- Faculty of Law, Hebrew University of Jerusalem, Jerusalem, Israel
The global effort to fight the Covid-19 pandemic triggered the adoption of unusual legal measures that restrict individual freedoms and raise acute legal questions. Yet, the conventional legal tools available to analyze those questions—including legal notions such as proportionality, equality, or the requisite levels of evidence—implicitly presume stable equilibria, and fail to capture the nonlinear properties of the pandemic. Because the pandemic diffuses in a complex system, using complexity theory can help align the law with its dynamics and produce a more effective legal response. We demonstrate how insights from complexity concerning temporal and spatial diffusion patterns, or the structure of the social network, can provide counter-intuitive answers to a series of pandemic-related legal questions pertaining to limitations of movement, privacy, business and religious freedoms, or prioritizing access to vaccines. This analysis could further inform legal policies aspiring to handle additional phenomena that diffuse in accordance with the principles of complexity.
Introduction
Covid-19 is presenting unprecedented legal challenges to traditional legal policy. The effort to curb the pandemic entails the adoption of unparalleled measures that seriously compromise fundamental legal rights, ranging from free movement, to privacy, to the right to conduct business, or to carry out religious practices. The uneven spread of the pandemic within geographical areas triggers differentiated policy responses that ostensibly collide with legal notions of equality, whereas the high levels of uncertainty and unpredictability concerning the diffusion of the disease challenge the traditional legal stance that policy measures must rely on solid evidence.
Law and policy proposals have so far concentrated on insights from behavioral economics, which could assist policy makers in designing their response to the pandemic, for example, by effectively nudging people toward adopting desirable behaviors (e.g., [1]; cf. [2, 3]). This contribution wishes to draw the attention to an additional perspective for improving the legal response to Covid-19, that of complex systems.
The pandemic is a systemic phenomenon. While there are still indeterminacies as to its precise attributes, there is ample evidence that its general diffusion is consistent with temporal exponential dynamics and spatial fractal patterns that characterize diffusion in complex systems. Yet, many of the legal principles that are employed in handling the current challenges—including notions such as “proportionality,” “equality,” or “requisite levels of evidence” — were designed against implicit assumptions of stable equilibria and linear processes, and are therefore misaligned with the traits of the pandemic. We seek to mitigate this disconnect, and show how engaging with complexity theory can help calibrate legal policies to produce a more effective legal response to Covid-19.
Methodologically, our analysis relies both on specific research concerning the Covid-19 pandemic and its diffusion patterns, as well as on a large body of scholarship from the recent decades, in physics, network science and adjacent areas, that investigated and delineated the general traits of complex systems. While this literature increasingly influences numerous domains, its use in theoretical legal analysis, or in legal policy design is still relatively limited (cf. [4–6]). The following analysis demonstrates how engaging with insights from complexity theory can shed light on a series of pandemic-related legal questions, concentrating on four acute examples: (1) the legality of regulatory measures that limit individual liberties; (2) evidentiary questions concerning pandemic-related decision making; (3) the application of differentiated policies to various geographical areas; and (4) the use of network tools for prioritizing tests and vaccines. Our examples are illustrative and non-exhaustive. Rather, this perspective invites additional research that would assist in integrating the science of complex systems into legal policy, to improve the legal response to the pandemic, as well as to additional phenomena that diffuse in accordance with the principles of complexity, including, prominently, environmental challenges.
Restrictive Measures: Proportionality and Exponential Dynamics
Many unusual measures that were adopted in the face of Covid-19 since March, 2020—including lockdowns, borders closures, the use of contact tracing technologies, the closure of businesses and restrictions on religious practices—were challenged before various courts worldwide (e.g., cases #4-21)1. In many jurisdictions, the legal scrutiny of those steps, and similar measures that restrict individual liberties, utilizes the notion of proportionality. Proportionality implies a balancing exercise: the steps imposed by governments or regulators must be weighted against the social harm they seek to prevent (e.g., [7, 8]). Relatedly, under prevalent legal doctrine, when the general social interest requires restricting or prejudicing individual rights, regulators must choose the “least restrictive means,” namely only the necessary and most lenient measures available, which least interfere with individual liberties (e.g., [9], p. 464; [10, 11]). Applying these principles, courts in various countries ruled that measures such as lockdowns (cases #16, #19), restrictions on worship (case #4–10), or business closures (cases #11, #18) are disproportionate and illegal.
Both the proportionality principle and the “least restrictive means” principle envisage ordinary circumstances, where the restrictive steps are balanced against potential harms that are either relatively stable, or increase linearly with time. Consider, for example, the case of preventing a possible terrorist attack that could harm dozens of people. Under standard legal analysis, taking certain steps that compromise individual freedoms—such as instructing people to take off their shoes at airport security—in order to prevent this outcome would be considered proportionate, whereas adopting more extreme measures, such as a complete closure of borders would likely fail both the proportionality and the least-restrictive-means tests.
However, the assumptions underlying the proportionality test do not accurately reflect phenomena diffusing in complex systems. Typically, such diffusion displays temporal growth patterns that are non-linear. More specifically, while during the initial stages the rate of diffusion could be relatively stable, at a certain point, once contagion processes take off, it is expected to rise exponentially, leading to a sharp increase in the spread of the relevant phenomenon (e.g., [12–14]). The evidence concerning Covid-19 instructs that the pandemic indeed diffuses in accordance with these patterns. Figure 1, which describes the cumulative number of Covid-19 cases in the UK during February-March 2020 illustrates these dynamics2.
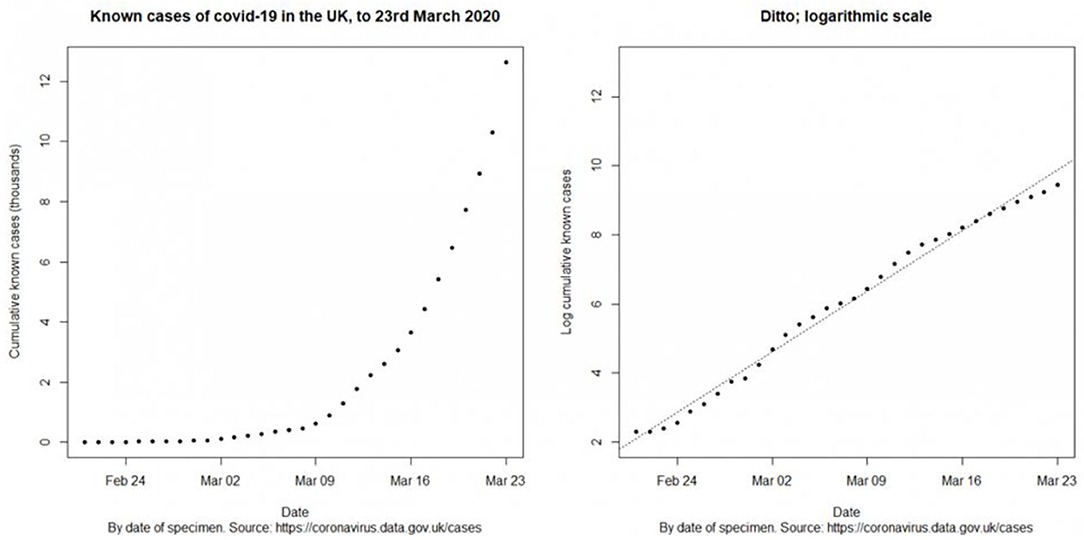
Figure 1. The cumulative number of Covid-19 cases in the UK as a function of time in linear (left) and a log-log (right) representations (source: Stevens et al. [15]).
As a result of the non-linear diffusion dynamics, the expected number of people contracting the disease is likely to grow exponentially, while the effectiveness of regulatory measures employed to restrain its diffusion is likely to significantly decline with time. To illustrate, let us consider three points in time in Figure 1. At T1 = Feb 24, the number of confirmed cases in the UK was still very small (13 cumulative cases), and the diffusion relatively slow. Two weeks later, at T2 = March 9, the number of cases was 677 and was growing exponentially, doubling itself every 3–4 days, so that at T3 = March 23, the disease was already widespread within the population, reaching 12,647 cumulative cases. Applying strict measures such as border closure at T1 can effectively curb a pandemic (provided, of course, it is accompanied by additional inter-state actions of contact tracing). Yet this same measure is much less effective if adopted only 2 weeks later (at T2), and almost insignificant at T3, when the disease has spread among the entire state's population so that a few more cases “imported” from other infected countries would not result in a substantial change.
Yet, from the perspective of law and policy making exponential growth may be hard to grasp: many serious problems that beg policy responses, from road accidents to the prevalence of cancer, do not exhibit these growth dynamics. Moreover, because diffusion starts slowly and steadily, it might be difficult, in early stages, to distinguish exponential growth from linear growth and realize the huge potential magnitude thereof, a phenomenon known as the “exponential growth bias” (e.g., [16, 17]). As a result, courts may be inclined to apply a conventional proportionality test, implicitly presuming that the pandemics' harms will grow in a stable and linear way.
The lens of complexity implies that, contrary to conventional legal analysis, the regulatory response to a pandemic may, in certain cases, warrant an “inverse proportionality test.” When the diffusion dynamics are nonlinear and the potential harm is likely to accumulate exponentially, strict measures to prevent it could be considered proportionate at an early stage, when the actual harm is least apparent and least certain. Counterintuitively, those very same measures might be less defensible at a later stage when the large harms of the pandemic have already materialized. To illustrate, a legal proportionality test that is adapted to nonlinear dynamics would ratify China's decision to test nine million people in October 2020, in light of only a dozen detected cases of Covid-19 [18]. Similarly, New Zealand's “Go Hard, Go Early” policy, which entailed early border closure and a full lockdown before there was even a single death from Covid-19 in the country [19] would be considered proportionate. Notably, this policy, which very much aligns with the dynamics of complex systems, has also turned out to be extremely effective in controlling the pandemic. Conversely, the same “inverse proportionality test” might not endorse Israel's second border closure, imposed in September 2020, when the number of active cases in the country has already soared and reached tens of thousands of cases [20]3.
From a legal perspective, one might argue that instead of an “inverse proportionality test” our insights can be incorporated into the current proportionality doctrine, so that courts would simply take into account the complex systems properties of the relevant phenomenon, when assessing the proportionality of the state's response. Ideally, this approach would yield similar results. Yet from a practical perspective, framing the test as an “inverse proportionality test” has several advantages. First, it signals to courts and policy makers (who might not always be familiar with complexity theory) that certain phenomena warrant a qualitatively different response relative to “ordinary” situations. Secondly, it facilitates recognition that seemingly small current problems might justify major interventions. Third, it illuminates that in certain circumstances, late interventions, which may seem proportionate, might actually be no longer effective4.
We do not delineate here a comprehensive set of circumstances that warrant an “inverse proportionality” test, beyond the case of Covid-19. As a rule of thumb, an inverse proportionality test could be appropriate when dealing with multiplicative (rather than additive) risks, that pose systemic threats, particularly when the relevant phenomenon diffuses in short time scales. These factors, and their further development in future research, could guide legal policy and prevent the misuse of the test in other, more ordinary circumstances.
Relatedly, a complexity-based analysis warrants the calibration of the legal requirement of “least restrictive means” to the stage of diffusion. The implicit assumptions underlying this legal doctrine—that the “second-best” and least restrictive measures are close-enough to the more restrictive measures, or that a gradual escalation of means is a feasible and reasonable policy—do not hold true in the face of exponential growth. Rather, under exponential diffusion dynamics adopting “very” or even “most” restrictive means at a very early stage may be orders of magnitude more effective than adopting “less” or “least” restrictive means.
A comparison of the measures imposed by Greece and Spain during the first wave of the pandemic, as described in Figure 2, is illustrative. The two countries started out with roughly the same number of cases. Within 2 weeks, Greece imposed “very restrictive measures,” including border closure and a full lockdown, while Spain applied a “least restrictive measures” approach, gradually escalating from a partial lockdown to a full lockdown after 4 weeks. After 30 days, the number of daily cases in Greece was less than a hundred, while the number of cases in Spain was in the range of tens of thousands5.
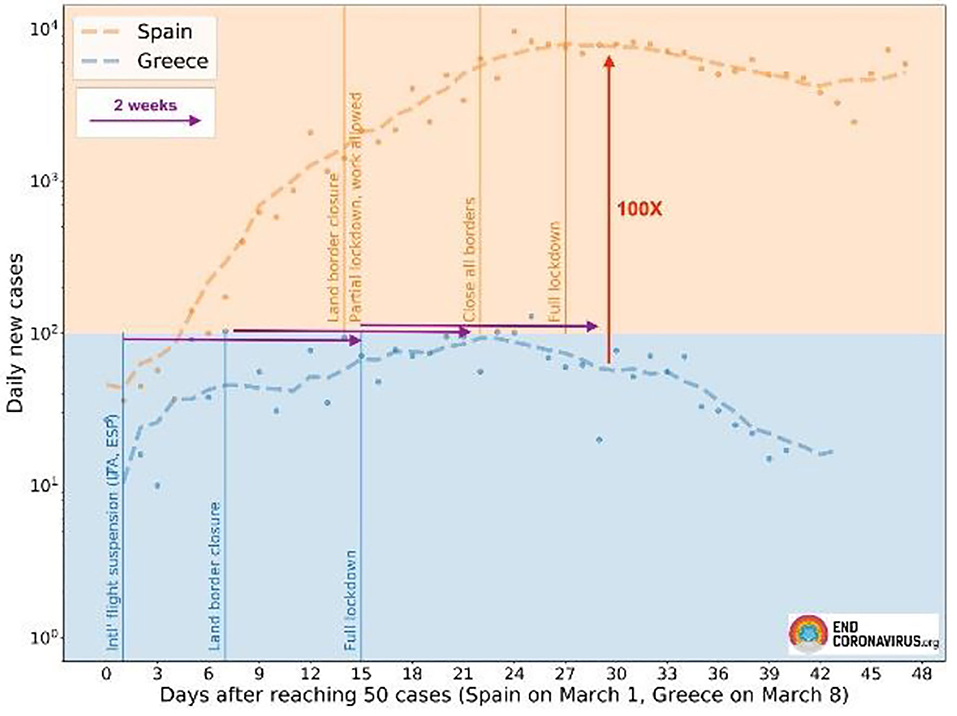
Figure 2. Escalation of Measures: Spain v. Greece (Source: Yaneer Bar-Yam, EndCoronavirus.org).
Adapting law to exponential diffusion dynamics therefore implies that, as part of the inverse proportionality test, the adoption of harsher (rather than least restrictive) means at an early stage of diffusion can be considered proportionate, and legal6.
Complexity, Evidence, and Decision Making
Understanding complex systems can further illuminate a series of legal questions concerning evidence and decision making during the pandemic.
First, which evidence is required before adopting pandemic-related decisions by states and regulators? Under conventional legal principles, common to numerous jurisdictions, government decision-making should be based on evidence, and informed by the best available data (e.g., [21]). These principles apply in particular force when state's policies infringe fundamental rights. In such circumstances the state needs to prove, on the basis of reliable evidence, that its actions are in fact necessary, and the standard of proof would generally be higher than in regular civil cases [e.g., [22]].
In the context of Covid-19, various scholars and policy makers advocated that the imposition of restrictive measures lacked sufficient evidentiary basis, and called for “more reliable data” before adopting “draconian countermeasures” (e.g., [23]). Concomitantly, there have been continuous scientific efforts to produce single point forecasts predicting the pandemic's spread, through the use of various mathematical models (such as the logistic model, the SIR model, agent-based models, and variations thereof).
The lens of complexity instructs that the nonlinear properties of the pandemic render the ordinary legal expectation for accurate, specific, predictions largely unrealistic. Every mathematical model inevitably involves a degree of simplification and idealization. In linear systems certain simplification—which entails neglecting certain properties of the modeled phenomenon—is unlikely to bring about significant differences in the overall prediction [24]. Conversely, in complex nonlinear dynamic systems like the Covid-19 pandemic, slight differences in initial conditions assumed by mathematical models, can yield extreme differences in the total outcome. The precise interactions and interdependencies among the various components comprising the system, the system's structure, the sequence of interactions and other factors, that might be minuscule or random, especially during the initial stages of diffusion, can all result in vast differences in the system's overall response (e.g., [25–27]).
Research pertaining to Covid-19 indeed demonstrates how very small differences in the pandemic's growth rate, in the precise implementation details of the regulatory interventions, or in the population's degree of compliance with those regulations, may yield vast changes in the disease's trajectory [28]. These nonlinear dynamics make single point forecasts essentially impossible, and even led some scholars to maintain that the turning point of the epidemic, namely the point where the growth in the number of cases starts decreasing, cannot be predicted with any certainty before it actually occurs [29].
Moreover, pandemics are “fat-tailed” events, which implies that “frequent” (median) data observations do not provide a good indication of the average, or the magnitude, of the overall phenomenon (e.g. [30–32])7. This property further explains why Covid-19 does not lend itself to simple predictions. It also clarifies that additional data, especially observations coming from the bulk of the distribution–for example, data on the daily numbers of cases during the beginning of a pandemic–does not guarantee extra knowledge that will allow more accurate prediction of the overall phenomenon.
Due to these properties, waiting for more positive evidence will not necessarily produce more meaningful information for policy making. Furthermore, in the face of exponential diffusion postponing interventions in the hope of gathering “more data” is expected to make such interventions less effective and costlier. Rather than insist on precise predictions that are likely impossible, courts and policy makers may have to “satisfice” with evidence concerning the general properties of the Covid-19 pandemic as a non-linear, unstable and essentially collective phenomenon that diffuses exponentially. These properties are familiar characteristics of complex systems for which there is ample proof. The foregoing analysis also implies that the legal approach to the pandemic should be responsive, maintain flexibility, and take into account its inherent unpredictability (cf. [33]).
Nevertheless, courts applying judicial review of pandemic-related decisions sometimes tend to apply the conventional legal prescription and insist on more accurate data that cannot be realistically obtained. One illustration is a decision of the United States Court of Appeals for the 3rd Circuit in the case of United States v. Raia from April, 2020 (Case # 1). The court denied a criminal defendant's motion for compassionate release from prison given the Covid-19 pandemic, despite his numerous medical risk factors. The decision held that the applicant must exhaust administrative remedies prior to seeking relief in court. The “mere possibility” that the pandemic would spread in the prison system and harm him was insufficient for immediate compassionate release. The implicit requirement for more precise and concrete evidence may constitute an appropriate legal standard of review under ordinary circumstances. Yet, due to the nonlinear properties of Covid-19, satisficing with “mere possibility” might be inevitable.
Secondly, understanding the properties of complex phenomena should also influence the weight and evaluation of evidence. Both policy makers and legal professionals are trained to evaluate evidence according to past life-experiences, which are assumed to remain more or less stable. In the context of Covid-19, this conventional method may lead courts and policy makers to interpret lack of evidence that a certain activity triggers infections, as positive evidence that this activity is in fact “safe” (cf. [34]). For example, a research that examined over 3,000 people who trained in a gym in Oslo during May 2020 found no infected cases and concluded that “provided good hygiene and social distancing measures, there was no increased Covid-19 spread at training facilities.” ([35], p. 2). This research was subsequently presented to the Israeli Parliament as positive evidence that opening gyms entails no added risk for infections ([36], p. 14). However, during the period of the study the pandemic in Oslo was in its early stages, and the number of new daily cases in the city was practically zero8. Taking into account the stage of diffusion, this absence of evidence about infections cannot be translated into meaningful knowledge about absence of risk for infections in gyms. The situation could be different in later stages of the pandemic's diffusion, where similar evidence, if obtained, may be more meaningful.
Finally, and relatedly, conventional legal analysis of evidence usually adopts a reductionist perspective that concentrates on an individual person or action (such as a particular crime, or a specific transaction) (cf. [37]) Therefore, policy makers adopting pandemic-related decisions, and courts reviewing such decisions, may wrongly apply a simple “additive” approach, assuming that because the risk to each individual and from each individual activity is low, the systemic risk caused by the pandemic is equally small ([31], p. 607). However, acquaintance with the properties of complex systems clarifies that, due to the interactions among individuals and the multiplicative dynamics of the pandemic, these small risks at the micro level can easily translate into a large systemic risk resulting in millions of death at the macro level, as indeed has been the case with Covid-199. A complexity-informed approach therefore prescribes that the general evidentiary trajectory, which should guide judicial and regulatory decision-making in light of the uncertainties entailed in Covid-19 is to err on the side of safety.
The legal doctrine known as “the precautionary principle” echoes this logic. According to this principle “[w]hen an activity raises threats of harm to human health or the environment, precautionary measures should be taken even if some cause-and-effect relationships are not fully established scientifically. In this context the proponent of an activity, rather than the public, should bear the burden of proof” ([39]; cf. [40]). Originating in environmental and international law, the precautionary principle was adopted by courts and regulators in various jurisdictions (e.g., [34, 41]). Concomitantly, it has been subject of intense criticism, maintaining, essentially, that it is vague and fails to provide guidance sensitive to cost-benefit analysis (e.g., [42]). A complexity-based approach can alleviate some of these concerns by delineating several factors, which may serve as guidelines for the principle's application: phenomena that spread exponentially, in short time-scales, and pose systemic, existential, risks10. Under such circumstances, the risk is multiplicative while the costs of adopting precautionary measures are often additive–which indicates that under a cost-benefit analysis the overarching trajectory points toward precaution. Covid-19 may be a paradigmatic example, especially given its rapid diffusion, but it is not a single case. Legal scholarship has already observed that global environmental threats, particularly climate change, may pose unique systemic challenges to the legal system (e.g., [43, 44]). Increasing evidence suggests that phenomena related to climate change display exponential properties, albeit on a different time-scale, and similarly entail embedded uncertainty (e.g., [34, 45, 46]). The foregoing analysis indicates that courts and policy makers should favorably consider applying the precautionary principle in these and similar instances.
Differential Treatment, Equality, and Fractals
In addition to the general legality of restricting basic freedoms, the response to the Covid-19 pandemic raises acute legal questions concerning equality. A central debate in this respect relates to the legitimacy of collective differential measures, primarily localized lockdowns implemented over a limited geographical area–ranging from small-scale units such as neighborhoods, to larger scales such as cities or regions.
Unlike nation-wide lockdowns, which limit basic rights equally, localized lockdowns create substantial inequalities in the limitations they impose on basic rights, and might be conceived as a violation of the right to be treated equally. Such differential lockdowns may seem particularly troublesome when the geographical region in question is mostly populated with ethnic minorities or disadvantaged groups, so that, even in the absence of intention to disadvantage the members of a particular group, the measures might be interpreted as “indirect discrimination” (cf. [47]).
Indeed, several lawsuits filed during the pandemic contested the legality of differential lockdowns, arguing discrimination and lack of evidence as to their effectiveness (e.g., Cases # 20–21). Another contentious question is the relevant scale for imposing differential measures (e.g., neighborhoods, cities, counties, etc.). For example, one lawsuit requested the court to order that a differential lockdown on a certain neighborhood be narrowed down to specific streets within that neighborhood (Case # 21).
The lens of complexity can shed light on questions pertaining to the legality of differential lockdowns, by providing a nuanced understanding of the spatiotemporal diffusion patterns of the pandemic.
The long-established SIR model for analyzing the spread of contagious diseases, traditionally assumed homogenous mixed populations [48]. However, complexity instructs that spatial diffusion in complex systems is usually non-homogenous. Rather, it often displays a spatial fractal pattern [49, 50]. In terms of visual display, systems whose spatial properties comprise a fractal tend to form clusters of high density, whereby objects concentrate in close spatial proximity to each other, surrounded by low-density areas (or “voids”), as displayed in Figure 3a (2). Fractal properties describe the spatial diffusion processes of a vast range of natural and social phenomena exhibiting contagious properties, including, for example, the growth of bacterial colonies [52], the evolution of cities [53], or the spread of local initiatives within the urban area [54]. The spatio-temporal diffusion of previous epidemics, such as the SARS-Cov in China and the MERS-Cov in the Middle East, displayed similar properties [55, 56].
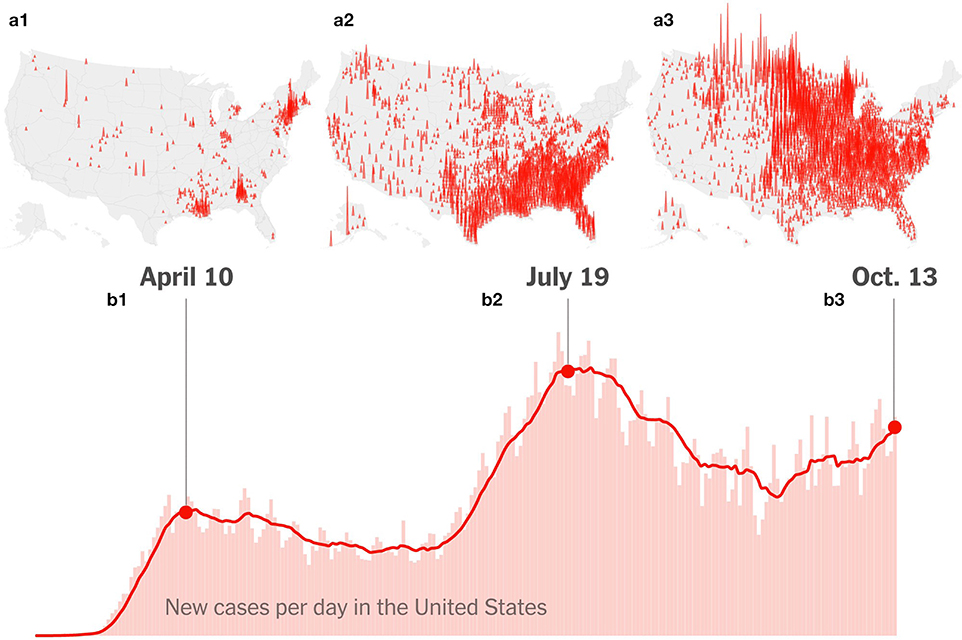
Figure 3. (a) (1)–(3): Spatial Diffusion of Covid-19 cases in the United States, March-October 2020. (b) (1)–(3): Temporal Diffusion of Covid-19 the United States, March-October 2020 (Source: Leatherby, [51] New York Times, full figure available at: https://www.nytimes.com/interactive/2020/10/15/us/coronavirus-cases-us-surge.html).
Given these spatial properties of complex systems, it is not surprising that the spatial diffusion of Covid-19 in various countries is highly nonhomogeneous and tends to exhibit a fractal pattern. This pattern coincides with the spatial distribution of populations, and is characterized by clusters of cases, with local diffusion within the clusters [e.g., [57] (China); [51] (US)]. Some evidence suggests that this pattern also characterizes the global diffusion of the pandemic [58, 59]11.
Figures 3a,b, which describe the diffusion of Covid-19 in the United States, illustrate how this spatial pattern evolves over time.
As is apparent from Figure 3, during the initial period the spatial diffusion pattern is characterized by clusters of cases, which are geographically spread and relatively isolated from each other [Figure 3a(1)]. With the lapse of time and the increase in the numbers of cases the clusters expand from core to periphery and the “voids” among them narrow [Figure 3a(2)]. Eventually, when the pandemic is widespread, it covers most of the geographical area, and the “clustering” pattern is hardly observable [Figure 3a(3)]12.
Acquaintance with these spatiotemporal patterns has significant implications for the legality of differential policies. First, when planning interventions to curb a pandemic, regulators should assume that it will not spread in a homogenous way. Rather, they can rely on decades of complex systems research and expect, from early stages, that the pandemic's spatial diffusion will exhibit a fractal pattern (cf. [54]).
Secondly, the foregoing analysis implies that during particular stages differential geographical treatment can be highly efficient in curbing a pandemic. To illustrate, the Chinese policy that isolated Wuhan and its surrounding region, which suffered from large-scale infections, from other regions in which the number of Covid-19 cases was much lower, was successful in quickly containing the spatial diffusion of the pandemic in China [61]. Likewise, mathematical modeling of the spatial diffusion dynamics indicates that isolating infected regions (“clusters”) while imposing social distancing measures shortly after experiencing community transmission, can lead to an exponential decrease in the number of infected regions, which may remain stable even after social distancing measures are lifted [62].
More specifically, understanding the spatiotemporal properties of the pandemic clarifies that the effectiveness of differential lockdowns, and the relevant scales for their implementation, are dynamic. During the very early stages of diffusion (or following a lockdown which brings down the number of infections), the fractal dimension of the entire pandemic (system) is practically zero [see, e.g., Figure 3a(1)]13. This implies that measures for isolating infected clusters are highly effective, and the effective scale for such isolation can be rather small (e.g., a city, or even a neighborhood within a city). As the fractal dimension increases with the number of infected cases, the scale of isolated units must increase too in order to be effective. During that intermediate stage, the typical fractal dimension of the epidemic's spatial spread would be 1 ≤ D ≤ 2, and the spread would lack a natural scale [see, e.g., Figure 3a(2)]. Accordingly, the appropriate scale for differential measures is indeterminate and somewhat arbitrary. At that point the entire effectiveness of differential measures becomes doubtful, and global measures such as a nation-wide lockdown may be the more efficient choice. Finally, when the pandemic essentially spreads over the entire system and covers the relevant geographical area almost entirely, the fractal dimension roughly approaches the Euclidean (integer) dimension 2, [see, e.g., Figure 3a(3)]14. During that stage differential geographical measures are ineffective and a general lockdown is likely inevitable.
Understanding the spatiotemporal diffusion of the pandemic can, therefore, provide regulators with tools to determine the relevant timeframe for implementing differential measures, and their relevant scale, and can be further used by courts reviewing the legality of such restrictions. To illustrate, a complexity based analysis supports the Israeli Supreme Court's rejection of a claim that a differential lockdown imposed on specific neighborhoods in Jerusalem during the first stages of the pandemic (in April 2020) was discriminatory and illegal. It also provides solid grounds to the Court's' refusal to narrow down the scale of the locked area to specific streets only, since the infected cases have already spread in various streets within that neighborhood (Case # 21).
Network Prioritizing and Privacy
The pandemic poses legal policy makers worldwide with acute challenges of prioritizing and dividing resources: how to allocate tests when testing capacity is limited? How to prioritize the distribution of vaccines among the population? Age, health risk factors, and medical-related occupation are frequently recognized as relevant considerations. Complexity provides policy makers with an additional tool for prioritizing, by using network analysis, which reveals, again, potential conflicts with legal doctrine.
Network analysis has become a prominent tool to describe and analyze complex systems of different kinds. By representing a relevant system as a network and mapping the links among the individuals comprising it (“nodes,” in network parlance), network analysis allows to identify and describe various individual and systemic traits (e.g., [14, 64–66]). Two traits are particularly important for our purposes: “degree centrality,” which describes the number of links that a particular node has (in our case: the number of in-person social ties a person has), and “betweenness centrality,” which describes the extent to which a node—in our case: a person—“bridges” between different groups (“clusters”) in the system [65]15.
Accumulating research of social networks from the past decades demonstrates that in-person networks typically exhibit power law distributions of social connections: a small number of people possess an exceptionally large number of social contacts, compared to the vast majority of the population (e.g., [13, 67])16. Studies further indicate that these “social hubs” play a crucial role in the effective diffusion of various objects through the network, from the flow of information to the spread of pandemics (e.g., [68–70]). To use an intuitive illustration, a person who meets hundreds of people per week is more likely to contract a contagious disease, and to spread it further, relative to someone whose network consists of only five weekly encounters. In the context of contagious diseases, then, high social connectivity can be regarded an additional risk factor.
More specifically, accumulating evidence on Covid-19 indicates that “super-spreading events” whereby “few individuals disproportionately infect a large number of secondary cases” are an extremely significant driver of the pandemic's diffusion (e.g., [71–73]). According to recent data, 10% of infectious individuals cause 80% of the Covid-19 infections [74]. Many super-spreading events have a spatial dimension, and occur in public gatherings, where large numbers of people concentrate in confined, typically indoor, spaces—weddings, places of worship, elderly homes, prisons, and meatpacking plants are a few prominent examples (e.g., [72, 73, 75]). Yet, significant spreading can also occur when an infected social hub contacts numerous people in different places during a short time span, even in the absence of large gatherings (cf. [72]). To illustrate, reports maintain that the first confirmed Covid-19 case of local transmission in the United States, also known as “patient zero,” met with more than 800 people during a few days, prior to being diagnosed in February 2020. These numerous in-person encounters presumably triggered the subsequent spread of Covid-19 in the state of New York [76]. Similarly, studies suggest that musicians traveling among bars were a major driver in the spread of Covid-19 in Hong Kong between January and April 2020 [73].
Network studies from the past two decades further indicate that policies focused on locating, testing, and vaccinating highly connected individuals can significantly contribute to curbing pandemics. Christakis and Fowler [70], investigating the spread of flu among college students, found that tracking the health of more-connected individuals can provide more up-to-date information about the progress of the disease, relative to testing a random sample of people. Moreover, network models found that immunization schemes which prioritize inoculating highly-connected individuals can lower the entire network's vulnerability to a pandemic, and substantially decrease the threshold required for reaching herd immunity [67–69]. Notably, network prioritizing can also be used as part of the contemporary efforts to quickly identify and track new mutations of the virus.
Research on network prioritizing has so far concentrated on people with high “degree centrality,” namely people with a large number of in-person encounters. Our analysis suggests that an additional network metric that is relevant for network prioritizing in the context of Covid-19 is “betweenness centrality.” Due to the spatial diffusion pattern of the disease, which we discussed in the previous Section, individuals with high betweenness centrality can significantly contribute to the transmission of the pandemic among clusters of different geographical locations, and among populations. This, in turn, might jeopardize differential-treatment policies. Overall, then, policies that take into account social connectivity metrics as a factor in prioritizing tests and vaccines can be an efficient tool in the effort to restrain the pandemic (cf. [67]).
From a law and policy perspective, implementing network prioritizing policies raises serious legal challenges, the most prominent of which concerns privacy. Such implementation obviously depends on the availability of connectivity data that enables to identify individuals with the highest degree centrality and betweenness centrality. However, the structure of the in-person social network is not readily observable. While information about highly connected individuals on online social networks (“influencers”) is publicly available, this type of connectivity does not necessarily overlap with high numbers of in-person interactions, which are the relevant type of links in the case of pandemic transmission. Some relevant connectivity data can be inferred by identifying certain occupations, which entail a large number of in-person encounters (e.g., teachers, or salespersons), or transition between geographical areas (e.g., drivers of public transportation) (cf. [77]). Focusing on occupations can therefore provide a helpful prioritizing method, and is indeed adopted by some countries as part of their inoculation program. Nevertheless, it is insufficient for identifying other social hubs, such as New York's “patient zero.”
Existing network studies extracted connectivity data from cellular companies, technology platforms (such as Facebook or Google) that collect mobility data, or epidemiological investigations (cf. [71, 73]). An alternative, indirect, strategy suggested by Cohen et al. [69] and applied by Christakis and Fowler [70] is the “friends” method. This strategy focuses on testing and treating the persons connected to a group of randomly selected individuals. This approach is based on the perception that, due to the structure of social networks, the “friends” group of randomly selected people possess, on average, more social contacts than the randomly selected group, so that focusing on the “friends” group increases the chances of reaching the social hubs [69]. While none of these methods is likely to yield perfect data about network structure, studies indicates that even incomplete data that identifies a sufficient number of hubs can make a significant difference in curbing a pandemic [67].
Nevertheless, the aforesaid methods for collecting and extracting information about individuals' connectivity in order to implement network prioritizing policies may conflict with legal rules protecting individual privacy. A prominent example is the European General Data Protection Regulations (“GDPR”), which limits the processing of “personal data,” including, inter alia, “location data” and information related to a person's “social identity.” The “repurposing” of data originally collected for a specific purpose (for example, targeted advertising), and using it for a different purpose (in our case: identifying social connectivity) is similarly restricted17.
While privacy concerns should not be underestimated, the case of Covid-19 highlights the networked dimensions of privacy, and their theoretical and practical implications for privacy law. Traditional legal theory perceives privacy through a reductionist, individualistic lens—a person's “right to be let alone” [79], a right that “rests upon an individualist concept of society” ([80], p. 958). This reductionist paradigm frames privacy as a private good, implying that its costs and benefits are confined to a particular individual. Therefore, the principal legal regimes that protect privacy empower individual control, and place substantial weight on individual consent ([81], p. 390).
More recent theoretical accounts, however, recognize that framing privacy as a strictly individual interest “ignores the interconnected nature of human behavior and of human interests” [82]. Broadly, this strand of literature highlights that privacy has collective and social attributes: privacy-related decisions of individuals can produce “privacy externalities” and affect other people. It therefore suggests that in certain circumstances privacy protection should be regulated, rather than left to individual choice and consent [81, 83].
The discussion of privacy externalities in the legal literature often concentrates on cases where individuals' decision to renounce their privacy may cause harm to other individuals or to society at large. For example, sharing one's genetic information may harm the privacy of their relatives [84], while the sharing of data with social media platforms may reduce the overall level of privacy in a society [81, 83]. In the foregoing analysis privacy externalities operate in an opposite way. In other words, an individual decision to maintain their privacy (by not disclosing certain information about social connectivity) may impose significant social costs on the effort to curb a pandemic. And due to the structure of the social network and the pandemic diffusion dynamics that we discuss above, the costs entailed in maintaining connectivity information private accumulate in a multiplicative, nonlinear way.
An appropriate theoretical framework for addressing the networked dimensions of privacy in the context of Covid-19 could be found in the literature that understands privacy as a mechanism for encouraging socially beneficial flows of personal information. Most prominently, Nissenbaum's influential theory of privacy as “contextual integrity” [85, 86] perceives privacy not in terms of individual control or consent, but rather in terms of flows of personal information that are normatively appropriate in a particular social context. Contextual integrity theory thus avoids binary and dogmatic distinctions (e.g., between sensitive vs. insensitive information, or between prohibited vs. permitted uses). Rather, it recognizes that transferring certain information (in our case: information about social connectivity) that may not be permitted in one context, may be permitted, and even required, in another context (e.g., to specific health authorities that need to prioritize Covid tests). This theory may therefore provide tools for incorporating the use of network science and its potential benefits in prioritizing Covid-19 interventions, into privacy analysis.
We do not suggest here a detailed regime for the use of connectivity data. However, understanding the significance of network prioritizing in responding to a pandemic, alongside the networked nature of privacy, can assist policy makers and judiciaries in devising such schemes, and in addressing ostensible tensions between privacy and (public and private) health in specific circumstances. More broadly, this analysis reveals that the law is not always sufficiently sensitive to interactions as a relevant factor in the design of legal policies. Complexity theory indicates that it should be.
Conclusions
The Covid-19 pandemic represents a great challenge for law and policy makers. The analysis above demonstrates that acquaintance with the properties of complex systems can provide regulators and judiciaries with an extremely valuable tool for devising and evaluating the response to Covid-19. Concomitantly, it exposes a gap between the principles governing complex systems, and extant legal doctrines, such as proportionality, equality, evidentiary requirements, and privacy, which implicitly presume stable equilibria, and fail to capture the nonlinear multiplicative properties of the pandemic. Embedding insights from complexity theory into legal analysis will thus help align legal policies with complex systems dynamics, and improve the legal response to the pandemic.
Our study is non-exhaustive, and is limited in various respects. Adopting a complexity perspective can likely illuminate additional questions related to the Covid-19 legal response. For example, while we concentrate on court cases and judicial doctrine, legal interventions through administrative regulation may be more flexible and therefore more apt to effectively adapt to the fast-changing realities of the pandemic (cf. [33, 87])18.
More broadly, our present focus is confined to situations in which nonlinearities associated with complex systems can lead to instability and cascading disasters, and does not cover all traits associated with such systems. Furthermore, the details of our analysis are specific to the case of Covid-19, and the proposals we make may not be applicable “as such” to all other cases concerning complex phenomena, and may require adaptation19. Nevertheless, our analysis calls for applying the “lens of complexity” to additional legal policies related to complex nonlinear collective phenomena, from global environmental challenges to financial crises, and for detailed explorations of particular legal responses. More research in this vein will hopefully follow.
Data Availability Statement
The original contributions presented in the study are included in the article/supplementary material, further inquiries can be directed to the corresponding author/s.
Author Contributions
Both authors contributed equally to the conceptualization, analysis, and drafting of this research, and approved it for publication.
Funding
This research was supported by ISF grant number 1322/18.
Conflict of Interest
The authors declare that the research was conducted in the absence of any commercial or financial relationships that could be construed as a potential conflict of interest.
Acknowledgments
We are grateful to the two referees of this paper for their extremely valuable suggestions. For helpful comments and discussions we thank Yaneer Bar-Yam, Doron Teichman, Eyal Zamir, and the participants of the Physics of Law conference (November 2020, online), and the Cyber Center Workshop at the Hebrew University of Jerusalem (January 2021, online). Stav Cohen and Roi Ohayon provided excellent research assistance.
Footnotes
1. ^We focus primarily on court cases and judge-made doctrines, rather than on administrative regulations that naturally vary significantly among jurisdictions.
2. ^Similar growth patterns were documented in many other countries—see, for example, the growth of Covid-19 cases in the United States during March, 2020, World Health Organization, https://www.who.int/countries/usa/.
3. ^Importantly, the aforesaid border closure preceded the emergence of new variants of the virus. The appearance of variants can set back the “diffusion clock” (with respect to the new variant) and in certain cases might create new justifications for closure.
4. ^In that sense, our argument for an “inverse proportionality test” can be conceived as a non-ideal theory, aiming at guiding actions in the real world.
5. ^It should be clarified that while this comparison is illustrative and suggestive, it cannot provide a definitive explanation of the differences between the countries, since we do not consider additional factors which may have affected the diffusion dynamics.
6. ^There are, of course, nuances among jurisdictions which we do not fully explore here. Some jurisdictions regard the “least restrictive means” test as a second step in the legal analysis, which comes into play only after it has been determined that an effective response is required. In such cases the state should still choose, among the range of effective means, those that impose the least restrictions on individual liberties, even under an “inverse proportionality test.” However, in practice, the application of the two steps is often entangled. Moreover, as we discuss in the following Section, due to complex systems' dynamics there are significant uncertainties as to the precise effectiveness of particular measures. It is therefore important to recognize that very restrictive means may be necessary in order not to render the entire response ineffective.
7. ^The cumulative distribution of a random variable X is “fat-tailed” if its tail decays slowly; e.g., like power law: . For such distributions, the moment of order k exists [i.e., E(X) is finite] if and only if α > k. Thus, if α ≤ 1, the mean does not exist. For 1 < α ≤ 2 the variance is infinite. In this case the mean exists but the sample mean will converge very slowly with the true mean, and the standard statistical errors will understate the true uncertainty of the phenomenon [31, 32].
8. ^See John Hopkins Corona Virus Resources Center. Available online at: https://coronavirus.jhu.edu/data.
9. ^As opposed to additive stochastic processes, which exhibit Gaussian distributions, multiplicative stochastic processes typically exhibit “fat tailed” power-law distributions of the form P(x) ~ x−1−∝ (see, e.g., [38]), As noted above, if α ≤ 1 the mean of the distribution goes to infinity. In the context of an epidemic, such fat-tailed power law distribution implies that the expected harm is immense.
10. ^These factors are similar to the guidelines we propose in the preceding Section, for applying the inverse proportionality test.
11. ^For an attempt to integrate these properties into a modified SIR model, in order to predict the diffusion of Covid-19, see, e.g., [60].
12. ^Notably, while Figure 3a is suggestive of the spatially inhomogeneous spread of a complex system, it is largely based on visual impression. Validating the fractal properties of the spatial diffusion pattern in the United States would require more ingrained spatio-temporal diffusion data, which we do not possess (cf. [54]).
13. ^A fractal dimension is a non-integer number that expresses the dimension of objects exhibiting similar structures over a range of length scales. There are various methods to evaluate the fractal dimension of an empirical fractal, all based on multiple resolution analysis in which one measures a property P of the system (mass, volume, etc.) as a function of the yardstick used in measuring it (given by a yardstick of linear size r). Fractal objects are characterized by the power-law formula P = kr−D, where D is the fractal dimension and k is a prefactor related to the lacunarity of the object (see, e.g., [63]).
14. ^Notably, if the epidemic spreads freely, its eventual spatial diffusion will likely coincide (approximately) with the spatial distribution of the population, which is typically nonhomogeneous and exhibits fractal properties.
15. ^A person's betweenness centrality is calculated by the number of shortest paths connecting all individuals in a network that pass through that person – (e.g.,[65], p. 334).
16. ^We should clarify that, while multiplicative processes often generate power law distributions, our analysis is applicable to “fat tailed” distributions more generally, and does not depend on whether the empirically observed distribution is “truly” power law or other “fat-tailed” distribution that exhibits large skewness.
17. ^Regulation (EU) [78], Art. 4(1), and Art. 5(1)(b), available at https://gdpr-info.eu/. Notably, alongside these restrictions, privacy legislation typically recognizes that public health concerns may justify certain exceptions to privacy protection – e.g., Art. 9(2)(i).
18. ^Obviously, such regulations, too, will eventually be subject to judicial scrutiny.
19. ^To illustrate, not all complex phenomena diffuse at a pace similar to a pandemic (cf. [54]), a factor which may be significant for assessing proportionality.
References
1. Bavel JJV, Baicker K, Boggio PS, Capraro V, Cichocka A, Cikara M, et al. Using social and behavioural science to support COVID-19 pandemic response. Nat Hum Behav. (2020) 4:460–71. doi: 10.1038/s41562-020-0884-z
2. Teichman D, Underhill K. Infected by bias: behavioral science and the legal response to COVID-19. Am J Law Med. Hebrew University of Jerusalem Legal Research. (2020). doi: 10.2139/ssrn.3691822
3. Zamir E, Teichman D. Governmental Decision-Making Regarding the Covid-19 Pandemic—a Behavioral Perspective. SSRN (2020). Available online at: https://ssrn.com/abstract=3698682 (accessed January 3, 2021).
4. Ruhl JB. Complexity theory as a paradigm for the dynamical law-and-society system: a wake-up call for legal reductionism and the modern administrative state. Duke Law J. (1996) 45:849–928. doi: 10.2307/1372975
5. Shur-Ofry M. IP and the lens of complexity. IDEA. (2013) 54:55–102. Available online at: http://papers.ssrn.com/sol3/papers.cfm?abstract_id=2268867
6. Ruhl JB, Katz D. Measuring, monitoring, and managing legal complexity. Iowa Law Rev. (2015) 101:191–244. Available online at: https://scholarship.kentlaw.iit.edu/fac_schol/865
7. Fallon RH. Strict judicial scrutiny. UCLA Law Rev. (2007) 54:1267–338. Available online at: https://www.uclalawreview.org/strict-judicial-scrutiny/
8. Jackson VC. Constitutional law in an age of proportionality. Yale Law J. (2015) 124:3094–196. Available online at: https://www.yalelawjournal.org/article/constitutional-law-in-an-age-of-proportionality
9. Note (1969). Less drastic means and the first amendment. Yale Law J. 78:464–74. doi: 10.2307/794878
11. Brems E, Lavrysen L. ‘Don't use a sledgehammer to crack a nut’: less restrictive means in the case law of the European court of human rights. Hum Rts Law Rev. (2015) 15:139–68. doi: 10.1093/hrlr/ngu040
12. Bass F. A new product growth for model consumer durables. Manag Sci. (1969) 15:215–27. doi: 10.1287/mnsc.15.5.215
14. Cohen R, Havlin S. Complex networks: structure, robustness and function. New York, NY: Cambridge University Press (2010). 238 p.
15. Stevens R, Perera R, Heneghan C, Hobbs R, Oke J. Exponential Growth: What It Is, Why It Matters, and How to Spot It. CEBM (2020). Available online at: https://www.cebm.net/wp-content/uploads/2020/09/exponential-growth (accessed January 3, 2021).
16. Stango V, Zinman J. Exponential growth bias and household finance. J. Finance. (2009) 64:2807–49. doi: 10.1111/j.1540-6261.2009.01518.x
17. Teichman D, Zamir E. Mathematics, Psychology, and Law: The Legal Ramifications of the Exponential Growth Bias. (2020). Available online at: https://papers.ssrn.com/sol3/papers.cfm?abstract_id=3804329
18. Covid-19: China's Qingdao to Test Nine Million in Five Days. BBC News (2020). Available online at: https://www.bbc.com/news/world-asia-54504785 (accessed January 3, 2021).
19. Jamieson T. “Go hard, go early”: Preliminary lessons from New Zealand's response to COVID-19. Am Rev Public Adm. (2020) 50:598–605. doi: 10.1177/0275074020941721
20. Israel Plans to Halt All International Flights as Part of Nationwide Shutdown News18. (2020). Available online at: https://www.news18.com/news/auto/israel-plans-to-halt-all-international-flights-as-part-of-nationwide-shutdown-2875653.html. (accessed January 3, 2021).
21. Sanderson I. Evaluation, policy learning and evidence-based policy making. Public Admin. (2002) 80:1–22. doi: 10.1111/1467-9299.00292
22. Gunn TJ. Deconstructing proportionality in limitations analysis. Emory Int. L. Rev. (2005) 19:465–98.
23. Ioannidis JPA. A Fiasco in the Making? As the Coronavirus Pandemic Takes Hold, We Are Making Decisions Without Reliable Data. Statistics (2020). Available online at: https://www.statnews.com/2020/03/17/a-fiasco-in-the-making-as-the-coronavirus-pandemic-takes-hold-we-are-making-decisions-without-reliable-data/ (accessed January 3, 2021).
24. Shaw R. Modeling chaotic systems. In: Haken H, editor. Chaos and Order In Nature. Berlin, Heidelberg: Springer. (1981). p. 218–31.
25. Lorenz EN. Deterministic nonperiodic flow. J Atmos Sci. (1963) 20:130–141. doi: 10.1175/1520-0469(1963)020<0130:DNF>2.0.CO;2
26. Prigogine I. Exploring complexity. Eur J Oper Res. (1987) 30:97–103. doi: 10.1016/0377-2217(87)90085-3
27. Prigogine I, Allen P. The challenge of complexity. In: Schieve WC, Allen PM, editors. Self-Organization and Dissipative Structures. Texas: University of Texas Press (1982). p. 3–39.
28. Siegenfeld AF, Taleb NN, Bar-Yam Y. Opinion: what models can and cannot tell us about COVID-19. PNAS. (2020) 117:16092–5. doi: 10.1073/pnas.2011542117
29. Castro M, Ares S, Cuesta JA, Manrubia S. Predictability: can the turning point and end of an expanding epidemic be precisely forecast? arXiv. (2020). doi: 10.1073/pnas.2007868117
30. Taleb NN, Bar-Yam Y, Cirillo P. On single point forecasts for fat-tailed variables. arXiv preprint arXiv:2004.08842. (2020). doi: 10.1016/j.ijforecast.2020.08.008
31. Cirillo P, Taleb NN. Tail risk of contagious diseases. Nat Phys. (2020) 16:606–13. doi: 10.1038/s41567-020-0921-x
32. Fukui M, Furukawa C. Power laws in superspreading events: evidence from Coronavirus outbreaks and implications for SIR models. medRxiv. (2020). doi: 10.1101/2020.06.11.20128058
33. Ruhl JB. General design principles for resilience and adaptive capacity in legal systems-with applications to climate change adaptation. North Carolina Law Rev. (2011) 89:1373–401. Available online at: https://scholarship.law.unc.edu/cgi/viewcontent.cgi?article=4506&context=nclr
34. Read R, O'Riordan T. The precautionary principle under fire. Environ Sci Pol Sustain Dev. (2017) 59:4–15. doi: 10.1080/00139157.2017.1350005
35. Helsingen LM, Løberg M, Refsum E, Gjøstein DK, Wieszczy P, Olsvik O, et al. Randomized re-opening of training facilities during the COVID-19 pandemic. medRxiv. (2020). doi: 10.1101/2020.06.24.20138768
36. Teichman D, Zamir E. How do you Cause Everyone to Start Wearing Facemasks: Nudges, Mandates, and the Legal Response to the Covid-19 Pandemic in Israel. SSRN (2020). Available online at: https://ssrn.com/abstract=3698731 (accessed January 3, 2021).
37. Allen R. Taming complexity: rationality, the law of evidence and the nature of the legal system. Law Probability Risk. (2013) 12:99–113. doi: 10.1093/lpr/mgs008
38. Malcai O, Biham O, Solomon S. Power-law distributions and Levy-stable intermittent fluctuations in stochastic systems of many autocatalytic elements. Phys Rev E. (1999) 60:1299. doi: 10.1103/PhysRevE.60.1299
39. The Wingspread Statement on the Precautionary Principle. (1998). Available online at https://www.gdrc.org/u-gov/precaution-3.html (accessed January 3, 2021).
40. Report of the United Nations Conference on Environment and Development. (“ Rio Declaration”), Principle 15 Rio de Janeiro (1992). Available online at: https://www.un.org/en/development/desa/population/migration/generalassembly/docs/globalcompact/A_CONF.151_26_Vol.I_Declaration.pdf (accessed January 3, 2021).
41. Nash JR. Standing and the precautionary principle. Colum Law Rev. (2006) 108:494–528. Available online at: https://chicagounbound.uchicago.edu/public_law_and_legal_theory/280/
42. Sunstein CR. Beyond the precautionary principle. U Pa Law Rev. (2003) 151:1003–58. doi: 10.2307/3312884
44. Ruhl JB. Working both (positivist) ends toward a new (pragmatist) middle in environmental law. Geo Wash Law Rev. (2000) 68:522. doi: 10.2139/ssrn.214528
45. Gleick PH. Climate change, exponential curves, water resources, and unprecedented threats to humanity. Clim Change. (2010) 100:125–9. doi: 10.1007/s10584-010-9831-8
46. Trisos CH, Merow C, Pigot AL. The projected timing of abrupt ecological disruption from climate change. Nature. (2020) 580:496–501. doi: 10.1038/s41586-020-2189-9
47. Delan D, Shannon G, Bhopal SS, Abubakar I. Racism and discrimination in COVID-19 responses. Lancet. (2020) 395:1194. doi: 10.1016/S0140-6736(20)30792-3
48. Kermack WO, McKendrick AG. A contribution to the mathematical theory of epidemics. Proc R Soc London. (1927) 115:700–21. doi: 10.1098/rspa.1927.0118
50. Bak P, Tang C, Wiesenfeld K. Self-organized criticality. Phys Rev A. (1988) 38:364–74. doi: 10.1103/PhysRevA.38.364
51. Leatherby L. U.S. Virus Cases Climb Toward a Third Peak. NY Times (2020). Available online at: https://www.nytimes.com/interactive/2020/10/15/us/coronavirus-cases-us-surge.html (accessed January 3, 2021).
52. Ben-Jacob E, Cohen I, Shochet O, Aranson I, Levine H, Tsimring L. Complex bacterial patterns. Nature. (1995) 373:566–7. doi: 10.1038/373566a0
54. Shur-Ofry M, Malcai O. Institutions for collective action and social contagion: community gardens as a case study. Regul Govern. (2021) 15:63–81. doi: 10.1111/rego.12256
55. Fang LQ, De Vlas SJ, Feng D, Liang S, Xu YF, Zhou JP, et al. Geographical spread of SARS in mainland China. Trop Med Int Health. (2009) 14:14–20. doi: 10.1111/j.1365-3156.2008.02189.x
56. Al-Ahmadi K, Alahmadi S, Al-Zahrani A. Spatiotemporal clustering of Middle East Respiratory Syndrome Coronavirus. (MERS-CoV) incidence in Saudi Arabia, 2012–2019. Int J Environ Res Public Health. (2019) 16:2520. doi: 10.3390/ijerph16142520
57. Kang D, Choi H, Kim JH, Choi J. Spatial epidemic dynamics of the COVID-19 outbreak in China. Int J Infect Dis. (2020) 94:96–102. doi: 10.1016/j.ijid.2020.03.076
58. Jiang B, de Rijke C. A Power-Law-Based Approach to Mapping COVID-19 Cases in the United States. (2020). Available online at: https://www.preprints.org/manuscript/202007.0607/v1 (accessed January 3, 2021).
59. Yeşilkanat C. M. Spatio-temporal estimation of the daily cases of COVID-19 in worldwide using random forest machine learning algorithm. Chaos Solitons Fractals. (2020) 140:110210. doi: 10.1016/j.chaos.2020.110210
60. Huang J, Zhang L, Liu X, Yun W, Liu C, Lian X, et al. Global prediction system for COVID-19 pandemic. Sci Bull. (2020) 65:1884–7. doi: 10.1016/j.scib.2020.08.002
61. Long Y, Chen Y, Li Y. Multifractal scaling analyses of the spatial diffusion pattern of COVID-19 pandemic in Chinese mainland. arXiv:2010.02747 (2020).
62. Siegenfeld AF, Bar-Yam Y. Eliminating COVID-19: the impact of travel and timing. arXiv:2003.10086 (2020).
63. Malcai O, Lidar DA, Biham O, Avnir D. Scaling range and cutoffs in empirical fractals. Phys Rev E. (1997) 56:2817–28. doi: 10.1103/PhysRevE.56.2817
64. Strandburg KJ, Csárdi G, Tobochnik J, Érdi P, Zalányi L. Law and the science of networks: an overview and an application to the “patent explosion”. Berkeley Tech Law J. (2006) 21:1293. doi: 10.2139/ssrn.926354
66. Borgatti SP, Everett MG, Johnson JC. Analyzing Social Networks. 2nd ed. Los Angeles: SAGE Publications (2018). 363 p.
67. Dezso Z, Barabasi AL. Halting viruses in scale-free networks. Phys Rev E. (2002) 65:055103. doi: 10.1103/PhysRevE.65.055103
68. Pastor-Satorras R, Vespignani A. Immunization of complex networks. Phys Rev E. (2002) 65:036104. doi: 10.1103/PhysRevE.65.036104
69. Cohen R, Havlin S, Ben-Avraham D. Efficient immunization strategies for computer networks and populations. Phys Rev Lett. (2003) 91:247901. doi: 10.1103/PhysRevLett.91.247901
70. Christakis NA, Fowler JH. Social network sensors for early detection of contagious outbreaks. PLoS ONE. (2010) 5:e12948. doi: 10.1371/journal.pone.0012948
71. Lau MS, Grenfell B, Thomas M, Bryand M, Nelsone K, Lopmane B. Characterizing superspreading events and age-specific infectiousness of SARS-CoV-2 transmission in Georgia, USA. PNAS. (2020) 117:22430–5. doi: 10.1073/pnas.2011802117
72. Kuperschmidt K. Why do some COVID-19 patients infect many others, whereas most don't spread the virus at all? Science. (2020). doi: 10.1126/science.abc8931
73. Dillon CA, Wu P, Wong JY, Lau EHY, Tsang TK, Cauchemez S, et al. Clustering and superspreading potential of SARS-CoV-2 infections in Hong Kong. Nat Med. (2020) 26:1714–9. doi: 10.1038/s41591-020-1092-0
74. Endo A, Kucharski AJ, Funk S. Estimating the overdispersion in COVID-19 transmission using outbreak sizes outside China. Wellcome Open Res. (2020) 5:67. doi: 10.12688/wellcomeopenres.15842.3
75. Leclerc QJ, Fuller NM, Knight LE, Funk S, Knight GM. What settings have been linked to SARS-CoV-2 transmission clusters? Wellcome Open Res. (2020) 5:83. doi: 10.12688/wellcomeopenres.15889.1
76. Cox C. The vulnerable can wait. Vaccinate the Super-Spreaders First, WIRED. (2020). Available online at: https://www.wired.com/story/covid-19-vaccine-super-spreaders/
77. Shur-Ofry M, Malcai O. Using Network-Science Tools for Combatting Covid-19: Prioritizing Tests and Vaccines. Memorandum to the Israeli Health Ministry (2020).
78. Regulation (EU) 2016/679 (General Data Protection Regulation) OJL 119, 04.05.2016; cor. OJL 127, 23.5.2018).
79. Warren SD, Brandeis LD. The right to privacy. Harvard Law Rev. (1890) 4:193–220. doi: 10.2307/1321160
80. Post RC. The social foundations of privacy, community and self in the common law tort. Calif Law Rev. (1989) 77:957–1010. doi: 10.2307/3480641
81. Fairfield JA, Engel C. Privacy as a public good. Duke Law J. (2015) 65:385–457. Available online at: https://scholarship.law.duke.edu/dlj/vol65/iss3/1
82. Bamberger KA, Evan Mayse A. Privacy in Society: Jewish Law Insights for the Age of Big Data. SSRN (2020). Available online at: https://papers.ssrn.com/sol3/papers.cfm?abstract_id=3731770 (accessed January 3, 2021).
83. MacCarthy M. New directions in privacy: disclosure, unfairness and externalities. ISJLP. (2011) 6:425–512. Available online at: https://core.ac.uk/download/pdf/159597718.pdf
84. Ram N. DNA by the entirety Colum Law Rev. (2015) 115:873–940. Available online at: https://columbialawreview.org/wp-content/uploads/2016/02/May-2015-14-Article-Ram_UPDATE-5.17.pdf
85. Nissenbaum H. Privacy as contextual integrity. Washington Law Rev. (2004) 79:119–58. Available online at: https://digitalcommons.law.uw.edu/wlr/vol79/iss1/10
86. Barth A, Datta A, Mitchell JC, Nissenbaum H. Privacy and contextual integrity: framework and applications. In: 2006 IEEE Symposium on Security and Privacy (S&P'06). Berkeley/Oakland, CA (2006). 198 p.
87. Arnold CA, Gunderson LH. Adaptive law and resilience. Env Law Rep. (2013) 43:10426. Available online at: https://www.researchgate.net/profile/Tony-Arnold-2/publication/257109831_Adaptive_Law_and_Resilience/links/53fe22680cf21edafd14f3f5/Adaptive-Law-and-Resilience.pdf
Appendix
Court Cases
1. United States v. Raia, No. 20-1033, 2020 WL 1647922 (3d Cir. Apr. 2, 2020) (US)
2. United States v. Kennedy, No. 18-20315, 2020 WL 1547878 (E.D. Mich. Apr. 1, 2020) (US)
3. United States v. Rivers, No. CR 2017-0023, 2020 WL 1676798 (D.V.I. Apr. 6, 2020) (US)
4. Legacy Church, Inc. v. Kunkel, No. CV 20-0327 JB\SCY, 2020 WL 3963764 (D.N.M. July 13, 2020)(US)
5. S. Bay United Pentecostal Church v. Newsom, 140 S. Ct. 1613, 207 L. Ed. 2d 154 (2020) (US)
6. S. Bay United Pentecostal Church v. Newsom, 592 U. S. <uscore> (2021)
7. Roman Catholic Diocese v. Cuomo, 208 L. Ed. 2d 206 (S. Ct., Nov. 25, 2020)(US)
8. Roman Catholic Diocese v. Cuomo, 2020 U.S. Dist. LEXIS 192292 (E.D.N.Y, Oct. 16, 2020)(US)
9. Calvary Chapel Dayton Valley v. Sisolak, 140 S. Ct. 2603 (July 24, 2020)(US)
10. Calvary Chapel Dayton Valley v. Sisolak, 2020 U.S. Dist. LEXIS 103234 (D. Nev., June 11, 2020)(US)
11. Rock House Fitness, Inc. v. Acton, No. 20CV000631, 2020 WL 3105522 (Ohio Com.Pl. May 20, 2020) (US)
12. LMV DEV SPE, LLC, DBA Kalahari Resorts & Conventions v. Amy Acton, No. 2020-CV-0201 (Erie Cty. Com.Pl. July 12, 2020) (US)
13. Sprague v. Her Majesty the Queen in right of Ontario, 2020 ONSC 2335, 317 A.C.W.S (3d) 352 (Can. Ont.)
14. HMQ v. A.C., 2020 ONSC 2870 (Can. Ont.)
15. Children's Aid Society of the Region of Halton v. R.O., 2020 ONCJ 209, 317 A.C.W.S (3d) 271 (Can. Ont.)
16. Reyno Dawid De Beer v. The Minister of Cooperative Governance and Traditional Affairs, No. 21542/2020, ZAGPPHC 184 (June 2, 2020) (S. Afr.)
17. Conseil d'Etat [CE] [Council of State] May 18, 2020, M.W et auters, No. 440366 (France)
18. VG Regensburg, Apr. 27, 2020, RO 14 E 20.687, https://www.vgh.bayern.de/media/vgregensburg/presse/20a00687b.pdf (Germany)
19. S.T.S.J. Madrid, Oct. 8, 2020 (R.O.J. No. 308/2020)(Spain)
20. HCJ 2435/20 Yedidya Loewenthal, Adv. v. Prime Minister Benjamin Nethanyahu (Apr. 7, 2020), available at Nevo Legal Database (by subscription, in Hebrew) (Isr.)
21. HCJ 2491/20 Ramot Alon Community Administration v. The Government of Israel, Prime Minister (Apr. 14, 2020), available at Nevo Legal Database (by subscription, in Hebrew) (Isr.)
Keywords: law, complexity, COVID-19, exponential diffusion, fractal, proportionality, evidence, networks and privacy
Citation: Malcai O and Shur-Ofry M (2021) Using Complexity to Calibrate Legal Response to Covid-19. Front. Phys. 9:650943. doi: 10.3389/fphy.2021.650943
Received: 08 January 2021; Accepted: 15 March 2021;
Published: 26 April 2021.
Edited by:
Daniel Martin Katz, Illinois Institute of Technology, United StatesReviewed by:
Katherine Strandburg, New York University, United StatesDaniel Rodriguez, Northwestern University, United States
Copyright © 2021 Malcai and Shur-Ofry. This is an open-access article distributed under the terms of the Creative Commons Attribution License (CC BY). The use, distribution or reproduction in other forums is permitted, provided the original author(s) and the copyright owner(s) are credited and that the original publication in this journal is cited, in accordance with accepted academic practice. No use, distribution or reproduction is permitted which does not comply with these terms.
*Correspondence: Michal Shur-Ofry, bWljaGFsc2h1ckBtYWlsLmh1amkuYWMuaWw=; orcid.org/0000-0002-3623-7769