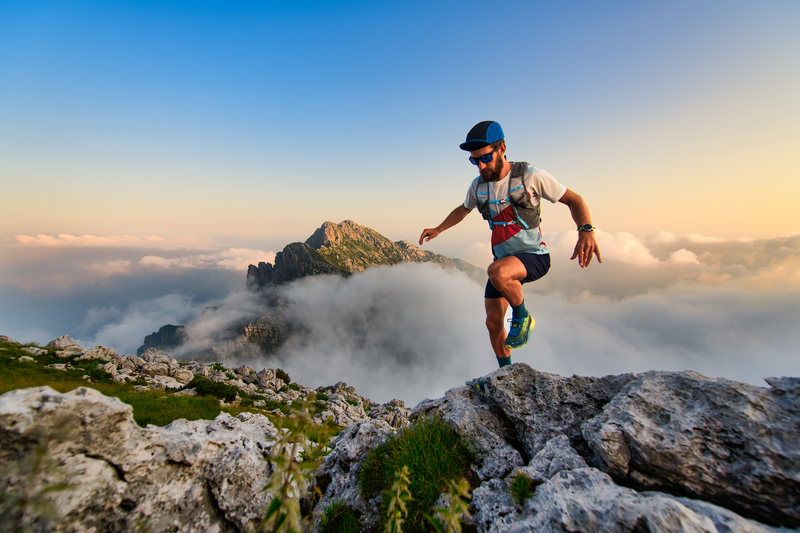
94% of researchers rate our articles as excellent or good
Learn more about the work of our research integrity team to safeguard the quality of each article we publish.
Find out more
EDITORIAL article
Front. Phys. , 19 January 2021
Sec. Interdisciplinary Physics
Volume 8 - 2020 | https://doi.org/10.3389/fphy.2020.633494
This article is part of the Research Topic Fundamentals and Applications of AI: An Interdisciplinary Perspective View all 12 articles
Editorial on the Research Topic
Fundamentals and Applications of AI: An Interdisciplinary Perspective
Machines, computers, and algorithms appear recurrently in the future imagined in science fiction pieces. Terminator’s Skynet, Space Odyssey’s HAL 9000, Psychohistory of Asimov’s Foundation, and Westworld’s Dolores are just a few examples of our collective imaginary of a (days) topic future. Interestingly, the year 2019 has represented the future in some works. Toronto Star in 1983 asked Asimov to predict the future, the world of 2019. This is also the case for Blade Runner, where the action runs in a dystopic LA, in 2019, with replicants having “almost” human cognitive capabilities. Although we have not reached most of the utopian pictures, the growth of Big Data and Artificial Intelligence algorithms is unquestionable (Figure 1). Thus, celebrating the unstoppable advance of AI, we collect in this RT several studies addressing fundaments and applications from a physics perspective.
FIGURE 1. Growth of the number of documents related to artificial intelligence in (A) the WoS and (B) Dimensions. (A) Between 1990 and 2010, the growth seems exponential with a doubling time of 8 years for artificial intelligence and 3.5 years for machine learning. In more recent years, the growth is even faster. Number of documents obtained in the Web of Science with the terms “artificial intelligence,” “machine learning,” and “deep learning.” (B) The number of documents identified in any Frontiers journal also shows an exponential growth with a doubling time smaller than 2 years. Number of documents obtained in Dimensions with the terms “artificial intelligence,” “machine learning,” and “deep learning” and filtering by Frontiers journals.
In 2020, AI has continued its penetration into classical fields and emerging technologies. Fueled by deep learning and the automatic generation of data, the techniques developed in AI are being applied to predict and control physical, biological, engineering, and even commercial systems. Given the two-way interaction between AI and different fields and including how these fields inspire novel methods and theory in AI, we had envisioned a volume illustrating such an interdisciplinary perspective. Contributions include quantum annealers and quantum neural networks, echo state networks, machine learning (reinforcement learning and graph-based methods), and applications to optimization, classification of heartbeats, animal collective movement, and climate forecast, and the use of AI to discover physical laws.
A fast machine learning model for ECG-based heartbeat classification and arrhythmia detection was developed, based on echo state networks [1]. The classifier requires a small number of features and a single ECG signal suffices. The possibility of using a combination of ensembles allows them to exploit parallelism to train the classifier with remarkable speed. The sensitivity and predictive values are comparable with those of the state of the art in fully automatic ECG classifiers and even outperform other ECG classifiers that follow more complex feature selection approaches.
Reservoir computers are investigated in two contributions. First, a coherent all-optical fiber-ring reservoir computer with distributed Kerr nonlinearity is investigated numerically and experimentally [2]. The system is based on a passive coherent optical fiber-ring cavity where part of the nonlinearity is due to the Kerr effect. They compare the nonlinear transformations of information in the reservoir’s input layer, the reservoir itself, and the readout layer. They find that the Kerr effect enhances the computational capability of the reservoir, in particular, its nonlinear computational capacity. Second, the trade-off between information processing capacity and rate is analyzed in Ref. [3] using a delay-based reservoir computer. Delay-based reservoir computers have a trade-off between computational capacity and processing speed due to the nonzero response time of the nonlinear node. They find that the computational capacity degrades for a sampling output rate that is higher than the inverse of the response time of the system. Moreover, the computational capacity also depends on the misalignment between the delay time of the nonlinear node and the data injection time.
In the realm of biology, the collective behavior of animals is a fascinating field aiming to understand how patterns of coordination among a large number of individuals emerge as a result of local interactions. Inferring the rules that give rise to a certain behavior is a challenging problem as any occasional observer of flocks and fish schools can attest. Ref. [4] uses the framework of reinforcement learning, machine learning techniques in which an agent acts in an environment and learns to maximize a reward signal, to model the problem of automatically discovering local rules of interaction that would lead to a desired collective behavior. To that end, Costa et al. apply evolutionary strategies to optimize a single policy (mapping from sensory inputs to actions) followed by the agents so that a desired collective behavior would emerge.
The potential of graph-based methods in combination with machine learning algorithms is addressed in Ref. [5]. The authors explore two methods to detect outliers, with applications to high-dimensional datasets. The first method measures the fragmentation of a graph, where the data samples are the nodes of the graph, while the second method is based on the Isomap algorithm, a dimensionality reduction technique. The performance is compared with alternative methods and assessed on the dependence on the size of an anomalous region within an image, a known problem in anomaly and outlier detection.
An example of large-scale coordination occurs in the climate and ocean circulation systems. Predicting temporal patterns such as El Nino oscillation is a problem of considerable practical and fundamental interest. Ref. [6] reviews different machine learning techniques to predict El Niño events for lead times larger than 12 months and studies which type of attributes is most relevant for an accurate forecast. The review focuses on feed-forward artificial neural networks from early work back in the late 90s to the more recent graph-based methods.
Quantum approaches are explored in three contributions. Refs. [7 and 8] implement quantum annealers to solve quadratic unconstrained binary optimization (QUBO) in two applications: an industrial problem, the control of automated guided vehicles in a factory [7], and the problem of item listing optimization for e-commerce [8]. The contributions probe the capacity of quantum annealers to address industrial and commercial problems stimulating further research of quantum annealers to solve optimization problems in real-world systems. Ref. [9] addresses the no-cloning theorem–the impossibility to duplicate a quantum state—and how to circumvent this limitation for applying quantum computing. In particular, they obtain lower bounds of input redundancy, that is, how many times the data must be reintroduced in parameterized quantum circuits (PQCs) (also referred to as quantum neural networks or variational quantum circuits). This contribution analyzes two different functions for the encoding (linear encoding and arcsin encoding) and proves that lower bounds are logarithmic in terms of a linear algebraic complexity measure of the target function.
A different approach is the implementation of internal processing capacity in models of complex systems, in particular, agent-based models. Ref. [10] introduces an agent-based model with cognitive and social dynamics, the Bayesian word learning model, to study the effects of cognitive and social dynamics on the emergence of linguistic consensus in the naming game. In the game, agents learn new words by generalization, using Bayes statistics, from previous experience. The novelty of the approach, with agents modeled after a Bayesian inference framework, captures important properties of human behavior and learning and opens the possibility to study applications to language, semiotics cognitive science, and complex systems.
Finally, a research line that is attracting interest is the development of an automated scientist, to extract interpretable dynamics and laws directly from data. In this direction, Ref. 11 uses machine learning and data-driven approaches, the sparse identification of nonlinear dynamics algorithm, to model the motion of falling objects. One conclusion is that blindly applying machine learning techniques can be inappropriate without using domain-specific knowledge. Additionally, the contribution addresses interesting issues related to model “discoverability” and “interpretability,” important concepts that we are only starting to understand, and that can play an important role in the future. Indeed, AI is already being used to learn models from quantum mechanics to statistical physics, and this trend can only be expected to grow.
All authors contributed to the writing of the editorial.
The authors declare that the research was conducted in the absence of any commercial or financial relationships that could be construed as a potential conflict of interest.
We acknowledge funding by Agencia Estatal de Investigación (AEI) and Fondo Europeo de Desarrollo Regional (FEDER) through project SPASIMM FIS2016-80067-P (AEI/FEDER, UE), the Spanish State Research Agency through the María de Maeztu Program for Units of Excellence in R&D (MDM-2017-0711 to IFISC). We also acknowledge support from Frontiers Editorial Office, in special to Marta Brucka who obtained the data for Figure 1B.
1. Alfaras M, Soriano MC, Ortín S. A fast machine learning model for ECG-based heartbeat classification and Arrhythmia detection. Front Phys (2019) 7:103. doi:10.3389/fphy.2019.00103
2. Pauwels J, Verschaffelt G, Massar S, Van der Sande G. Distributed Kerr non-linearity in a coherent all-optical fiber-ring reservoir computer. Front Phys (2019) 7:138. doi:10.3389/fphy.2019.00138
3. Ortín S, Pesquera L. Tackling the trade-off between information processing capacity and rate in delay-based reservoir computers. Front Phys (2019) 7:210. doi:10.3389/fphy.2019.00210
4. Costa T, Laan A, Heras FJH, de Polavieja GG. Automated discovery of local rules for desired collective-level behavior through reinforcement learning. Front Phys (2020) 8:200. doi:10.3389/fphy.2020.00200
5. Amil P, Almeira N, Masoller C. Outlier mining methods based on graph structure analysis. Front Phys (2019) 7:194. doi:10.3389/fphy.2019.00194
6. Dijkstra HA, Petersik P, Hernández-García E, López C. The application of machine learning techniques to improve El Niño prediction skill. Front Phys (2019) 7:153. doi:10.3389/fphy.2019.00153
7. Ohzeki M, Miki A, Miyama MJ, Terabe M. Control of automated guided vehicles without collision by quantum annealer and digital devices. Front Comput Sci (2019) 1:9. doi:10.3389/fcomp.2019.00009
8. Nishimura N, Tanahashi K, Suganuma K, Miyama MJ, Ohzeki M. Item listing optimization for E-commerce websites based on diversity. Front Comput Sci (2019) 1:2. doi:10.3389/fcomp.2019.00002
9. Gil Vidal FJ, Theis DO. Input redundancy for parameterized quantum circuits. Front Phys (2020) 8:297. doi:10.3389/fphy.2020.00297
10. Marchetti G, Patriarca M, Heinsalu E. A Bayesian approach to the naming game model. Front Phys (2020) 8:10. doi:10.3389/fphy.2020.00010
Keywords: artificial intelligence, applications, fundamentals, multidisciplinary and interdisciplinary approach, machine learning
Citation: Eguíluz VM, Mirasso CR and Vicente R (2021) Editorial: Fundamentals and Applications of AI: An Interdisciplinary Perspective. Front. Phys. 8:633494. doi: 10.3389/fphy.2020.633494
Received: 25 November 2020; Accepted: 30 November 2020;
Published: 19 January 2021.
Edited and reviewed by:
Alex Hansen, Norwegian University of Science and Technology, NorwayCopyright © 2021 Eguíluz, Mirasso and Vicente. This is an open-access article distributed under the terms of the Creative Commons Attribution License (CC BY). The use, distribution or reproduction in other forums is permitted, provided the original author(s) and the copyright owner(s) are credited and that the original publication in this journal is cited, in accordance with accepted academic practice. No use, distribution or reproduction is permitted which does not comply with these terms.
*Correspondence: Víctor M. Eguíluz, dmljdG9yQGlmaXNjLnVpYi1jc2ljLmVz
Disclaimer: All claims expressed in this article are solely those of the authors and do not necessarily represent those of their affiliated organizations, or those of the publisher, the editors and the reviewers. Any product that may be evaluated in this article or claim that may be made by its manufacturer is not guaranteed or endorsed by the publisher.
Research integrity at Frontiers
Learn more about the work of our research integrity team to safeguard the quality of each article we publish.