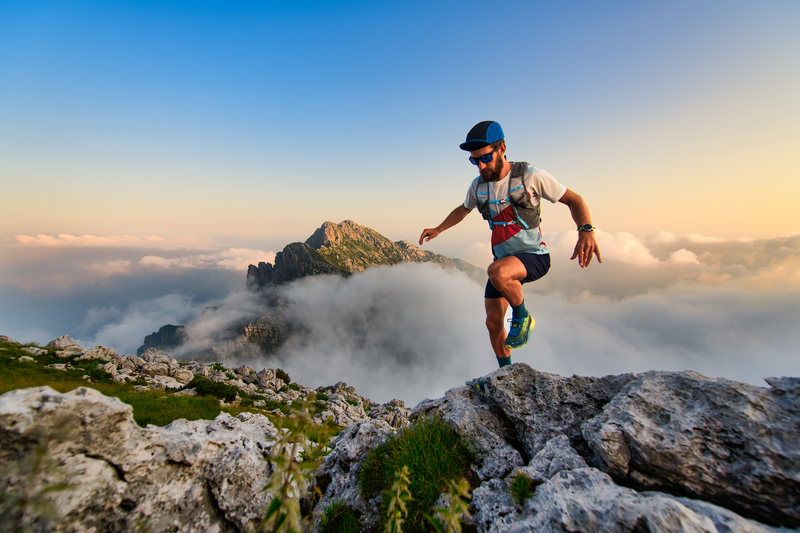
95% of researchers rate our articles as excellent or good
Learn more about the work of our research integrity team to safeguard the quality of each article we publish.
Find out more
MINI REVIEW article
Front. Phys. , 21 February 2020
Sec. Interdisciplinary Physics
Volume 8 - 2020 | https://doi.org/10.3389/fphy.2020.00035
This article is part of the Research Topic Complexity and Self-Organization View all 11 articles
In a recent round table organized by the Santa Fe Institute, the complexity of commerce captured the attention of those interested in understanding how complex systems science can be applicable for settings where consumers and providers interact. Despite the usefulness of applied complexity for commerce-related phenomena, few works have attempted to provide insightful ideas. This mini-review aims at providing a succinct discussion of how the metrics of emergence, self-organization, and complexity might benefit the research agenda of applied complexity and commerce/consumer studies. In particular, the paper argues possible pragmatic ways to understanding the valuable information present in word-of-mouth data found on electronic commerce platforms.
Emergence, self-organization, and complexity are three fundamental concepts in complex systems science [1, 2]. Nonetheless, the application of these concepts to the understanding of human behavior in the realm of commerce/consumer studies is far from being well-understood. In fact, on September 12, 2019, the Santa Fe Institute organized a discussion of this topic (https://wiki.santafe.edu/index.php/Complexity_of_Commerce_Agenda). As an extension of this matter, a well-deserved exposition would consist of providing a discussion on how the metrics of emergence, self-organization, and complexity [3] might benefit the research agenda of applied complexity and commerce/consumer studies. A warning note, however, should be stated beforehand. As commerce/consumer research is wide enough to be considered in one single paper, this circumstance demands the choice of a particular phenomenon. Accordingly, the remaining of this paper focuses on the consumers' “electronic word-of-mouth” (EWOM). Word-of-mouth [4] takes place when customers produce informal communications directed at other consumers about the ownership, usage, or characteristics of particular goods and services. When these communications are produced and shared through social media or electronic platforms, they are also known as “electronic word-of-mouth” [5].
Although the analysis of EWOM through statistical techniques is well-known in behavioral sciences [6, 7], the application of concepts coming from the framework of applied complexity is less frequent in the literature, being the works of Reingen and Kernan [8] and Jun et al. [9] two remarkable exceptions. Mathematical modeling or computerized simulations are also available from sociophysics [10, 11] by analyzing synthetic data.
A related yet different approach is the conceptual discussion provided here, which elaborates upon the idea of collecting natural EWOM data, preprocessing, and transform it as network data to calculate the emergence, self-organization, and complexity of its network structure. To achieve this goal, the organization of this mini-review is as follows. The idea of EWOM as a case study for applied complexity is present in the next section which also illustrates the computational steps to follow for collecting and preprocessing EWOM data and transform it as network data. Such illustration is not an analytical coverage. Also in section 2, is present the formalization of emergence, self-organization, and complexity, by summarizing the ideas of previous works [3, 12]. Section 3, then, enumerates possible benefits and challenges for applied researchers. In section 4, the paper closes presenting possible research questions that could be used for guiding empirical studies focusing on EWOM from the perspective of applied complexity.
From a data science perspective [13], EWOM data are not intrinsically structured, and it demands the application of natural language processing and text mining techniques [14, 15] to structure them following principles of tidy data [16]. The utility of tidying up this data lies in the possibility to leverage information mechanics (e.g., production, storage, and transmission) to gain insights into essential phenomena, such as customer engagement in online reviews [17], or quantifying the effect of online consumer reviews on new product sales [18].
In online food delivery platforms [19], it might be interesting, for example, to know possible differences among customers' experiences when consuming products of globalized fast-food chains. Because the preparation of each product follows a standardized industrial procedure in each of these globalized restaurants, several research projects can be conducted. One of these projects, for example, could be the empirical validation of agent-based models focusing on word-of-mouth dynamics with information seeking [20]. In projects of this sort, it might be revealing the description of how the dynamics of customers' positive word-of-mouth differ from the dynamics of negative word-of-mouth. With web scraping techniques for collecting real data from different globalized platforms, the possibility to characterize complaints vs. recommendations, and the estimation of customers' cultural customs when they recommend outstanding products, are certainly two other fruitful ventures. If applied researchers wish to turn their attention to customers' word-of-mouth semantics, the use of text-network analyses [21], based on principles of social network analysis [22, 23] might provide exciting answers. Working with these topics might be fruitful for those who acknowledge the imperfect nature of real-world data and yet wish to use it for theoretical development. Arguably, a brief description of how to collect and preprocess EWOM data might be illustrative for applied researchers.
The use of web scraping techniques [24] is a convenient means for collecting EWOM data from online food delivery platforms. Web scraping refers to the process of extracting data from websites automatically. The specifics on how web scraping works are beyond the scope of this paper, but the preprocessing of EWOM data deserves some mention. By its nature, EWOM data is not structured, but a convenient way to structure it is to transform customers' comments into a document-term matrix [15], whose entries show the frequency of appearance of every single word in each comment (i.e., words are arranged as rows, while comments are arranged as columns). As the number of comments generally exceeds the number of unique words that customers use for expressing their experiences, the resulting dimensionality of this matrix makes it equivalent to an incidence matrix [22]. This document-term matrix can then be re-expressed as a similarity matrix whose entries show the Jaccard index that quantifies the similarity between every word-comment unit [25]. The calculation of the Jaccard index here allows appreciating subtle semantic differences in customers' comments (e.g., a strong recommendation without hesitation on any aspect of the service vs. a recommendation accompanied by a warning regarding food variety). The knowledge of these semantic differences proves to be important for estimating the number of states for EWOM data. As these states are related to the concepts of emergence, self-organization, and complexity, it is convenient to describe them.
In a recent paper, Santamaría-Bonfil et al. [3] summarized both the discrete and continuous measures of emergence (E), self-organization (S), and complexity (C) which are applicable to any dataset or probability distributions [12], and rely on Shannon's information theory, as pioneered by the Santa Fe Institute [1]. A few ideas about the implications of using these concepts for analyzing EWOM data are necessary at this point. The first idea posits that EWOM is a dynamic property of an open system composed of customers and sellers that interact by using an electronic platform. The second idea states the possibility of analyzing EWOM at different scales. While from a microscopic scale, one would see a series of written characters (i.e., letters, emojis, words) with a particular frequency distribution, from a macroscopic scale, one would see a set of possible semantic states (i.e., complaint, recommendation, or suggestion). As these semantic states are not trivially detectable at a microscopic level, the coordinated production of written characters allows the emergence of new behaviors (e.g, satisfied vs. unsatisfied customers, and successful vs. non-successful restaurants in the online food delivery platform). This idea is compatible with that of emergence [12] that refers to properties of a phenomenon which are present at one scale (e.g., a satisfied client) and are not at another scale (e.g., the words written by a client). According to Santamaría-Bonfil et al. [3], the concept of emergence (E) for discrete probability distribution measures the average ratio of uncertainty a process produces by new information that is a consequence of changes dynamics or scale. For continuous distributions, the interpretation of E is constrained to the average uncertainty a process produces under a specific set of statistical parameters, such as the standard deviation in a normal distribution. The discrete and continuous versions of E are defined as
Equation (1) defines discrete E, where pi = P(X = x) is the probability of element i. Equation (2) defines continuous E, where XΔ corresponds to discretized version of X, and Δ is the integration step, and K is a normalizing constant that constrains E in the range [0 ≤ E ≤ 1] and is estimated as
where b corresponds to the number of bins of a probability mass function, or, in the continuous case, to the states that satisfies P(xi) > 0 (i.e., recommendations, complaints, or suggestions). In addition, log2(b) represents the maximum entropy for a distribution function with alphabet size of b (i.e., the number of characters used by customers when writing their comments). Thus, E can be deemed as the ratio between the entropy for given empirical distribution H(X), and the maximum entropy for the same alphabet size H(U). Now, let's turn the attention to self-organization. According to Fernández et al. [12] self-organization (S) is related to an increase in order or a reduction of entropy. Put it differently, as emergence supposes an increase of information, S should be anti-correlated with E, and this is formally expressed as
The numerical result of Equation (4) is also in the range [0 ≤ S ≤ 1]. With this final result, we can now realize the notion of complexity. Here, complexity represents a balance between change and regularity, allowing EWOM to adapt to contextual contingencies (e.g., showing dynamic changes as a function of the service quality of food providers or the increasing competitiveness among restaurants). While the regularity ensures the survival of information (e.g., a systematic positive opinion), change leads to the exploration of new possibilities (e.g., the emergence of recommendations for new products or services); that is, complexity describes the behavior of a system as the average uncertainty produced by emergent and regular global patterns as described by its probability distribution [3], which is formally expressed as follows:
In Equation (5), C is maximal when E equals S, and the highest value of C is achieved when one (or just a few) of the states is highly probable. C becomes zero when all of the states share the same probability of occurrence. The pragmatic interpretation of these metrics derive from a perspective called “the world as evolving information” [26]. An essential ingredient of this perspective posits the benefits of describing energy, matter, life and cognition in terms of information. These benefits neither deny the utility of physics for describing physical phenomena, nor chemistry for chemical events, nor biology for life-related facts. Nonetheless, this perspective is meant only for the cases when the approaches of physics, chemistry, or biology are not sufficient for comprising phenomena with manifestations at different scales. The eight tentative laws of information proposed by Gershenson [26] are useful for understanding the benefits of employing the concepts of emergence, self-organization, and complexity for EWOM research.
The recognition that EWOM is an emergent dynamic property of an open system composed of customers and sellers that interact by using an electronic platform is admittedly compatible with the idea that it changes as time goes by; i.e., the law of information transformation as proposed in the world as evolving information. As any customer can perceive (i.e., read) the information provided by other customers regarding their experiences in dealing with a particular seller, this sort of customer-to-customer interaction is also compatible with the law of information propagation. If, for example, the seller-to-customer interaction preserves itself in terms of a systematic presence of customers' complaints (i.e., one of the probable semantic states), this circumstance opens the possibility for the electronic platform to penalizing the seller (e.g., when Amazon automatically returns the money paid by the customer after reporting any irregularity with the quality of the product shipped by the seller). In this last case, the so-called law of requisite complexity would be taking place, resulting from the platform and the seller. The ability of a seller to generate the best service possible so as to create a critical balance between a stable positive EWOM with a rather minimum amount of negative EWOM, would be deemed as the law of information criticality. If we accept the idea that EWOM is a powerful online information source that influences online shopping [27], then we can realize that this information is having a certain control over its environment, which conforms to the law of information organization. The law of information self-organization stating that information tends to its preferred, most probable state also has an implication for EWOM studies. Because customers engage in the so-called “collaborative consumption” [19], the publication of opinions aiming at persuading other's decisions will create the possibility of a shared and dominant opinion regarding seller's conduct. This fact also relates to the law of information potentiality, according to which a customer can give different potential meanings to information. Finally, the law of information perception implies that the perception of customers might be generalized so as to respond to novel information. Even though the precise situation and context are always unique, this creates some sort of uncertainty, and this is intrinsically related to Shannon's entropy, as explained by Fernández et al. [12]. This last concept permits me to enumerate some challenges for researchers who acknowledge the narrowness in the scope, lenses, and epistemology of their discipline [28].
The first challenge for researchers with little or no knowledge of applied complexity is the idea that all economic agents (i.e., consumers, sellers, and platforms) can be seen as interacting components of a dynamic system. As agents can be seen as systems too, a second challenge is the use of social network analysis [22] to understand the relationships of economic agents from a systemic viewpoint. Few works have followed this orientation without using the concepts of emergence, self-organization, and complexity [29]. For example, Henderson et al. [30] showed several empirical examples of consumer associative networks to mapping an extensive array of branding effects, including branded features, driver brands, complements, co-branding, cannibalization, brand parity, brand dilution, brand confusion, counter-brands, and segmentation. The idea of consumer associative networks proved to be essential for the so-called “goal systems theory” proposed by Kopetz et al. [31]. This theory posits that the study of the goal-action interaction, taking place in a cognitive and motivational processes of the consumer, might be revealing for understanding a set of consumer-related phenomena including product variety search, impulsive buying, preferences, choices, and regret. Rocha and Holme [32] showed another applied perspective when they studied the network organization of consumer complaints. Although the orientation of these works might be the standard for scientific associations, such as the complex systems society, my own impression is that they remain widely ignored by members of other applied-oriented associations, such as the society for consumer psychology, or the association for consumer research.
The ideas mentioned above call for the development of interdisciplinary perspectives that demand the search for novel insights. For example, the concept of “antifragility” [33] might be fruitful to explain why some products become best-sellers even after receiving a bunch of negative reviews. The search for novel insights also demands the use of other tools for collecting and analyzing EWOM data. It is beyond the scope of this mini-review to provide a thorough description of these tools, but they include the use of agent-based modeling and simulation [20], web scraping, natural language processing, text mining and network analysis [34]. As these techniques are easily implemented in object-oriented programming languages, applied researchers might regard strategic their learning. After all, these programming languages offer other benefits, such as reproducibility; allowing others to follow the computational procedures that allow them to get the same results reported in a publication [35], or scalability; employing technologies capable of collecting and analyzing massive amounts of data [36]. The goals of scientific projects, such as FutureICT [37] that promote the use of the power of information to explore social and economic life, certainly call for multidisciplinary collaboration. All that is needed is the proposal of empirical studies where commerce/consumer studies and applied complexity can meet. EWOM research from an applied complexity perspective might be deemed as one of the several cases aligned with these goals.
Until this point, it should be clear how applied complexity can provide several contributions to the study of EWOM research. While the concept of consumer associative network is useful for understanding EWOM data from a psychological viewpoint [30, 31], the concepts of emergence, self-organization, and complexity have not been integrated. This integration might be better understood with an example. Figure 1 shows two consumer associative networks resulting from the procedures described in section 2.1. Although both of these figures reveal the network structure of EWOM data for two different brands of pizzas, the network on the left shows a different structure of the network on the right.
Figure 1. Two consumer associative networks resulting from text-network analysis. (A) Shows a hypothesized network of customers' comments for brand A. (B) Shows a hypothesized network of customers' comments for brand B.
With the calculus of E, S, and C, commerce/consumer researchers end up with a set of proxies to the degree of customers' comments diversification, customers' comments polarization, and the diversification-polarization balance, respectively. Because the network structure of EWOM data might change as time goes by, then a dynamic analysis of these changes might help commerce/consumer researchers understand the (external) factors that act upon these structures (e.g., How effective are promotions to increase and maintain the number of positive comments?). The comparison between these structures is another issue to explore (e.g., How similar are the network structures of EWOM data for two restaurants of a globalized fast-food chain operating in different countries?). Finally, the power of emergence, self-organization, and complexity for predicting future sales could be another related topic (How sensitive are sales to significant changes in the network structure of EWOM data?). These topics are relevant when we consider the case of Uber Eats, Just-Eat, Food Panda, or Delivery Hero, as business models that facilitate the interaction between customers and restaurants [19]. Working with EWOM data collected from these globalized platforms turns out to be an empirical field with unknown opportunities for complex systems scientists. The reason behind this statement is the gap between theory and observation. In network analysis, for example, idealized-mathematical illustrations make use of networks with few nodes and edges, but what would happen if we need to work with vast data sets of comments for a numerous collection of food providers? How much scalability would be required for analyzing a disproportionate set of data? These questions posit important challenges for developers of cloud computing technologies, such as Data bricks or Google Cloud.
JC conceived and wrote the paper.
This research was funded by Fundación Universitaria Konrad Lorenz under research grant number 9IN11191.
The author declares that the research was conducted in the absence of any commercial or financial relationships that could be construed as a potential conflict of interest.
1. Prokopenko M, Boschetti F, Ryan AJ. An information-theoretic primer on complexity, self-organization, and emergence. Complexity. (2009) 15:11–28. doi: 10.1002/cplx.20249
2. Polani D, Prokopenko M, Yaeger LS. Information and self-organization of behavior. Adv Complex Syst. (2013) 16:1303001. doi: 10.1142/S021952591303001X
3. Santamaría-Bonfil G, Gershenson C, Fernández N. A package for measuring emergence, self-organization, and complexity based on Shannon entropy. Front Robot AI. (2017) 4:1–12. doi: 10.3389/frobt.2017.00010
4. Berger J. Word of mouth and interpersonal communication: a review and directions for future research. J Consum Psychol. (2014) 24:586–607. doi: 10.1016/j.jcps.2014.05.002
5. Chen M, Chen J, Xue W. Research on the influence mechanism of eWOM on selection of tourist destinations—the intermediary role of psychological contract. In: Xu J, Ahmed SE, Cooke FL, Duca G, editors. Proceedings of the Thirteenth International Conference on Management Science and Engineering Management. Cham: Springer International Publishing (2019). p. 654–67.
6. Godes D, Mayzlin D. Using online conversations to study word-of-mouth communication. Market Sci. (2004) 23:545–60. doi: 10.1287/mksc.1040.0071
7. Chevalier JA, Mayzlin D. The effect of word of mouth on sales: online book reviews. J Market Res. (2006) 43:345–54. doi: 10.1509/jmkr.43.3.345
8. Reingen PH, Kernan JB. Analysis of referral networks in marketing: methods and illustration. J Market Res. (1986) 23:370–8. doi: 10.1177/002224378602300407
9. Jun T, Kim JY, Kim BJ, Choi MY. Consumer referral in a small world network. Soc Netw. (2006) 28:232–46. doi: 10.1016/j.socnet.2005.07.001
10. Chakrabarti AS, Sinha S. “Hits” emerge through self-organized coordination in collective response of free agents. Phys Rev E. (2016) 94:042302. doi: 10.1103/PhysRevE.94.042302
11. Ishii A, Kawahata Y. Sociophysics analysis of the dynamics of peoples' interests in society. Front Phys. (2018) 6:89. doi: 10.3389/fphy.2018.00089
12. Fernández N, Maldonado C, Gershenson C. Information measures of complexity, emergence, self-organization, homeostasis, and autopoiesis. In: Prokopenko M, editor. Guided Self-Organization: Inception. Vol. 9. Berlin; Heidelberg: Springer (2014). p. 19–51.
13. Wickham H, Grolemund G. R for Data Science: Import, Tidy, Transform, Visualize, and Model Data. Boston, MA: O'Reilly Media, Inc. (2017).
14. Silge J, Robinson D. Tidytext: text mining and analysis using tidy data principles in R. J Open Source Softw. (2016) 1:37. doi: 10.21105/joss.00037
15. Benoit K, Watanabe K, Wang H, Nulty P, Obeng A, Müller S, et al. Quanteda: an R package for the quantitative analysis of textual data. J Open Source Softw. (2018) 3:774. doi: 10.21105/joss.00774
17. Thakur R. Customer engagement and online reviews. J Retail Consum Serv. (2018) 41:48–59. doi: 10.1016/j.jretconser.2017.11.002
18. Cui G, Lui HK, Guo X. The effect of online consumer reviews on new product sales. Int J Electron Commerce. (2012) 17:39–58. doi: 10.2753/JEC1086-4415170102
19. Correa JC, Garzón W, Brooker P, Sakarkar G, Carranza SA, Yunado L, et al. Evaluation of collaborative consumption of food delivery services through web mining techniques. J Retail Consum Serv. (2019) 46:45–50. doi: 10.1016/j.jretconser.2018.05.002
20. Thiriot S. Word-of-mouth dynamics with information seeking: information is not (only) epidemics. Phys A. (2018) 492:418–30. doi: 10.1016/j.physa.2017.09.056
21. Choi Y, Kweon SH. A semantic network analysis of the newspaper articles on big data. J Cybercommun Acad Soc. (2014) 31:241–86.
22. Wasserman S, Faust K. Social Network Analysis: Methods and Applications. Cambridge: Cambridge University Press (1994).
24. Munzert S, Rubba C, Meißner P, Nyhuis D. Automated Data Collection With R: A Practical Guide to Web Scraping and Text Mining. New York, NY: John Wiley & Sons (2014).
25. Sun J, Tang J. A survey of models and algorithms for social influence analysis. In: Aggarwal CC, editor. Social Network Data Analytics. New York, NY: Springer (2011). p. 177–214.
26. Gershenson C. The world as evolving information. In: Minai A, Braha D, Bar-Yam Y, editors. Unifying Themes in Complex Systems. Vol. VII. Heidelberg: Springer (2012). p. 100–15.
27. Bag S, Tiwari MK, Chan FT. Predicting the consumer's purchase intention of durable goods: an attribute-level analysis. J Bus Res. (2019) 94:408–19. doi: 10.1016/j.jbusres.2017.11.031
28. Pham MT. The seven sins of consumer psychology. J Consum Psychol. (2013) 23:411–23. doi: 10.1016/j.jcps.2013.07.004
29. Seoane LF, Solé R. The morphospace of language networks. Sci Rep. (2018) 8:10465. doi: 10.1038/s41598-018-28820-0
30. Henderson GR, Iacobucci D, Calder BJ. Brand diagnostics: mapping branding effects using consumer associative networks. Eur J Oper Res. (1998) 111:306–27. doi: 10.1016/S0377-2217(98)00151-9
31. Kopetz CE, Kruglanski AW, Arens ZG, Etkin J, Johnson HM. The dynamics of consumer behavior: a goal systemic perspective. J Consum Psychol. (2012) 22:208–23. doi: 10.1016/j.jcps.2011.03.001
32. Rocha LEC, Holme P. The network organisation of consumer complaints. Europhys Lett. (2010) 91:28005. doi: 10.1209/0295-5075/91/28005
33. Pineda OK, Kim H, Gershenson C. A novel antifragility measure based on satisfaction and its application to random and biological Boolean networks. Complexity. (2019) 2019:3728621. doi: 10.1155/2019/3728621
34. Amancio DR, Silva FN, Costa LdF. Concentric network symmetry grasps authors' styles in word adjacency networks. Europhys Lett. (2015) 110:68001. doi: 10.1209/0295-5075/110/68001
36. Bakshi S, Jagadev AK, Dehuri S, Wang GN. Enhancing scalability and accuracy of recommendation systems using unsupervised learning and particle swarm optimization. Appl Soft Comput J. (2014) 15:21–9. doi: 10.1016/j.asoc.2013.10.018
Keywords: emergence, self-organization, applied complexity, commerce-consumer research, electronic word-of-mouth
Citation: Correa JC (2020) Metrics of Emergence, Self-Organization, and Complexity for EWOM Research. Front. Phys. 8:35. doi: 10.3389/fphy.2020.00035
Received: 31 October 2019; Accepted: 05 February 2020;
Published: 21 February 2020.
Edited by:
Carlos Gershenson, National Autonomous University of Mexico, MexicoReviewed by:
Diego R. Amancio, University of São Paulo, BrazilCopyright © 2020 Correa. This is an open-access article distributed under the terms of the Creative Commons Attribution License (CC BY). The use, distribution or reproduction in other forums is permitted, provided the original author(s) and the copyright owner(s) are credited and that the original publication in this journal is cited, in accordance with accepted academic practice. No use, distribution or reproduction is permitted which does not comply with these terms.
*Correspondence: Juan C. Correa, anVhbmMuY29ycmVhbkBrb25yYWRsb3JlbnouZWR1LmNv
Disclaimer: All claims expressed in this article are solely those of the authors and do not necessarily represent those of their affiliated organizations, or those of the publisher, the editors and the reviewers. Any product that may be evaluated in this article or claim that may be made by its manufacturer is not guaranteed or endorsed by the publisher.
Research integrity at Frontiers
Learn more about the work of our research integrity team to safeguard the quality of each article we publish.