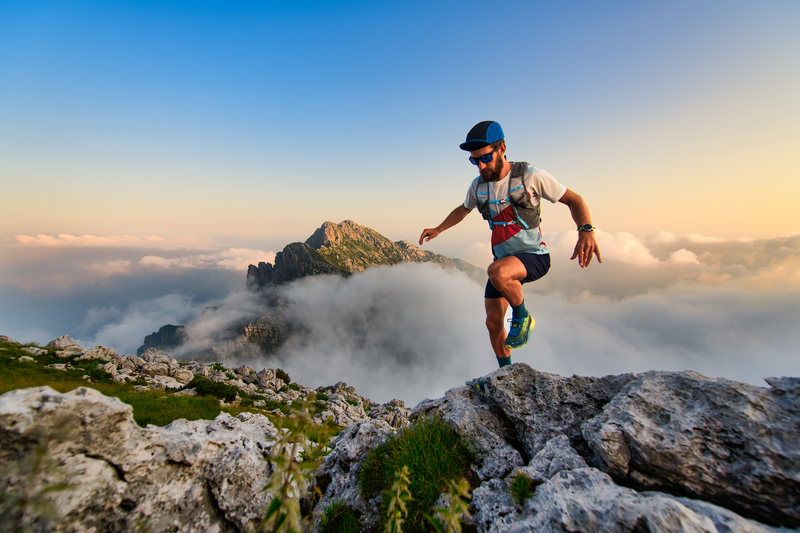
94% of researchers rate our articles as excellent or good
Learn more about the work of our research integrity team to safeguard the quality of each article we publish.
Find out more
ORIGINAL RESEARCH article
Front. Phys. , 11 March 2020
Sec. Social Physics
Volume 8 - 2020 | https://doi.org/10.3389/fphy.2020.00059
This article is part of the Research Topic Cooperation View all 6 articles
In evolutionary games, pair interactions are defined by payoff matrices that can be decomposed into four types of orthogonal elementary games that represent fundamentally different interaction situations. The four classes of elementary interactions are formed by games with self- and cross-dependent payoffs, coordination games, and cyclic games. At the level of two-person games, social traps (dilemmas) can not occur for symmetric payoff matrices, which are combinations of coordination games and symmetrically paired self- and cross-dependent components, because individual and common interests coincide in them. In spatial evolutionary games that follow the logit evolutionary dynamics, however, the total payoff is still not maximized at certain noise levels in certain combinations of symmetric components. This phenomenon is similar to the appearance of partially ordered phases in solid state physics, which are stabilized by their higher entropy. In contrast, it is the antisymmetric part of their self- and cross-dependent components that is responsible for the emergence of traditional social dilemmas in games like the two-strategy donation game or the prisoner's dilemma. The general features of these social dilemmas are inherited by n-strategy games in the absence of cyclic components, which would prevent the existence of a potential and thus thermodynamic behavior. Using the mathematical framework of matrix decomposition, we survey the ways in which the interplay of elementary games can lead to a loss of total payoff for a society of selfish players. We describe the general features of different illustrative combinations of elementary games, including a game in which the presence of a cyclic component gives rise to the tragedy of the commons via a paradoxical effect.
In evolutionary games [1–9], the players can represent particles, biological species, human individuals, or their communities. For realistic and simple systems, it is generally assumed that the players are located at the sites of a lattice or network and they interact with their neighbors. These pair interactions are described by payoff matrices adopted from traditional game theory [10–12], and players are assumed to be intelligent and selfish, meaning that they aim to maximize their own income irrespective of others. In evolutionary games, the players can change their own strategy in a way prescribed by a dynamical rule that depends on payoff differences. The stationary macroscopic behavior of these systems is determined by the pair interactions, the connectivity structure, and the evolutionary rule.
In this article, we deal with the possible behaviors of those n-strategy evolutionary games in which equivalent players are distributed on the sites of a square lattice and the evolution is controlled by the logit rule [13–18], a rule that resembles the dynamics used in kinetic Ising-type models in physics [19–23]. When their payoff matrix is symmetric, these evolutionary games can be mapped onto suitable physical systems, which exhibit different types of order–disorder transitions as the noise level of the logit rule is changed. More precisely, the probability distribution of the microscopic states of these systems is described by the Boltzmann distribution, with the negative potential energy replaced by a potential derived from the pair interactions. A symmetric payoff matrix provides equivalent payoffs to both players for any given strategy pair. In this case, individual and common interests coincide, therefore selfish behavior (which is always assumed in traditional game theory) does not reduce a player's income. The increase of noise in the decision process, however, decreases the average income and can lead to a phase transition to a macroscopic state with lower income. This transition can be interpreted as a social dilemma caused by entropy. We will show examples of this type of behavior in this article.
In the traditional analysis of two-player games, social dilemmas are represented by prisoner's dilemma [24] and donation [12, 25] games. In these games, it is the antisymmetric part of the 2 × 2 payoff matrix that is responsible for the appearance of the social dilemma: Selfishness dictates that players choose a strategy pair that is not optimal. Quantifying the strength of social dilemmas in multiagent systems is complicated by the fact that their appearance is influenced by a multitude of features, including the specifics of the interactions, the structure of the underlying connectivity network, the evolutionary rules, the initial state of the system, etc. Recent efforts [26–29] have focused on the payoff differences that incentivize unilateral deviations from the optimum. In this article, we reinvestigate these systems from a new viewpoint based on the decomposition of payoff matrices into a linear combination of four types of elementary interactions. In the next section, we briefly describe the mathematical details of the models in question. Subsequently, we review the decomposition approach and discuss the general features of elementary interactions with self- and cross-dependent payoffs, coordination between strategy pairs, and cyclic dominance, which prevents the existence of a potential and thus thermodynamic behavior. Due to the large number of possible combinations of elementary games, we will not attempt to give a complete overview, but instead discuss just a handful of interesting special cases which demonstrate some different versions of social dilemmas. Most of the present analyses are restricted to systems with players distributed on a square lattice whose strategy evolution is controlled by the logit rule, which randomly selects a player in each round, who then unilaterally changes her strategy according to a probability distribution that exponentially favors choices that provide higher individual incomes. It is known that the properties of the final stationary states of these evolutionary processes are different if the players are located at the sites of a network with fundamentally different connections among the players or if the evolutionary process is governed by (stochastic) imitation of a better performing neighbor [5, 30, 31] or other more complex dynamics that aim to model further aspects of human behavior [32, 33] like the response to incentives in the form of rewards and punishments [9, 34, 35], the heterogeneity of the players or their roles in different interactions [36–38], voluntary participation in the game [39, 40], the reputation of players [41, 42], or the mutability of interaction networks [43, 44], to name just a few that were studied recently in a similar framework. We will point out some of these differences.
We consider evolutionary games in which equivalent players are located at the sites of a square lattice (with periodic boundary conditions). The players each use one of n pure strategies represented by the n-dimensional Cartesian unit vectors and play the same game against their z = 4 nearest neighbors. The strategy of the player at site x is denoted by sx. The payoff Ux of player x comes from multiple games with her neighbors and can be expressed as
where the sum runs over the z neighbors located at the sites x+δx. The Aij (i, j = 1, ⋯ , n) element of the payoff matrix A defines the income of player x if she chooses the i-th strategy and her coplayer uses the j-th strategy.
The time evolution of the models treated in this article is governed by the logit rule [13–18]: All players play the same game repeatedly using the same strategy against all of their neighboring opponents. Between each round of play, a single player x is chosen at random, who then changes her strategy from sx to any one of the n available strategies drawn according to the probability distribution
while the strategies of the other players remain unchanged. The parameter K is called the noise parameter, because it quantifies how well players react to their surroundings. In the low-noise, K → 0 limit, the logit distribution concentrates on the best responses of the player that provide the highest possible payoff given the strategy profile of her set of coplayers. In the opposite, high-noise, K → ∞ limit, the players can no longer make an informed decision, and all n available strategies are chosen with equal probability. In between these two extreme limits, strategy choices promising higher payoffs are favored exponentially, and K acts as an attenuation coefficient.
One of the important properties of the logit strategy update rule is that under certain circumstances it drives systems toward the Boltzmann distribution. More precisely, the game model outlined above defines an irreducible Markov chain if K ≠ 0, which converges to a unique stationary equilibrium distribution regardless of its initial state. For so-called potential games this steady state is given by a Boltzmann distribution. In potential games, the payoff differences realized by unilateral strategy changes can be described by a single function for all players, that is, there exists a single function U of the strategy profile s = {sx} of the whole system for which
is always satisfied whenever for all y ≠ x in the strategy profiles s′ and s. U is called the potential of the game. The Boltzmann distribution
is in detailed balance with the logit update rate of Equation (2), that is, for unilateral strategy changes in opposing directions, which ensures the stationarity of p(s).
In our multiagent lattice game models, the whole game only has a potential if its constituent pair interactions have a potential, and the potential of the whole game is built up from contributions from each player–player interaction as
where V is the potential matrix that defines the potential of the pair interaction game defined by the payoff matrix A. The linear decomposition approach, which we discuss in detail in the next section, provides a simple set of rules for the evaluation of the potential of any two-player matrix game.
In the systems we consider, the uniform pair interaction is defined by the matrix A. On the analogy of vectors, this matrix can be built up as a linear combination
where the “Cartesian type” matrices (containing a single 1) can be considered as a set of orthogonal basis matrices which indicate the coordinate axes of the n2-dimensional parameter space. In this notation the matrices A and B are orthogonal if
By rotating the coordinate axes, we can choose another parametrization for the quantification of pair interactions. Previous studies have justified the usefulness of basis matrices that are defined by the dyadic products of n-dimensional basis vectors which include the all-one vector [45]. The dyadic product of the all-one vector with itself is the all-one matrix, which represents an irrelevant (constant) term that provides the same payoff to the players regardless of their strategy choices. The elements of the component of A in this direction are given by
the average of the possible payoffs.
Beside this irrelevant component, we distinguish four other orthogonal elementary interaction types so that
The self- (A(se)) and cross-dependent (A(cr)) components contain uniform elements in their rows and columns, respectively, and can be parameterized as
and
where the expansion coefficients are
The sums of these coefficients satisfy the conditions
which in turn ensure the orthogonality of the irrelevant, self-dependent, cross-dependent components:
The remaining components are separated according to their symmetry. The coordination component is the symmetric, A(co) = A(co)T and the cyclic component is the anti-symmetric, A(cyc) = −A(cyc)T part of the remainder of A. These two terms are evidently orthogonal to each other. A(co) is called the coordination component of the game, because it can be built up from voluntary pair coordination games, that is,
These elementary components contain two +1s in their diagonal and two -1s outside of their diagonal in such a way that the sum of the matrix elements is zero in each row and column. In other words, A(co) summarizes all possible elementary coordinations between possible strategy pairs (i, j) (i < j) with weight factors νij. When a coefficient is negative (νij < 0), its contribution describes an anticoordination game between the two corresponding strategies.
The cyclic component A(cyc) also gets its name from its building blocks. It can be written as
where each term of the sum is a voluntary cyclic dominance (rock–paper–scissors) game. The expansion coefficient λ1ij (1 < i < j ≤ n) is the weight of the contribution of the elementary cyclic game in which strategy 1 beats strategy i, strategy i beats strategy j, and strategy j beats strategy 1 when the coefficient is positive. The entries of an elementary cyclic matrix are three +1s and three −1s distributed in such a way that none of them are along the diagonal of the matrix, two-two of each type are in the first row and the first column of the matrix, and the entries sum up to 0 in each row and each column of the matrix.
One of the advantages of the matrix decomposition presented here is that it readily identifies potential games. A matrix game only admits a potential in the absence of the cyclic component, that is, when A(cyc) = 0. Then, its potential matrix can be given using the simple expression [23]
Whether an evolutionary game manifests a tragedy of the community depends on the payoff matrix, the connectivity structure, and the stochastic dynamical rules. Due to the large number of parameters, we restrict our current analysis to discussing a handful of simple examples in which the concept of matrix decomposition can extend our understanding of the nature of social dilemmas.
The two-strategy donation game represents the simplest linear combination of self- and cross-dependent elementary games. Many versions of the game also include an irrelevant term A(av) [12]. The players have two options: to pay (strategy 1) or not to pay (strategy 2) the cost c > 0 of an investment which provides a benefit b > c to the other player regardless of her choice of strategy. The corresponding payoff matrix is
whose non-zero expansion coefficients are μ = (b − c)/2, ε1 = −ε2 = −c/2, and γ1 = −γ2 = b/2. The game's potential matrix can be written as
This game has a single pure Nash equilibrium, the strategy pair (2, 2), selected by the maximal entry of the potential matrix max(Vij) = V22, so traditional game theory tells players not to invest. This poses a social dilemma, since both players would realize a higher payoff if they both chose to invest. In the square-lattice, logit-rule-driven setup described in section 2, however, the average payoff of the players varies monotonously from 0 to as the noise parameter K is increased from 0 to ∞. So the presence of noise increases the total payoff of the community, thus alleviating, but not completely eliminating, the social dilemma.
These results can be directly derived from the logit rule Equation (2) by exploiting the structural properties of self- and cross-dependent payoff matrices. Because the irrelevant and cross-dependent parts of a player's payoff only depend on the strategy choices of her opponents, they have the same contribution to the arguments of all exponentials in Equation (2), so the fraction can be simplified, and as a result the logit rate is independent of irrelevant and cross-dependent payoffs. This means that the donation game's strategy update rates are determined only by its self-dependent component. Since the payoff a player receives in a self-dependent game depends only on her own strategy choice and not on her opponent's, the probability of any player switching to strategy i is
in every microstate of the whole system if this game is played with z coplayers. It follows that this is also the equilibrium probability of any randomly chosen player using strategy i. The same reasoning can be applied to any general n-strategy game made up of only irrelevant, cross-, and self-dependent payoffs on any z-regular lattice. In other words, the frequency of players following strategy i is ϱi = w(i). These games do not describe proper player–player interactions, the players do not meaningfully affect each other's payoff, they each play their own independent game against an external entity, which has a role in the game that is similar to the role an external magnetic field has in magnetic systems.
General n-strategy games composed only of self- and cross-dependent components can be considered as generalized donation games in which εi defines the investment choosing the i-th strategy entails while γj is the benefit a player receives for each of her coplayers who selected the j-th strategy. Because of the zero-sum constraints on the self- and cross-dependent parameters (Equation 13), some of the εi are positive and represent divestments, and some of the γj are negative and represent penalties. Adding an irrelevant term on top of these self- and cross-dependent components does not change the preferences of the players, it just shifts everyone's payoff by the same constant on a regular lattice, so we will assume μ = 0 without any loss of generality. The entries of the generalized donation game's potential matrix are Vij = εi + εj. If the game has a unique maximum self-dependent coefficient εi = maxεj—which should almost always be the case when n − 1 of the coefficients are independently chosen random numbers—, then the maximum entry of the potential matrix is Vii and the game has a unique, pure, and strict Nash equilibrium, the symmetric strategy pair (i, i). This obviously poses no social dilemma, if the payoff matrix itself happens to be symmetric, that is, if εj = γj for ∀j. The condition for the generalized donation game to be a social dilemma is at least one of the other (j, j) (j ≠ i) symmetric strategy pairs providing a higher payoff than the Nash equilibrium. This can be easily achieved by tuning the γj parameters. In fact, for a fixed set of εj, one can always find a set of γj that makes the Nash equilibrium have a lower payoff than that of all other symmetric strategy pairs. The fact that whether a game presents a social dilemma depends on the game's cross-dependent components may seem surprising, because the cross-dependent components do not contribute to the payoff changes realized by unilateral strategy changes, which can be used to measure the strength of a dilemma [26–29]. Changing the cross-dependent components can change the identity of the highest paying strategy profile, the reference point of the dilemma, instead of its incentives.
The multiagent, evolutionary version of the game provides an average payoff of
on a regular lattice when the players update their strategies according to the logit rule, which is definitely lower than the highest possible per capita payoff maxz(εj + γj) for any finite K. The introduction of noise always induces a social dilemma, since it precludes the maintenance of specific strategy arrangements. What is more interesting is that there are homogeneous states (all players playing the same strategy) that have a lower payoff z(εj + γj) than 〈U〉. If the Nash equilibrium is one of those states, then the presence of noise actually alleviates the social dilemma instead of causing it. Since the ρj equilibrium frequencies only depend on the εj self-dependent components, the average payoff 〈U〉 is simply the sum of the average payoff of the γj = 0, fully self-dependent game and the expectation value of the additional cross-dependent payoffs.
The above feature of cross-dependent components can be utilized when we wish to explore the average payoff as a function of the payoff parameters in evolutionary games controlled by the logit rule. Namely, if one modifies only the cross-dependent components (), then this change leaves the stationary state unchanged, while the variation of the average payoff can be given as,
Adding additional cross-dependent components to a game has no effect on the logit evolutionary process. Consequently, the resulting game has the same steady states as the original. In the donation game treated in section 4.1, this property allows us to create or eliminate certain social dilemmas simply by tuning cross-dependent components. In this section, we examine the effects of adding cross-dependent components to two-strategy potential games with both self-dependent and coordination components. The payoff matrix of a general two-strategy game can be written as
in terms of the decomposition coefficients described in section 2. In the following, we consider logit-rule-driven games on square lattices.
The two-strategy coordination game (ε1 = ε2 = γ1 = γ2 = 0 and ν12 = 1 for the sake of simplicity) is equivalent to the zero-field Ising model [46, 47], one of the simplest models of ferromagnetic materials in statistical physics, when the players update their strategies according to the logit rule. The Ising model exhibits a continuous phase transition between a disordered phase stable at high temperatures and an ordered phase stable at low temperatures. The system's behavior near the transition point at is universal in the sense that it describes numerous seemingly different two-dimensional systems [48]. The transition is illustrated by the solid lines in Figure 1, which show the temperature dependence of the equilibrium frequencies of the two strategies. In the disordered phase, strategy 1 and strategy 2 are present in equal proportions. In the ordered phase, this balance is broken spontaneously, and one of the strategies becomes more frequent than the other. The difference between the frequencies keeps increasing as the temperature is lowered, and the system becomes homogeneous in the K → 0 limit. Because of the symmetry of the payoff matrix, either one of the strategies may become the majority strategy with equal probability if the system's initial state is randomly chosen.
Figure 1. Strategy frequencies vs. K in two-strategy potential games played on a square lattice for three different self-dependent components and ν12 = 1. The solid lines show Onsager's exact results [49] in the ε1 = ε2 = 0 case. The upper (lower) dashed and dotted lines show the frequency of the favored strategy 1 (suppressed strategy 2) for ε1 = −ε2 = 0.001 and ε1 = −ε2 = 0.03.
The introduction of a cross-dependent component (γ1 = −γ2 ≠ 0) changes only one thing about the system. The average payoffs are no longer equal to each other in the two differently ordered phases. The difference between the two average payoffs is proportional to the order parameter |ϱ1 − ϱ2| by Equation (22) and γ1 = −γ2. More precisely, it is equal to z|γ1(ϱ1 − ϱ2)|. Each average payoff is shifted by an amount that goes to z times the majority strategy's γi coefficient in the K → 0 limit, as demonstrated by the data shown in Figure 2. This constitutes a kind of social dilemma. Sometimes, depending on the initial conditions and how the stochastic evolution of the system turns out, the average payoff of the system will be lower than it could be in the other, just as stable state. This is a qualitatively different social dilemma than the one in the donation game (section 4.1), because the two states it compares are both feasible stationary states of the system's dynamics. We also find that the average payoff remains unchanged in the disordered phase, because half of the players provide γ1 and the other half provide γ2 = −γ1 payoff to each of their neighbors. The appearance of the social dilemma accompanies the phase transition.
Figure 2. Average payoffs as a function of K in the evolutionary games shown in Figure 1 with additional cross-dependent components (γ1 = −γ2 = 0.5 and −0.5, the former leading to higher and the latter leading to lower average payoffs). The solid, dashed, and dotted lines refer to the same self-dependent parameter values as in Figure 1.
Adding a self-dependent component to the elementary coordination game (ν12 = 1 and ε1 = −ε2 ≠ 0) smooths out the order–disorder transition, as demonstrated by the Monte Carlo data plotted with dashed and dotted lines in Figure 1. The self-dependent components break more than just the payoff symmetry of the two strategies, they affect their symmetry with respect to the dynamics as well. The system no longer has two equivalent stable states at low temperatures. The one in which the strategy favored by the self-dependent components is in the minority loses its stability, while the other remains stable. Of course, the changes in the dynamics also modify the temperature-dependence of the equilibrium strategy frequencies, increasing the difference between the two strategy frequencies at all temperatures.
Cross-dependent payoff components can again be used to shift the average payoff without affecting the system's steady state. Figure 2 shows some examples. We again find that the more polarized the strategy distribution is, the more effectively its average payoff can be changed. The highest possible average payoff in this system is maxz(ν12 + εi + γi), but this is obviously not realized when K > 0, which constitutes a social dilemma. How changing the temperature affects this social dilemma depends on the payoff parameters, just like in the case of the generalized donation game. In the K → 0 limit, the stationary state is dominated by the strategy for which the diagonal element of the potential matrix ν12 + 2εj is maximal. Should this coincide with the maximum payoff strategy pair, the social dilemma becomes less and less severe as the noise level is decreased and it disappears for K = 0. In the opposite case, increasing the noise level actually shrinks the amount of total payoff the whole community misses out on due to the dynamics.
Remarkably, anticoordination games (games with ν12 < 0) have almost exactly the same properties as their coordinated counterparts when the network structure of the pair interactions is bipartite. The sites of bipartite lattices can be divided into two disjoint groups in such a way that no neighboring sites end up in the same group. For example, the square lattice is a bipartite lattice, whose two parts are arranged in a checkerboard pattern. This property allows us to map anticoordination games onto coordination games by simply exchanging the labels of the strategies on one of the two sublattices, which formally swaps the two columns of the payoff matrix. Under this transformation, the coordination component's sign is flipped, the cross-dependent components are swapped, and the self-dependent components remain unchanged, turning the ν12 < 0, ε1, ε2, γ1, γ2 anticoordination game into the coordination game. We are already familiar with this latter game's properties, so we just have to apply the (inverse) strategy swap transformation to those results to learn about anticoordination games. In a nutshell, an anticoordination game system is disordered at high temperatures and sublattice-ordered at low temperatures, and it undergoes a continuous phase transition when ε1 = ε2 = 0. The cross-dependent components do not affect the system's dynamics, but they do change the payoffs, which may lead the community into a social trap. When the game is composed of overlapping coordination components that share one of their coordinated strategies or when the lattice is not bipartite (or frustrated, in the language of condensed matter physics), this label swapping trick cannot be applied successfully, and finding the zero-noise Nash equilibrium and predicting the properties of the system can become a much harder task [50].
The phenomena described in this section are not specific to two-strategy games. The three-strategy Potts model [51, 52], a generalization of the Ising model, displays very similar behavior. The payoff matrix that corresponds to the three-strategy Potts model is the permutation symmetric combination of all three-strategy elementary coordination games. Normalizing the highest payoff to 1, the expansion coefficients are ν12 = ν13 = ν23 = 1/2 and the payoff matrix reads
When this game is played by players who are located at the sites of a square lattice and whose strategy updates follow the logit rule, the resulting system exhibits a continuous order–disorder phase transition at a critical noise level of . This is demonstrated by the Monte Carlo simulation data presented in Figure 3. One of the differences between the models is that the Potts model has three equivalent stationary states instead of two, reflecting the permutation symmetry of the three Potts strategies. The effects of adding cross-dependent components to the Potts model are very similar to those observed in the case of the Ising model, as illustrated by Figure 4. The cross-dependent components again shift the average payoffs of the otherwise equivalent steady states in the ordered phase, which poses a social dilemma, this time with two possible social trap situations that may be realized instead of the optimal outcome.
Figure 3. Strategy frequencies vs. K in one of the three equivalent stationary states of the three-strategy Potts model defined by Equation (24).
Figure 4. Average payoffs as a function of K in the three possible low-noise steady states of the Potts model defined by Equation (24) with additional cross-dependent components (γ1 = −γ3 = 0.5, γ2 = 0). The labels above the curves refer to the majority strategy.
If we further perturb this model with self-dependent components, the ordered stationary state whose majority state has the highest self-dependent component (εi = maxεj > 0) will be selected by the logit dynamics at low noises independently of the cross-dependent components. If the highest εi and the highest εj + γj both belong to the same strategy, this selection constitutes no social dilemma in the K → 0 limit. In any other case, however, the system will evolve toward one of the two tragedy of the commons situations, whose K-dependent average payoff approaches one of the two lower curves in Figure 4 in the εi → 0 limit. The most dangerous, lowest-paying social traps have an interesting property: Increasing the noise level results in some increase in their average payoff in the vicinity of the critical transition point, as illustrated by Figure 2 for the two-strategy system and curve 3 in Figure 4 for the three-strategy system.
In light of the results above, we can predict that the n-strategy game composed of n permutation symmetric coordination components, which is equivalent to the n-state Potts model, has similar features. We expect that in this game one can distinguish (n − 1) social traps in which the homogeneous state favored by the self-dependent components does not provide the highest income because of the game's cross-dependent terms.
It is also worth briefly mentioning that for stochastic imitation-type dynamical rules [1, 30] the evolution can avoid the social traps in a certain region of the parameter space. According to this sequential strategy update rule, a randomly selected player (at site x) adopts its neighbor's strategy (at site x + δ) with a probability [31] that depends on the payoff differences as
which prefers the adoption of strategies with higher payoffs. Just like in the case of the logit rule, the K parameter quantifies the level of noise here too.
Imitation favors the formation of a homogeneous absorbing state, while it also increases the frequency of symmetric strategy pairs (neighboring players using the same strategy) that provide higher payoffs, which takes into account the contribution of cross-dependent components, which are ignored by the logit rule. The systematic investigation [53] of two-strategy games revealed an additional phenomenon caused by the interplay of elementary components that can be observed along interfaces separating homogeneous domains. The direction of invasion was found to be dependent on the orientation of the interface at low noise levels. Monte Carlo simulations have clearly demonstrated (for details and snapshots see Szabó et al. [53]) that if the minority strategy forms a circular domain in the initial state, its shape first transforms into a square with horizontal and vertical or tilted sides (with slopes of ±1), then this distorted domain shrinks and, finally, vanishes. In Figure 5, solid lines show the time-dependence of the average payoff at low noise levels as the system evolves into two different absorbing (homogeneous) states. The selected final homogeneous state depends on the initial strategy distribution. For large linear sizes, L → ∞, the above mechanism favors the homogeneous state that percolates first. The noise causes irregularities along the interfaces and diminishes the anisotropy of the invasion velocity for high values of K. As a result, high-noise stochastic imitation drives the system toward the homogeneous absorbing state with the higher cross-dependent component, as demonstrated by the dashed lines in Figure 5. We emphasize that this is again a process in which increasing the noise level helps the system avoid the social trap.
Figure 5. Evolution of average payoffs in a two-strategy game governed by stochastic imitation when the MC simulations are started from an initial state containing a circular island (as shown in the inserts on the left) of radius r = 90 on a square lattice of linear size L = 400. The final homogeneous states are shown in the inserts on the right. (Parameters: ν12 = 1, ε1 = −ε2 = −0.03, γ1 = −γ2 = 0.5). The solid (dashed) lines show MC data averaged over 100 runs for K = 0.3 (K = 3).
Different combinations of elementary coordination games may lead to even more kinds of social dilemmas. One such example is the model treated in Király and Szabó [54], in which the logit dynamics prefers a steady state characterized by a lower average payoff over another metastable state because of its higher entropy. The model's payoff matrix combines Ising- and Potts-type combinations of elementary coordinations in an n = 5-strategy game to read
The game contains the two-strategy Ising and the three-strategy Potts coordinations as independent subgames of the first two and last three strategies, respectively, and provides zero payoff to both players when they choose strategies that do not belong to the same subgame. It has five Nash equilibria, the five symmetric strategy pairings, which all provide a payoff of 1 to both players. In the following, we give a brief overview of the results presented in Király and Szabó [54].
When this game is played on a square lattice by players who update their strategies according to the logit rule, the resulting system's equilibrium properties are qualitatively similar to those of the Ising model. The system undergoes a continuous phase transition at a critical temperature (Kc ≈ 1.067) between a disordered state and an ordered state, in which the symmetry of the two Ising-coordinated strategies is spontaneously broken. The disordered phase respects the symmetry of both the first two and the last three strategies, but the Ising-coordinated strategies are slightly more frequent than the other three. In the ordered phase one of the two Ising-coordinated strategies has the highest frequency. Not too close to the critical point, the other Ising-coordinated strategy is the least frequent in the system. The three Potts-coordinated strategies are present in equal abundance at all noise levels.
The game also has a metastable state, which is never preferred by the system at finite noise levels, but can nonetheless be observed at low enough noise levels when Monte Carlo simulations are started from a pre-ordered initial state. This metastable state breaks the symmetry of the Potts-coordinated strategies instead of that of the Ising-coordinated strategies, one of the last three strategies has the highest frequency in it, while the other two are equally abundant and less frequent than the two also equally abundant Ising-coordinated strategies.
The social dilemma in this game lies in the fact that the Potts-majority metastable state provides a higher average payoff to the community than the stable Ising-majority state preferred by the dynamics. In potential games that are governed by the logit strategy update rule, the stable equilibrium state maximizes the system's so called free energy. Metastable states maximize this free energy only locally in the sense that only small enough perturbations of the state lower the free energy. The free energy F is the sum of two quantities, the system's potential U and its entropy S multiplied by the noise level K, that is, F = U + TS. The two terms act in opposite directions, as the potential favors ordered strategy arrangements, whereas the entropy term prefers disordered states. Comparing the two terms for the Ising-majority stable state and the Potts-majority metastable state, we find that the average potential—which in games composed only of coordination components equals the average payoff—is higher in the metastable state, so the stable state is a social trap, and conversely, the entropic term is higher in the stable state, so the more disordered of the two competing states is stabilized by its higher entropy, and it is this stabilization effect that maintains the social dilemma.
The presence of cyclic components prevents the existence of a potential and thus can significantly modify many related features. The evolutionary three-strategy rock–paper–scissors game has been investigated in many previous studies, because this interaction plays a fundamental role in the maintenance of biodiversity [5, 8, 11, 55–57]. If two players play this game repeatedly with unilateral strategy changes, then the loser can always become the winner, and this possibility drives a circular flow in their strategy choices.
The original rock–paper–scissors game has a single mixed Nash equilibrium, in which the three options are selected with equal (1/3) probability. For imitation-based population dynamics, the equation of motion has four fixed points including the symmetric Nash equilibrium (ϱi = 1/3) and the three homogeneous states. Additionally, this system has a set of oscillating solutions too. On d-dimensional lattices (d > 1) these evolutionary games show local cyclic oscillations around the symmetric solution, while global oscillations can also be observed on networks with sufficiently large neighbor degrees (for references, see the reviews mentioned above).
Combinations of several voluntary rock–paper–scissors components exhibit more complex behaviors including the formation and competition of strategy associations [58], parity effects caused by feedback along the interaction cycle [59], and the enhancement of the role of fluctuations in spatial systems [60, 61]. Even a brief survey of the wide scale of possible behaviors goes beyond the scope of this work. Instead of it, our attention will be focused on a lattice model in which the community falls into a social trap due to the paradoxical effect described by Tainaka [62]. This paradoxical effect can be observed in three-state systems with non-uniform invasion velocities. Contrary to the naive expectation, having the highest invasion velocity actually benefits the predator of the most aggressive state (species) in both the mean-field and the spatial model [63–65].
This curious effect can also be recognized in many three-strategy models, as illustrated by Figure 6, by considering a model in which the invasion rate of strategy 1 is increased by the introduction of a suitable self-dependent component.
Figure 6. Average payoffs as a function of K for a logit-rule controlled model on a square lattice whose pair interaction is defined by Equation (27) when ε = 0.05 and λ = 0.1. The dashed line corresponds to the highest possible average payoff, which is achieved when all players choose strategy 1. The vertical arrow shows the noise level at which the dominance of strategy 3 turns sharply into the dominance of strategy 1.
In this model, the expansion coefficients of the three elementary coordination components are identical, just as they are in the Potts model. Additionally, the pair interaction includes a self-dependent term that provides extra income to strategy 1, and a cyclic (rock–paper–scissors) component so that
For weak perturbations (|ε|, |λ| ≪ 1), this game has three homogeneous Nash equilibria, in which the players select the same strategy. In lattice systems, combining the three-state Potts model with the rock–paper–scissors game results in a self-organizing pattern in which rotating spiral arm interfaces separate homogeneous domains of the three strategies, whose formation is supported by the coordination component. Along these moving interfaces, the domains of strategy 1 invade the territory of strategy 2, which in turn invades domains of strategy 3, which invades domains of strategy 1. In the zero-noise limit the average domain size (correlation length) is proportional to 1/|λ| [66].
In this model, positive ε values enhance the invasion velocity from domains of strategy 1 to domains of strategy 2. Monte Carlo simulations [67] have indicated that the paradoxical effect [62] governs the system's behavior as long as ε remains below a threshold value, which depends on K and λ. In this case, strategy 3 (the predator of strategy 1) dominates the system at low noises. At the same time, this state is unstable. More precisely, if a sufficiently large domain of strategy 2 emerges due to the stochasticity of the evolution, then this nucleus grows until the appearance of a similar nucleus of its mortal enemy (strategy 1) that stops and reverses the growth process and, finally, eliminates the domain of strategy 2, as illustrated by Figure 7. In the absence of food, strategy 1 becomes extinct. In short, after this burst event, the system evolves back into the state dominated by strategy 3. These bursts become rarer and larger when K is decreased. Increasing K modifies the invasion velocities, which causes relevant changes in the spatio-temporal patterns of the system's evolution, as well as in the average payoff shown in Figure 6. Due to these changes, the increase of K leads to the dominance of the preferred strategy 1 via a sharp transition at a noise level of K≃0.85. This process is accompanied by a sharp increase in the average payoff that is reduced by mistakes whose frequency increases with K.
Figure 7. The appearance, growth, and extinction of a burst is accompanied by a macroscopically detectable variation in the frequency of strategy 2. These MC data were obtained in a large system (L = 1, 000) at K = 0.44 for the same interaction parameters that were given in the caption of Figure 6.
The above model is again an example of a complex phenomenon that develops a local maximum in the K-dependence of the system's average payoff. Evidently, this peak in the average payoff can be increased by modifying the payoff matrix with suitable cross-dependent components (see Equation 22).
In the present work, we have surveyed several mechanisms that drive evolutionary games into the trap (or traps) of social dilemmas. We have demonstrated that the concept of matrix decomposition allows us to study systematically and separately the interactions and processes that are responsible for the appearance of a wide scale of social dilemmas. In this approach, we distinguish four types of elementary n-strategy pair interactions: cross-dependent, self-dependent, coordination-type, and cyclic dominance games. Out of these four types, only cyclic dominance games do not admit a potential. Potential games, combinations of the other three elementary game types, are closely connected to statistical physics. When a potential game's evolution follows the logit strategy update rule, it becomes equivalent to a classical spin model. In this analogy, it is the negative of the game's potential, and not its payoff, that plays the role of the spin system's Hamiltonian. Due to this analogy, the ground state corresponds to the preferred Nash equilibrium that maximizes the system's potential. The corresponding ordered strategy distribution determines the system's behavior at low noise levels.
We have shown that the system's behavior can be described analytically if the pair interactions contain only self- and cross-dependent components. This set of games can be considered as a generalized version of the donation game, in which players have n different investment options, each with its own benefit to the coplayer. Since the cross-dependent component does not contribute to the potential, the system's dynamics and equilibrium steady state are determined just by the self-dependent component, which can lead to a social dilemma, which is either exacerbated or alleviated by decreasing the noise level depending on the payoff parameters.
Similar social traps that are also caused by a cross-dependent mismatch between a game's potential and payoff may arise in general potential games too. However, when a game has multiple equivalent steady states as a result of some non-trivial symmetry in its potential matrix, the presence of cross-dependent components can also cause a different kind of social dilemma. In this case, there is a mismatch between the potential and the payoff of equally feasible steady states of the (logit) dynamics, so whether the community falls into a social trap is determined by the initial state of the system and the actual outcome of its stochastic evolution. Additionally, we have pointed out similar phenomena in a system with an imitation-based evolutionary rule, in which K-dependent interfacial invasion processes can drive the system into a social trap.
The appearance of social dilemmas is intimately related to the antisymmetric components of the payoff matrix that represent individual but not common interests. In two-strategy games such antisymmetric components can only arise from the self- and cross-dependent terms. In n-strategy games (n > 2), however, the presence of cyclic components can also lead the system into a social trap, as we have demonstrated in the case of a three-strategy model. Cyclic components do not directly affect the average payoff, because they are zero-sum games. We have discussed a model in which the emergence of social tragedy is related to a paradoxical effect observed in many models characterized by sufficiently strong cyclic dominance. In these systems, K-dependent interfacial phenomena play a relevant role in the generation of burst events and also in the formation of self-organizing patterns.
In some of the above-mentioned social dilemma types, one can observe a local maximum in the noise-dependence of the average payoff that defines an optimal noise level at which the community's losses are minimized. In some models, these phenomena have a significant influence on the formation and motion of interfaces.
Finally, we emphasize that the systematic analysis of the combinations of coordination components is still not complete, despite the fact that these interactions can occur in, and thus are relevant to, many multi-particle systems to which the traditional methods of statistical physics can be easily applied. This is also true for the combinations of cyclic components that play a fundamental role in the behavior of ecological systems. In the light of the above results, we expect further curious phenomena to emerge from the systematic investigation of the interplay between the wide range of elementary interactions.
The datasets generated for this study are available on request to the corresponding author.
All authors listed have made a substantial, direct and intellectual contribution to the work, and approved it for publication.
This work was supported by the Hungarian National Research Fund (OTKA TK-120785).
The authors declare that the research was conducted in the absence of any commercial or financial relationships that could be construed as a potential conflict of interest.
1. Maynard Smith J. Evolution and the Theory of Games. Cambridge: Cambridge University Press (1982).
2. Hofbauer J, Sigmund K. The Theory of Evolution and Dynamical Systems. Cambridge: Cambridge University Press (1988).
5. Szabó G, Fáth G. Evolutionary games on graphs. Phys Rep. (2007) 446:97–216. doi: 10.1016/j.physrep.2007.04.004
6. Roca CP, Cuesta JA, Sánchez A. Evolutionary game theory: temporal and spatial effects beyond replicator dynamics. Phys Life Rev. (2009) 6:208–49. doi: 10.1016/j.plrev.2009.08.001
7. Sandholm WH. Population Games and Evolutionary Dynamics. Cambridge, MA: MIT University Press (2010).
8. Frey E. Evolutionary game theory: theoretical concepts and applications to microbial communities. Physica A (2010) 389:4265–98. doi: 10.1016/j.physa.2010.02.047
9. Perc M, Jordan JJ, Rand DG, Wang Z, Boccaletti S, Szolnoki A. Statistical physics of human cooperation. Phys Rep. (2017) 687:1–51. doi: 10.1016/j.physrep.2017.05.004
10. von Neumann J, Morgenstern O. Theory of Games and Economic Behaviour. Princeton, NJ: Princeton University Press (1944).
11. Hofbauer J, Sigmund K. Evolutionary Games and Population Dynamics. Cambridge: Cambridge University Press (1998).
13. Brown GW. Iterative solution of games by fictious play. In: Koopmans TC, editor. Activity Analysis of Production and Allocation. New York, NY: Wiley (1951). p. 373–6.
14. Blume LE. The statistical mechanics of strategic interactions. Games Econ Behav. (1993) 5:387–424. doi: 10.1006/game.1993.1023
15. Blume LE. The statistical-mechanics of best-response strategy revision. Games Econ Behav. (1995) 11:111–45. doi: 10.1006/game.1995.1046
16. Monderer D, Shapley LS. Fictious play property for games with identical interests. J Econ Theory. (1996) 68:258–65. doi: 10.1006/jeth.1996.0014
17. Monderer D, Shapley LS. Potential games. Games Econ Behav. (1996) 14:124–43. doi: 10.1006/game.1996.0044
18. Cramer JS. Logit Models From Economics and Other Fields. Cambridge, MA: Cambridge University Press (2003). doi: 10.1017/CBO9780511615412
19. Herz AVM. Collective phenomena in spatially extended evolutionary games. J Theor Biol. (1994) 169:65–87. doi: 10.1006/jtbi.1994.1130
20. Bouchaud JP. Crisis and collective socio-economic phenomena: simple models and challenges. J Stat Phys. (2013) 151:567–606. doi: 10.1007/s10955-012-0687-3
21. Krause SM, Bornholdt S. Spin models as microfoundation of macroscopic market models. Physica A. (2013) 392:4048–54. doi: 10.1016/j.physa.2013.04.044
22. Sornette D. Physics and financial economics (1776-2014): puzzles, Ising and agent-based models. Rep Prog Phys. (2014) 77:062001. doi: 10.1088/0034-4885/77/6/062001
23. Szabó G, Borsos I. Evolutionary potential games on lattices. Phys Rep. (2016) 624:1–60. doi: 10.1016/j.physrep.2016.02.006
25. Dawes RM. Social dilemmas. Ann Rev Psychol. (1980) 31:169–93. doi: 10.1146/annurev.ps.31.020180.001125
26. Tanimoto J, Sagara H. A study on emergence of alternating reciprocity in a 2 × 2 game with 2-length memory strategy. Biosystems. (2007) 90:728–37. doi: 10.1016/j.biosystems.2007.03.001
27. Wang Z, Kokubo S, Jusup M, Tanimoto J. Universal scaling for the dilemma strength in evolutionary games. Phys Life Rev. (2015) 14:1–30. doi: 10.1016/j.plrev.2015.04.033
28. Wang Z, Kokubo S, Jusup M, Tanimoto J. Dilemma strength as a framework for advancing evolutionary game theory: Reply to comments on “universal scaling for the dilemma strength in evolutionary games”. Phys Life Rev. (2015) 14:56–8. doi: 10.1016/j.plrev.2015.07.012
29. Ito H, Tanimoto J. Scaling the phase-planes of social dilemma strengths shows game-class changes in the five rles governing the evolution of cooperation. R Soc Open Sci. (2018) 5:181085. doi: 10.1098/rsos.181085
30. Nowak MA, May RM. Evolutionary games and spatial chaos. Nature. (1992) 359:826–9. doi: 10.1038/359826a0
31. Szabó G, Tőke C. Evolutionary prisoner's dilemma game on a square lattice. Phys Rev E (1998) 58:69–73. doi: 10.1103/PhysRevE.58.69
32. Nowak MA. Five rules for the evolution of cooperation. Science. (2006) 314:1560–3. doi: 10.1126/science.1133755
33. Capraro V, Perc M. Challenges in social physics: in pursuit of moral behavior. Front Phys. (2018) 6:107. doi: 10.3389/fphy.2018.00107
34. Sigmund K, Hauert C, Nowak MA. Reward and punishment. Proc Natl Acad Sci USA. (2001) 98:10757–62. doi: 10.1073/pnas.161155698
35. Li X, Wang H, Xia C, Perc M. Effects of reciprocal rewarding on the evolution of cooperation in voluntary social dilemmas. Front Phys. (2019) 7:125. doi: 10.3389/fphy.2019.00125
36. Szabó G, Szolnoki A. Cooperation in spatial prisoner's dilemma with two types of players for increasing number of neighbors. Phys Rev E. (2009) 79:016106. doi: 10.1103/PhysRevE.79.016106
37. Fort H. A minimal model for the evolution of cooperation through evolving heterogeneous games. EPL (2008) 81:48008. doi: 10.1209/0295-5075/81/48008
38. Capraro V, Perc M, Vilone D. The evolution of lying in well-mixed populations. J R Soc Interf. (2019) 16:20190211. doi: 10.1098/rsif.2019.0211
39. Szabó G, Hauert C. Evolutionary prisoner's dilemma games with voluntary participation. Phys Rev E. (2002) 66:062903. doi: 10.1103/PhysRevE.66.062903
40. Xia CY, Meloni S, Perc M, Moreno Y. Dynamic instability of cooperation due to diverse activity patterns in evolutionary social dilemmas. EPL. (2015) 109:58002. doi: 10.1209/0295-5075/109/58002
41. Droz M, Szwabinski J, Szabó G. Motion of influential players can support cooperation in prisoner's dilemma. Eur Phys J B. (2009) 71:579–85. doi: 10.1140/epjb/e2009-00160-1
42. Chen MH, Wang L, Sun SW, Wang J, Xia CY. Evolution of cooperation in the spatial public goods game with adaptive reputation assortment. Phys Lett A. (2016) 380:40–7. doi: 10.1016/j.physleta.2015.09.047
43. Pacheco JM, Traulsen A, Nowak MA. Coevolution of strategy and structure in complex networks with dynamical linking. Phys Rev Lett. (2006) 97:258103. doi: 10.1103/PhysRevLett.97.258103
44. Perc M, Szolnoki A. Coevolutionary games – a mini review. BioSystems. (2010) 99:109–25. doi: 10.1016/j.biosystems.2009.10.003
45. Szabó G, Szolnoki A. Congestion phenomena caused by matching pennies in evolutionary games. Phys Rev E. (2015) 91:032110. doi: 10.1103/PhysRevE.91.032110
46. Ising E. Beitrag zur Theorie des Ferromagnetismus. Z Physik. (1925) 31:253–8. doi: 10.1007/BF02980577
47. Domb C. Ising model. In: Domb C, Green MS, editor. Phase Transitions and Critical Phenomena, Vol. 3. London: Academic Press (1974). p. 357–484.
48. Stanley HE. Introduction to Phase Transitions and Critical Phenomena. Oxford: Clarendon Press (1971).
49. Onsager L. Crystal statistics. I. A two-dimensional model with an order-disorder transition. Phys Rev. (1944) 65:117–49. doi: 10.1103/PhysRev.65.117
50. Anglès d'Auriac JC, Preissmann M, Sebő A. Optimal cuts in graphs and statistical mechanics. Mathl Comput Modell. (1997) 26:1–11. doi: 10.1016/S0895-7177(97)00195-7
51. Potts RB. Some generalized order-disorder transitions. Math Proc Camb Phil Soc. (1952) 48:106–9. doi: 10.1017/S0305004100027419
53. Szabó G, Varga L, Szabó M. Anisotropic invasion and its consequences in two-strategy evolutionary games on a square lattice. Phys Rev E. (2016) 94:052314. doi: 10.1103/PhysRevE.94.052314
54. Király B, Szabó G. Entropy affects the competition of ordered phases. Entropy. (2018) 20:115. doi: 10.3390/e20020115
55. May RM, Leonard WJ. Nonlinear aspects of competition between three species. SIAM J Appl Math. (1975) 29:243–53. doi: 10.1137/0129022
56. Szolnoki A, Mobilia M, Jyian LL, Szczesny B, Rucklidge AM, Perc M. Cyclic dominance in evolutionary games: a review. J R Soc Interface. (2014) 11:20140735. doi: 10.1098/rsif.2014.0735
57. Baker R, Pleimling M. The effect of habitats and fitness on species coexistence in systems with cyclic dominance. J Theor Biol. (2020) 486:48110084. doi: 10.1016/j.jtbi.2019.110084
58. Szabó G, Czárán T. Defensive alliances in spatial models of cyclical population interactions. Phys Rev E. (2001) 64:042902. doi: 10.1103/PhysRevE.64.042902
59. Kobayashi K, Tainaka K. Critical phenomena in cyclic ecosystems: parity law and selfstructuring extinction pattern. J Phys Soc Jpn. (1997) 66:38–41. doi: 10.1143/JPSJ.66.38
60. Kang Y, Pan Q, Wang X, He M. A golden point rule in rock–paper–scissors–lizard–spock game. Physica A. (2013) 392:2652–59. doi: 10.1016/j.physa.2012.10.011
61. Vukov J, Szolnoki A, Szabó G. Diverging fluctuations in a spatial five-species cyclic dominance game. Phys Rev E. (2013) 88:022123. doi: 10.1103/PhysRevE.88.022123
62. Tainaka K. Paradoxical effect in a three-candidate voter model. Phys Lett A. (1993) 176:303–6. doi: 10.1016/0375-9601(93)90923-N
63. Frean M, Abraham ED. Rock-scissors-paper and the survival of the weakest. Proc R Soc Lond B. (2001) 268:1323–7. doi: 10.1098/rspb.2001.1670
64. Hauert C, De Monte S, Hofbauer J, Sigmund K. Replicator dynamics in optional public goods games. J Theor Biol. (2002) 218:187–94. doi: 10.1006/jtbi.2002.3067
65. Hauert C, De Monte S, Hofbauer J, Sigmund K. Volunteering as Red Queen mechanism for cooperation in public goods game. Science (2002) 296:1129–32. doi: 10.1126/science.1070582
66. Szolnoki A, Szabó G, Ravasz M. Three-state Potts model in combination with the rock-scissors-paper game. Phys Rev E. (2005) 71:027102. doi: 10.1103/PhysRevE.71.027102
Keywords: evolutionary games, potential games, elementary games, social dilemmas, lattice models
Citation: Király B and Szabó G (2020) Interplay of Elementary Interactions Causing Social Traps in Evolutionary Games. Front. Phys. 8:59. doi: 10.3389/fphy.2020.00059
Received: 29 November 2019; Accepted: 26 February 2020;
Published: 11 March 2020.
Edited by:
Valerio Capraro, Middlesex University, United KingdomReviewed by:
Jun Tanimoto, Kyushu University, JapanCopyright © 2020 Király and Szabó. This is an open-access article distributed under the terms of the Creative Commons Attribution License (CC BY). The use, distribution or reproduction in other forums is permitted, provided the original author(s) and the copyright owner(s) are credited and that the original publication in this journal is cited, in accordance with accepted academic practice. No use, distribution or reproduction is permitted which does not comply with these terms.
*Correspondence: György Szabó, c3phYm9AbWZhLmtma2kuaHU=
Disclaimer: All claims expressed in this article are solely those of the authors and do not necessarily represent those of their affiliated organizations, or those of the publisher, the editors and the reviewers. Any product that may be evaluated in this article or claim that may be made by its manufacturer is not guaranteed or endorsed by the publisher.
Research integrity at Frontiers
Learn more about the work of our research integrity team to safeguard the quality of each article we publish.