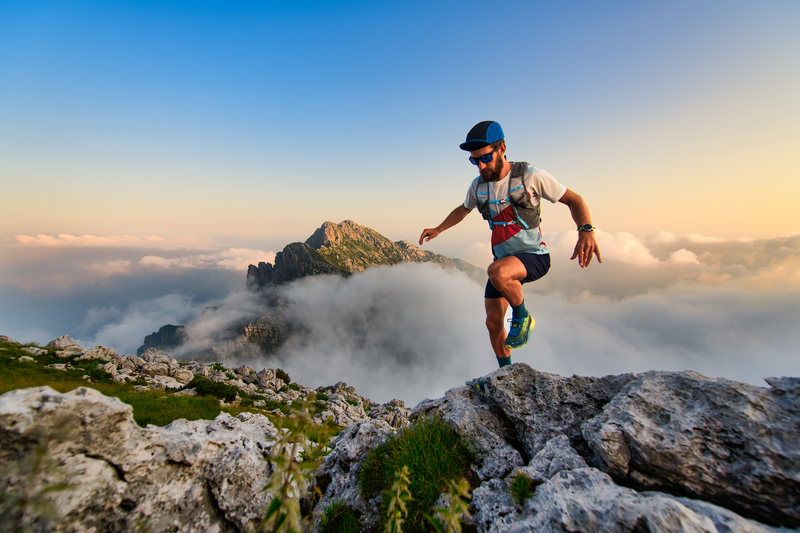
94% of researchers rate our articles as excellent or good
Learn more about the work of our research integrity team to safeguard the quality of each article we publish.
Find out more
ORIGINAL RESEARCH article
Front. Phys. , 15 September 2021
Sec. Social Physics
Volume 9 - 2021 | https://doi.org/10.3389/fphy.2021.715996
This article is part of the Research Topic Physics of Social Interactions View all 12 articles
The collective dynamics and structure of animal groups has attracted the attention of scientists across a broad range of fields. A variety of agent-based models have been developed to help understand the emergence of coordinated collective behavior from simple interaction rules. A common, simplifying assumption of such collective movement models, is that individual agents move with a constant speed. In this work we critically re-asses this assumption. First, we discuss experimental data showcasing the omnipresent speed variability observed in different species of live fish and artificial agents (RoboFish). Based on theoretical considerations accounting for inertia and rotational friction, we derive a functional dependence of the turning response of individuals on their instantaneous speed, which is confirmed by experimental data. We then investigate the interplay of variable speed and speed-dependent turning on self-organized collective behavior by implementing an agent-based model which accounts for both these effects. We show that, besides the average speed of individuals, the variability in individual speed can have a dramatic impact on the emergent collective dynamics: a group which differs to another only in a lower speed variability of its individuals (groups being identical in all other behavioral parameters), can be in the polarized state while the other group is disordered. We find that the local coupling between group polarization and individual speed is strongest at the order-disorder transition, and that, in contrast to fixed speed models, the group’s spatial extent does not have a maximum at the transition. Furthermore, we demonstrate a decrease in polarization with group size for groups of individuals with variable speed, and a sudden decrease in mean individual speed at a critical group size (N = 4 for Voronoi interactions) linked to a topological transition from an all-to-all to a distributed spatial interaction network. Overall, our work highlights the importance to account for fundamental kinematic constraints in general, and variable speed in particular, when modeling self-organized collective dynamics.
The emergent, highly coordinated, collective movements of schools of fish, flocks of birds and insect swarms, are fascinating examples of biological self-organization. Our understanding of these collective systems has been significantly advanced over the past years through diverse research efforts in biology [1–5], mathematics [6–8], computer science [9, 10], engineering [11, 12], and statistical physics [13–17].
In addition to empirical observations, mathematical models are an important tool for studying self-organization and collective behavior, and have been instrumental in uncovering general principles of how robust, large-scale coordination can emerge from simple, local interactions between self-propelled agents [10, 18, 19].
When formulating models, in general and for animal collectives, one has to balance simplicity/generality and detailed resemblance to experimental systems. From a statistical physics point of view, it is viable to assume some sort of universality of the collective dynamics even in far-from-equilibrium situations. Thus, as long as the model accounts for crucial aspects of the microscopic dynamics, other microscopic details become irrelevant for the macroscopic behavior for sufficiently large systems over a long temporal scale. However, 1) there is no general way to tell when the system is sufficiently large, and 2) animal groups consist of tens to hundreds, rarely thousands or more, individuals. Therefore, animal collectives should be rather viewed as mesoscopic systems, where the actual details of individual movement behavior may play an important role [20], and caution is advised when simplifying modeling assumptions.
A particularly prominent simplification often encountered in models of collective behavior is the assumption of constant speed of individual agents [13, 21–23]; for exceptions see [24–28]. However, although animals may generally tend to move at a certain, often preferred, speed, they are also able to flexibly modify their speed, ranging from non-moving to the maximum of their movement capacity. Speed adaptation due to environmental factors or social interactions [2]—ignored in constant speed models—as well as heterogeneity thereof, may play a decisive role in the ability of groups to coordinate their movement and thereby the structure of animal groups [29]. Indeed, experiments demonstrated that speed influences the collective behavior strongly, via a coupling to polarization/alignment [3, 22, 25, 29–31] which could also be shown at the local scale [25], i.e. regions in the shoal with faster fish are more polarized. In most former simulation studies, agents’ speed was modelled to modify the turning rate or the assumed social forces, but was generally set to be fixed rendering speed to a mere parameter [3, 21, 22, 32]. However, a couple of studies have shown that variable speed has repercussions on group-level patterns and can lead to qualitatively new, emergent phenomena on the group level as for example bi-stable behavior with respect to polarization [24, 25]. In this bistable region, the group remains in the initiated collective state (ordered vs. disordered) because 1) the strong alignment force maintains the order (stable order) or 2) the velocity alignment reduces the speed, because the magnitude of the mean neighbor velocity is low, which allows a faster turning initiated by noise (stable disorder). These findings demonstrate the important role of feedbacks between speed, turning, and social interactions for the emergence and stability of collective states.
These feedbacks incorporate both, the physics and behavioral side of collective behavior. Due to this combination, they have not been explored, to our best knowledge, in the field of 1) active aligning particle models [16, 18, 33, 34], which are often variants of the Vicsek model that either lack inertia, repulsion and/or speed variability (none of those is present in the Vicsek model), 2) active Brownian particles [35] which consider speed variablility and repulsion but operate normally in the over-damped limit (no inertia) and rarely take alignment into account, 3) burst-coast models [26–28, 36] which model swimming behavior in greater detail (distinct phases of de- and acceleration) but either speeds are picked randomly independent of social interactions and current state [27, 36] or the focus was to resemble experimentally observed individual behavior in detail without investigating emergent effects on the collective level [26, 28].
In general, individuals in a group can differ in their phenotype (e.g. animal personality) that can be strongly linked and result in differences in movement speed [3, 37–39]). The inter-individual variability in preferred movement speed has been found to influence spatial self-sorting (faster individuals sort to the front of the shoal), cohesion and polarization of groups [3, 21, 30], but its role decreases with larger group sizes [39]. Importantly, already in behaviorally homogeneous groups, with individuals having highly similar preferred speeds, their instantaneous speed will dynamically vary over time due to individuals’ direct response to social and environmental cues as well as internal decision processes. Both these types of speed variability will be important for the collective movement dynamics.
In this study, we focus on investigating the role of within-individual speed variability on emergent, self-organized collective movement using an agent-based model. It is meant to represent real animal groups, and we will discuss the role of inertia and friction and how these link speed, turning and social interactions. We will first provide an experimental motivation for our modeling ansatz by showing the ubiquity of speed variability in living and robotic fish and providing evidence for coupling between turning behavior and instantaneous speed, which can be theoretically understood by considering self-propelled movement with inertia. Inspired by these results, we will then investigate an agent-based model and demonstrate how the ability of individuals to flexibly adapt their speed in response to social interactions and fluctuations has major consequence for the emergent collective dynamics.
In order to determine the extent of within-individual variability in movement speeds, we analyzed previously published data sets of individual movement of the Trinidadian guppy [30] and individual and group movement of the clonal Amazon molly [38, 40] as well as a biomimetic robot (“RoboFish”, [3]). We include the RoboFish to highlight that the movement constraints due to inertia and turning friction are general and not limited to biological agents. All data sets consist of positional tracking data from laboratory observations with a sampling frame rate of 30 fps, circular or rectangular arenas smaller than 1 square meter in size and only female fish, as summarized in Table 1 and explained in more detail in Supplementary Material Section I.
TABLE 1. List of previously published tracking data used in our analysis. The table lists major characteristics of the datasets we used to show within-individual speed variability. The # of tracks indicates the number of individual tracks used for the analysis. Du to the initial study designs and questions, tracks may represent repeated measures of the same (Guppy: 20 individuals, RoboFish 1 replica) or different individuals (Molly single ind, Molly groups: 8 with 4 ind. per group). Please find exact study designs in the respective references.
The tracking data obtained for the different species and the robotic fish encodes the position
We can approximate the direction of motion of individual i by
The fundamental equation of motion for a self-propelled agent i reads:
with vi as the velocity vector of the agent, m it’s mass and Fi being total force acting on it. Please note that, in the following, we omit the explicit time dependence for simplicity. The velocity vector can be expressed via the speed vi and the heading angle φi to
Therefore, without any further assumptions, we see that the turning is inversely proportional to the current speed, i.e. dφi/dt ∝ 1/vi. However, the inverse proportionality results in instantaneous turning for vi = 0, which is unrealistic and is caused by assuming a point-like object. To provide a simple correction for this unreasonable assumption, we follow [43] and introduce a rotational friction force acting on the velocity
with α as rotational friction coefficient. If we repeat the steps from above analogously, the speed dynamics remain unchanged (Eq. 3) but the change in heading angle reads now:
In the context of self-propelled agents, the above relation implies that the turning rate of an individual, in response to a force Fi acting on the agent or generated by the agent itself, depends on its speed vi. For a constant force |Fi| = const., faster agents will turn slower. Alternatively, in order to turn at the same rate, individuals moving at different speeds have to adjust the strength of their turning force linearly with their current speed. We emphasize that this fundamental relation, ignored in most models of collective behavior explicitly modeling turning rates, holds both for fixed speeds vi≔v0,i = const., as well as for variable speeds vi≔vi(t).
We introduced in the preceding Section 2.3 Eq. 4 that accounts for inertia effects and Eq. 6 that additionally accounts for constraints due to turning friction. Here we treat the force in angular direction
As explained in Section 2.3, our model mimics the movement behavior of real fish by obeying fundamental physics relations (inertia and friction). This is mathematically expressed in Eqs 5, 6. Additionally, the interaction between fish is modelled by using the continuous version (i.e. overlapping instead of discrete zones) of a well established three-zone model that traditionally uses fixed speed [19]. The force acting on an individual i has a self-propulsion term (including noise) and a social term. We can express this as: Fi(t) = Fi,sp(t) + Fi,social(t). The self-propulsion force takes into account two main factors: 1) the tendency of an individual to keep a preferred speed v0 and 2) the fluctuations on the linear speed v and the angular speed
where β is the speed relaxation coefficient, leading to the relaxation of the speed towards the preferred speed v0 in the absence of other perturbations with the time constant τv = β−1. For solitary agents in the absence of external forces, the width of the speed distribution will be inversely proportional to β, i.e. low β corresponds to large speed variability and high β to small variability around v0. Dv and Dφ are diffusion coefficients setting the noise intensity in v and φ, respectively, whereas ξv and ξφ are independent, Gaussian white noise processes. The social interactions are explained in detail in the following.
We consider a social force that combines two fundamental types of interactions among individuals: 1) an alignment force Fi,alg and 2) a distance-regulating force Fi,d (Figures 1A,B). Thus, we can express the total social force as Fi,social(t) = Fi,alg(t) + Fi,d(t). We use Voronoi tesselation to define the neighborhood of a focal individual i, which is labeled as
where μalg is the alignment strength and vji(t) = vj(t) −vi(t). The distance-regulating social force assumes a preferred distance rd that individuals try to maintain between each other. It is defined as:
where
FIGURE 1. Implementation of social interactions. A focal agent (black triangle) responds to neighbors (red and blue triangle) via a velocity alignment force Fi,alg, which aims at minimizing the velocity difference to the mean neighbor velocity
By considering the self-propulsion and social forces described above, we can write the explicit equations of motion for individuals, which resemble the equations in [24]:
with
All simulations are performed with no boundary conditions (open space) and the model parameters are summarized in Table 2. The length scale is defined by the preferred distance rd = 1, which can be associated to the body size. With a preferred speed of v0 = 1 the agents travel on average in one time unit their preferred distance. With a distance regulating force strength of μd = 2 and β ≤ 2 agents are able to prevent a collision by stopping. The angular noise Dφ = 1 is the counterpart of the alignment strength μalg = 2 (same magnitude as μd), i.e. increasing one has the same effect as decreasing the other. Since the latter is varied in this study, the effect of both is explored. The same holds for the velocity noise Dv = 0.4 and the speed relaxation coefficient β. Note that with dimensionless equations, e.g. declaring the characteristic length and time as L = rd and T = rd/v0, two parameters could be reduced. However, to allow an easier interpretation, we refrain from doing so.
TABLE 2. Default model parameters. If figures represent simulation with a different set of parameters, it is explicitly stated in the caption. The units are given in general length L and time T units.
The two species of fish as well as the robotic agent exhibited qualitatively highly similar behavior: 1) non-negligible speed variability (Figures 2A–C), and 2) a strong decrease of turning rate with increasing speed (Figures 2E–G), which reflects the potential effects of inertia (see methods). Applying the same analyses on trajectories of our model simulation, we find the same patterns Figures 2D,H.
FIGURE 2. Speed and turning of individuals. The speed probability distributions P(v) for the experimental data (RoboFish, guppy, molly) and for model simulations of individuals (A–D). Red transparent lines represent single tracks and the solid black line is the distribution of all tracks summarized. The absolute turning rate
We find for all cases a speed distribution that shows a strong variation in speed (Figures 2A–D). The Coefficient of variation (COV(v) = σv/⟨v⟩) of individual speed for the different datasets is: COV(5) = 0.92 ± 28 (RoboFish), 0.57 ± 0.1 (guppy), 0.92 ± 0.78 (single molly), 0.68 ± 0.03 (model).
The model-fit to explain the four datasets was significantly (ΔAIC ∈ [31, 85], ΔBIC ∈ [28, 81]) improved by taking turning friction into account (Figures 2E–H; Table 3; same for the group molly data: inset Figure 2G).
TABLE 3. Statistical model comparison. Akaike (AIC) and Baysian (BIC) information criterion for a model without (
The individual turning of our simulated agents resembles qualitatively (in terms of the functional dependence on speed) the behavior of real fish. This allows us now to explore how social interactions in combination with variable speed and turning restriction affect collective behavior in groups with N = 400.
Animals can vary in their preferred speed v0, but also in their speed variability over time. We parametrized the latter by the speed relaxation coefficient β (see methods). For socially interacting agents, we find the mean individual speed ⟨v⟩ close to the preferred speed v0 but only in the ordered state (Figures 3A,B). Interestingly, it is possible to change groups from an ordered to a disordered state by just changing the preferred speed and/or the speed variability. As shown for real, robotic and simulated fish (Figures 2E–H) individuals’ turning rate is slower the higher their speed. This causes rotational random forces to be damped for groups with larger speeds, facilitating order due to inertial restrictions on turning (Figure 3B).
FIGURE 3. Influence of preferred speed and speed variability on polarization and speed-polarization coupling. The preferred speed v0 and the speed variability (modulated by the speed relaxation β) both affect the individual speed (A) and modulation of either of these parameters may induce orientational order marked by a high polarization Φ (B). The transition to order can be understood by a local coupling between the local average speed ⟨v⟩R and the local polarization ΦR, which we quantified via their correlation Corr (⟨v⟩R, ΦR) (C). The local averages consider all individuals within a circle of radius R = 3 around a focal one (red circle in inset (E). Note that ⟨v⟩R is not the local group velocity (where a positive correlation with order is trivial) but the local average of the individual speed magnitudes. The specific dependence between local speed and order for three specific parameter choices (marked by square, circle and cross in (C) is shown for the disordered state (D), the phase transition region (E) and the ordered state (F). A snapshot of a simulation (inset (E)) where agents with higher speed are darker colored, with parameters corresponding to the black circle in (C). The parameters of the simulations are listed in Table 2; Supplementary Table S5.
In contrast, large speed-variability (low β) may lead to disorder, while a narrow individual speed distribution (large β) induces order. If the speed of an agent can vary, the velocity alignment can reduce the average speed of individuals: A focal agent i matches its velocity (direction and magnitude) with the mean velocity of its neighbors
The speed variability has another highly robust emergent consequence. It allows agents of the same collective to differ in their instantaneous speed and since higher speeds induce order, we observe correlations on the local level between mean individual speed ⟨v⟩R and local polarization ΦR with R as the radius of the circle from which the average is computed (Figures 3C–F). Please note that as we consider individual speed, the above correlation is different from the trivial correlation between local polarization and local group speed. The correlations between individual speed and local polarization is always positive and largest at the transition between disorder and order. The latter is a signature of phase transitions, where the susceptibility, i.e. the response to weak signals/fluctuations, is maximal. It means that information encoded in speed is best translated to a directional response at the transition region, and vice versa (likely to be beneficial in collective computation tasks).
The local coupling is an emergent consequence of the fundamental dependence of turning on speed. Thus, it is highly robust and the qualitatively same non-linear functional form was observed in experiments (compare Figures 3D–F with Figure 1 in [25]). Most importantly, it weakens with low speed variability (Figure 3C) and does not exist for fixed speed models.
We have demonstrated above that the preferred speed and its variability can induce an order-disorder transition. Now, we keep the preferred speed fixed at v0 = 1 and change the alignment strength μalg. By repeating this for different speed relaxation β we investigate how the collective behaves in the ordered and disordered state (controlled by μalg) under different speed variabilities.
The higher the speed variability of individuals, the larger alignment strengths are necessary for the collective to reach the ordered state (Figure 4A). This shift of the phase transition is more clearly depicted by shifting peaks of the susceptibility χ (Figure 4A inset), which is defined by the fluctuations of the polarization χ = N (⟨Φ2⟩ − ⟨Φ⟩2).
FIGURE 4. Variable speed affects collective behavior in different states. Polarization Φ(A), the susceptibility χ (inset A), the individual speed ⟨s⟩ (B), the area of the convex hull (C) and the nearest neighbor distance NND (inset C) are shown in dependence on the velocity alignment strength μalg. Black crosses (B,C) mark the peak of the susceptibility, i.e. the location of the phase transition. The lines are color coded according to the speed relaxation as indicated at the colorbar, i.e. β ∈ {1, 2, 4, 8, 16, 32, 64}. The parameters of the simulations are listed in Table 2; Supplementary Table S5.
The collective phase transition impacts the individual dynamics as well: The mean individual speed ⟨v⟩ shows a distinct minimum at the transition which vanishes for a high speed variability (β = 1, Figure 4B). The minimum in speed is related to the velocity alignment where a focal agent adjusts its velocity vi to the average velocity vector of its neighbors
A very general qualitative change from fixed to variable speed can be observed in the group cohesion close to the phase transition. The area of the convex hull of the collective is maximal at the transition for fixed speeds. This maximum becomes less pronounced and finally vanishes with increasing speed variability (Figure 4C). The same holds for the nearest neighbor distance (Figure 4C inset). At the transition the directional correlation of the agents is maximal (i.e. susceptibility peaks, Figure 4A inset) and the directional fluctuations cause subgroups of the collective to head in different directions, leading to an expansion of the collective [19]. This expansion weakens with increasing speed variability because the distance regulating force can now lower the speed from a subgroup if it moves away from the shoal, effectively inhibiting expansion. A common consequence of weak cohesion is an increased probability for groups to split. However, for simplicity we assumed an unlimited attraction and alignment range, disabling fragmentation. The trend, that groups with a higher speed variability are more cohesive, is most striking at the transition, but holds in general in our model.
Group size is among the most biologically most important and variable parameters in the context of grouping. Thus, we investigate in this last part how group and individual measures change with group size N.
For low speed variability, the polarization remains high Φ ≲ 1 independent on N. If speed variability is high, polarization decreases with increasing group size and we expect Φ → 0 for even larger N (Figure 5A). Note that only in a narrow range close to the transition (marked by a large susceptibility, Figure 5B), the polarization saturates to intermediate values for large groups. The order-disorder transition for intermediate values of speed variability (β), is of the same nature as discussed for Figure 3A.
FIGURE 5. Group size effects depend on speed variability. The polarization Φ (A), the susceptibility χ(B) and the mean individual speed ⟨v⟩ (C) are shown for different speed relaxation β and group sizes N. The parameters of the simulations are listed in Table 2; Supplementary Table S5 with the difference of a preferred speed of v0 = 2.
It is of particular interest, given that the size of animal groups is a key parameter of collective behaviour, if there is a specific threshold where the collective patterns of the system show a qualitative change. We find that when modelling agents with high speed variability, the mean individual speed ⟨v⟩ in our model undergoes a sudden change at N = 3 (Figure 5C). Until N = 3 the individual speed ⟨v⟩ is larger than v0 and saturates towards v0 with decreasing speed variability. The reason for ⟨v⟩ > v0 is that the speed distribution of individuals is asymmetric, with a long-tail at large speeds but cutoff at low speeds at v = 0 (Figures 2A–D), i.e. a maximum of the distribution is at v = v0 but the mean is larger. For larger groups with N ≥ 4, the speed is lower than the preferred speed but saturates also to v0 in the fixed speed limit (β → ∞).
This abrupt change can be understood through the interplay of individual dynamics and fundamental property of the interaction network: 1) A focal agent decelerates stronger the more its heading deviates from the average polarization of its neighborhood, i.e.
FIGURE 6. Qualitative topological change with group size. Average Voronoi neighbor number for different group sizes. The dashed purple line (inset) marks the numbers of neighbors of a fully connected group. Illustrations of a typical spatial constellation for a group of size N = 4. The focal agent (0), its neighbors (1, 3) and an agent that is not connected to the focal agent (2) are shown. Note that only for group sizes N > 3 the latter exists, which hampers directional synchronization of the collective. The parameters of the simulations are listed in Table 2; Supplementary Table S5 with the difference of a preferred speed of v0 = 2.
In summary, for N ≤ 3 we have an all-to-all coupling, thus all agents receive the same social input, whereas for N > 3, centrally located individuals receive “independent” social inputs from different neighbors on different sides, which are not neighbors themselves, i.e. are not directly interacting. Thus, for N > 3 the centrally located individuals seek a compromise between two independent sources of information. As a consequence, the neighborhood of a focal agent located on the edge and the edge-agent itself, agree less in velocity. This results in slowing down the focal edge-agent, which in turn feeds back on the group behavior. To support this explanation we computed the vector product
We provide detailed empirical insights of speed variability in fish, providing evidence that inertia together with rotational friction explain the reduced turning ability of individuals at higher speeds. With our model that incorporates both, we explored the effect of speed variability on the emergent collective behavior. We found, among others, that 1) besides differences in (average) speed, also differences in individual speed variability (keeping preferred individual speed constant) can result in a change in polarization, 2) local coupling between speed and order is largest at the order-disorder transition, 3) individual speed variability decreases speed and increases cohesion at the phase transition and 4) the mean individual speed drops suddenly at a threshold group size (N > 3) but only at sufficiently high speed variability, which is intrinsically linked to the fundamental structure of the interaction network.
Our finding that higher speeds increase the polarization, is explained by the decrease of individuals turning rate at higher individual speeds that will inhibit individual directional noise, and thus facilitate stronger group polarization. Importantly, this speed-dependent turning effect comes on top of previously identified positive impact of higher speeds on group order [3]. The transition from ordered to disordered motion with speed was reported in experiments [22, 25, 29, 31]. However, corresponding models incorporating a dependence of turning rate on speed were based on fitting experimental data and not on the fundamental physics of inertia and rotational friction (see e.g. [22, 25]). This order-disorder transition induced by speed might enhance collective computation, such as the collective gradient sensing reported in golden shiners [1]: Fish swam fast in brighter and slow in darker regions and due to cohesion could collectively stay in the shade. A variable speed model that correctly accounts for inertia, could enhance the tendency of the collective to stay in the desired environment because there, low speeds increase disorder and thereby further decrease group speed prolonging the time in shade.
Additionally, we found that individual speed variability as well can change the polarization. The reason behind this dependence is speed matching, part of the social velocity alignment, that can lead to a decelerating social friction, where an agent adapts to the mean local group speed of its neighborhood [24]. We confirmed numerically (not shown) that this dependence vanishes if agents only align their orientation but do not match their speed. However, 1) this leads to extremely elongated groups that are unable to stay cohesive which is biologically unrealistic, 2) if agents ignore the speed of their conspecifics, flight cascades [51, 52] would not exist and 3) speed matching is experimentally confirmed for fish [3, 39]. Thus, our implementation of the alignment force, which incorporates speed matching, is very justified, and thus we also expect that the polarization dependence on speed variability is robust (implying our related findings as group size influence on speed and polarization).
We report a maximum of the local correlation between polarization and speed at the disorder-order transition and therefore elaborate the connection to collective computation by supporting the criticality hypothesis [53, 54]: information encoded in speed is most strongly linked to directional information at the transition, or the “critical point”. In other words, individuals within a group show the strongest response to directional information via speed adaptations and vise versa at this transition. We also found that a minimum in cohesion at the transition exists for fixed-speed models, but weakens or even vanishes with increasing speed variability. This has important implications for studies that investigate collective behavior at criticality [19]. For example, a weak cohesion is associated with a high probability of group splits (smaller group size) and lower densities. Both effects decrease the defense against predators (safety in number [55], confusion effect [56]) and thus make the transition region less biological favorable. This effect would weaken/vanish in a variable speed model. Bode et al. [36] predict an opposite effect of speed variability on cohesion in a burst coast model where the speed variability is modulated via the length of the bursts (phases of acceleration), whereby they refer to fish with shorter bursts as more agitated fish. Shorter bursts allow more direction changes per time and thus also a better response to positional information of con-specifics, enhancing cohesion while reducing speed variability. This discrepancy shows, that it is important to clarify in future research how the general speed variability (explored in our model) plays together with the characteristics of distinct movement phases.
In our model with a high speed variability (low β), we observe a decrease in polarization with increasing group size. Linking our results again to criticality: only at the disorder-order transition does the polarization saturates for large groups to intermediate values. Recently, the same functional dependence was experimentally observed and reproduced in a model with only pairwise interactions [23]. Thus, we present an alternative explanation only based on variability in individual speed. In general, specific experimental data can be mimicked by a multitude of models which differ strongly in their microscopic interactions [21, 28, 57–59]. However, those models are most often fit to a specific experimental setup, i.e. to a certain group size, tank size and depth, and need to be recalibrated if the setup changes [22]. We avoid this with a distance regulating and alignment force that have simple, yet generic forms motivated by experimental observations [2, 27, 49, 50]. Additionally, we ensured robustness of the local coupling by repeating the simulations with circular reflecting boundary conditions.
We report a sudden speed decrease in our variable speed model at a critical group size of N = 3, linked to a transition from an all-to-all network to a distributed spatial network. It might offer alternative means to test hypotheses about the underlying interaction network in real animal groups [48, 60]. In our model Voronoi-interactions cause the specific size threshold at N = 3, but for example for k-nearest neighbor interaction a group is all-to-all connected up to a threshold size directly set by k, i.e. for N < k. However, in order to observe this qualitative change the neighbors also need to match their speeds [3, 39] instead of only matching their movement direction, but, as discussed above, this assumption is reasonable. There might be also other limitations to this approach (e.g. we assume individual behavior does not change with group size [59]), however the emergent speed-interaction network coupling clearly shows how taking into account variable speed may introduce novel effects at the group level via the self-organized interplay of speed and orientation dynamics and social interactions.
To summarize, we have shown that speed variability affects polarization (on the local- and group level), cohesion (especially at the order-disorder transition) and can lead to new emergent transitions at specific groups sizes. Thus, we conclude that extreme caution should be taken when drawing strong conclusions on collective behavior of animal groups based on agent-based models with fixed speed.
The experimental data sets are available in repositories from previous articles ([38], [40], [30]). The code to run the agent-based model is available at github (https://github.com/PaPeK/swarm-variable-speed).
PK, LGN, and PR designed the analysis and wrote the paper. TL, JJ, and DB provided the experimental data and commented on the draft.
We received financial support by the DFG (German Research Foundation) under BI 1828/2-1, RO 4766/2-1, LA 3534/1-1 and under Germany’s Excellence Strategy—EXC 2002/1 “Science of Intelligence”—project number 390523135.
The authors declare that the research was conducted in the absence of any commercial or financial relationships that could be construed as a potential conflict of interest.
All claims expressed in this article are solely those of the authors and do not necessarily represent those of their affiliated organizations, or those of the publisher, the editors and the reviewers. Any product that may be evaluated in this article, or claim that may be made by its manufacturer, is not guaranteed or endorsed by the publisher.
We are thankful to Jens Krause for stimulating discussions.
The Supplementary Material for this article can be found online at: https://www.frontiersin.org/articles/10.3389/fphy.2021.715996/full#supplementary-material
1. Berdahl A, Torney CJ, Ioannou CC, Faria JJ, Couzin ID. Emergent Sensing of Complex Environments by Mobile Animal Groups. Science (2013) 339:574–6. doi:10.1126/science.1225883
2. Katz Y, Tunstrom K, Ioannou CC, Huepe C, Couzin ID. Inferring the Structure and Dynamics of Interactions in Schooling Fish. Proc Natl Acad Sci (2011) 108:18720–5. doi:10.1073/pnas.1107583108
3. Jolles JW, Boogert NJ, Sridhar VH, Couzin ID, Manica A. Consistent Individual Differences Drive Collective Behavior and Group Functioning of Schooling Fish. Curr Biol (2017) 27:2862–8. e7. doi:10.1016/j.cub.2017.08.004
4. Ward AJW, Herbert-Read JE, Sumpter DJT, Krause J. Fast and Accurate Decisions Through Collective Vigilance in Fish shoals. Proc Natl Acad Sci (2011) 108:2312–5. doi:10.1073/pnas.1007102108
5. Krause J, Godin JJ. Shoal Choice in the Banded Killifish (Fundulus diaphanus, Teleostei, Cyprinodontidae): Effects of Predation Risk, Fish Size, Species Composition and Size of Shoalsnkkk. Ethology (1994) 98:128–36. doi:10.1111/j.1439-0310.1994.tb01063.x
6. Torney CJ, Lorenzi T, Couzin ID, Levin SA. Social Information Use and the Evolution of Unresponsiveness in Collective Systems. J R Soc Interf (2015) 12:20140893. doi:10.1098/rsif.2014.0893
7. Carrillo JA, Fornasier M, Toscani G, Vecil F. Particle, Kinetic, and Hydrodynamic Models of Swarming. Math Model collective Behav Socio-Economic Life Sci (2010) 297–336. doi:10.1007/978-0-8176-4946-3_12
8. Ihle T. Kinetic Theory of Flocking: Derivation of Hydrodynamic Equations. Phys Rev E Stat Nonlin Soft Matter Phys (2011) 83:030901. doi:10.1103/PhysRevE.83.030901
9. Hidalgo J, Grilli J, Suweis S, Munoz MA, Banavar JR, Maritan A. Information-Based Fitness and the Emergence of Criticality in Living Systems. Proc Natl Acad Sci (2014) 111:10095–100. doi:10.1073/pnas.1319166111
10. Olson RS, Hintze A, Dyer FC, Knoester DB, Adami C. Predator Confusion Is Sufficient to Evolve Swarming Behaviour. J R Soc Interf (2013) 10:20130305. doi:10.1098/rsif.2013.0305
11. Li L, Nagy M, Graving JM, Bak-Coleman J, Xie G, Couzin ID. Vortex Phase Matching as a Strategy for Schooling in Robots and in Fish. Nat Commun (2020) 11:5408. doi:10.1038/s41467-020-19086-0
12. Landgraf T, Bierbach D, Nguyen H, Muggelberg N, Romanczuk P, Krause J. RoboFish: Increased Acceptance of Interactive Robotic Fish With Realistic Eyes and Natural Motion Patterns by Live Trinidadian Guppies. Bioinspir Biomim (2016) 11:015001. doi:10.1088/1748-3190/11/1/015001
13. Vicsek T, Zafeiris A. Collective Motion. Phys Rep (2012) 517:71–140. doi:10.1016/j.physrep.2012.03.004
14. Cavagna A, Cimarelli A, Giardina I, Parisi G, Santagati R, Stefanini F, et al. Scale-Free Correlations in Starling Flocks. Proc Natl Acad Sci (2010) 107:11865–70. doi:10.1073/pnas.1005766107
15. Feinerman O, Pinkoviezky I, Gelblum A, Fonio E, Gov NS. The Physics of Cooperative Transport in Groups of Ants. Nat Phys (2018) 14:1–11. doi:10.1038/s41567-018-0107-y
16. Chaté H, Ginelli F, Grégoire G, Peruani F, Raynaud F. Modeling Collective Motion: Variations on the Vicsek Model. Eur Phys J B (2008) 64:451–6. doi:10.1140/epjb/e2008-00275-9
17. Peruani F, Klauss T, Deutsch A, Voss-Boehme A. Traffic Jams, Gliders, and Bands in the Quest for Collective Motion of Self-Propelled Particles. Phys Rev Lett (2011) 106:128101. doi:10.1103/physrevlett.106.128101
18. Vicsek T, Czirók A, Ben-Jacob E, Cohen I, Shochet O. Novel Type of Phase Transition in a System of Self-Driven Particles. Phys Rev Lett (1995) 75:1226–9. doi:10.1103/physrevlett.75.1226
19. Klamser PP, Romanczuk P. Collective Predator Evasion: Putting the Criticality Hypothesis to the Test. PLOS Comput Biol (2021) 17:1–21. doi:10.1371/journal.pcbi.1008832
20. Jolles JW, King AJ, Killen SS. The Role of Individual Heterogeneity in Collective Animal Behaviour. Trends Ecol Evol (2020) 35:278–91. doi:10.1016/j.tree.2019.11.001
21. Couzin ID, Krause J, James R, Ruxton GD, Franks NR. Collective Memory and Spatial Sorting in Animal Groups. J Theor Biol (2002) 218:1–11. doi:10.1006/jtbi.2002.3065
22. Gautrais J, Ginelli F, Fournier R, Blanco S, Soria M, Chaté H, et al. Deciphering Interactions in Moving Animal Groups. Plos Comput Biol (2012) 8:e1002678–11. doi:10.1371/journal.pcbi.1002678
23. Jhawar J, Morris RG, Amith-Kumar UR, Danny Raj M, Rogers T, Rajendran H, et al. Noise-Induced Schooling of Fish. Nat Phys (2020) 16. doi:10.1038/s41567-020-0787-y
24. Großmann R, Schimansky-Geier L, Romanczuk P. Active Brownian Particles With Velocity-Alignment and Active Fluctuations. New J Phys (2012) 14:073033. doi:10.1088/1367-2630/14/7/073033
25. Mishra S, Tunstrøm K, Couzin ID, Huepe C. Collective Dynamics of Self-Propelled Particles With Variable Speed. Phys Rev E Stat Nonlin Soft Matter Phys (2012) 86:011901. doi:10.1103/PhysRevE.86.011901
26. Harpaz R, Tkačik G, Schneidman E. Discrete Modes of Social Information Processing Predict Individual Behavior of Fish in a Group. Proc Natl Acad Sci USA (2017) 114:10149–54. doi:10.1073/pnas.1703817114
27. Calovi DS, Litchinko A, Lecheval V, Lopez U, Pérez Escudero A, Chaté H, et al. Disentangling and Modeling Interactions in Fish With Burst-And-Coast Swimming Reveal Distinct Alignment and Attraction Behaviors. Plos Comput Biol (2018) 14:e1005933. doi:10.1371/journal.pcbi.1005933
28. Sbragaglia V, Klamser PP, Romanczuk P, Arlinghaus R. Evolutionary Impact of Size-Selective Harvesting on Shoaling Behavior: Individual-Level Mechanisms and Possible Consequences for Natural and Fishing Mortality. review Am Naturalist (2020). doi:10.1101/809442
29. Kent MIA, Lukeman R, Lizier JT, Ward AJW. Speed-Mediated Properties of Schooling. R Soc Open Sci (2019) 6:181482. doi:10.1098/rsos.181482
30. Jolles JW, Weimar N, Landgraf T, Romanczuk P, Krause J, Bierbach D. Group-Level Patterns Emerge From Individual Speed as Revealed by an Extremely Social Robotic Fish. Biol Lett (2020) 16:20200436. doi:10.1098/rsbl.2020.0436
31. Tunstrøm K, Katz Y, Ioannou CC, Huepe C, Lutz MJ, Couzin ID. Collective States, Multistability and Transitional Behavior in Schooling Fish. Plos Comput Biol (2013) 9:e1002915. doi:10.1371/journal.pcbi.1002915
32. Calovi DS, Lopez U, Ngo S, Sire C, Chaté H, Theraulaz G. Swarming, Schooling, Milling: Phase Diagram of a Data-Driven Fish School Model. New J Phys (2014) 16:015026. doi:10.1088/1367-2630/16/1/015026
33. Toner J, Tu Y. Flocks, Herds, and Schools: A Quantitative Theory of Flocking. Phys Rev E (1998) 58:4828–58. doi:10.1103/PhysRevE.58.4828
34. Toner J, Guttenberg N, Tu Y. Swarming in the Dirt: Ordered Flocks With Quenched Disorder. Phys Rev Lett (2018) 121:248002. doi:10.1103/physrevlett.121.248002
35. Cates ME, Tailleur J. Motility-Induced Phase Separation. Annu Rev Condens Matter Phys (2015) 6:219–44. doi:10.1146/annurev-conmatphys-031214-014710
36. Bode NWF, Faria JJ, Franks DW, Krause J, Wood AJ. How Perceived Threat Increases Synchronization in Collectively Moving Animal Groups. Proc R Soc B (2010) 277:3065–70. doi:10.1098/rspb.2010.0855
37. Réale D, Reader SM, Sol D, McDougall PT, Dingemanse NJ. Integrating Animal Temperament Within Ecology and Evolution. Biol Rev (2007) 82:291–318. doi:10.1111/j.1469-185X.2007.00010.x
38. Bierbach D, Laskowski KL, Wolf M. Behavioural Individuality in Clonal Fish Arises Despite Near-Identical Rearing Conditions. Nat Commun (2017) 8. doi:10.1038/ncomms15361
39. Herbert-Read JE, Krause S, Morrell LJ, Schaerf TM, Krause J, Ward AJW. The Role of Individuality in Collective Group Movement. Proc R Soc B (2013) 280:20122564. doi:10.1098/rspb.2012.2564
40. Doran C, Bierbach D, Laskowski KL. Familiarity Increases Aggressiveness Among Clonal Fish. Anim Behav (2019) 148:153–9. doi:10.1016/j.anbehav.2018.12.013
41. Mönck HJ, Jörg A, von Falkenhausen T, Tanke J, Wild B, Dormagen D, et al. BioTracker: An Open-Source Computer Vision Framework for Visual Animal Tracking. arXiv (2018).
42. Romanczuk P, Bär M, Ebeling W, Lindner B, Schimansky-Geier L. Active Brownian Particles. Eur Phys J Spec Top (2012) 202:1–162. doi:10.1140/epjst/e2012-01529-y
43. Romanczuk P. Active Motion and Swarming. From Individual to Collective Dynamics. Berlin: Logos Verlag (2011) 12.
44. Akaike H. A New Look at the Statistical Model Identification. IEEE Trans Automat Contr (1974) 19:716–23. doi:10.1109/TAC.1974.1100705
45. Schwarz G. Estimating the Dimension of a Model. Ann Statist (1978) 6:461–4. doi:10.1214/aos/1176344136
46. Ward EJ. A Review and Comparison of Four Commonly Used Bayesian and Maximum Likelihood Model Selection Tools. Ecol Model (2008) 211:1–10. doi:10.1016/j.ecolmodel.2007.10.030
47. Wit E, Heuvel Ev. d., Romeijn J-W. 'All Models Are Wrong.': an Introduction to Model Uncertainty. Stat Neerlandica (2012) 66:217–36. doi:10.1111/j.1467-9574.2012.00530.x
48. Strandburg-Peshkin A, Twomey CR, Bode NWF, Kao AB, Katz Y, Ioannou CC, et al. Visual Sensory Networks and Effective Information Transfer in Animal Groups. Curr Biol (2013) 23:R709–R711. doi:10.1016/j.cub.2013.07.059
49. Lukeman R, Li Y-X, Edelstein-Keshet L. Inferring Individual Rules From Collective Behavior. Proc Natl Acad Sci USA (2010) 107:12576–80. doi:10.1073/pnas.1001763107
50. Herbert-Read JE, Perna A, Mann RP, Schaerf TM, Sumpter DJT, Ward AJW. Inferring the Rules of Interaction of Shoaling Fish. Proc Natl Acad Sci (2011) 108:18726–31. doi:10.1073/pnas.1109355108
51. Rosenthal SB, Twomey CR, Hartnett AT, Wu HS, Couzin ID. Revealing the Hidden Networks of Interaction in Mobile Animal Groups Allows Prediction of Complex Behavioral Contagion. Proc Natl Acad Sci USA (2015) 112:4690–5. doi:10.1073/pnas.1420068112
52. Sosna MMG, Twomey CR, Bak-Coleman J, Poel W, Daniels BC, Romanczuk P, et al. Individual and Collective Encoding of Risk in Animal Groups. Proc Natl Acad Sci USA (2019) 116:20556–61. doi:10.1073/pnas.1905585116
53. Mora T, Bialek W. Are Biological Systems Poised at Criticality? J Stat Phys (2011) 144:268–302. doi:10.1007/s10955-011-0229-4
54. Muñoz MA. Colloquium: Criticality and Dynamical Scaling in Living Systems. Rev Mod Phys (2018) 90:31001. doi:10.1103/RevModPhys.90.031001
56. Milinski M. Experiments on the Selection by Predators against Spatial Oddity of Their Prey. Z für Tierpsychologie (1977) 43:311–25. doi:10.1111/eth.1977.43.issue-2
57. Bastien R, Romanczuk P. A Model of Collective Behavior Based Purely on Vision. Sci Adv (2020) 6:eaay0792–10. doi:10.1126/sciadv.aay0792
58. Romanczuk P, Couzin ID, Schimansky-Geier L. Collective Motion Due to Individual Escape and Pursuit Response. Phys Rev Lett (2009) 102:010602. doi:10.1103/PhysRevLett.102.010602
59. Bode NWF, Seitz MJ. Using Hidden Markov Models to Characterise Intermittent Social Behaviour in Fish shoals. Sci Nat (2018) 105:7. doi:10.1007/s00114-017-1534-9
Keywords: collective motion, biophysics, mathematical models, variable speed, social interactions, group size, phase transition
Citation: Klamser PP, Gómez-Nava L, Landgraf T, Jolles JW, Bierbach D and Romanczuk P (2021) Impact of Variable Speed on Collective Movement of Animal Groups. Front. Phys. 9:715996. doi: 10.3389/fphy.2021.715996
Received: 27 May 2021; Accepted: 25 August 2021;
Published: 15 September 2021.
Edited by:
Elena Agliari, Sapienza University of Rome, ItalyReviewed by:
Shradha Mishra, Indian Institute of Technology (BHU), IndiaCopyright © 2021 Klamser, Gómez-Nava, Landgraf, Jolles, Bierbach and Romanczuk. This is an open-access article distributed under the terms of the Creative Commons Attribution License (CC BY). The use, distribution or reproduction in other forums is permitted, provided the original author(s) and the copyright owner(s) are credited and that the original publication in this journal is cited, in accordance with accepted academic practice. No use, distribution or reproduction is permitted which does not comply with these terms.
*Correspondence: Pawel Romanczuk, cGF3ZWwucm9tYW5jenVrQGh1LWJlcmxpbi5kZQ==
†ORCID: Pascal Klamser, orcid.org/0000-0003-2208-4391; David Bierbach, orcid.org/0000-0001-7049-2299; Pawel Romanczuk, orcid.org/0000-0002-4733-998X; Luis Gómez-Nava, orcid.org/0000-0002-2426-5906; Jolle W. Jolles, orcid.org/0000-0001-9905-2633
Disclaimer: All claims expressed in this article are solely those of the authors and do not necessarily represent those of their affiliated organizations, or those of the publisher, the editors and the reviewers. Any product that may be evaluated in this article or claim that may be made by its manufacturer is not guaranteed or endorsed by the publisher.
Research integrity at Frontiers
Learn more about the work of our research integrity team to safeguard the quality of each article we publish.