- 1ICFO - Institut de Ciències Fotòniques, The Barcelona Institute of Science and Technology, Barcelona, Spain
- 2Facultat de Ciències i Tecnologia, Universitat de Vic - Universitat Central de Catalunya (UVic-UCC), Vic, Spain
- 3Center for Quantum Physics, University of Innsbruck, and Institute for Quantum Optics and Quantum Information, Austrian Academy of Sciences, Innsbruck, Austria
- 4Departament de Física, Universitat Autònoma de Barcelona, Bellaterra, Spain
- 5ICREA, Barcelona, Spain
We study the random walk of a particle in a compartmentalized environment, as realized in biological samples or solid state compounds. Each compartment is characterized by its length L and the boundaries transmittance T. We identify two relevant spatio-temporal scales that provide alternative descriptions of the dynamics: (i) the microscale, in which the particle position is monitored at constant time intervals; and (ii) the mesoscale, in which it is monitored only when the particle crosses a boundary between compartments. Both descriptions provide—by construction—the same long time behavior. The analytical description obtained at the proposed mesoscale allows for a complete characterization of the complex movement at the microscale, thus representing a fruitful approach for this kind of systems. We show that the presence of disorder in the transmittance is a necessary condition to induce anomalous diffusion, whereas the spatial heterogeneity reduces the degree of subdiffusion and, in some cases, can even compensate for the disorder induced by the stochastic transmittance.
1. Introduction
The characterization of the diffusive behavior in complex environments is crucial in many fields, ranging from biology [1], via physics and chemistry, to geology [2]. Recently, it has been shown that a large number of systems display anomalous diffusion associated to spatial and/or energetic disorder of the environment. Often, the motion of particles in such systems has been shown to be subdiffusive, i.e., 〈x2(t)〉 ~ tσ with anomalous exponent 0 < σ < 1. The characterization of this movement provides important information on the disorder of the media and on the laws governing the system [3]. The advances in this field have been mainly driven by developments in fluorescence microscopy, which enable us to record movies of single particles diffusing in living matter, with a spatial precision of a few nanometers at the millisecond time scale [4].
The presence of barriers that prevent the particles to freely diffuse in the environments is a general mechanism used to explain subdiffusion [5]. Indeed, there exists a plethora of works treating the effect of these barriers in various forms, from local maxima in potential landscapes [6] to thin slices of poorly diffusive materials [7]. Recently, an analytical approach has been proposed for sufficiently regular geometries [8]. Recent experimental observations in cellular biology have shown that the actin cytoskeleton acts as a compartmentalization scaffold for proteins diffusing in the plasma membrane [9, 10], hence stressing the importance of studying the motion in such environments. Moreover, the evidence of the occurrence of ergodic and non-ergodic processes in the diffusion of biomolecules [11] has triggered the description of models in which geometric and energetic disorders coexist [12, 13].
In this article, we study a general barrier model, where a particle performs an unbiased random walk through a complex environment made by a mesh of compartments separated by barriers with random transmittance. A schematic of the system is shown in Figure 1. We show that even though the particle performs a Brownian motion within each compartment, the stochasticity of the barrier's transmittance induces anomalous diffusion for the overall movement. We also explore the effect of the stochasticity in the length of the compartments, showing that it generally increase the anomalous exponent, up to restoring normal diffusion.
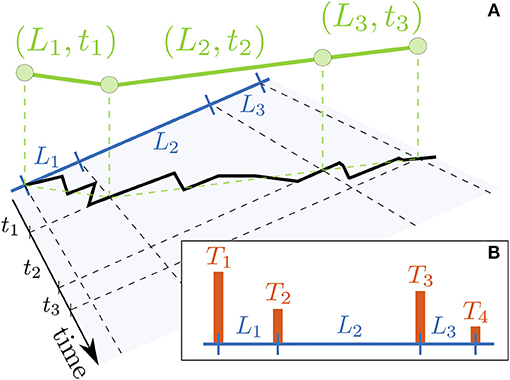
Figure 1. Schematic of the system. (A) Example of a one dimensional compartmentalized environment, with compartment size L and boundary transmittance T. Higher boundaries represent lower transmittance. For simplicity we plot the meshwork as formed by the segments of a line. (B) Motion of the particle in such environment. The dark line represents the microscale description of the motion and the green one indicates its mesoscale description, in this case a Lévy walk with steps given by their length and flight time (L, t).
In order to study the behavior of the particle, we propose a coarse-graining approach transforming the rather complex walk of the particle (mainly due to the interaction with the boundaries) into two very well known theoretical models describing anomalous diffusion: continuous time random walks and Lévy walks. The former, introduced by Montroll and Weiss [14], consists in a random walk where the time between successive steps is a stochastic value. Similarly, a Lévy Walk [15] consists in a random walk where not only the step time is a stochastic variable, but also its length. For a comprehensive introduction to both models, we refer the readers to Klafter and Sokolov [16] and Zaburdaev et al. [17].
In the most general description of our system, we show how the walk of the particle can be mapped into a Lévy walk with rests, where flight times depend on the step size. In our system, the steps and rests are not alternate but have complementary probabilities at each event. We show how the existing theory for a Lévy walk with rests can be extended to study such kind of walk. We determine the relationship between the stochasticity of the environment and the anomalous diffusion of the particle by solving different configurations of our system, characterized by fixed or random compartment sizes and boundary transmittances.
2. Materials and Methods
The motion takes place on an environment characterized by a set of compartments with size , with N ≫ 1 and Li ∈ [1, ∞). We treat the size of the compartments as a stochastic variable, following the probability distribution function (PDF) g(L). The compartments form a meshwork with unbounded connectivity, which we assume to be always sufficiently large such to make very unlikely that the particle returns to the same compartment after leaving it. The boundary between the compartments is partially reflective, i.e., a particle reaching a boundary has a finite probability T of moving through the boundary to the next compartment and a complementary probability R = 1 − T of being reflected. The transmittance of each segment , T ∈ (0, 1] is a random variable drawn from the PDF q(T).
For the sake of simplicity, we focus on the case where the compartments consist in one-dimensional segments (see Figure 1A). The extension of this theory to two- or three-dimensional supports, like circles or spheres, is conceptually straightforward but more elaborated and geometry-dependent, since it requires the determination of the stochastic time that the particle spends in each support. The particle performs an unbiased, discrete, random walk through the environment, temporarily confined between the boundaries until it is transmitted to the next compartment.
The motion of particles in disordered media has been thoroughly studied in the past [18]. The usual approach is to explicitly solve the diffusion equation for the system under study. For instance, such direct approach has been recently applied to subdiffusive particles through the barrier separating two liquids [19]. However, when considering systems like the one presented above, where both the boundary transmittance and compartment length are stochastic variables, the direct approach is complicated and does not lead to exact analytical results. Therefore, we use an alternative method to solve the motion of the particle through such a system. First, we distinguish between a microscale description, in which the position of the particle is monitored at constant times < < L2/D with D being the diffusivity, and a mesoscale description, in which the position is sampled at times subordinated to the exit from a compartment. We note here that, by definition, the asymptotic behavior of the motion of the particle coincides on both scales. Therefore, studying the movement at the mesoscale provides a correct description of the movement at long times.
In the mesoscale description, the microscopic walk of the particle (represented by the black line in the same figure) is reduced to a collection of lengths (Li) and times (ti) traveled to exit the compartments, as shown by the green line of Figure 1B. As a matter of fact, the length traveled by the particle in each step corresponds to the size of the compartment itself. The flight time ti is the stochastic time the particle spent bouncing between the boundaries before being transmitted to next compartment. In our case, this time is related to the transmittance T and length L of the compartment through the conditional probability ϕ(t|T, L). One can then write the joint probability for the particle to be in a compartment of length L and boundary transmittance T at time t as
Once inside a compartment, the particle has two options: leaving through the same boundary through which it entered, or through the opposite one. Since our approach monitors the particle only when exiting a boundary, in the latter case, the particle has traveled a distance equal to the size of the compartment. However, in the former, the particle is not effectively moving, since it occupies the same position when entering and exiting the compartment. This translates into a rest with duration equal to the time taken to exit the compartment. Therefore, after entering each compartment, the particle has a probability of resting φr(L, T) and the complementary probability of walking φw(L, T) = 1 − φr(L, T).
Through this coarse-graining approach, we convert the microscale walk into a Lévy walk with rests, with flight times depending on the jump length [20]. Previous works have extensively studied such kind of walks, both with alternating walks and rests [16] or with an equal probability of resting and walking [17]. However, our system shows a substantial difference, since it displays different probabilities of resting or walking, φr + φw = 1, that can be used to calculate the PDFs of walk [ψw(t)] and rest times [ψr(t)] as
and, in the spirit of Zaburdaev et al. [17], to derive the density of particles at position x and time t in the Fourier–Laplace space
where
Here, P0(x) corresponds to the initial distribution of particles, to the survival probability, i.e., the probability of not jumping until time t, to the PDF of the displacement of the walker during the last uncompleted step, and {f(x)}k to the Fourier transform of f(x). For constant step/rest probabilities, e.g., φw = φr = 1/2, Equation (4) leads to the known result for the Lévy walk with rests [17].
However, when the previous condition is not fulfilled, solving Equation (4) requires the calculation of φw(L, T). A case in which φw(L, T) is easily solvable is when the boundaries are completely transmitting, i.e., q(T) = δ(T − 1). In that case, one finds
For T≠1, obtaining an analytical expression for φw(L, T) is a challenging task [21]. A trick commonly used to avoid this difficulty consists in considering an annealed system [18], i.e., assuming that each time the particle exits a compartment, it reappears at the center of the next one. In this case, the particle will always travel a distance Li/2 to escape the ith-segment, independently on the exit side, hence eliminating the presence of rests. In this case φw(L, T) = 1 ∀L, T and the motion of the particle is then a Lévy walk with flying times depending on the jump length [20]. This is also analogous to the case in which, once the particle enters a compartment, it cannot cross again the same edge it entered from and thus will always travel a distance Li. For this reason, in the following we will refer to this approximation as the osmotic approach, in contrast with the general case that we name non-osmotic.
From now on we will focus on the osmotic approach, which allows for a thorough theoretical description in the different configurations considered. In the osmotic approach, Equation (4) takes the much simpler form
where .
To characterize the motion of the particle, we will use the mean squared displacement (MSD), defined as , which can be rewritten as Massignan et al. [22]
As we will show later through numerical simulations of the microscopic walk, in spite of the simpler description, the osmotic approach displays the same long time behavior as the non-osmotic one.
3. Results
In the following, we will use the method described above to solve the motion of the particle in different configurations of the system. We will first consider the case in which each boundary has a different transmittance, drawn stochastically from the PDF q(T), but all the compartments have equal length. We will then briefly comment about the case in which the stochasticity is only present in the compartment length. Last, we will consider the case where both the length and boundary transmittance are random variables. For each case, we will give the analytical solutions of the mesoscopic walk and compare it to numerical simulations of the microscopic description.
The form of the conditional probability of the exit time given a compartment of size L and transmittance T is common to all the cases. A reasonable assumption based on the Brownian motion is that, independently on the expression of this conditional probability, it should give an average time for exiting a compartment 〈t〉 which depends on the length as L2. We can further assume that the dependence on T is such that 〈t〉∝(L/T)2. We checked that this behavior is consistent with the numerical results for a collection of T and L, finding that the average exit time follows an exponential behavior, ∝exp−tL2/T2, for large L and small T. For all cases numerically considered, even when the distribution did not match an exponential behavior, we found a quadratic dependence on L/T. Therefore, we assume the simplest distribution which produces the expected behavior of the average exit time, which is
This form of the conditional time also has the advantage of simplifying the analytical expressions and, as we discuss below, allows us to correctly model the microscopic motion in all the cases considered. The analytical calculation of this conditional probability falls beyond the scope of this work. We note that previous works have focused in the investigation on the exit time in similar structures [23, 24], but do not provide a useful expression for our particular system nor a practical way to derive it.
We will now consider the case in which the boundaries have all the same transmittance, i.e., , with . We will consider that each compartment has a different length, retrieved from the PDF
Our first step is to calculate the distribution of flight times, which is done by convolving Equation (1) over all possible values of L
Using this result and Equations (1) and (7), we find that 〈 x2(t)〉~t ∀ T, i.e., the particle performs normal diffusion. Therefore, the stochasticity of the compartment length does not imply any effect on the MSD and, indeed, similar results are obtained when using regular compartment size. We would like to emphasize that this result holds for any finite T different from zero. In fact, as shown in Lapeyre [25], for T = 0 subdiffusion occurs. In the presence of transmitting boundary, there is no mechanism that confines the particle for pathologically long times, so particles diffuse normally in the asymptotic limit.
A very different result arises when considering disordered boundary transmittances distributed according to a power law PDF
We first analyze the case in which the compartments have all the same size, i.e., the lengths are distributed according to the PDF , where . We refer to this system as the spatially ordered case. In the osmotic approach, the walk consists on a collection of steps of size with flight times drawn from the PDF
As all the steps have equal length, the walk reduces to a continuous time random walk with waiting time PDF given by Meroz et al. [12]. Thus, in the spatially ordered case the MSD is given by Charalambous et al. [26]
showing that the particle undergoes subdiffusive motion for 0 < α < 1. In Figure 2A we show the numerical results corresponding to MSD calculated for a single value of α = 0.2 and different values of by using the microscale description for the spatially ordered case. The plot shows that the motion is initially Brownian and become subdiffusive at longer times. The time at which the onset of subdiffusion occurs increases as grows, corresponding to the time needed to reach the boundary, of a compartment. The asymptotic value of the MSD for any is given by Muñoz Gil et al. [13]. This is a first indication that anomalous diffusion can only be obtained by considering stochastic boundary transmittance with a heavy-tail PDF. In the spatially ordered case, the distribution of transmittances of the media can be directly inferred from the asymptotic behavior of the MSD of the particle.
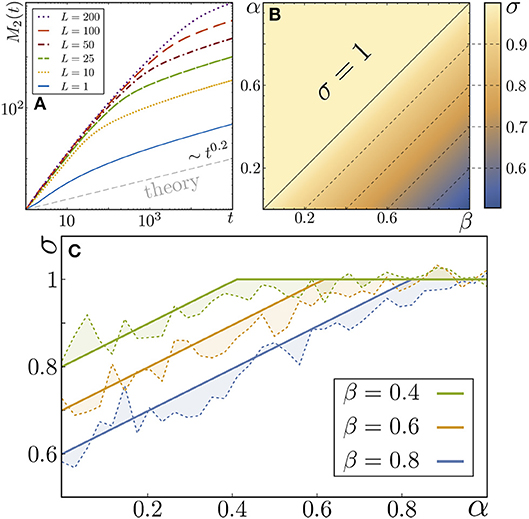
Figure 2. (A) MSD of a particle moving in an system of compartments of equal length and boundary transmittances distributed following (11), with α = 0.2. All curves are calculated for the microscale and tend to the predicted subdiffusive motion given by (13). A larger L leads to a larger time for the onset of subdiffusion to occur. The dashed bottom line corresponds to the mesoscale and coincides with the theoretical prediction. (B) Value of the exponent of the MSD in a system with stochastic compartment sizes and boundary transmittance, given by (15). (C) Comparison between the predicted results of the previous case and numerical simulations of the microscopic walk (dashed lines).
We will now consider the case where both compartment length and boundary transmittance are stochastic variables. As stated before, this situation can be modeled at the mesoscale as a Lévy walk with flight times depending on the step size. We consider that the transmittances are distributed according to Equation (11) and the compartment lengths as described by Equation (9). Following the method used to derive Equation (12), we can calculate the PDF of flight times by convolving the conditional probability ϕ(t|T, L) with Equations (11) and (9), to find
By using the previous result and Equation (1) we can determine the MSD through its Laplace transform as in Equation (7). In the time domain we find
The values of the MSD exponent obtained for different values of α and β are shown in Figure 2B. In Figure 2C we further show the values of the MSD exponent calculated from numerical simulations for the microscale description of the walk (dashed lines) and the theoretical value given by Equation (15). The numerical calculation and the theoretical prediction show a good agreement. It can be noticed that, when α > β (and thus γ = β), the particle movement is normally diffusive (see Equation (15) and Figures 2B,C). Therefore, the stochasticity in the length of the segments is capable of compensating for the disorder that would be induced by the stochasticity in transmittance, that would generate a subdiffusive motion with anomalous exponent σ = α/2 in the case the segments lengths were regular. In addition, for β > α, the motion is subdiffusive, but with a higher anomalous exponent as compared to the case in which the lengths were regular. Therefore, in this case the two disorders compete, producing a weaker subdiffusion.
4. Discussion
In this article, we introduce a coarse-graining method that we use to study diffusion through complex environments. This method is useful to study systems in which the microscopic behavior of the particles is too involved to be described analytically. To obtain a description of the motion in such cases, we propose a procedure that allows one to transform the microscopic walk into well-known theoretical models, such as Lévy Walks or continuous time random walks. The coarse-grained transformation maps the original walk performed at the microscale into a simplified movement at a larger scale (which we term mesoscale) that captures the relevant properties of the environment. This allows for a complete analytical characterization of the diffusion in terms its observables, such as the mean square displacement.
To illustrate the use of the proposed method, we consider the diffusion in an environment consisting of compartments with random sizes and/or transmittances. To resolve the diffusion of the system at the microscale, one needs to consider the complex interaction of the particle with the boundary of each compartment. For some simple systems, e.g., when all the compartments have the same size, it is possible to get an analytical solution of the microscale motion. In this cases, we show that a heavy-tailed distribution of boundary transmittance is a necessary requirement to induce subdiffusion. However, for more intricate spatially-disordered environments, it is often difficult to obtain an analytical solution at the microscale. This is the scenario where our method allows to get insights on the motion while neglecting microscopic details. As an example, we demonstrate that when the compartments length is a stochastic variable, geometric disorder alone cannot generate subdiffusion. However, it can affect the one generated by the heterogeneity in the boundary transmittance. Namely, increasing the geometric disorder reduces the degree of subdiffusion, as it increases the value of the anomalous exponent toward one. We thus fully characterize the mean-square displacement exponent as a function of the parameters controlling the heavy-tailed distributions of both the lengths and barrier heights.
The model presented in this article might be a useful framework to interpret diffusion in a variety of systems composed of compartment of varying size and barriers. A striking example of such kind of system is provided by eukaryotic cells, highly compartmentalized at different spatial scales to provide optimal conditions to perform specific functions [27]. The presence of compartments has been shown to affect the diffusion of transmembrane proteins in the plasma membrane, e.g., as a consequence of a self-similar actin network acting as semipermeable barrier [9].
An interesting outlook of our model could consist in the possibility of its further generalization, as to include previously proposed models for diffusion in complex environment. For example, our approach shares important features with the previously proposed comb model [28]. In fact, the comb model can be considered as a continuous-time random walk with stochastic waiting time, the latter derived from first-passage time. This system can be analyzed through our coarse-grained approach upon conversion of the waiting time distribution into a stochastic transmittance. The realization of the comb model including convective terms [29], could be further implemented in our approach, e.g., through the use of asymmetric transmittance.
Author Contributions
All authors contributed conception and design of the study. GM-G, AC, and MG-M developed the theory. GM-G performed the simulations. GM-G, MG-M, and CM wrote the paper. ML supervised research. All authors contributed to manuscript revision, read and approved the submitted version.
Funding
This work has been funded by the Spanish Ministry MINECO (National Plan15 Grant: FISICATEAMO No. FIS2016-79508-P, SEVERO OCHOA No. SEV-2015-0522, FPI), European Social Fund, Fundació Cellex, Generalitat de Catalunya (AGAUR Grant No. 2017 SGR 1341 and CERCA/Program), ERC AdG OSYRIS, EU FETPRO QUIC, and the National Science Centre, Poland-Symfonia Grant No. 2016/20/W/ST4/00314. CM acknowledges funding from the Spanish Ministry of Economy and Competitiveness and the European Social Fund through the Ramón y Cajal program 2015 (RYC-2015-17896) and the BFU2017-85693-R, and from the Generalitat de Catalunya (AGAUR Grant No. 2017SGR940). AC acknowledges financial support from the ERC Synergy Grant UQUAM and the SFB FoQuS (FWF Project No. F4016-N23). GM acknowledges financial support from Fundació Social La Caixa. AC acknowledges support from the UAB Talent Research program and from the Spanish Ministry of Economy and Competitiveness under Contract No. FIS2017-86530-P.
Conflict of Interest Statement
The authors declare that the research was conducted in the absence of any commercial or financial relationships that could be construed as a potential conflict of interest.
Acknowledgments
We acknowledge Oriol Rubies for the initial numerical exploration of the problem and John Lapeyre and Vasily Zaburdaev for inspiring and useful discussions.
References
1. Tan P, Liang Y, Xu Q, Mamontov E, Li J, Xing X, et al. Gradual crossover from subdiffusion to normal diffusion: a many-body effect in protein surface water. Phys Rev Lett. (2018) 120:248101. doi: 10.1103/PhysRevLett.120.248101
2. Berkowitz B, Cortis A, Dentz M, Scher H. Modeling non-Fickian transport in geological formations as a continuous time random walk. Rev Geophys. 44:RG2003. (2006) doi: 10.1029/2005RG000178
3. Metzler R, Jeon JH, Cherstvy AG, Barkai E. Anomalous diffusion models and their properties: non-stationarity, non-ergodicity, and ageing at the centenary of single particle tracking. Phys Chem Chem Phys. (2014) 16:24128–164. doi: 10.1039/C4CP03465A
4. Manzo C, Garcia-Parajo MF. A review of progress in single particle tracking: from methods to biophysical insights. Rep Prog Phys. (2015) 78:124601. doi: 10.1088/0034-4885/78/12/124601
6. Bernasconi J, Beyeler HU, Strässler S, Alexander S. Anomalous frequency-dependent conductivity in disordered one-dimensional systems. Phys Rev Lett. (1979) 42:819. doi: 10.1103/PhysRevLett.42.819
7. Novikov DS, Fieremans E, Jensen JH, Helpern JA. Random walks with barriers. Nat Phys. (2011) 7:508. doi: 10.1038/nphys1936
8. Moutal N, Grebenkov D. Diffusion across semi-permeable barriers: spectral properties, efficient computation, and applications. arXiv preprint arXiv:180706336 (2018).
9. Sadegh S, Higgins JL, Mannion PC, Tamkun MM, Krapf D. Plasma membrane is compartmentalized by a self-similar cortical actin meshwork. Phys Rev X. (2017) 7:011031. doi: 10.1103/PhysRevX.7.011031
10. de Wit G, Albrecht D, Ewers H, Kukura P. Revealing compartmentalized diffusion in living cells with interferometric scattering microscopy. Biophys J. (2018) 114:2945–50. doi: 10.1016/j.bpj.2018.05.007
11. Weigel AV, Simon B, Tamkun MM, Krapf D. Ergodic and nonergodic processes coexist in the plasma membrane as observed by single-molecule tracking. Proc Natl Acad Sci USA. (2011) 108:6438–43. doi: 10.1073/pnas.1016325108
12. Meroz Y, Sokolov IM, Klafter J. Subdiffusion of mixed origins: when ergodicity and nonergodicity coexist. Phys Rev E. (2010) 81:010101. doi: 10.1103/PhysRevE.81.010101
13. Muñoz Gil G, Charalambous C, García-March MA, Garcia-Parajo MF, Manzo C, Lewenstein M, et al. Transient subdiffusion from an Ising environment. Phys Rev E. (2017) 96:052140. doi: 10.1103/PhysRevE.96.052140
15. Shlesinger M, Klafter J, Wong Y. Random walks with infinite spatial and temporal moments. J Stat Phys. (1982) 27:499–512. doi: 10.1007/BF01011089
16. Klafter J, Sokolov IM. First Steps in Random Walks: From Tools to Applications. Oxford: Oxford University Press (2011).
17. Zaburdaev V, Denisov S, Klafter J. Lévy walks. Rev Mod Phys. (2015) 87:483. doi: 10.1103/RevModPhys.87.483
18. Bouchaud JP, Georges A. Anomalous diffusion in disordered media: statistical mechanisms, models and physical applications. Phys Rep. (1990) 195:127–293. doi: 10.1016/0370-1573(90)90099-N
19. Kosztołowicz T, Wasik S, Lewandowska KD. How to determine a boundary condition for diffusion at a thin membrane from experimental data. Phys Rev E. (2017) 96:010101. doi: 10.1103/PhysRevE.96.010101
20. Zaburdaev VY. Random walk model with waiting times depending on the preceding jump length. J Stat Phys. (2006) 123:871–81. doi: 10.1007/s10955-006-9104-0
21. Lehner G. One dimensional random walk with a partially reflecting barrier. Ann Math Stat. (1963) 34:405–12. doi: 10.1214/aoms/1177704151
22. Massignan P, Manzo C, Torreno-Pina J, García-Parajo M, Lewenstein M, Lapeyre G Jr. Nonergodic subdiffusion from Brownian motion in an inhomogeneous medium. Phys Rev Lett. (2014) 112:150603. doi: 10.1103/PhysRevLett.112.150603
23. Khantha M, Balakrishnan V. First passage time distributions for finite one-dimensional random walks. Pramana. (1983) 21:111–22. doi: 10.1007/BF02894735
24. Dybiec B, Gudowska-Nowak E, Hänggi P. Lévy-Brownian motion on finite intervals: Mean first passage time analysis. Phys Rev E. (2006) 73:046104. doi: 10.1103/PhysRevE.73.046104
25. Lapeyre GJ Jr. Anomalous diffusion from Brownian motion with random confinement. arXiv preprint arXiv:150407158 (2015).
26. Charalambous C, Munoz-Gil G, Celi A, Garcia-Parajo M, Lewenstein M, Manzo C, et al. Nonergodic subdiffusion from transient interactions with heterogeneous partners. Phys Rev E. (2017) 95:032403. doi: 10.1103/PhysRevE.95.032403
27. Trimble WS, Grinstein S. Barriers to the free diffusion of proteins and lipids in the plasma membrane. J Cell Biol. (2015) 208:259–71. doi: 10.1083/jcb.201410071
28. Weiss G, Havlin S. Some properties of a random walk on a comb structure. Phys A. (1986) 134:474–82. doi: 10.1016/0378-4371(86)90060-9
Keywords: random walk, anomalous diffusion, stochastic processes, complex systems, barriers
Citation: Muñoz-Gil G, Garcia-March MA, Manzo C, Celi A and Lewenstein M (2019) Diffusion Through a Network of Compartments Separated by Partially-Transmitting Boundaries. Front. Phys. 7:31. doi: 10.3389/fphy.2019.00031
Received: 20 November 2018; Accepted: 21 February 2019;
Published: 18 March 2019.
Edited by:
Carlos Mejía-Monasterio, Polytechnic University of Madrid, SpainReviewed by:
Diego R. Amancio, University of São Paulo, BrazilHaroldo Valentin Ribeiro, Universidade Estadual de Maringá, Brazil
Copyright © 2019 Muñoz-Gil, Garcia-March, Manzo, Celi and Lewenstein. This is an open-access article distributed under the terms of the Creative Commons Attribution License (CC BY). The use, distribution or reproduction in other forums is permitted, provided the original author(s) and the copyright owner(s) are credited and that the original publication in this journal is cited, in accordance with accepted academic practice. No use, distribution or reproduction is permitted which does not comply with these terms.
*Correspondence: Miguel Angel Garcia-March, miguel.garcia-march@icfo.eu
Carlo Manzo, carlo.manzo@uvic.cat