- 1Department of Physics, Virginia Commonwealth University, Richmond, VA, United States
- 2Department of Pathology, Virginia Commonwealth University, Richmond, VA, United States
- 3Wright Center for Clinical and Translational Research, Virginia Commonwealth University, Richmond, VA, United States
- 4Massey Cancer Center, Virginia Commonwealth University, Richmond, VA, United States
The development of resistance to initially successful cancer therapies is a major cause of the morbidity and mortality associated with cancer. Identifying evolving resistance at an early stage could inform clinical decision making to adapt therapies before resistant cancer cell phenotypes have become clonally dominant or metastasized. This goal of early detection has prompted heavy investments in liquid biopsy, organoid, and high-throughput screening methodologies. Recently, High-Speed Live-Cell Interferometry (HSLCI), a quantitative phase imaging (QPI) methodology, was shown to predict triple-negative breast cancer (TNBC) patient-derived xenograft (PDX) sensitivity to carboplatin only 40 h after tumor removal from a mouse. In this paper we discuss barriers to applying HSLCI to therapy selection in human TNBC patients, and present preliminary results addressing some of those barriers. Our results include engineering improvements to increase sample throughput and demonstrating that HSLCI can measure drug response of agents with a variety of mechanisms of action. Finally, we show proof of concept data for direct testing of samples obtained from minimally invasive, fine needle biopsies.
Introduction
Approximately one third of triple-negative breast cancer (TNBC) patients experience drug-resistant metastatic disease that is often fatal [1]. Current guidelines attempt to personalize cancer therapy based on a patient's general state of health, clinical tumor staging, hormone receptor status, and mutation profile. But these guidelines are often inadequate at predicting therapeutic resistance before bulk tumor growth restarts and potentially metastasizes [2–4].
Liquid biopsies, which detect circulating biomarkers, exosomes, microRNAs, circulating tumor DNA, and tumor cells in blood, accurately reflect tumor heterogeneity and are easy to acquire. Yet, this approach still depends on sequencing and requires an understanding of the clinical significance of each detected mutation. This dependency leads to a high specificity (>95%) but poor sensitivity (66%) for predicting therapeutic resistance in patients [5]. If a known resistance-related mutation is detected, liquid biopsy methodologies reliably predict patient therapeutic sensitivity. However, in more than one third of cases, potential resistance-related mutations are not found, or the significance of detected mutations is unknown, resulting in the incorrect prediction of susceptibility to therapeutics.
Recent improvements in tumoroid culture allow for the ex vivo proliferation of primary patient samples. These advances make it feasible to screen patient samples for therapeutic sensitivity against a large panel of potential drugs [6, 7]. However, this technology suffers from cost and procedural complexity drawbacks that limit its utility in clinical settings. Furthermore, as implemented, these assays rely on the bulk averaging of fluorescent viability signals acquired from an entire sample well (representing a heterogeneous cell population), making the detection of minute subpopulations inherently impractical.
High-Speed Live-Cell Interferometry (HSLCI) is one approach our laboratory has been exploring as an ex vivo assay method to predict therapeutic response in cancer. HSLCI uses rapid, microscopic quantitative phase imaging (QPI) to detect changes in the biomass of single living cells or cell clusters in response to cytotoxic or cytostatic drug exposure. The system (Figure 1) consists of a custom inverted microscope equipped with a modified Shack-Hartman wavefront sensing camera (SID4Bio, Phasics) for rapid, vibration-insensitive cell mass measurements. Dynamic focus stabilization enables continuous image collection over the entire sample area without pause. Cells are imaged in a glass-bottomed, standard-footprint multi-well plate (24–96 wells). Whole microscope enclosures provide long-term environment stability for imaging under standard cell culture conditions.
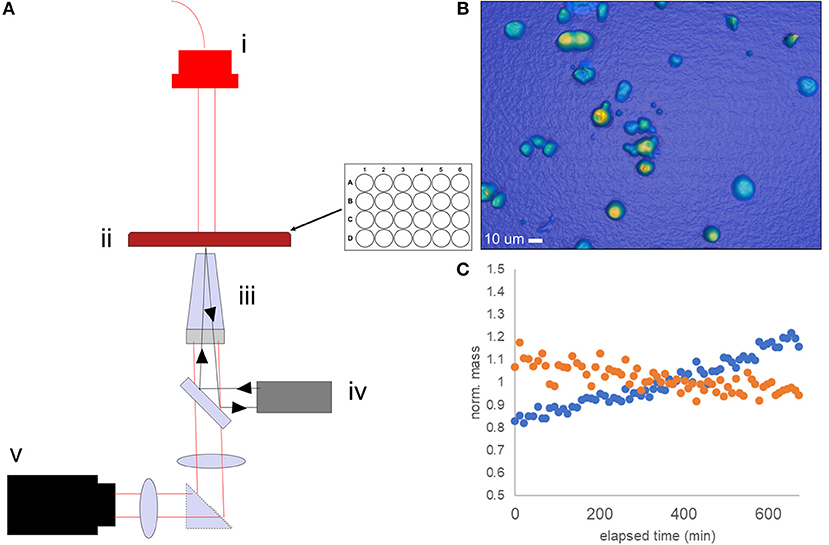
Figure 1. Schematic of the HSLCI multi-well biomass accumulation assay. (A) The HSLCI system is configured with (i) fiber-coupled LED illumination source, (ii) standard footprint 24 well plate with X-Y motion controlled by motorized stages, (iii) a 40x Nikon Plan Flourite, N.A. 0.75, objective on top of piezo actuator (light gray) with focus maintained by autofocus system (iv), and (v) a wide field phase detection camera (SID4Bio, Phasics). (B) Example image of TNBC PDX cells. (C) Individual cells are tracked between images; biomass vs. time plots are generated. Shown is a typical normalized mass vs. time plot for a single drug sensitive (orange) and drug-resistant (blue) cell.
This technology can non-invasively measure the dry mass of living cells in real-time over the course of 12 h to 5 days [8, 9]. Earlier work by our group has established that live cell mass accumulation rates measured by QPI can be used to assess response to cytostatic or cytotoxic cancer drugs. These mass accumulation-based responses were found to be concordant with traditional cell counting and fluorescent reporter viability assays in a variety of cancer types, including breast, multiple myeloma, and melanoma [9–11].
More recently, we showed similar results in a small pilot study using carboplatin treated patient-derived xenograft (PDX) models of Triple Negative Breast Cancer (TNBC) [9]. While establishing that “fresh out of the animal” tissues could be tested in a similar fashion to cell lines, this work was limited in both the diversity of the tumors (three PDXs were tested) and the scope of the therapeutic mechanisms of action studied. To firmly establish the preclinical efficacy of the HSLCI assay, as a prelude to testing human patients, the technology must be compared to a spectrum of measures which serve as a proxy for response in patients. These proxies include both in vitro assays, such as the luciferase assay, and tumor animal models. In detail, the effectiveness of a drug as measured by the decrease in mass accumulation of single cells by HSLCI must be compared to current preclinical assays which rely on metabolic indicators for a proxy of cell number [12]. This work must be completed in multiple tumor models and with multiple drugs of different mechanisms. Finally, the response measured by HSLCI would be compared to clinical results in a Phase 1 correlative clinical trial, as we have previously done correlating T-Cell mass to the severity of graft vs. host disease in stem cell transplantation [13]. Through these studies and future clinical studies, a threshold parameter such as a level of median growth can be established to predict effectiveness in patients.
Additionally, drugs and drug combinations representing current and emerging therapies for TNBC need to be evaluated simultaneously, as would be required for any therapy selection assay. Finally, in practice the amount of live cell tumor material obtainable for an in vitro assay will be quite limited to possibly only hundreds to thousands of cells. Ideally, accurate measurements would be obtainable using the least invasive biopsies.
In this paper we describe recent improvement in the HSLCI methodology designed to address these hurdles. Hardware modifications are employed that increase imaging throughput by 8-fold, enabling the effective screening of a wide range of cell concentrations, as is required when the incoming sample quantity is unknown and may vary greatly from sample to sample. We also show that HSLCI delivers qualitatively and quantitatively similar measurements using two classes of investigational TNBC therapeutics, proteasome inhibitors and CDK 4/6 inhibitors.
TNBC therapy is currently limited to chemotherapeutics, as TNBC tumors lack typical targets for drugs such as human epidermal growth factor receptor 2 (HER2), estrogen receptor (ER), and progesterone receptor (PR) [2]. This has led to the pursuit of new targeted agents such as proteasome inhibitors and CDK 4/6 inhibitors, both of which have both shown promise in TNBC cell lines and TNBC mouse models.
Throughput Considerations
Our recent work with TNBC PDX models [9] demonstrated that “fresh from the animal” samples generate mostly non-adherent spheroids when processed, and that the sample contains a significant amount of remnant cell debris which must be excluded from analysis. The total number of cells in each sample well can vary widely depending on the individual tumor. To compensate for these issues, which result in low or variable viable cell counts, we sought to increase the overall throughput vs. that reported previously [10].
The raw imaging throughput was increased roughly 8-fold, from 4 to 32 fps, by increasing the bandwidth of the autofocus feedback loop using a custom microcontroller-based PID control circuit. The current system operates at a refresh rate >1 kHz, enabling much faster point-to-point translation of the sample. At the operating depth of field (0.5 μm; Nikon 40x air objective), testing demonstrated the PID loop kept the microscope within ±0.295 μm of the commanded focus position 95% of the time (Supplementary Figure 1).
The substantial increase in speed is necessary for ex-vivo samples which can be highly variable in cell number and must all be screened in a limited time window shortly after biopsy. While Figure 1B illustrates a representative image, images can vary from having no cells to cells so closely grouped that they become challenging to track depending on the cellularity of the sample and success of the biopsy.
The short window of viability of patient samples requires that all data must be collected within a tight time frame. Cells must be exposed to drugs for between 24 and 48 h to give time for the drugs to take effect, and then imaged for around 12–16 h before viability is lost. To properly track cells from image to image, an image must be taken in the same location about every 10 min. Additionally, drug resistant cancer cells which must be detected may be present in as low as 1/1,000–1/10,000 [14]. Due to the time constraints discussed above, the number of cells screened cannot be increased by lengthening the experiment. The number of cells can only be increased by faster imaging. By taking 8x more images in the same time period as earlier platforms, the increases in speed have enabled us to increase the number of conditions in a single experiment from five to twelve.
In contrast to imaging speed, processing speed is only limited by convenience and can be upgraded through increasing the number of GPU and CPUs without technical changes. Therefore, as it is critical to obtain as much data as possible in the limited time window after biopsy, images are processed after the data has been collected so the processing time does not serve as barrier to data collection. The imaging throughput is not the same as processing throughput.
In the past, processing throughput was limited in practice by the time required to calculate the phase image from the raw interferogram (typically ~500 ms on a single Intel Core i7 processor). To process this large amount of data in a timely fashion, we employed a new GPU-based (NVIDIA Quadro P2000) phase unwrapping algorithm provided by the camera manufacturer (Phasics). This new implementation reduced the comparable frame processing time to 85 ms. Further increases in speed can be accomplished by increasing the number of CPU or GPU cores.
Proteasome Inhibitors (PIs)
Proteasome inhibitors (PIs) target the process of protein degradation via the ubiquitin-proteasome system. Degradation of proteins through proteasome activity is essential for the maintenance of cell homeostasis. Blocking the activity of the proteasome leads to the accumulation of toxic poly-ubiquitinated proteins within the cell. This accumulation is more pronounced in cancer cells given their high rate of proliferation and subsequently increased rate of protein synthesis [15]. PIs have become very successful in treating hematologic malignancies, and are being investigated for use in solid cancers, including TNBC.
Results from experiments on TNBC cell lines and xenograft models have shown that a subpopulation of cells usually survive after treatment with PIs [16]. This has been attributed to the ability of the resistant cells' proteosomes to utilize alternative enzymatic sites not blocked by the inhibitor molecule [16]. These findings provide a rationale for screening with a method like HSLCI that can resolve intra-tumor heterogeneity to better identify risks of failure due to minority resistant populations. Furthermore, since proteasome inhibitors directly interfere with the protein synthesis functions of the cell, one would expect that mass accumulation would serve as a good reporter for response in this therapeutic class.
We used HSLCI to measure the response of two TNBC PDX tumors to the FDA-approved PI bortezomib, a drug currently used to treat multiple myeloma and certain lymphomas, and the same class of drug that is currently being tested in TNBC (ClinicalTrials.gov Identifier: NCT02993094). Tumors were excised from mice and enzymatically digested to single-cell suspensions following previously-described protocols [9, 17]. Cells were then treated for 24 h and then monitored by HSLCI for the following 16 h (24–40 h after treatment). Each HSLCI assay included parallel measurements of cells exposed to a log-scale dose range of bortezomib (Figure 2). For comparison, we also conducted standard luciferase viability assays under the same conditions. These assays differ from HSLCI in that rather than measuring cell-by-cell kinetic responses, they quantify average ATP content of the sample at a fixed time point (usually 72 h after dosing).
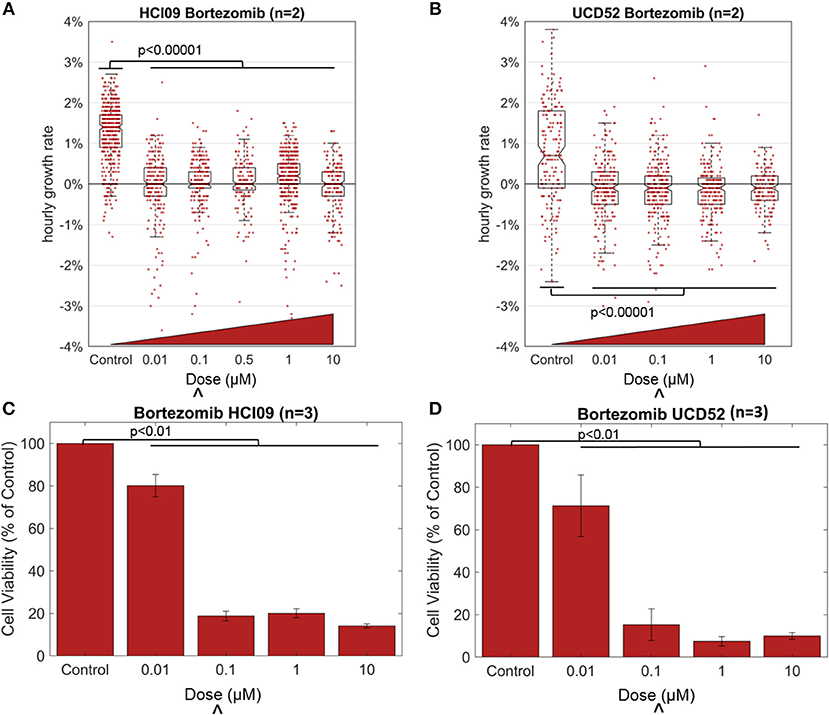
Figure 2. Effect of the proteasome inhibitor bortezomib in TNBC PDXs HCI09 and UCD52, measured by HSLCI (A,B) and standard luciferase assays (C,D). Individual dots in the overlaying the box plot represent the mass accumulation rates of single cells measured over the interval 24–40 h post-dosing. Box-plot notches are indicative of 95% confidence intervals for the medians. The “∧” symbol indicates the in vitro dose that corresponds to the maximum tolerated serum concentration in humans [18]. In HSLCI experiments, the data shown was pooled from two replicates. In (C,D), error bars represent standard deviation. Two sample t-tests were performed between all populations compared to the control populations.
Bortezomib was effective in reducing the mass accumulation rates of both PDX tumors, even at the lowest dose of 10 nM from ~1 to ~0%. In previous studies, a median growth rate of 0% at a dose equivalent to the highest concentration seen in patients reduced tumor burden in mice [9]. But more studies are required to determine the exact level of mass accumulation reduction that will correlate in patients. The data in Figure 2 is pooled from two replicates. For the individual replicates the median growth rates for each condition were within 0.1% of each other with p-values > 0.11 in both PDX models, demonstrating reproducibility of these HSLCI screens. Despite the drastic decrease in median growth, at all doses we observed many individual cells that vigorously accumulated mass during the observation period, indicating little or no drug response. This included doses well above the maximum tolerated serum concentration in patients (0.1 μM). The mass vs. time behavior for one such “resistant” cell from a UCD52 PDX is shown in Figure 3. As discussed previously, it is possible that these “resistant” cells are effectively utilizing non-targeted proteasome sites [16]. We note that the actual fraction of drug resistant cells is likely smaller than measured, as many responsive cells have died and become debris after 24 h.
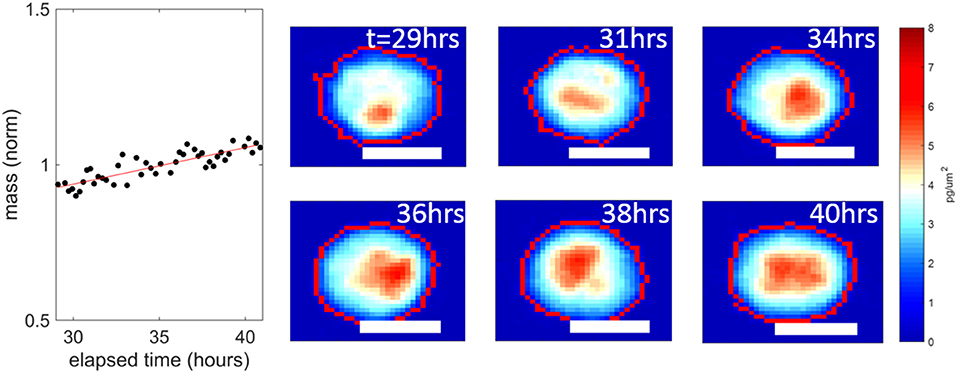
Figure 3. An example UCD52 cell resistant to 1 μM bortezomib, from the data set presented in Figure 2B. This cell continued accumulating mass a 1.2 ± 0.1% per hour over the interval 30–40 h post dosing. The white scale bars are all 10 μm.
In concordance with the HSLCI results, luciferase assays found significant decreases in the median sample viability due to bortezomib treatment for both PDXs at concentrations of 0.1 μM and above (Figure 2). The luciferase assay indicated a less dramatic response for both PDXs at low drug concentrations (0.01 μM) than did the mass accumulation assay. Our interpretation of these results is that at the 0.01 μM concentration, bortezomib effectively inhibits biomass synthesis, which is primarily due to protein production. However bortezomib may only modestly impact cell viability as measured by ATP production at 72 h. The clinical significance of this difference could be resolved by comparison to in vivo anti-tumor response at the same dose. As tumor growth can be variable from mouse to mouse and the need for a large number of cells to re-seed tumors in the next generation of mice, the number of doses tested in the luciferase assay was limited by the amount of material available at the time of testing.
CDK 4/6 Inhibitors
CDK 4/6 inhibitors block cyclin dependent kinases, which serve to progress cells through the cell cycle. They are typically upregulated in cancer cells as part of an increase in proliferation. Several drugs of this class are approved for treatment of hormone receptor-positive, HER2-negative breast cancer. These drugs are not effective in the common basal type TNBCs, however recent work has shown that luminal androgen receptor-positive TNBCs are sensitive to CDK 4/6 inhibitors [19], and thus they are becoming a more clinically relevant class of drugs for this disease.
Drugs that target the CDK cell cycle control pathway have not been previously tested using HSLCI. Because they specifically target the G1 cell cycle checkpoint, timing of exposure and the window in which the mass accumulation measurement occurs may impact the observed response differently than do drugs such as boretezomib, which are not cell cycle specific.
To gain insight into the timing and magnitude of response measurable with HSLCI, we prepared cells from the luminal androgen receptor-positive PDX HCI09 as described in the Methods and exposed them to CDK4/6 inhibitors palbociclib or abemaciclib at a range of escalating concentrations for 60 h. We monitored the samples for 12 h with HSLCI, starting at hour 48 after dosing (Figure 4, Supplementary Table 1).
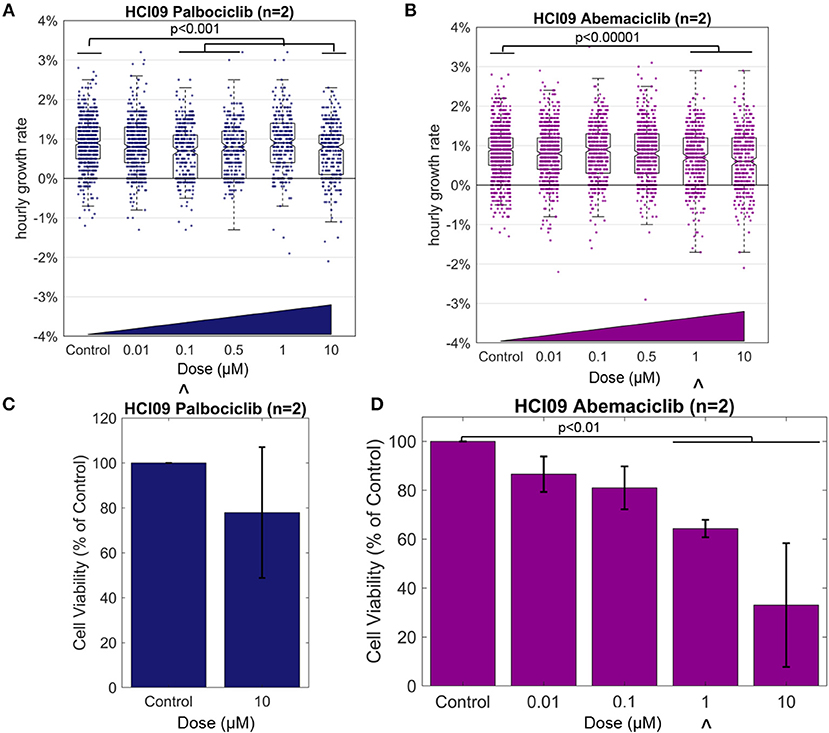
Figure 4. Effect of the CDK4/6 inhibitors on TNBC PDX HCI09, measured by HSLCI (A,B) and standard luciferase assays (C,D). Individual dots in the overlaying the box plot represent the mass accumulation rates of single cells measured over the interval 24–40 h post-dosing. Box-plot notches are indicative of 95% confidence intervals for the medians. The “∧” symbol indicates the in vitro dose that corresponds to the maximum tolerated serum concentration in humans [18]. In HSLCI experiments, the data shown was pooled from two replicates. In (C,D), error bars represent standard deviation. Two sample t-tests were performed between all populations compared to the control populations.
The median hourly mass accumulation rate of cells treated with palbociclib was slightly reduced for three of the four doses above 0.01 μM (median treated growth rate ~0.8% per hour vs. 0.9% for control; p < 0.001). Though statistically significant, the small magnitude of response and the lack of a clear relationship to escalating doses suggests that pablociclib would not be an effective treatment in vivo for this tumor. Past experience with HSLCI dose response curves informs us that a fluctuation of this magnitude (~0.1%) is relatively insignificant and within typical random error (such as tumor sampling heterogeneity or pipetting error) for such samples [9, 10]. This conclusion is supported by the results of a luciferase assay, which showed a similar marginal response at the highest tested dose of 10 μM. However, we note that 10 μM is well above the maximum tolerated serum concentration in humans (0.01 μM).
In the HSLCI assay, the magnitude of response to abemaciclib was similar to that seen with pablociclib, except that the dose response was more clearly defined. Above 1 μM, the maximum tolerated serum concentration in humans, the reduction in median mass accumulation rate vs. control became statistically significant. Similarly, the luciferase assay showed a scaled reduction in viability corresponding to increasing doses which became significant also at 1 μM, but again the small magnitude of response indicates abemaciclib may only be marginally effective in vivo. Due to smaller than anticipated tumor size, the number of doses tested in the luciferase assay was limited by the amount of material available at the time.
Tests Using Fine Needle Biopsy-Derived Samples
Prior HSLCI PDX experiments employed bulk excision and enzymatic digestions of murine tumors, resulting in the acquisition of millions of cells [9]. This approach is not feasible in the clinic where procedures need to be minimally invasive for the patients and samples are often divided for multiple analyses, including sequencing and histology. Fine needle biopsy (FNB) is a frequently-utilized procedure which provides minimally invasive access to patient tissue through the use of small needles. A key question to be resolved in use of FNB with HSLCI is whether or not the process is so perturbative to the cells' physiologic state that it masks the effects of the drug under study.
To address this question, we developed a simple needle biopsy protocol and compared the results obtained with it to those previously obtained using a bulk tumor extraction (Figure 5). We tested this protocol using PDX model HCI09, previously shown to be resistant to carboplatin at the therapeutically relevant dose of 100 μM in vivo [9]. HCI09 tumors were biopsied with a 22-gauge needle, yielding between 10,000 and 40,000 viable cells. Debris and dead cells were removed using the Easy Sep® Dead Cell removal kit, which selects for inner leaflet phospholipid Annexin V [20]. The remaining viable cells were then directly plated into standard-footprint 24-well plates, treated with high dose carboplatin (100 μM), and imaged 24–36 h after drug administration. For comparison, a standard bulk enzymatic digestion was performed in parallel.
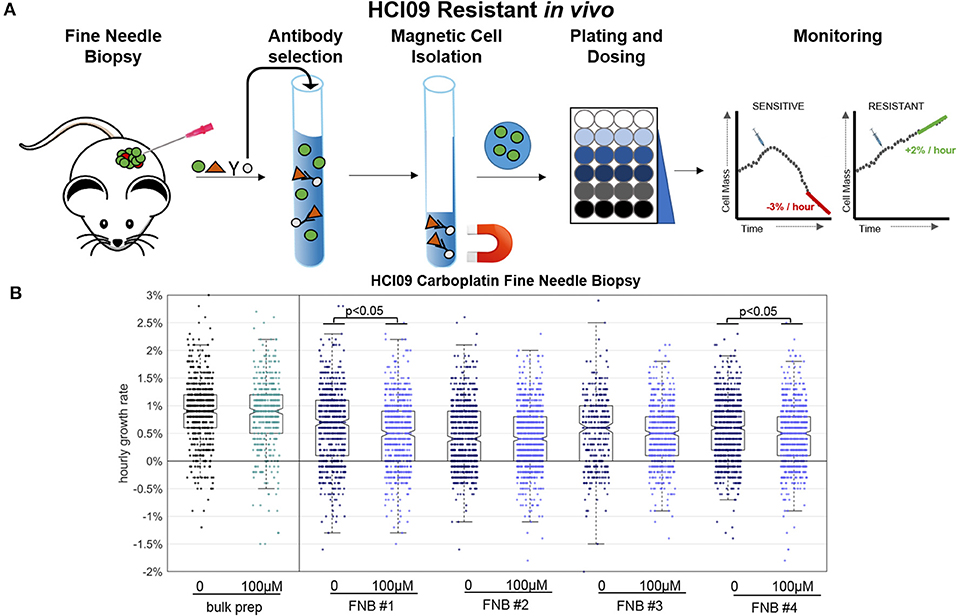
Figure 5. Fine needle biopsy (FNB) sample preparation compared to bulk prep. (A) Timeline for key steps in the FNB. (B) HSLCI results for carboplatin-resistant PDX HCI09. Box plot notches are indicative of 95% confidence intervals for the medians, and each dot represents the growth rate of one cell.
Four independent fine-needle biopsies were performed, labeled as FNB #1 etc. in Figure 5. Between 700 and 1,500 cells were isolated and monitored from each biopsy. We found that the negative purification process was absolutely required due to the relatively large fraction of debris present in the FNB as compared to the bulk sample prep, which could be used without this step.
Compared to the bulk prep, both the treated and untreated FNB samples displayed slightly lower median hourly mass accumulation rates, down ~0.3%, from ~0.9% per hour in the bulk prep to ~0.6% per hour in the FNB prep. FNB and bulk prep samples showed a comparable spread of single cell growth rates about the median. The four FNB replicates behaved similarly to one another, with minor differences between replicates in line with our previous PDX results [9]. Two of the four carboplatin treated cases showed a slight (~0.2%) but statistically meaningful median reduction in mass accumulation rate, again within the previously observed range for PDX bulk prep replicates (Supplementary Table 2).
We conclude that the isolation process results in a slight reduction in median population mass accumulation rates relative to the bulk prep approach (<0.3% suppression), but this effect is small enough that it does not mask carboplatin resistance in this particular PDX, nor does it appear to substantially alter the intra-sample growth rate heterogeneity. The mass accumulation suppression induced by FNB could become more meaningful in cases where the untreated samples have naturally low mass accumulation rates and is clearly an issue requiring further study. However, our initial experiments support the viability of FNB as a route to obtaining HSLCI-measurable samples from human patients.
Discussion and Conclusions
In these experiments, HSLCI and standard luciferase assays proved to be generally concordant. There were slight differences between the two methods with regard to the measured magnitude of dose response for both PIs and CDK 4/6 inhibitors. The ultimate significance of these differences will have to be determined by comparison with in vivo experiments in the PDX models, an area currently under study.
The methodological improvements in throughput and sample preparation allowed us to conduct measurements using fine needle biopsy material, vs. the prior requirement of bulk quantities of disaggregated tissues. In the particular PDX model tested, HCI09, the main artifact attributed to the needle biopsy was a slight decrease on median sample mass accumulation rate. While this perturbation was too small to obscure the results here, this effect needs to be assessed across a range of different PDX tumors. Nevertheless, we feel that the success of the fine needle biopsy experiments support the potential viability of HSLCI as an ex vivo assay technique which can be practically applied in the clinic.
Multiple other analytical methodologies are being explored to address the practical issues of in vitro chemosensitivity assays. For instance, to distinguish tumor response heterogeneity, fluorescent signals from individual cells can be directly counted [21]. However, these assays must be designed carefully as fluorescence can be influenced by several factors such as cell attachment, intracellular calcium, and drug induced artifacts [12, 21]. Additionally, fluorescence is typically transient, lasting a couple of hours at peak intensity [21]. Alternatively, unlabeled chemical spectroscopy, such as Raman [22–24] or fluorescence lifetime imaging [25] can provide details on changes in metabolism or DNA content of cells due to drugs. Compared to HSLCI, these techniques currently have limited throughput and research is ongoing to improve these methods for patient tissue screening [10, 24, 26].
Methods
HSLCI Drug Screen
The HSLCI platform is a custom-built inverted optical microscope coupled to an off-axis quadriwave lateral shearing interferometric camera (SID4BIO, Phasics, Inc.). Cells are imaged in single, standard-footprint (128 × 85 mm), glass-bottomed, multi-well plates. Acquired images are analyzed by a downstream PC using NVIDIA K2000 GPU. All of the platform's hardware and software components are available commercially. A 40x objective (Nikon, NA 0.75) was used for the growth kinetics studies described. The HSLCI platform was installed inside a standard cell culture incubator. Cells were plated on 24-well glass bottom plates at 5–7.5 × 104 cells per well in M87 medium [17]. Cells were treated for 24 or 48 h depending on whether the drug was cell cycle specific and then monitored for the following 16 h.
To account for the potential noise introduced by drifting cells and cell debris that could artificially impact measured growth rates of stable cells, data was quality filtered such that only biomass tracks (mass vs. time) exhibiting linear fit standard errors of less than 0.002 normalized mass units per hour and a total mass of greater than 300 pg but less than 3,000 pg were included. These error bounds ensure our confidence in the hourly mass accumulation rates is ±0.2% and that only true physiologic cell growth is measured. The minimum mass filter ensures that our data only include individual cells or two-three cell clusters, and not cell debris.
Luciferase Assays
HCI09 and UCD52 cells were plated in M87 medium [17], in triplicate (25,000 cells/100 μl per well) in 96-well plates and incubated at 37°C for 3 days with six different concentrations of carboplatin. To assess cell viability over time, D-luciferin (10 μl/well) was added and plates were imaged using an In Vivo Imaging System (IVIS) on day 3.
Fine Needle Biopsy
Tumors were excised and then biopsied with a 22-gauge needle. Debris and dead cells were removed with Easy Sep® Dead Cell Removal kits. Cells were then plated and dosed for 24 h, and then monitored by HSLCI for 12 h.
Statistical Tests
Two-sample t-tests were performed between each drug condition and the appropriate control condition for both HSLCI and luciferase assay. For HSLCI, the null hypothesis was that the growth rates of single cells in the control and drugged populations were from independent random samples from a normal distribution with equal means and equal but unknown variances. The data was pooled from two replicates for these experiments. Similarly, in luciferase assays, the normalized fluorescence values for each test were compared.
Author Contributions
GM, KL, DG, and JR developed the HSCLI instrumentation. GM, JH, and JR designed experiments, analyzed data, and wrote the manuscript. GM, TT, MA, and JH performed experiments. All authors discussed data and edited the manuscript.
Funding
Funding was provided by National Institutes of Health grant R01CA185189 to JR, and in part, by the NCI Cancer Center Support Grant P30CA016059 to the VCU Massey Cancer Center. Additional funding was provided by VCU Presidential Research Quest fund awarded to JR.
Conflict of Interest
The authors declare that the research was conducted in the absence of any commercial or financial relationships that could be construed as a potential conflict of interest.
Supplementary Material
The Supplementary Material for this article can be found online at: https://www.frontiersin.org/articles/10.3389/fphy.2019.00158/full#supplementary-material
References
1. Noone AM, Howlader N, Krapcho M, Miller D, Brest A, Yu M, et al. (editors). SEER Cancer Statistics Review, 1975-2015. Bethesda, MD: National Cancer Institute (2017). Available online at: https://seer.cancer.gov/csr/1975_2015/
2. Gradishar WJ, Anderson BO, Balassanian R, Blair SL, Burstein HJ, Cyr A, et al. Breast cancer, Version 4.2017, NCCN clinical practice guidelines in oncology. J Natl Compr Canc Netw. (2018) 16:310–20. doi: 10.6004/jnccn.2018.0012
3. Giordano SH, Elias AD, Gradishar WJ. NCCN guidelines updates: breast cancer. J Natl Compr Canc Netw. (2018) 16:605–10. doi: 10.6004/jnccn.2018.0043
4. Bevers TB, Helvie M, Bonaccio E, Calhoun KE, Daly MB, Farrar WB, et al. Breast cancer screening and diagnosis, version 3.2018, NCCN clinical practice guidelines in oncology. J Natl Compr Canc Netw. (2018) 16:1362–89. doi: 10.6004/jnccn.2018.0083
5. Merker JD, Oxnard GR, Compton C, Diehn M, Hurley P, Lazar AJ, et al. Circulating tumor DNA analysis in patients with cancer: american society of clinical oncology and college of american pathologists joint review. J Clin Oncol. (2018) 36:1631–41. doi: 10.1200/JCO.2017.76.8671
6. Sachs N. A living biobank of breast cancer organoids captures disease heterogeneity. Cell. (2018) 172:373–86. doi: 10.1016/j.cell.2017.11.010
7. Aboulkheyr EH, Montazeri L, Aref AR, Vosough M, Baharvand H. Personalized cancer medicine: an organoid approach. Trends Biotechnol. (2018) 36:358–71. doi: 10.1016/j.tibtech.2017.12.005
8. Saleh T, Tyutyunyk-Massey L, Murray GF, Alotaibi MR, Kawale AS, Elsayed Z, et al. Tumor cell escape from therapy-induced senescence. Biochem Pharmacol. (2018) 162:202–12. doi: 10.1016/j.bcp.2018.12.013
9. Murray GF, Turner TH, Leslie KA, Alzubi MA, Guest D, Sohal SS, et al. Live cell mass accumulation measurement non-invasively predicts carboplatin sensitivity in triple-negative breast cancer patient-derived xenografts. ACS Omega. (2018) 3:17687–92. doi: 10.1021/acsomega.8b02224
10. Huang D, Leslie K, Guest D, Yeshcheulova O, Roy I, Piva M, et al. High speed live cell interferometry: a new method for rapidly quantifying tumor drug resistance and heterogeneity. Anal Chem. (2018) 90:3299–306. doi: 10.1021/acs.analchem.7b04828
11. Chun J, Zangle TA, Kolarova T, Finn RS, Teitell MA, Reed J. Rapidly quantifying drug sensitivity of dispersed and clumped breast cancer cells by mass profiling. Analyst. (2012) 137:5495–8. doi: 10.1039/c2an36058f
12. Blom K, Nygren P, Larsson R, Andersson CR. Predictive value of ex vivo chemosensitivity assays for individualized cancer chemotherapy: a meta-analysis. SLAS Technol. (2017) 22:306–14. doi: 10.1177/2472630316686297
13. Leslie KA, Rasheed M, Sabo RT, Roberts CC, Toor AA, Reed J. Reconstituting donor T cells increase their biomass following hematopoietic stem cell transplantation. Analyst. (2018) 143:2479–85. doi: 10.1039/C8AN00148K
14. Kim C, Gao R, Sei E, Brandt R, Hartman J, Hatschek T, et al. Chemoresistance evolution in triple-negative breast cancer delineated by single-cell sequencing. Cell. (2018) 173:879–93 e13. doi: 10.1016/j.cell.2018.03.041
15. Crawford LJ, Walker B, Irvine AE. Proteasome inhibitors in cancer therapy. J Cell Commun Signal. (2011) 5:101–10. doi: 10.1007/s12079-011-0121-7
16. Weyburne ES, Wilkins OM, Sha Z, Williams DA, Pletnev AA, de Bruin G, et al. Inhibition of the proteasome beta2 site sensitizes triple-negative breast cancer cells to beta5 inhibitors and suppresses Nrf1 activation. Cell Chem Biol. (2017) 24:218–30. doi: 10.1016/j.chembiol.2016.12.016
17. DeRose YS, Gligorich KM, Wang G, Georgelas A, Bowman P, Courdy SJ, et al. Patient-derived models of human breast cancer: protocols for in vitro and in vivo applications in tumor biology and translational medicine. Curr Protoc Pharmacol. (2013) Chapter 14:Unit14.23. doi: 10.1002/0471141755.ph1423s60
18. LoRusso PM, Venkatakrishnan K, Ramanathan RK, Sarantopoulos J, Mulkerin D, Shibata SI, et al. Pharmacokinetics and safety of bortezomib in patients with advanced malignancies and varying degrees of liver dysfunction: phase I NCI Organ Dysfunction Working Group Study NCI-6432. Clin Cancer Res. (2012) 18:2954–63. doi: 10.1158/1078-0432.CCR-11-2873
19. Asghar US, Barr AR, Cutts R, Beaney M, Babina I, Sampath D, et al. Single-cell dynamics determines response to CDK4/6 inhibition in triple-negative breast cancer. Clin Cancer Res. (2017) 23:5561–72. doi: 10.1158/1078-0432.CCR-17-0369
21. Miles FL, Lynch JE, Sikes RA. Cell-based assays using calcein acetoxymethyl ester show variation in fluorescence with treatment conditions. J Biol Methods. (2015) 2:e29. doi: 10.14440/jbm.2015.73
22. Schie IW, Huser T. Methods and applications of Raman microspectroscopy to single-cell analysis. Appl Spectrosc. (2013) 67:813–28. doi: 10.1366/12-06971
23. Smith R, Wright KL, Ashton L. Raman spectroscopy: an evolving technique for live cell studies. Analyst. (2016) 141:3590–600. doi: 10.1039/C6AN00152A
24. Zhang Q, Zhang P, Gou H, Mou C, Huang WE, Yang M, et al. Towards high-throughput microfluidic Raman-activated cell sorting. Analyst. (2015) 140:6163–74. doi: 10.1039/C5AN01074H
25. Meyer-Almes FJ. Fluorescence lifetime based bioassays. Methods Appl Fluoresc. (2017) 5:042002. doi: 10.1088/2050-6120/aa7c7a
Keywords: quantitative phase imaging, personalized medicine, fine needle biopsy, patient derived xenografts, breast cancer, high speed live cell interferometry, proteasome inhibitors, CDK 4/6 inhibitors
Citation: Murray GF, Turner TH, Guest D, Leslie KA, Alzubi MA, Radhakrishnan SK, Harrell JC and Reed J (2019) QPI Allows in vitro Drug Screening of Triple Negative Breast Cancer PDX Tumors and Fine Needle Biopsies. Front. Phys. 7:158. doi: 10.3389/fphy.2019.00158
Received: 04 February 2019; Accepted: 30 September 2019;
Published: 16 October 2019.
Edited by:
YongKeun Park, Korea Advanced Institute of Science & Technology (KAIST), South KoreaReviewed by:
Martina Mugnano, Institute of Applied Sciences and Intelligent Systems (ISASI), ItalyKelvin Chak Man Lee, The University of Hong Kong, Hong Kong
Copyright © 2019 Murray, Turner, Guest, Leslie, Alzubi, Radhakrishnan, Harrell and Reed. This is an open-access article distributed under the terms of the Creative Commons Attribution License (CC BY). The use, distribution or reproduction in other forums is permitted, provided the original author(s) and the copyright owner(s) are credited and that the original publication in this journal is cited, in accordance with accepted academic practice. No use, distribution or reproduction is permitted which does not comply with these terms.
*Correspondence: J. Chuck Harrell, am9zaHVhLmhhcnJlbGxAdmN1aGVhbHRoLm9yZw==; Jason Reed, amNyZWVkQHZjdS5lZHU=