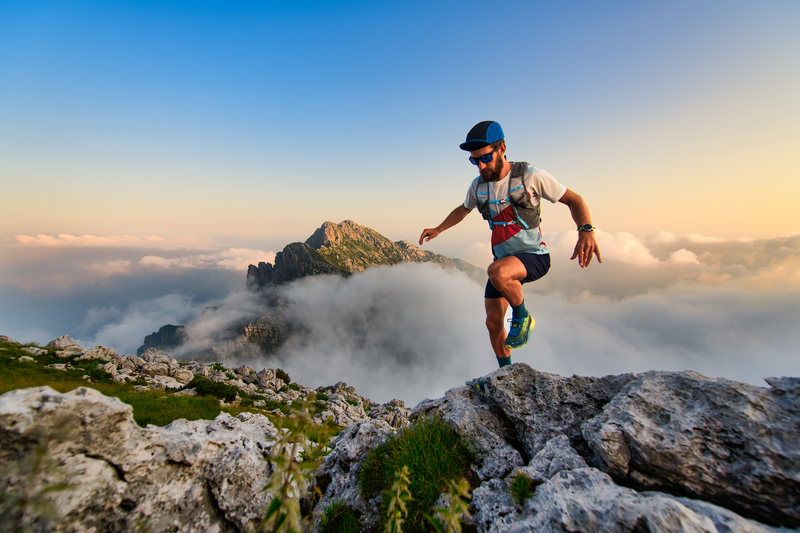
94% of researchers rate our articles as excellent or good
Learn more about the work of our research integrity team to safeguard the quality of each article we publish.
Find out more
ORIGINAL RESEARCH article
Front. Phys. , 22 April 2021
Sec. Soft Matter Physics
Volume 9 - 2021 | https://doi.org/10.3389/fphy.2021.635269
This article is part of the Research Topic 50 years of Statistical Physics in Mexico: Development, State of the Art and Perspectives View all 17 articles
The dynamics of colloidal particles at infinite dilution, under the influence of periodic external potentials, is studied here via experiments and numerical simulations for two representative potentials. From the experimental side, we analyzed the motion of a colloidal tracer in a one-dimensional array of fringes produced by the interference of two coherent laser beams, providing in this way an harmonic potential. The numerical analysis has been performed via Brownian dynamics (BD) simulations. The BD simulations correctly reproduced the experimental position- and time-dependent density of probability of the colloidal tracer in the short-times regime. The long-time diffusion coefficient has been obtained from the corresponding numerical mean square displacement (MSD). Similarly, a simulation of a random walker in a one dimensional array of adjacent cages with a probability of escaping from one cage to the next cage is one of the most simple models of a periodic potential, displaying two diffusive regimes separated by a dynamical caging period. The main result of this study is the observation that, in both potentials, it is seen that the critical time
A colloidal particle undergoing Brownian motion presents deviations from pure diffusion when such a particle interacts with an external potential or when it moves in a crowded environment. Some examples of crowded environments that affects colloidal motion include the colloidal motion of proteins or organelles in the interior of a cell [1–3], the motion of a tracer particle in complex fluids [4–9] and colloidal motion near the glass transition [10, 11]. On the other hand, examples of external fields that affect Brownian motion are electric and magnetic fields [12], gravitational forces [13–15] and optical manipulation induced by light [16, 17]. The diffusion of tracers in periodic, quasiperiodic, and random external potentials has been also studied in underdamped and overdamped conditions theoretically, experimentally and via numerical simulations [18–21].
On the other hand, the main effect of external potentials, or crowded environments, in colloidal dynamics is to promote the appearance of time regimes in which particles might slow down (subdiffusion) or speed up (superdiffusion) their motion as compared with normal diffusion. Generally speaking, a colloidal particle senses its environment when it moves in Brownian motion. As a result, each time regime is related to a particular length scale. The dynamics of a colloidal tracer provides useful information about the concentration of other colloidal macromolecules, the degree of coupling between the tracer and an applied external field, as well as the competition between the energy associated to the external potential and the thermal energy [4, 10, 17, 22].
One of the most simple cases where the appearance of different time regimes has been reported, due to the interaction of a particle with an inhomogeneous external field, is a one dimensional periodic potential interacting with a 2D colloidal suspension [23–25]. Such a system has many advantages: it can be simulated via BD simulations and can be experimentally realized by using the interference of two coherent beams, providing energy barriers of height close to the thermal energy, for laser powers smaller than 1 W [26]. A colloidal particle interacting with a periodic potential presents three time regimes related with different effects: free diffusion with a linear mean square displacement can be observed at short times; a plateau in the mean square displacement associated to “caging,” produced by the existence of an energy barrier, can be seen at intermediate times; and a hopping motion of the colloidal tracer between adjacent periodic fringes in a second diffusive regime can be observed at long times [23, 24]. Similarly, a random walker simulation have been used extensively to simulate Brownian motion [27–29]. In this type of simulations, the trajectories are obtained from random numbers just as the BD simulations, but the displacement is chosen more simply, not derived from a force. Thus, the confinement is introduced as a spatial condition of no escaping from a region. The height barrier effect is then introduced as a cage-to-cage hopping probability. This allow us to observe a process similar to that displayed by a Brownian particle in a periodic potential, showing a short- and a long-time diffusive regime as a function of time. Note, however, that in the random walker simulation the particle is free to move within the cage in contrast to the cosine-like potential used in the Brownian dynamics approach.
It is important to note that the three time regimes presented above mainly depend on the periodicity of the external potential and the amplitude of the energy barrier, or the hopping probability, and they can extend over several time decades [23, 24, 30]. For example, the short time regime is related with free diffusion and thus is limited to the time the particle takes to diffuse at the bottom part of the potential; the extension of the plateau region is directly related with the amplitude of the potential, and finally the long time diffusion results of a balance between hopping time, and thus related with the amplitude of the potential, and the periodicity of the potential. Furthermore, this potential had been also studied for mean first passage time calculations, giving theoretical predictions for the ratio between the diffusion coefficients associated with its dynamics [31]. In such a scenario, colloidal dynamics can be described by the ratio between the short- and long-time diffusion coefficients [23]. Other quantities of interest are the time at which the caged motion begins, and the time at which the hopping motion starts. As indicated before, the phenomenology found in this potential can be mapped to several other situations. Thus, for complex fluids, the long-time diffusion coefficient is related to the zero shear viscosity [22], whereas the glass transition is related to the so-called alpha relaxation [32]. Despite numerous theoretical, simulation, and experimental studies that had been performed since last century, a complete characterization of the prediction of the short- and long-times diffusion coefficient, using available theoretical models, as well as the study of the critical caging time and the distribution of hoping times, is still lacking.
In this work, the dynamics of a colloidal particle in periodic potentials is studied by using experiments and simulations, focusing in the short- and long-times dynamics. We are particularly interested in the behavior of the critical time at which the MSD changes its curvature when the caging effect appears, and in the distribution of hoping times between adjacent periodic cells. We also compare our results with available theoretical predictions. Our results give a full characterization of the phenomena in terms of energy barriers and the associated dynamical caging, providing a simple model to understand the colloidal dynamics under different, but equivalent scenarios.
The experimental system consists of a highly dilute water suspension of 1 µm polystyrene spherical particles (Thermo Scientific) confined between one microscope slide and a cover slip. The sample has been prepared by following the procedure described by Carbajal-Tinoco et al. [33].
The experimental set up for the light potential is based on the interference of two beams in the plane of the sample, which produces a periodical array of bright fringes of a specific width. This experimental setup has been fully described elsewhere [34], here we only discuss the main points. The laser beam used has a spectral width <200 kHz (Azur Light Systems) and range powers between 40 and 500 mW. A half-wave plate and a fixed polarizer allow us to vary the input power by rotating the half-wave plate. The laser beam passes through a beam splitter, which divides the beam in two with the same laser power. Both beams are directed into a prism mirror that reflects the beams parallel each other. The prism is mounted on a base that can be moved manually to change the distance between the beams. A spherical lens initializes the convergence of both beams in order to produce the interference pattern in the focal plane of the lens. The distance of separation between the beams determines the angle ψ of incidence, which defines the periodicity of the intensity pattern. However, it also changes the effective focal distance of the lens due to spherical aberration. Thus, a focusing lens is mounted on a moving stage to correct such effect. This interferometer is coupled using a dichroic mirror to an inverted microscope (OLYMPUS U-LH100-3) with a 60X objective and numerical aperture 0.6, that allows us to obtain high quality clear field images in order to get the time evolution of the system.
The interference of the coherent beams produce an intensity pattern expressed as [35].
where
The time evolution of the system has been recorded by using standard video equipment at 30 frames per second, giving an experimental time resolution of 0.033 s. The tracking of the particles has been performed by using Trackpy [38], which implements and extends the Crocker-Grier algorithm in Python language [39]. Furthermore, by placing neutral density filters on the lens of the recording camera, the intensity profile generated by the interference of the beams can be imaged, and thus the spatial position of the minima has been estimated. This is very helpful for the calculation of the spatial-dependent statistical properties. It is important to note that we analyze the trajectories of particles at the center of the field of view since the spatial distribution of light is not homogeneous at the edges due to the Gaussian envelope. This ensures that the variation of the intensity in the region of interest is less than 10%, where the particles interact with a similar external potential. The area in the region of interest corresponds to 1908.5 µm2, and a total of 2 h of recording, divided in three videos, were analyzed, giving almost 2,000 trajectories. However, most of them are very short, corresponding to particles that remained within the region of interest only for a few frames. Such trajectories contributed to the short time dynamics, and thus giving a reliable quantification of the mean squared displacement at short times as well as of the density of probability of displacements. However, the long time dynamics lacks of statistics as only a few particles remain during the total duration of the video. The duration of each experiment was defined due to the presence of a small drift in the laser beam, producing a motion of the fringes in one direction. We found that during intervals of 20 min there is a very small variation of the position of the fringes, giving confidence in the position of the fringes. Fringes were also found to vibrate due to external mechanical noise, even tough the experiment was performed on an isolated optical table. Once we get the trajectories of the particles we are able to calculate the total average displacement and the mean square displacement of all trajectories of particles in a time interval. A schematic representation of the protocol we have described is shown in Figure 1.
FIGURE 1. (Color online): Experimental measurement protocol of the dynamic properties of spherical tracers in periodic cosine-like potentials: (A) long exposure photograph showing the light path producing the periodic distribution of light; (B) schematic representation of the experimental set-up; (C) light intensity profile generated experimentally; (D) typical bright field images of spherical tracers interacting with the external field; (E) trajectories obtained from the experiment using Trackpy; (F,G) the maxima and minima of the periodic potentials are estimated from the bright and dark regions displayed in the panel (C); and (H) the density of probability is calculated from the information obtained from the trajectories of the particles over the fringes.
In this work, we consider that a tracer particle is under the influence of a periodic cosine potential of the form:
where
The Brownian dynamics simulations were performed using the method proposed by Ermak and McCammon without hydrodynamic interactions [40]. As the experimental particle concentration is very low, we consider a single particle in a one-dimensional simulation box with periodic boundary conditions along the
where
where
On the other hand, Bellour et. al. [9]. have proposed the following functional form to fit the MSD of tracer particles in worm-like micelle solutions:
This equation correctly describes the above mentioned dynamical regimes: short time diffusion within the network of micelles, cage effect at intermediate times, and hopping motion due to breaking of the living polymer giving a second linear regime at long times and thus can be used as a model to estimate some parameters characterizing the MSD of particles in periodical potentials.
where
If the exponential is linearized, it is easy to see that at short times
Even though this last equation is able to reproduce the MSD of a tracer immersed in a semidilute solution of worm-like micelles at short- and long-times, in general fails to describe the onset of the plateau around the inflexion point. Thus, Bellour proposed to add a parameter α in order to adjust the onset of the experimental plateau of the MSD. As one can appreciate here, the same basic arguments employed by Bellour in this heuristic derivation of Eq. 5 can be used in the case of the systems studied here.
Another methodology to characterize the dynamical behavior of a particle in hindered motion is related with the logarithmic derivative, that corresponds to the temporal behavior of the exponent γ in
In order to calculate the long-time diffusion coefficient
where
Thus, Eq. 9 can be written in terms of the MFPT as:
On the other hand, the diffusion coefficient of a Brownian particle in the presence of a periodic potential, according to the Kramers approach in the overdamped limit, can be written as [43].
where
which is the Dalle-Ferrier et al. [23] (DF) formula for a Brownian particle in a periodic cosine potential. According to Lifson and Jackson [30], the ratio of the long-time diffusion coefficient of a Brownian particle in the presence of a periodic potential
where the brackets
The corresponding MFPTs can be obtained by equating Eq. 11 with either Eq. 12 or Eq. 15 to yield:
and
where
is the time that a particle needs to move a distance L in pure Brownian motion, that is, in the absence of any external potential, when the diffusion constant of the particle is
A random walker simulation follows a similar recipe to obtain a trajectory as the Ermak and McCammon algorithm, however the choice of the displacement is generated randomly either following a distribution, or with a defined step size but without specification of the force or diffusion coefficient [27–29]. In our case, such distribution is flat of fixed width
FIGURE 2. (Color online)
In order to introduce the cage effect, the particle is located inside a periodic cell of length
The random walker model is also useful to study the typical mean first passage time problem, that will be used later to analyze our results in terms of the distribution of escape times. However, as in this case the particle either cross the barrier or not, in principle, the random walker undergoes escaping processes, characterized by an escape time
where
In order obtain the short time diffusion coefficient
FIGURE 3. (Color online): Mean square displacement MSD(t) of a spherical tracer as a function of time. The red solid squares and the blue dashed line correspond to the total mean square displacement (associated to the free diffusion in the
A more stringent test for the estimated value of
FIGURE 4. (Color online): Density of probability per unit length of finding a tracer
In the absence of an external field,
At longer times, the tracer starts to experience the external field and it is seen that: 1) the rate at which the maximum height
FIGURE 5. (Color online): Maximum of the density of probability of finding a tracer
The good agreement observed between experimental and simulation results presented above, motivates a study of long time dynamic properties of the tracer via Brownian dynamics simulations for a fixed periodicity L. The MSD of a spherical tracer is displayed in Figure 6 as a function of time and the amplitude of the periodic potential
FIGURE 6. (Color online): Mean square displacement MSD(t) of a spherical tracer as a function of time in the presence of a periodic cosine-like potential of amplitude
TABLE 1. Bellour fitting parameters
FIGURE 7. (Color online): Long-time diffusion coefficient
In Figure 6 it appears that the time
FIGURE 8. (Color online): Logarithmic derivative of the mean square displacement MSD(t) of a spherical tracer as a function of time in the presence of a periodic cosine-like potential of amplitude
FIGURE 9. (Color online): (A) Critical time
On the other hand, another interesting feature regarding the Bellour fitting is that the parameter
The above mentioned effect related with the decrease of the
FIGURE 10. (Color online) Random walker MSD fitting of Brownian dynamics simulations in which the size of the periodic cell is
In order to gain further insight about the independence of
where F is the frequency of events in the escape time histogram, A is the asymptotic value of F when
FIGURE 11. (Color online):Brownian dynamics histograms of the first passage time (FPT) τ for different barrier heights
On the other hand, all histograms displayed in Figure 11 are normalized dividing the value of F by the corresponding value of A, that is:
These normalized histograms are shown in Figure 12. Here, we observe that all normalized MFPT histograms collapse onto the same curve for different bin values. Moreover, it is observed that the most likely FPT
FIGURE 12. (Color online): Normalized histograms of the first passage times for different barrier heights
FIGURE 13. (Color online): Escape time
As pointed out above, important features of the motion of a colloidal particle in a periodic potential are also presented in the simple model of a random walker. Another interesting quantity is the escape time whose histograms for the random walker simulation were also calculated and shown in Figure 14. The normalization of the histograms were performed following the same protocol used in the BD simulations. Here, it can be observed a behavior analogous to that observed by BD simulations: the frequency of short escape times increases as the escape time increases. This augment of the frequency of occurrence of the escape time is observed until a critical value
FIGURE 14. (Color online) Normalized histograms of the escape times obtained from the random walker simulations. The data are labeled according to the target Brownian dynamics simulations displayed in Figure 10. Vertical blue, green, and red dashed lines represent the mean time
Let us define now
In this work, we have studied some dynamic properties of a Brownian tracer in two periodic potentials at short- and long-times. For the experimentally obtained harmonic potential, at short-times, the proposed protocol allowed us to estimate the short time diffusion coefficient
At the level of the MSD, it was observed that a plateau and a change of curvature appear and become more conspicuous when the
In order to locate clearly the time
The two periodic potentials studied here via experiments, Brownian dynamics, and random walker simulations constitute very simple models useful to characterize or describe more complex systems such as dense polyelectrolyte solutions, jammed spheres, and even crowded biological structures as those found inside living cells. In this sense, if our simple models are able to provide the long time diffusion coefficient
The raw data supporting the conclusions of this article will be made available by the authors, without undue reservation.
ES-G and JA-L designed the experiments. ES-G and DP-G implemented the experimental set-up. DP-G performed the experiments. GG-G and DP-G implemented the BD simulations. ES-G designed and implemented the random walker simulation. GG-G, DP-G, JA-L and ES-G analyzed the data. GG-G, DP-G, JA-L and ES-G wrote the manuscript.
GG-G acknowledges the Marcos Moshinsky Fellowship, the SEP-CONACYT CB-2016 grant 286105, the PRODEP grant UASLP-PTC-652 511-6/2020-8585, the CONACYT grants 261210 and FC-2015-2-1155, and the Laboratorio Nacional de Ingeniería de la Materia Fuera de Equilibrio-279887-2017 for the financial funding. ES-G acknowledges CONACYT Mexico through grant A1-S-9098. Authors thanks Prof. Stefan Egelhaaf for useful discussions.
The authors declare that the research was conducted in the absence of any commercial or financial relationship that could be construed as a potential conflict of interest.
The authors thankfully acknowledge computer resources, technical advice and support provided by the Laboratorio Nacional de Supercómputo del Sureste de México (LNS), a member of the CONACYT national laboratories, with project No. 202001024N; as well as the Centro Nacional of Supercómputo (CNS) for the computing time provided at THUBAT KAAL II. GG-G. expresses his gratitude for the assistance from the computer technicians at the IF-UASLP.
As both the Dalle-Ferrier et al. formula, and the Bellour fitting parameter
FIGURE 15. (Color online): (A) Error of the long-time diffusion coefficient obtained from the Dalle-Ferrier formula
1. Golding I, Cox EC. Physical nature of bacterial cytoplasm. PRL (2006) 96:098102. doi:10.1103/physrevlett.96.098102
2. Tolić-Nørrelykke IM, Munteanu EL, Thon G, Oddershede L, Berg-Sørensen K. Anomalous diffusion in living yeast cells. Phys Rev Lett (2004) 93:078102. doi:10.1103/PhysRevLett.93.078102
3. Weiss M, Elsner M, Kartberg F, Nilsson T. Anomalous subdiffusion is a measure for cytoplasmic crowding in living cells. Biophys J (2004) 87:3518–24. doi:10.1529/biophysj.104.044263
4. Xia Q, Xiao H, Pan Y, Wang L. Microrheology, advances in methods and insights. Adv Coll Int Sci (2018) 257:71–85. doi:10.1016/j.cis.2018.04.008
5. Sarmiento-Gomez E, Lopez-Diaz D, Castillo R. Microrheology and characteristic lengths in wormlike micelles made of a zwitterionic surfactant and sds in brine. J Phys Chem B (2010) 114:12193–202. doi:10.1021/jp104996h
6. Mason TG, Weitz DA. Optical measurements of frequency-dependent linear viscoelastic moduli of complex fluids. Phys Rev Lett (1995) 74:1250–3. doi:10.1103/physrevlett.74.1250
7. Schnurr B, Gittes F, MacKintosh FC, Schmidt CF. Determining microscopic viscoelasticity in flexible and semiflexible polymer networks from thermal fluctuations. Macromolecules (1997) 30:7781–92. doi:10.1021/ma970555n
8. Amblard F, Maggs AC, Yurke B, Pargellis A, Leibler S. Subdiffusion and anomalous local viscoelasticity in actin networks. Phys Rev Lett (1999) 77:4470. doi:10.1103/PhysRevLett.77.4470
9. Bellour M, Skouri M, Munch JP, Hébraud P. Brownian motion of particles embedded in a solution of giant micelles. Eur Phys J E (2002) 8:431–6. doi:10.1140/epje/i2002-10026-0
10. Hunter GL, Weeks ER. The physics of the colloidal glass transition. Rep Prog Phys (2012) 75:066501. doi:10.1088/0034-4885/75/6/066501
11. Weeks ER. Introduction to the colloidal glass transition. ACS Macro Lett (2017) 6:27–34. doi:10.1021/acsmacrolett.6b00826
12. Löwen H. Colloidal dispersions in external fields: recent developments. J Phys Condens Matter (2008) 20:404201. doi:10.1088/0953-8984/20/40/404201
13. Zhu J, Li M, Rogers R, Meyer W, Ottewill RH, Russel WB, et al. Crystallization of hard-sphere colloids in microgravity. Nature (1997) 387:883–5. doi:10.1038/43141
14. Sprenger HJ, Marquardt P, Tadros TF. The scope of microgravity experiments in colloid science: major conclusions from the workshop. Adv Coll Int Sci (1993) 46:343–7. doi:10.1016/0001-8686(93)80048-g
15. Bailey AE, Poon WCK, Christianson RJ, Schofield AB, Gasser U, Prasad V, et al. Spinodal decomposition in a model colloid-polymer mixture in microgravity. Phys Rev Lett (2007) 99:205701. doi:10.1103/physrevlett.99.205701
16. Pesce G, Volpe G, Maragó OM, Jones PH, Gigan S, Sasso A, et al. Step-by-step guide to the realization of advanced optical tweezers. J Opt Soc Am B (2015) 32:B84–98. doi:10.1364/josab.32.000b84
17. Evers F, Hanes RDL, Zunke C, Capellmann RF, Bewerunge J, Dalle-Ferrier C, et al. Colloids in light fields: particle dynamics in random and periodic energy landscapes. Eur Phys J Spec Top (2013) 222:2995–3009. doi:10.1140/epjst/e2013-02071-2
18. Romero AH, Sancho JM. Brownian motion in short range random potentials. Phys Rev E (1998) 58:2833–7. doi:10.1103/physreve.58.2833
19. Sancho JM, Lacasta AM, Lindenberg K, Sokolov IM, Romero AH. Diffusion on a solid surface: anomalous is normal. Phys Rev Lett (2004) 92:250601. doi:10.1103/physrevlett.92.250601
20. Schmiedeberg M, Roth J, Stark H. Brownian particles in random and quasicrystalline potentials: how they approach the equilibrium. Eur Phys J E Soft Matter (2007) 24:367–77. doi:10.1140/epje/i2007-10247-7
21. Hanes RDL, Schmiedeberg M, Egelhaaf SU. Brownian particles on rough substrates: relation between intermediate subdiffusion and asymptotic long-time diffusion. Phys Rev Lett (2013) 88:062133. doi:10.1103/physreve.88.062133
23. Dalle-Ferrier C, Krüger M, Hanes RDL, Walta S, Jenkins MC, Egelhaaf SU. Dynamics of dilute colloidal suspensions in modulated potentials. Soft Matter (2011) 7:2064–75. doi:10.1039/c0sm01051k
24. Velarde SH, Castaneda-Priego R. Diffusion in two-dimensional colloidal systems on periodic substrates. Phys Rev E (2009) 79:041407. doi:10.1103/PhysRevE.79.041407
25. Wei QH, Bechinger C, Rudhardt D, Leiderer P. Experimental study of laser-induced melting in two-dimensional colloids. Phys Rev Lett (1998) 81:2606. doi:10.1103/physrevlett.81.2606
26. Capellmann RF, Bewerunge J, Platten F, Egelhaaf SU. Note: using a Kösters prism to create a fringe pattern. Rev Scientific Instr (2017) 88:056102. doi:10.1063/1.4982587
27. Metzler R, Jeon JH, Cherstvy AG, Barkai E. Anomalous diffusion models and their properties: non-stationarity, non-ergodicity, and ageing at the centenary of single particle tracking. Phys Chem Chem Phys (2014) 16:24128–64. doi:10.1039/c4cp03465a
28. Metzler R, Klafter J. The random walk’s guide to anomalous diffusion: a fractional dynamics approach. Phys Rep (2000) 339:1–77. doi:10.1016/s0370-1573(00)00070-3
29. Muñoz-Gil G, Garcia-March MA, Manzo C, Celi A, Lewenstein M. Diffusion through a network of compartments separated by partially-transmitting boundaries. Front Phys (2019) 7:31. doi:10.3389/fphy.2019.00031
30. Lifson S, Jackson JL. On the self-diffusion of ions in a polyelectrolyte solution. J Chem Phys (1962) 36:2410–4. doi:10.1063/1.1732899
31. Hänggi P, Talkner P, Borkovec M. Reaction-rate theory: fifty years after kramers. Rev Mod Phys (1990) 62:251–341. doi:10.1103/revmodphys.62.251
32. Megen WV, Underwood SM. The glass transition in colloidal hard spheres. J Phys Condens Matter (1994) 6:A181–186. doi:10.1088/0953-8984/6/23a/026
33. Carvajal-Tinoco MD, de León GC, Arauz-Lara JL. Brownian motion in quasibidimensional colloidal suspensions. Phys Rev E (1997) 56:6962–9. doi:10.1103/PhysRevE.56.6962
34. Sarmiento-Gómez E, Rivera-Morán JA, Arauz-Lara JL. Energy landscape of colloidal dumbbells in a periodic distribution of light. Soft Matter (2019) 15:3573–9. doi:10.1039/c9sm00472f
35. Jenkins MC, Egelhaaf SU. Colloidal suspensions in modulated light fields. J Phys Condens Matter (2008) 20:404220. doi:10.1088/0953-8984/20/40/404220
36. Ashkin A. Forces of a single-beam gradient laser trap on a dielectric sphere in the ray optics regime. Biophys J (1992) 61:569–82. doi:10.1016/s0006-3495(92)81860-x
37. Harada Y, Asakura T. Radiation forces on a dielectric sphere in the Rayleigh scattering regime. Opt Commun (1996) 124:529–41. doi:10.1016/0030-4018(95)00753-9
38. Allan DB, Caswell TA, Keim NC. trackpy: Trackpy v0.3.2 (Version v0.3.2). Zenodo (2019). doi:10.5281/zenodo.60550
39. Crocker JC, Grier DG. Methods of digital video microscopy for colloidal studies. J Colloid Interf Sci (1996) 179:298–310. doi:10.1006/jcis.1996.0217
40. Ermak DL. A computer simulation of charged particles in solution. i. technique and equilibrium properties. J Chem Phys (1975) 62:4189–96. doi:10.1063/1.430300
41. Pérez-Guerrero D. Statistical transitions of colloidal particles into two-dimensional periodic external potentials (in Spanish). [Bachelor’s thesis]. San Luis (México): Facultad de Ciencias de la Universidad Autónoma de San Luis Potosí (2018).
42. Ferrando R, Spadacini R, Tommei GE. Kramers problem in periodic potentials: jump rate and jump lengths. Phys Rev E (1993) 48:2437–51. doi:10.1103/physreve.48.2437
43. Pavliotis GA, Vogiannou A. Diffusive transport in periodic potentials: underdamped dynamics. Fluct Noise Lett (2008) 08:L155–L173. doi:10.1142/s0219477508004453
44. McLeish TCB. Thermal barrier hopping in biological Physics. Boca Raton, Florida: CRC Press (2006). p. 123–35.
45. Sarmiento-Gómez E, Villanueva-Valencia JR, Herrera-Velarde S, Ruiz-Santoyo JA, Santana-Solano J, Arauz-Lara JL, et al. Short-time dynamics of monomers and dimers in quasi-two-dimensional colloidal mixtures. Phys Rev E (2016) 94:012608. doi:10.1103/physreve.94.012608
46. Rupprecht JF, Bénichou O, Grebenkov DS, Voituriez R. Exit time distribution in spherically symmetric two-dimensional domains. J Stat Phys (2014) 158:192–230. doi:10.1007/s10955-014-1116-6
47. Mattos TG, Carlos MM, Metzler R, Oshanin G. First passages in bounded domains: when is the mean first passage time meaningful?. Phys Rev E (2012) 86:031143. doi:10.1103/physreve.86.031143
48. Singer A, Schuss Z, Holcman D. Narrow escape, part iii: non-smooth domains and riemann surfaces. J Stat Phys (2006) 122:491–509. doi:10.1007/s10955-005-8028-4
49. Mejía-Monasterio C, Oshanin G, Schehr G. First passages for a search by a swarm of independent random searchers. J Stat Mech Theor Exp (2011) 2011:P06022. doi:10.1088/1742-5468/2011/06/p06022
50. Chupeau M, Gladrow J, Chepelianskii A, Keyser UF, Trizac E. Optimizing brownian escape rates by potential shaping. Proc Natl Acad Sci (2020) 117:1383–8. doi:10.1073/pnas.1910677116
51. Coughlan ACH, Torres-Díaz I, Zhang J, Bevan MA. Non-equilibrium steady-state colloidal assembly dynamics. J Chem Phys (2019) 150:204902. doi:10.1063/1.5094554
52. Edwards T, Yang Y, Beltran-Villegas D, Bevan M. Colloidal crystal grain boundary formation and motion. Scientific Rep (2014) 4:6132. doi:10.1038/srep06132
Keywords: periodic potential, external field, spherical tracer, long time diffusion, most likely escape time
Citation: Pérez-Guerrero D, Arauz-Lara JL, Sarmiento-Gómez E and Guerrero-García GI (2021) On the Time Transition Between Short- and Long-Time Regimes of Colloidal Particles in External Periodic Potentials. Front. Phys. 9:635269. doi: 10.3389/fphy.2021.635269
Received: 30 November 2020; Accepted: 03 February 2021;
Published: 22 April 2021.
Edited by:
Atahualpa Kraemer, National Autonomous University of Mexico, MexicoReviewed by:
Michael Schmiedeberg, Friedrich-Alexander-Universität Erlangen-Nürnberg, GermanyCopyright © 2021 Pérez-Guerrero, Arauz-Lara, Sarmiento-Gómez and Guerrero-García. This is an open-access article distributed under the terms of the Creative Commons Attribution License (CC BY). The use, distribution or reproduction in other forums is permitted, provided the original author(s) and the copyright owner(s) are credited and that the original publication in this journal is cited, in accordance with accepted academic practice. No use, distribution or reproduction is permitted which does not comply with these terms.
*Correspondence: Erick Sarmiento-Gómez, ZXNhcm1pZW50b0BmaXNpY2EudWd0by5teA==; Guillermo Iván Guerrero-García, Z2l2YW5AdWFzbHAubXg=
Disclaimer: All claims expressed in this article are solely those of the authors and do not necessarily represent those of their affiliated organizations, or those of the publisher, the editors and the reviewers. Any product that may be evaluated in this article or claim that may be made by its manufacturer is not guaranteed or endorsed by the publisher.
Research integrity at Frontiers
Learn more about the work of our research integrity team to safeguard the quality of each article we publish.