- 1Department of Mechanical Engineering, Northern Illinois University, DeKalb, IL, United States
- 2Department of Mechanical and Aerospace Engineering, Tandon School of Engineering, New York University, Brooklyn, NY, United States
- 3Department of Biomedical Engineering, Tandon School of Engineering, New York University, Brooklyn, NY, United States
- 4Center for Urban Science and Progress, Tandon School of Engineering, New York University, Brooklyn, NY, United States
In an emergency evacuation, people almost always come in close proximity as they quickly leave a built environment under a potential threat. With COVID19, this situation presents yet another challenge: that of getting unintentionally exposed to an infected individual. To assess the epidemiological consequences of an emergency evacuation, we expanded a popular pedestrian dynamic model to enable social distancing during a normal exit and analyze the effect of possible transmission through respiratory droplets and aerosol. Computer simulations point to a troubling outcome, whereby the benefits of a quick exit could be outweighed by the risk of infection.
Introduction
As schools and universities continue to evaluate various social distancing strategies to mitigate the spread of COVID19, a critical feature of human behavior is being overlooked—the response to a sudden alarm in a built environment that may trigger an emergency evacuation. The alarm may come from a fire in the building, the presence of an active shooter, or even a simple drill to prepare for true emergencies. Perhaps, in the current context, even someone blatantly unwilling to comply with social distancing regulations and use of masks could trigger an alarm. Whatever the source of the alarm is, during an evacuation, individuals will likely weigh the risks of being injured from the perceived threat heavily against the possibility of contracting an infection from a classmate or the instructor. Upon exiting however, people may wonder if they got too close to an infected person and if they breathed the same air for too long. These aspects represent an important discussion in relation to airborne transmission of COVID19 [1, 2].
The recommended separation distance of 2 m (six feet) is largely based on the transport of “respiratory droplets produced when an infected person coughs, sneezes, or talks.” [2]. These droplets can be propelled through air for up to 2 m and “land in the mouths or noses of people who are nearby or possibly be inhaled into the lungs.” [2]. More recently, results from fluid mechanics research have shown that aerosol could be the dominant driving mechanism for transmission between people in close proximity [3]. In comparison with respiratory droplets, aerosol includes much smaller particles that remain suspended in air for long periods of time to be inhaled by others. Irrespective of the driving mechanism, proximity to an infected individual is likely to increase the risk of infection, especially in the presence of screaming.
Risk of Infection From an Individual as a Function of Their Physical Proximity
Under the premise that the risk of an infection increases with the proximity to an infected individual, we could quantify the epidemiological consequences of an evacuation by tracking the separation distance within the crowd. We assume that the risk of infection decays exponentially with distance [3], and that this risk accumulates over time. More specifically, we measure the risk of exposure in a crowd of N people from an infected individual I as the instantaneous exposure integrated over time for the duration of the evacuation:
An estimate of the value of
Simulating Emergency Evacuations and Normal Exit With Social Distancing
Emergency evacuations represent a dire situation where people exit a built environment as quickly as possible to escape the perceived danger. In an evacuation, the resulting crowd dynamics arise from a complex interplay between psychological, social, and physical factors. Individuals use social, cognitive, visual, and physical cues to stay with friends and family [5], look for the exit [6], and avoid collisions and injury [7]. Evacuation is therefore a cognitively demanding situation, which makes it inevitable for individuals to come close to each other—much less than the stipulated 2-m distance. Could this increase the risk of contracting COVID19?
Experiments on evacuation are impractical and potentially dangerous to conduct. A number of agent-based, mathematical models have been proposed over the years to predict human response and support hypothesis-driven experiments to clarify the mechanisms of the crowd dynamics. Among those, the social force model originally proposed by Helbing et al. [8] constitutes a viable compromise between model complexity and predictive power. The social force model is a physics-based model that captures interactions between finite-sized particles (agents) in the form of four kinds of forces: a social force that keeps agents apart; a goal force that makes them orient and move toward a goal location; a physical force in the event of friction and collision between agents; and a wall force, which is the same as the social force but captures interaction with walls and obstacles instead of other agents. Computer simulations can reproduce several real-world phenomena, including occurrences of bottlenecks near exits, injuries during an evacuation of a large crowd, and lane formation in corridors. The social force model has been validated in laboratory experiments [9], as well as real-world scenarios [7], thereby constituting a valid framework for exploring the potential epidemiological implications of an evacuation. By combining the classical evacuation model from Helbing et al. [8] with the proposed definition of risk of exposure, it is possible to provide a first assessment of the epidemiological consequences of an evacuation, compared to a normal exit where people can exercise social distancing.
The social force model [8] captures the motion of agent
To quantify and compare the risk associated with an emergency evacuation, we simulated two scenarios: evacuation and normal exit with social distancing. To simulate these two scenarios, we varied the interaction range (parameter B in the model), interaction repulsive force (parameter A in the model), and desired speed (parameter
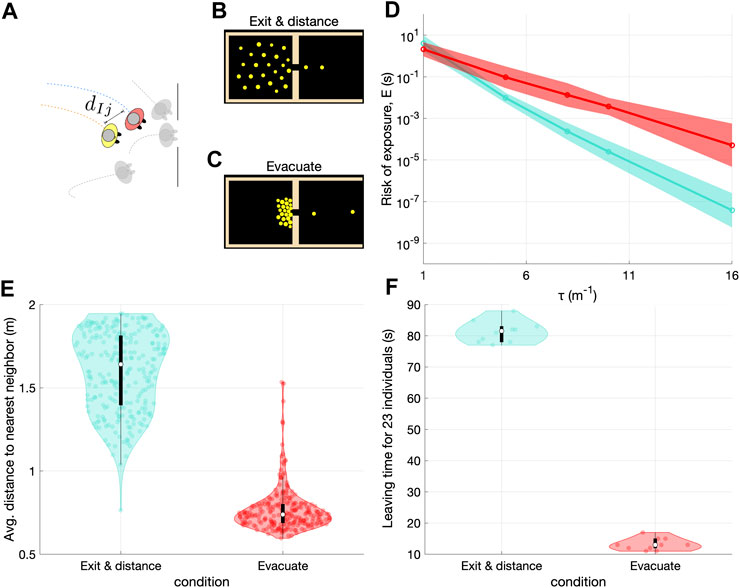
FIGURE 1. The epidemiological risks from coming close to an individual during an evacuation could outweigh the benefits of being able to quickly leave a room under a potential threat (A) The framework used here combines a pedestrian dynamic model and an exposure model to quantify the risk of exposure during an emergency evacuation (B) and (C), Snapshots from a simulation of the social force model [8] as 25 agents exit a 10 × 10 m room through a 1-m wide door while maintaining social distance or evacuate without maintaining social distance (D), Risk of exposure of an individual in the crowd as a function of the decay rate of the transmission; a low value of τ indicates high transmission at larger separation distances (red denotes evacuating, and turquoize identifies exiting and social distancing) (E), Average distance to the nearest neighbor during the two types of simulations performed (red denotes evacuation without distancing, and turquoize identifies exiting with distancing) (F), Leaving time for more than ninety percent of the crowd for the two scenarios.
Specifically, evacuation was simulated by setting
To prevent goal and interaction forces from balancing out to an equilibrium for the exit with social distancing scenario, the goal force was multiplied by a factor
For each simulation, we randomly placed 25 agents (modeled as finite-sized circles) within a 10 × 10 m room with a single 1 m wide exit; this number of individuals is sufficiently low to allow for maintaining a separation distance of 2 m within the room. Randomness in the simulation was introduced through two means: first, ten simulations were performed in each scenario, where each simulation corresponded to a different initial condition and the distribution of agent size (circles with diameters ranging uniformly between 0.5 and 0.7 m), and, second, by selecting a different agent as the single infected agent within the crowd. This amounted to hundred different realizations of each scenario. The risk of exposure, E, was computed for each scenario for different values of
Figure 1D shows that the exposure for an agent within the evacuating crowd without social distancing is much larger than when the crowd leaves normally and maintains social distance, despite the evacuating crowd leaves the arena much sooner than a crowd that normally exits and maintains social distance (Figure 1F). Figure 1E confirms that the agents maintain larger distances as a result of the higher interaction range and repulsive force encoded into the model. Should the simulation be performed with a larger group of 100 agents within a room of
Discussion
Our simulations show that despite the evacuating crowd taking only a sixth of the time to leave the room than when the crowd which is exiting normally, evacuation presents a far greater threat for possible transmission of COVID19 than a crowd that is exiting with social distancing. For example, in the case of aerosol transmission, evacuating in the presence of an infected individual who is coughing will yield a risk of exposure due to aerosol transmission of about 0.1 s (above the estimate of the threshold of close contact), while exit with social distancing will cause an average exposure ten time smaller (below the estimate of the threshold of close contact).
A vast community of researchers is focused on understanding how the flow of individuals during an emergency evacuation can be eased to avoid bottlenecks and high pressures that could lead to injuries and fatalities [11]. COVID19 presents yet another complication, where we must also weigh our compulsion to run away from a potential threat against the possible risks involved in being in proximity to an infected individual. Our results indicate that maintaining social distancing during an exit could increase the time required to leave the built environment by a factor of ten, which may be fatal in the case of a fire or a mass shooting. At the same time, evacuating without maintaining a social distance dramatically increases the risk of exposure, potentially leading to further infections. Face coverings can certainly help mitigate these risks, although more research is required to precisely evaluate the reduction in the decay rate associated with the proper use of masks, especially in the context of aerosol intake. Overall, this study points to a critical gap in the current guidelines for resuming in-presence learning, as well as opening up businesses during the coming fall.
Our analysis is not free of limitations, which should be investigated in further efforts, beyond the scope of this perspective. First of all, the pedestrian dynamics is described by one of the very first mathematical models in the field [8], which has seen several refinements throughout the last 20 years [12]. As such, one may attempt at more complex simulation of the evacuation process to capture perceptual and psychological factors that are missing in this model. Second, the simple evacuation scenario presented here is not able to fully capture the combination of exposure risks one may face when evacuating a building with multiple rooms, floors, stairways, and doorways. In such a complex scenario, it will also be important to trace the individuals who have experienced the strongest exposure; toward this aim it may be possible to borrow techniques from community detection in networks [13–15]. Third, the contact process examined herein does not account for individual orientation, which is likely to play an important role on droplet-based exposure, and, to a lesser extent, on airborne transmission [3]. This limitation for example could be overcome by following the approach proposed by Ronchi & Lovreglio [16] to combine risks of infections across a range of viable scenarios in a built environment. Overall, this study contributes to the general topic of safety-related issues during the current pandemic [17], by bringing forward preliminary evidence for the expected risks of infection during evacuations.
Data Availability Statement
The original contributions presented in the study are included in the article/Supplementary Material, further inquiries can be directed to the corresponding authors.
Author Contributions
All authors listed have made a substantial, direct, and intellectual contribution to the work and approved it for publication.
Funding
SB was supported by the National Science Foundation, under grant # CMMI-2027988, and MP was supported by the National Science Foundation, under grant # CMMI-2027990.
Conflict of Interest
The authors declare that the research was conducted in the absence of any commercial or financial relationships that could be construed as a potential conflict of interest.
References
1. Morawska L, Tang JW, Bahnfleth W, Bluyssen PM, Boerstra A, Buonanno G, et al. How can airborne transmission of COVID-19 indoors be minimised?. Environ Int (2020) 142:105832. doi:10.1016/j.envint.2020.105832
2. Seibert T, Allen DB, Eickhoff JC, Carrel AL. How to protect yourself and others. Centers for Disease Control and prevention (CDC) (2020). https://www.cdc.gov/coronavirus/2019-ncov/prevent-getting-sick/prevention.html.
3. Chen W, Zhang N, Wei J, Yen H-L, Li Y. Short-range airborne route dominates exposure of respiratory infection during close contact. Build Environ (2020) 176:106859. doi:10.1016/j.buildenv.2020.106859
4. Seibert T, Allen DB, Eickhoff JC, Carrel AL. When to quarantine. Centers for Disease Control and prevention (CDC) (2020). https://www.cdc.gov/coronavirus/2019-ncov/if-you-are-sick/quarantine.html.
5. Mawson AR. Mass panic and social attachment: the dynamics of human behavior. London: Routledge (2017).
6. Moussaïd M, Helbing D, Theraulaz G. How simple rules determine pedestrian behavior and crowd disasters. Proc Natl Acad Sci USA (2011) 108(17):6884–8. doi:10.1073/pnas.1016507108
7. Helbing D, Buzna L, Johansson A, Werner T. Self-organized pedestrian crowd dynamics: experiments, simulations, and design solutions. Transport Sci (2005) 39(1):1–24. doi:10.1287/trsc.1040.0108
8. Helbing D, Farkas I, Vicsek T. Simulating dynamical features of escape panic. Nature (2000) 407:487–90. doi:10.1038/35035023
9. Moussaïd M, Helbing D, Garnier S, Johansson A, Combe M, Theraulaz G. Experimental study of the behavioural mechanisms underlying self-organization in human crowds. Proc Biol Sci (2009) 276(1668):2755–62. doi:10.1098/rspb.2009.0405
10. Lee HRL, Bhatia A, Brynjarsdóttir J, Abaid N, Barbaro A, Butail S. Speed modulated social influence in evacuating pedestrian crowds. Collect Dyn (2020) 5:1–24. doi:10.17815/cd.2020.25
11. Schadschneider A, Klingsch W, Klüpfel H, Kretz T, Rogsch C, Seyfried A. Evacuation dynamics: Empirical results, modeling and applications In: R Meyers, editor Encyclopedia of complexity and systems science. New York: Springer (2009) p 3142–3176.
12. Martinez-Gil F, Lozano M, García-Fernández I, Fernández F. Modeling, evaluation, and scale on artificial pedestrians. ACM Comput Surv (2017) 50(5):1–35. doi:10.1145/3117808
13. Li HJ, Wang L, Zhang Y, Perc M. Optimization of identifiability for efficient community detection. New J Phys (2020) 22(6):063035. doi:10.1088/1367-2630/ab8e5e
14. Fortunato S, Hric D. Community detection in networks: a user guide. Phys Rep (2016) 659:1–44. doi:10.1016/j.physrep.2016.09.002
15. Cazabet R, Boudebza S, Rossetti G. Evaluating community detection algorithms for progressively evolving graphs. arXiv:2007.08635 (2020).
16. Ronchi E, Lovreglio R. EXPOSED: an occupant exposure model for confined spaces to retrofit crowd models during a pandemic. arXiv:2005.04007 (2020).
Keywords: COVID19, evacuation, aerosol, crowds, proximity
Citation: Butail S and Porfiri M (2021) The Effect of An Emergency Evacuation on the Spread of COVID19. Front. Phys. 8:631264. doi: 10.3389/fphy.2020.631264
Received: 19 November 2020; Accepted: 30 December 2020;
Published: 01 February 2021.
Edited by:
Lin Wang, University of Cambridge, United KingdomReviewed by:
Jianbo Wang, Southwest Petroleum University, ChinaHui-Jia Li, Beijing University of Posts and Telecommunications, China
Copyright © 2021 Butail and Porfiri. This is an open-access article distributed under the terms of the Creative Commons Attribution License (CC BY). The use, distribution or reproduction in other forums is permitted, provided the original author(s) and the copyright owner(s) are credited and that the original publication in this journal is cited, in accordance with accepted academic practice. No use, distribution or reproduction is permitted which does not comply with these terms.
*Correspondence: Sachit Butail, sbutail@niu.edu; Maurizio Porfiri, mporfiri@nyu.edu