- 1Key Lab of Medical Optical Technology and Instrument, Ministry of Education, Institute of Biomedical Optics and Optometry, University of Shanghai for Science and Technology, Shanghai, China
- 2Institute of Food Microbiology, School of Medical Instruments and Food Engineering, University of Shanghai for Science and Technology, Shanghai, China
- 3Key Laboratory of Modern Optical System, Engineering Research Center of Optical Instrument and System, Ministry of Education, University of Shanghai for Science and Technology, Shanghai, China
- 4School of Biomedical Engineering, Med-X Research Institute, Shanghai Jiao Tong University, Shanghai, China
- 5School of Physics, Foshan University, Foshan, China
Rapid detection of foodborne pathogens is one of the most effective ways to solve food safety problems. To achieve rapid and noninvasive detection and classification of foodborne pathogens, we modified a fiber confocal backscattering micro-spectral system to suit an extremely small biological sample, that is, a bacterium. This system offers single-bacterium level, label-free, convenient, and environmentally friendly characterization. Three categories of common foodborne pathogens (Salmonella typhimurium, Escherichia coli, and Staphylococcus aureus) were measured. The scattering spectrum ranging from 450 to 900 nm was selected, and by the model of principal component analysis (PCA) and error back propagation algorithm of back propagation neural network (BPNN), the backscattering microscopic spectra of three categories of pathogens were dimensionally reduced, identified, and classified. The results showed that the identification accuracy of three categories of pathogens was above 90%, under neutral, acidic, and alkaline culturing conditions, respectively. The preliminary results demonstrated the feasibility of using confocal backscattering microscopic spectra combined with PCA and BPNN algorithm to identify and classify single bacterium in a rapid, noninvasive, and label-free manner.
Introduction
At present, foodborne illness poses a serious public health threat. Foodborne pathogens are the major cause of foodborne illness [1]. According to World Health Organization (WHO) estimates, pathogenic microorganisms are responsible for 70% of the world's foodborne disease patients [2]. In the worldwide, there are 1.5 billion diarrhea cases caused by biocontamination of food every year, of which about 3 million resulted in death among children <5 years old [3]. Contaminants are widely distributed in nature, coming from air, water, or the feces of animals. Moreover, they are difficult to eliminate because some of them often adhere to the surface of food or medical devices and form a biofilm that resists sterilization. For instance, Staphylococcus aureus is commonly associated with device-related infections after an implantation or operation [4]. Hence, the detection of foodborne pathogens is an effective first step in controlling food safety and reducing clinical infection rates.
In recent years, numerous methods of detecting foodborne pathogens have been deployed, for example, enzyme-linked immunosorbent assay (ELISA) [5], bioluminescence detection [6], gene chip technology, and polymerase chain reaction (PCR) technology [7]. However, conventional pathogen detection methods for the detection and identification of different bacteria still have some defects. For example, if the marker of a gene probe is a radioactive group, it may harm the human body, with its subsequent treatment relatively difficult. An adenosine triphosphate (ATP) luminescence detection technology can detect the total number of microorganisms contained in food but cannot specifically detect a target microorganism. Enzyme-linked immunity usually has a high false-positive rate, which affects the accuracy of detection, and is time-consuming and laborious. In addition, traditional detection methods do not apply to many clinical instruments. Therefore, at present, in the field of rapid detection of foodborne pathogens, optical means are playing an increasingly important role [8]. Raman spectroscopy has been found to have increasing applications in the field of foodborne pathogen detection due to its rapid and ultra-sensitive properties [9, 10]. Pan et al. reported a near infrared (NIR) laser scattering imaging system for rapid and noninvasive classification of foodborne pathogens [11]. The study results explored the potential of this system combined with multivariate calibrations for classifying three categories of common bacteria. Wilson and Vigil proposed a dark-field imaging technique capable of automated identification of individual bacterium [12]. Various reports in the literature have demonstrated that strategies using optical methods are potentially able to identify foodborne pathogens noninvasively, in real time, and in vivo.
Optical scattering is the main form of the interaction of a tiny particle and light, while the characteristics of the scattered light are directly related to the characteristics of the scattering particles [13]. It is used to detect the structural variations of mitochondria, measurements of macromolecular dipole moments, structural changes in cells, mechanisms of swelling, and lysis of isolated mitochondria, among other applications [14–17]. All of these studies are based on scattering theory, that is, the principle that structural differences may cause scattering differences. The optical detection method based on elastic scattered light can measure and analyze the internal structure distribution and changes of living cells in a natural state without introducing an external medium. Backscattering spectra carry structural and chemical component information from inside the bacterial cell.
Here, a pilot study was carried out to identify three categories of label-free bacteria using a fiber confocal backscattering micro-spectrometer (FCBS). Every bacterial smear was measured by the FCBS. The collected spectra were dimensionally reduced based on principal component analysis (PCA), identified and classified based on back propagation neural network (BPNN) algorithm. Because artificial neural network algorithm has strong nonlinear mapping function, which the representative model is BPNN [18]. It is a multilayer feedforward network trained by an error back propagation algorithm and can get good prediction results.
Materials and Methods
Experimental Device
The FCBS is a combination of optical fiber confocal and elastic scattering to obtain the single-cell backscattering spectrum. This method has been published in our earlier study and was used for classification and identification of cancerous cells in previous studies [15]. Here, we introduced mainly a slight modification on FCBS suitable for bacteria detection. We improved the original optical fiber confocal backscattering spectrum system to reduce its light field diameter to fit small biological samples. The detailed improved methods are as following. The light is coupled by Port 3 of the fiber coupler to the optic probe, which is made up of a flat-field apochromatic (PLAN Apo) objective (NA = 0.4, 20X, Olympus, Japan) as a collimator and another PLAN Apo objective (NA = 0.65, 40X, Olympus, Japan) as an objective. The diameter of the optical field for the modified FCBS is about 3.5 μm.
Bacterial Samples
The cell samples for this experiment were Salmonella typhimurium (S. typhimurium, ATCC14028), Escherichia coli (E. coli, ATCC25922), and S. aureus (S. aureus, ATCC6538). All bacterial samples were obtained from the Laboratory of Microbiology, University of Shanghai for Science and Technology. All cytological samples were free of sedimentation. The experiment was completed within 24 h at room temperature.
The bacteria were separated in the following manner: The three strains were cultured in a nutrient broth for 16 h, fixed with 1% methanol (v/v), and then made the bacteria smear. The procedure steps are as following: firstly, 200 μL of the bacteria were placed in the Eppendorf centrifuge tube and centrifuged for 5 min at 5,000 rpm. Secondly, the supernatant was removed. Thirdly, the precipitated part was resuspended with 1mL of deionized water to make the bacteria suspension. These procedures were repeated three times before the bacteria were finally resuspended in 500 μL of deionized water. After the clean oil-free slides were baked on an alcohol lamp, 20 μL of bacterial suspension were smeared on the prepared slide using a sterilized coating rod.
Spectral Collection and Pretreatment
We used a silicon slice whose reflectivity was about 30% in the visual and NIR range as the standard reflector for all bacteria backscattering spectral analyses to accurately obtain the true spectral characteristics of the measured samples. S. typhimurium, E. coli, and S. aureus were individually placed on the microscope slide, while the stage was manually adjusted to move along the surroundings so that the spot of convergence could be scanned in all directions. The microscopic information of the sample at this point was measured at the single bacterium level.
Analysis and Identification Method
In the spectrum range from 450 to 800 nm, the dimensionality of spectrum is too high, making analysis and automatic identification very difficult. Principal component analysis (PCA) method is a multivariate statistical analysis technique. The core idea is to compress and extract the data, compress the linearly dependent variables into a few linearly independent comprehensive variables. The new obtained comprehensive variables contain most of the information of original variables. The PCA method is used to reduce the dimensionality. The main characteristics of the data are extracted by data decorrelation [19]. Then the main information is included in the first few principal components (PCs). In the process of comprehensive analysis, the first few PCs with the largest variance are selected and can be used in preliminary treatment for other machine learning methods [20].
The basic idea of BPNN is to use the steepest descent method to obtain the constant adjustment of the network weight and threshold through back propagation, so as to obtain the smallest square error of network error. To identify and classify the spectra of three categories of pathogens, the samples of the test set are pre-processed by PCA. The principal component vectors of cumulative contributions over 85% are inputted into the BPNN model are extracted for prediction. We set the deviation of prediction results within ±0.15 as the correct prediction. Conversely, there are two types of misjudgments: [1] when the prediction value is not in the setting value range, the misjudgment is occurred. However it is not recognized as another type of bacteria; [2] when the prediction value is in another bacteria value range, it will be recognized as other bacteria. The number of trainings used is 5,000 and the target error is 0.01.
Experimental Results Under Neutral Culturing Conditions
In this experiment, the backscattering micro-spectra of S. typhimurium, E. coli, and S. aureus that were cultured under neutral condition, were measured using the modified FCBS.
Three Bacterial Spectra
The light scattering spectra of the three kinds of foodborne pathogens were obtained and demonstrated by using BWspec software. The experimental data for the backscattering micro-spectra of the three kinds of bacteria are shown in Figure 1.
In the spectra range of 450 to 800 nm, the contribution rate and cumulative contribution rate of variance for the first five principal components calculated by PCA are shown in Table 1. The cumulative contribution rate of the first two principal components (PC1 and PC2) reaches 99.39%, indicating that they already contain the primary information of the original variables.
A PCA scatter diagram based on the first two principal component scores, PC1 and PC2, is shown in Figure 2. Fifty spectral data for each of the three kinds of bacteria are used in the PCA. This result has demonstrated that the micro-spectra of S. typhimurium, E. coli, and S. aureus obtained using the modified FCBS can be distinguished by the first two principal components in the PCA.
Identification Results by BPNN
Spectral data of 150 samples were collected as prediction set samples, 1–50, 51–100, and 101–150 cases were S. typhimurium, E. coli, and S. aureus spectral data, respectively. The effective spectral components of the microscopic spectra of bacteria collected after the data dimension of spectra were reduced by PCA method. The first and second principal component vectors were selected as the input of the BPNN model for testing. The classification and identification results have been shown in Table 2 for 150 prediction sample sets. The experimental results have shown that the identification rate of all three bacteria is above 90%.
Experimental Results Under Different Culturing Conditions
During the actual detection process, the growth of foodborne pathogens will also change with the external environment. The use of back scattering spectroscopy for label-free classification and identification of foodborne pathogens is an ongoing research. To prove the ability of classification and identification of the modified FCBS, it is necessary to consider the backscattering of foodborne pathogens in on-site detection. The same three foodborne pathogens are selected. Most of the oils and fats are weakly alkaline, while most of the eggs and milk foods are weakly acidic. Therefore, two pH values (pH = 6.6, pH = 8.4) culturing condition are designed. The backscattering spectra of three foodborne pathogens are studied by spectroscopy combined with statistical methods, chemometric methods, and deep learning algorithms.
Spectra of Three Foodborne Pathogens Under Two pH Conditions
The experimental data of the backscattering micro-spectra of the three kinds of bacteria under two conditions of pH value are shown in Figure 3.
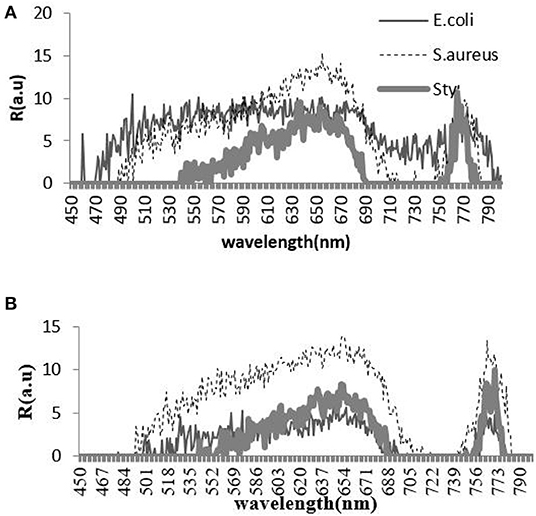
Figure 3. Backscattering micro-spectra of three kinds of bacteria under acidic condition (A) and alkaline condition (B).
The contribution rate and cumulative contribution rate of the variance of two pH value conditions for the first five principal components calculated by PCA are shown in Tables 3, 4. In the spectra range of 450 to 800 nm, the cumulative contribution of the first two principal components (PC1 and PC2) reaches 86.179 and 98.6%, respectively, indicating that they already contain the primary information of the original variables.
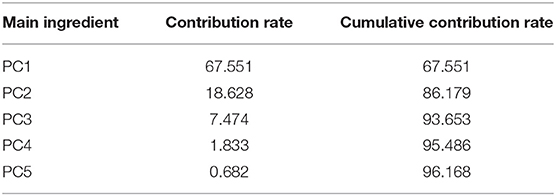
Table 3. Contribution rate and cumulative contribution rate of the first five principal components (acidic condition).
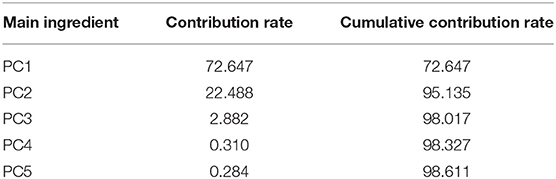
Table 4. Contribution rate and cumulative contribution rate of the first five principal components (alkaline condition).
Under two conditions of pH values, PCA plots based on the first two principal component scores, PC1 and PC2, are presented in Figures 4, 5, respectively. A total of 50 samples of spectral data for each of the three kinds of bacteria are used in the PCA. This result has demonstrated that under acidic and alkaline culturing conditions, the micro-spectra of S. typhimurium, E. coli, and S. aureus obtained using the modified FCBS can be distinguished by the first two principal components in the PCA.
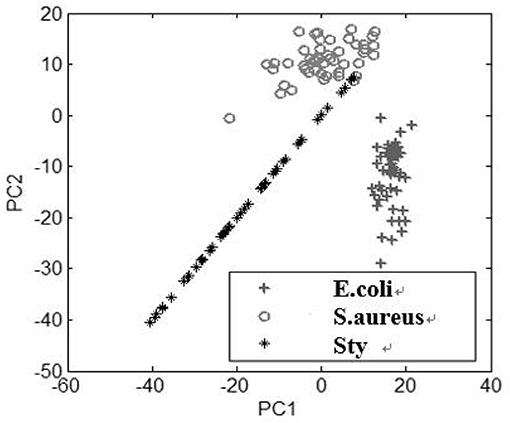
Figure 4. Discrimination of the spectra of the three kinds of bacteria under acidic condition by PCA.
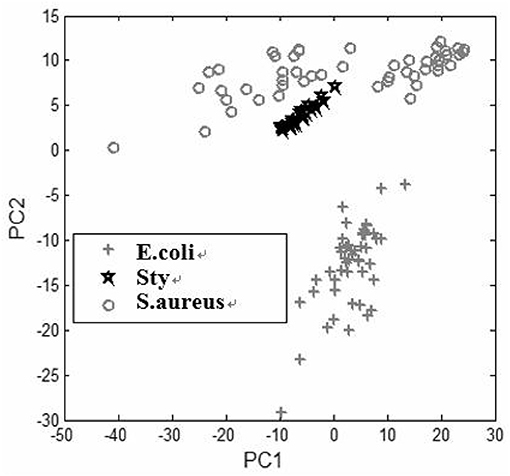
Figure 5. Discrimination of the spectra of the three kinds of bacteria under alkaline condition by PCA.
Identification Results by BPNN Under Two pH Conditions
Under each of two pH conditions, spectral data of 150 samples are collected as prediction set samples respectively. Same as under neutral culturing conditions, the samples of the test set are also pre-processed by PCA, while the first two principal component input BPNN model are extracted for testing. The classification and identification result have been shown in Tables 5, 6. The experimental results have shown that the identification rates of all three bacteria are above 94%.
Discussions and Conclusion
Food safety issues are associated with the national economy and people livelihood. Traditional techniques of bacterial identification are time-consuming and offer poor detection sensitivity. Our proposed test method here is noninvasive and label free. It can extract the spatial information of the bacterial cells into the spectral information and obtain the scattering characteristics of various bacterial cells without complicated pre-processing. By extracting the characteristic of the scattering spectrum, different cells can be identified and classified. Compared with the backscattering detection technology, such as the Raman spectrum, the elastic light scattering spectrum can better describe the cell morphological characteristics. Our method has a number of advantages, such as the simplicity of the system, good repeatability, and high precision of spectral signals. Moreover, the system is more conductive to the design and construction of portable detection equipment, thereby meeting the requirements of detection in-situ. Backscattering micro-spectral data from S. typhimurium, E. coli, and S. aureus are collected from three foodborne pathogens based on our modified FCBS. By utilizing PCA and BPNN algorithm, the three kinds of bacteria were classified and identified, with good results obtained. At the same time, the identification accuracy was improved, which provided a new method for cell identification. The results have shown that the identification accuracy of three categories of common foodborne pathogens under neutral, acidic and alkaline culturing conditions were all above 90%. In addition, it could be observed that the spectral curves of the same species of bacteria were significantly different under different culture conditions. In response to this phenomenon, we speculate that the morphological characteristics of bacteria changed under acidic and alkaline culturing conditions, compared with neutral culturing condition. Thus the elastic light scattering spectrum changed [21].
With a greater understanding of food microorganisms, we believe that additional foodborne pathogen analysis methods and detection techniques can be applied in real-life situations. In future, we will continue to optimize the identification algorithm and collect a larger number of samples to confirm our current data before establishing a database of bacterial feature information. In addition, miniaturization equipment can provide guarantee for in-situ rapid detection. However, considerable work remains to be done in the future for applying the discrimination of the bacteria based on the modified FCBS. Given the complicated effect of the variety of foodborne pathogens that usually exist in food, automatic classification of mixed bacteria and its application for clinical detection and treatment needs further research. Full detection of pathogenic bacteria in food cannot be accomplished using a single method and requires a combination of various techniques to enhance detection sensitivity and reduce detection time. With the rapid development of microbiology, biochemistry, molecular biology, and other disciplines, the development of various multifunctional microbial detection systems has become an important topic. Combining the optical technology with other detection techniques can achieve accurate and efficient detection of foodborne pathogens and requires further research.
Data Availability Statement
The raw data supporting the conclusions of this article will be made available by the authors, without undue reservation, to any qualified researcher.
Author Contributions
DZ and XW guided our work. CW and SZ designed the experiments. SL and QL provided the bacteria samples. MC and GZ improved the equipment. BL carried out experiments and analyzed the data. CW and BL wrote the manuscript.
Funding
This work was supported by grants from the National Natural Science Foundation of China Nos. 61775140 and 81771850, and the SJTU Medicine Engineering Interdisciplinary Research Fund No. YG2017MS19.
Conflict of Interest
The authors declare that the research was conducted in the absence of any commercial or financial relationships that could be construed as a potential conflict of interest.
Acknowledgments
We thank LetPub (www.letpub.com) for its linguistic assistance during the preparation of this manuscript.
References
1. Nyachuba DG. Foodborne illness: is it on the rise? Nutr Rev. (2010) 68:257–69. doi: 10.1111/j.1753-4887.2010.00286.x
2. Groundwater PW, Todd A, Worsley AJ, Anderson RJ. The technology and techniques used in the detection of pathogenic bacteria. Pharm J. (2009) 283:281–2. Available online at: https://www.pharmaceutical-journal.com/files/rps-pjonline/pdf/pj_20090912_detection.pdf
3. Li HM, Xu HY, Xiong YH. Research progress on the application of immunochromatographic test strip technology in foodborne pathogen detection. Food Sci. (2011) 32:380–3. doi: 10.1111/j.1759-6831.2010.00113.x
4. Lian YZ, Xue D, Yong L, Yue L, Jing A, Xin L. Study on measuring rapidly bacterium in water with ATP bioluminescence technique. Chin J Health Lab Technol. (2007) 17:1859–60.
5. Galikowska E, Kunikowska D, Tokarska-Pietrzak E, Dziadziuszko H, Loś JM, Golec P, et al. Specific detection of Salmonella enterica and Escherichia coli strains by using ELISA with bacteriophages as identification agents. Eur J Clin Microbiol Infect Dis. (2011) 30:1067–73. doi: 10.1007/s10096-011-1193-2
6. Zulkiply NA, Sjafri FR, Ismail A, Ismail A. An in-house multiplex PCR for detection of S. typhi and S. paratyphi A: a comparison between detection method by agarose gel electrophoresis and lateral flow assay. Int J Infect Dis. (2012) 16:395. doi: 10.1016/j.ijid.2012.05.526
7. Arbault P, Buecher V, Poumerol S, Sorin ML. Study of an ELISA method for the detection of E. coli O157 in food. Prog Biotechnol. (2000) 17:359–68. doi: 10.1016/S0921-0423(00)80093-3
8. Jo Y, Jung J, Kim MH, Park H, Kang SJ, Park Y. Label-free identification of individual bacteria using Fourier transform light scattering. Optics Exp. (2015) 23:15792–805. doi: 10.1364/OE.23.015792
9. Duan N, Chang B, Zhang H, Wang Z, Wu S. Salmonella typhimurium detection using a surface-enhanced raman scattering-based aptasensor. Int J Food Microbiol. (2016) 218:38–43. doi: 10.1016/j.ijfoodmicro.2015.11.006
10. Wang P, Pang S, Chen J, McLandsborough L, Nugen SR, Fan M, et al. Label-free mapping of single bacterial cells using surface-enhanced Raman spectroscopy. Analyst. (2016) 141:1356–62. doi: 10.1039/C5AN02175H
11. Pan W, Zha J, Chen Q. Classification of foodborne pathogens using near infrared (nir) laser scatter imaging system with multivariate calibration. Sci Rep. (2015) 5:9524. doi: 10.1038/srep09524
12. Wilson BK, Vigil GD. Automated bacterial identification by angle resolved dark-field imaging. Biomed Opt Exp. (2013) 4:1692. doi: 10.1364/BOE.4.001692
13. Wyatt PJ. Identification of bacteria by differential light scattering. Nature. (1969) 221:1257–8. doi: 10.1038/2211257a0
14. Li S, Hu P, Malmstadt N. Confocal imaging to quantify passive transport across biomimetic lipid membranes. Anal Chem. (2010) 82:7766–71. doi: 10.1021/ac1016826
15. Wang C, Guo XD, Fang B, Song C. Study of back-scattering micro-spectrum for stomach cells at single cell scale. J Biomed Opt. (2010) 15:040505. doi: 10.1117/1.3469782
16. Murugova TN, Solodovnikova IM, Yurkov V, Gordeliy VI, Kuklin AI, Ivankov O, et al. Potentials of small-angle neutron scattering for studies of the structure of “Live” mitochondria. Neutron News. (2011) 22:11–14. doi: 10.1080/10448632.2011.598800
17. Zhang Lu, Qin Y, Li KX, Zhao X, Xing YF, Zhao H, et al. Light scattering properties in spatial planes for label free cells with different internal structures. Opt Quantum Electr. (2015) 47:1005–25. doi: 10.1007/s11082-014-9957-4
18. Zhang Y, Yunxiang LI, Quan Q. Prediction model of PM_(2.5) based on attribute reduction and BP neural network. Environ Sci Technol. (2017) 40:341–6.
19. Wang C, Wen M, Bai L, Zhang T. Auto-classification for confocal back-scattering micro-spectrum at single-cell scale using principal component analysis. Optik. (2015) 127:1007–10. doi: 10.1016/j.ijleo.2015.10.066
20. Mao ZH, Zhang XX, Yue-Chao WU, Yin JH, Xia Y. Fourier transform infrared microscopic imaging and fisher discriminant analysis for identification of healthy and degenerated articular cartilage. Chin J Anal Chem. (2015) 43:518–22. doi: 10.1016/S1872-2040(15)60816-7
Keywords: foodborne pathogens, elastic scattering, scattering spectra, classification, principal component analysis
Citation: Wang C, Liu B, Li S, Liu Q, Chen M, Zheng G, Zhuang S, Zhang D and Wei X (2020) Rapid Classification of Single Bacterium Based on Backscattering Microscopic Spectrum—A Pilot Study. Front. Phys. 8:97. doi: 10.3389/fphy.2020.00097
Received: 09 November 2019; Accepted: 13 March 2020;
Published: 08 April 2020.
Edited by:
Chao Tian, University of Science and Technology of China, ChinaReviewed by:
Liwei Liu, Shenzhen University, ChinaDelong Zhang, Zhejiang University, China
Wei Gong, Zhejiang University, China
Copyright © 2020 Wang, Liu, Li, Liu, Chen, Zheng, Zhuang, Zhang and Wei. This is an open-access article distributed under the terms of the Creative Commons Attribution License (CC BY). The use, distribution or reproduction in other forums is permitted, provided the original author(s) and the copyright owner(s) are credited and that the original publication in this journal is cited, in accordance with accepted academic practice. No use, distribution or reproduction is permitted which does not comply with these terms.
*Correspondence: Dawei Zhang, dwzhang@usst.edu.cn; Xunbin Wei, xwei01@sjtu.edu.cn