily mobility and the formation of urban structure, the administrative divisions of the city affect human daily mobility, and human daily mobility reacts on the urban structure.
Materials and Methods
Data Extraction and Processing
A largest urban living–working tracking dataset using demographic census logs in the year 2015 in Shenzhen is used in this study. The dataset consists of living–working location records of 5.6 million anonymized workers, and these locations include 71 geographically neighboring subdistricts over 10 districts. An individual worker is investigated by the Shenzhen Municipal Human Resources and Social Security Bureau (http://hrss.sz.gov.cn/) from the social security systems in Shenzhen used by workers to buy the social security and health insurance for every working individual.
The reliability of location and time information of workers’ living and working locations in the network data largely depends on the reliability of the underlying source data. We verify the consistency via the geographically explicit distribution of locations. Although this dataset is fine-grained, it has several limitations. First, even though the dataset covers a cohort of millions of workers, it is only for 2015. Second, the individual’s working position is the last known recorded location of this individual in the year 2015. This recording might cause bias. The individual might leave Shenzhen after 2015.
Construction of Living–Working Network
To simulate human mobility within Shenzhen, we construct the living–working network (a directed mobility network) based on the dataset by taking each place (a city or a country) as multiple meta-populations in different locations. Each location is represented as a node in the network. Edges are directed, connecting nodes where users move from origin (living locations) to the destination (working locations) and weighted by the total number of workers in this scenario. An individual worker–directed movement from the living location i to the working location j denotes that in a user’s living–working record. We reinterpret the flow matrices F as adjacency matrices that describe the daily living–working mobility network, the vertices are the 71 locations, and the edge between a given vertex pair, i and j, is weighted by the flow Nij (the number of workers from location i to location j). As shown in Table 1, the dataset is processed into two data tables: Network table and Location table. In the Network table, each row represents the total number of daily movements by workers from locations i to j, and there are three columns ordered by the living location, the working location, and the corresponding directional weight. In the Location table, there are two columns ordered by the location identifier and the corresponding charactered name.
Mobility Analysis of Living–Working Network
In the urban living–working mobility network, nodes are defined as locations, and edges are weighted by the population flows from living locations to working locations. To analyze the intensity of human mobility, the network centrality of individual locations is calculated, and the consistency of the network with the degree distribution is verified. We consider the network defined by the daily living–working mobility matrix F, and the in-degree and out-degree of the location i are then given by
Structure Analysis of Living–Working Network
Additionally, to explore the characteristic of the mobility network formed by the commuting of urban residents and what impact does it have on the internal structure of the city, we analyze the community structure of the urban living–working mobility network using the Louvain community detection algorithm [14–17]. It identifies disjoint subsets of locations such that their intra-connectivity far exceeds their inter-connectivity. All the locations in the network are divided into seven community-groups, in which the meta-populations in locations are highly intra-connected within the group but only loosely inter-connected across the group [18–20]. Then, the detected community structure is used to map the Shenzhen administrative divisions in 2017 and 2005 to analyze whether human daily mobility is infected by the administrative division, and whether there is interaction between the human daily mobility and the formation of urban structure.
Results
Mobility Pattern of Living–Working Network
In the analysis of the intensity and range of human mobility, Figure 1A shows the distribution of the directed network’s degrees and the number of resident workers in living and working locations. The x-axis denotes the network degree of locations, and the y-axis denotes the number of resident workers in those locations. The degree of a node denotes the total number of living–working movements passing through the corresponding node. We can see that, with the increase of network degree, the number of resident workers decreases rapidly, that is to say, locations with more active external relations have fewer resident populations. Figure 1B shows the distance distribution as compared to that of a mobility network by taxi trips in Shenzhen [18]. We can observe that living–working pairs with less than 80 km account for over 99.96% of the total, and the median living–working distance is 16 km, in contrast with a shorter median traveling distance of 3 km by taxi trips.
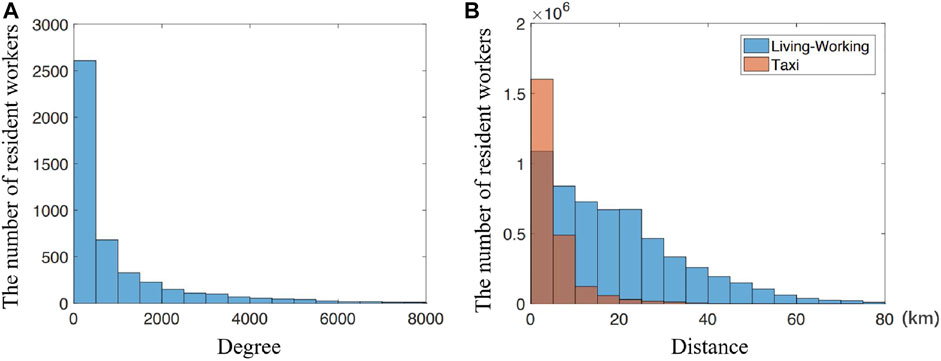
FIGURE 1. Distribution plots. (A) Empirical degree distribution. The x-axis denotes the network degree of locations. The y-axis denotes the number of workers from the living to the working location. (B) Empirical distance distribution with this living–working network. The x-axis denotes the commuting distance of workers, and the unit is kilometer. The y-axis denotes the number of associated individuals. In contrast, we show the distance distribution of a static mobility network with zones as nodes and passenger flows as edges, aggregating 2 million taxi trips in Shenzhen from April 18, 2011, to April 26, 2011, over 1,634 zones [18]. The median distance is 16 km for our published dataset, in contrast with 3 km for those taxi trips.
Community Structure of Living–Working Network
In the analysis of the relationship between the human daily mobility and the formation of urban structure, Figure 2 shows the community structures for each day with colors denoting different detected communities. After mapping communities to the administrative divisions, we can observe locations within a division tend to be in the same community. Interestingly, the administrative divisions in 2005 are larger and can group locations within the same community better, especially in the Bao’an division.
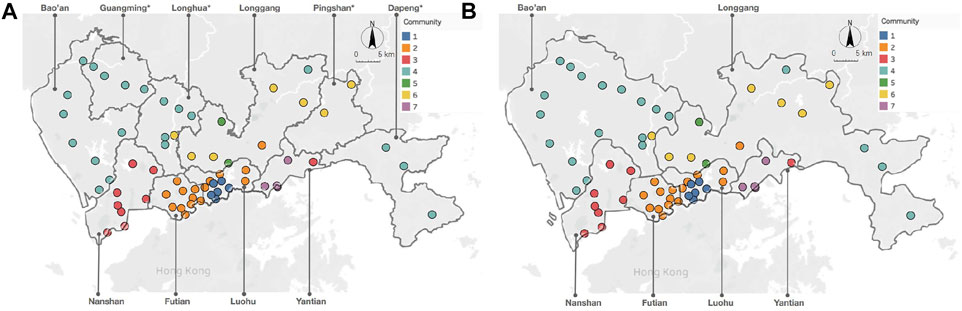
FIGURE 2. Community structures of the Shenzhen living–working network. We construct the directed network via aggregating all workers’ living and working records. The Louvain community detection algorithm serves to probe community structures based on this network. We map this community structure with colors denoting different communities to Shenzhen administrative divisions in 2017 (A) and 2005 (B). The newly established divisions are marked by asterisks in (A), but not in (B). The spatial map was created using the OpenStreetMap online platform (http://www.openstreetmap.org/) under the license of CC BY-SA (http://www.openstreetmap.org/copyright). More details of the license can be found at http://creativecommons.org/licenses/by-sa/2.0/. Line graphs were drawn using Tableau Software for Desktop version 9.2.15 (https://www.tableau.com/zh-cn/support/releases/9.2.15). The layouts were modified with Keynote version 6.6.2 (http://www.apple.com/keynote/).
Discussion and Conclusion
Urban living and working tracking can provide fine-grained traveling data within cities on daily scales, giving us a feasible way to explore human daily mobility, especially in working days. Although there are different choices of transportation patterns between locations within the city, neglecting the limitation of transportation, origin–destination is representative in some study of human mobility. Many urban problems are related to the commuting of urban residents, such as traffic congestion in the morning and evening rush hours, jobs-housing balance in the urban structure, and the fairness of urban facilities. Moreover, human daily mobility plays an important role in understanding the formation of urban structure. On the one hand, there is an interaction between the human daily mobility and the formation of urban structure, and the daily life of urban residents is usually within a certain range, within which there are more internal connections and less connections with other external areas. In other words, human daily mobility shapes the urban structure, and in each structure, intra-connectivity far exceeds inter-connectivity. On the other hand, the administrative divisions of the city affect human daily mobility, and human daily mobility reacts on the urban structure. The administrative division will affect the scope of daily activities of urban residents because some things will be more convenient in the same administrative division. To some extent, most of residents’ social relationships are within the region. This study reinforces the importance of the living–working interaction pattern of meta-populations over urban divisions within cities in urban management. Human mobility is an important research direction in urban research, and human living–working traces served as essential reasons of motivation for human daily mobility.
Data Availability Statement
The original contributions presented in the study are included in the article/Supplementary Material, and further inquiries can be directed to the corresponding author.
Author Contributions
ZY and HL conceived the study, designed statistical and modeling methods, conducted analyses, and wrote the article. ZY, HL, ST, and RG interpreted the results and revised the article.
Funding
We acknowledge the financial support by the National Natural Science Foundation of China (Project Nos. 71901147, 41801392, 41901329, 41971354, and 41971341), the Research Program of Shenzhen S&T Innovation Committee (Project Nos. JCYJ20210324093600002 and JCYJ20210324093012033), the Science Foundation of Guangdong Province (Project Nos. 2121A1515012574 and 2019A1515010748), the Open Fund of Key Laboratory of Urban Land Resources Monitoring and Simulation, MNR, China (No. KF-2019-04-014), Natural Science Foundation of Jilin Provincial Science and Technology Department (No. 20180101332JC), the Science and Technology Project of the Jilin Provincial Education Department (No. JJKH20210135KJ), and Jilin Science and technology development plan project (No.20190303124SF).
Conflict of Interest
The authors declare that the research was conducted in the absence of any commercial or financial relationships that could be construed as a potential conflict of interest.
Publisher’s Note
All claims expressed in this article are solely those of the authors and do not necessarily represent those of their affiliated organizations, or those of the publisher, the editors, and the reviewers. Any product that may be evaluated in this article, or claim that may be made by its manufacturer, is not guaranteed or endorsed by the publisher.
References
1. Du Z, Yang B, Liu J. Understanding the Spatial and Temporal Activity Patterns of Subway Mobility Flows (2017).
2. Gao C, Fan Y, Jiang S, Deng Y, Liu J, Li XJITOITS. Dynamic Robustness Analysis of a Two-Layer Rail Transit Network Model (2021).
3. Long Y, Han H, Tu Y, Shu X. Evaluating the Effectiveness of Urban Growth Boundaries Using Human Mobility and Activity Records. Cities (2015) 46:76–84. doi:10.1016/j.cities.2015.05.001
4. Zhang D, He T, Zhang F, Xu C. Urban-Scale Human Mobility Modeling with Multi-Source Urban Network Data. Ieee/acm Trans Networking (2018) 26:671–84. doi:10.1109/tnet.2018.2801598
5. Bagrow JP, Lin Y-R. Mesoscopic Structure and Social Aspects of Human Mobility. PLoS ONE (2012) 7:e37676. doi:10.1371/journal.pone.0037676
6. Barbosa H, Barthelemy M, Ghoshal G, James CR, Lenormand M, Louail T, et al. Human Mobility: Models and Applications. Phys Rep (2018) 734:1–74. doi:10.1016/j.physrep.2018.01.001
7. Deng Y, Wang J, Gao C, Li X, Wang Z, Li X, Applications Assessing Temporal-Spatial Characteristics of Urban Travel Behaviors from Multiday Smart-Card Data. Physica A: Stat Mech its Appl (2021) 576:126058. doi:10.1016/j.physa.2021.126058
8. Leana CR, Meuris J. Living to Work and Working to Live: Income as a Driver of Organizational Behavior. Annals (2015) 9:55–95. doi:10.5465/19416520.2015.1007654
9. Yan XY, Wang WX, Gao ZY, Lai YC. Universal Model of Individual and Population Mobility on Diverse Spatial Scales. Nat Commun (2017) 8:1639–9. doi:10.1038/s41467-017-01892-8
10. Tan J, Huang Y, Li Z, Wang L, Guo W, Li H. The Characteristics on Commuting Travel Mode Split and Origin Destination (OD) Distribution in Shenzhen, China, 2017 6th Data Driven Control and Learning Systems (DDCLS). IEEE (2017). p. 716–20.
11. Du Z, Yang Y, Gao C, Huang L, Huang Q, Bai Y. The Temporal Network of mobile Phone Users in Changchun Municipality, Northeast China. Sci Data (2018) 5:180228–7. doi:10.1038/sdata.2018.228
12. Du Z, Yang Y, Ertem Z, Gao C, Bai YJED. Inter-urban Mobility via Cellular Position Tracking in the Southeast Songliao Basin, Northeast China (2019). p. 6.
14. Blondel VD, Guillaume J-L, Lambiotte R, Lefebvre E, experiment. Fast Unfolding of Communities in Large Networks. J Stat Mech (2008) 2008:P10008. doi:10.1088/1742-5468/2008/10/p10008
15. De Meo P, Ferrara E, Fiumara G, Provetti A. Generalized Louvain Method for Community Detection in Large Networks, 2011 11th International Conference on Intelligent Systems Design and Applications. IEEE (2011). p. 88–93.
16. Que X, Checconi F, Petrini F, Gunnels JA. Scalable Community Detection with the Louvain Algorithm. In: 2015 IEEE International Parallel and Distributed Processing Symposium. IEEE (2015). p. 28–37.
17. Fazlali M, Moradi E, Tabatabaee Malazi H. Adaptive Parallel Louvain Community Detection on a Multicore Platform. Microprocessors and Microsystems (2017) 54:26–34. doi:10.1016/j.micpro.2017.08.002
18. Yan X-Y, Zhao C, Fan Y, Di Z, Wang W-X. Universal Predictability of Mobility Patterns in Cities. J R Soc Interf (2014) 11:20140834. doi:10.1098/rsif.2014.0834
19. Zhang Z, Pu P, Han D, Tang M, Applications Self-Adaptive Louvain Algorithm: Fast and Stable Community Detection Algorithm Based on the Principle of Small Probability Event. Physica A: Stat Mech its Appl (2018) 506:975–86. doi:10.1016/j.physa.2018.04.036
Keywords: human mobility, community structures, network analysis, Shenzhen, commuting pattern
Citation: Yuan Z, Lin H, Tang S and Guo R (2021) Geographically Explicit Network Analysis of Urban Living and Working Interaction Pattern in Shenzhen City, South China. Front. Phys. 9:795641. doi: 10.3389/fphy.2021.795641
Received: 15 October 2021; Accepted: 17 November 2021;
Published: 17 December 2021.
Edited by:
Chao Gao, Southwest University, ChinaReviewed by:
Hongzhi Miao, Dalian Maritime University, ChinaHongfei Jia, Jilin University, China
Yongxing Li, Beijing University of Technology, China
Copyright © 2021 Yuan, Lin, Tang and Guo. This is an open-access article distributed under the terms of the Creative Commons Attribution License (CC BY). The use, distribution or reproduction in other forums is permitted, provided the original author(s) and the copyright owner(s) are credited and that the original publication in this journal is cited, in accordance with accepted academic practice. No use, distribution or reproduction is permitted which does not comply with these terms.
*Correspondence: Haojia Lin, linhaojia@whu.edu.com