- 1Institute of Physics, Universidade de Brasília, Brasília, Brazil
- 2Centro de Ciências Exatas e Tecnológicas, Universidade Federal do Recôncavo da Bahia, Cruz das Almas, Bahia, Brazil
- 3Interdisciplinary Center for Natural Sciences, UNILA, Foz do Iguaçu, Brazil
- 4Institute of Physics, Universidade Federal do Rio Grande do Sul, Porto Alegre, Brazil
- 5Physics of Livings Systems, Department of Physics, Massachusetts Institute of Technology, Cambridge, MA, United States
In this article we review classical and recent results in anomalous diffusion and provide mechanisms useful for the study of the fundamentals of certain processes, mainly in condensed matter physics, chemistry and biology. Emphasis will be given to some methods applied in the analysis and characterization of diffusive regimes through the memory function, the mixing condition (or irreversibility), and ergodicity. Those methods can be used in the study of small-scale systems, ranging in size from single-molecule to particle clusters and including among others polymers, proteins, ion channels and biological cells, whose diffusive properties have received much attention lately.
“In water, this flowing matter, there is nothing permanent”
Jan Heweliusz
1. Introduction
1.1. General Concepts
Diffusion is a basic transport process involved in the evolution of many non-equilibrium systems toward equilibrium [1–13]. By diffusion, particles (or molecules) spread from regions of high concentration to those of low concentration leading, via a gradual mixing, to a situation in which they become evenly dispersed. Diffusion is of fundamental importance in many disciplines; for example, in growth phenomena, the Edwards-Wilkinson equation is given by a diffusion equation plus a noise [14]. In cell biology it constitutes a main form of transport for amino acids and other nutrients within cells [15].
For more than 200 years [1], diffusion has been a widely studied phenomena in natural science due to it's large number of applications. Initially, the experiments carried out by Robert Brown [16, 17] called the attention to the random trajectories of small particles of polen and also of inorganic matter. This irregular motion, later named Brownian motion, can be modeled by a random walk in which the mean square displacement is given by Einstein's relation [1]
where Δr is the displacement of the Brownian particle in a given time interval t, d is the spatial dimension, and D the diffusion coefficient. Whereas a single Brownian particle trajectory is chaotic, averaging over many trajectories reveals a regular behavior.
The purpose of this article is to review recent efforts aiming at formulating a theory for anomalous diffusion processes– i.e., those where the mean square displacement does not follow Equation (1) which can be applied to many different situations from theoretical physics to biology.
In the next section, we introduce the pioneering works on diffusion, and then call attention to the existence of anomalous diffusion. We discuss the main methods to treat anomalous diffusion and concentrate our efforts on the discussion of the generalized Langevin formalism.
1.2. The Tale of the Three Giants
At the birth of the gravitational theory Isaac Newton mentioned that he build up his theory based on the previous works of giants. Also, one cannot talk about diffusion without underscoring the works of Albert Einstein, Marian Smoluchowski, and Paul Langevin. At the dawn of the last century, the atomic theory was not widely accepted by the physical community. Einstein believed that the motion observed by Brown was due to the collisions of molecules such as proposed by Boltzmann in his famous equation. However, Boltzmann's equation was difficult to solve and therefore he proposed a simpler analysis combining the kinetic theory of molecules with the Fick's law, from which he obtained the diffusion equation
where ρ(x, t) is the density of particles at position x and time t. The solution of the above equation yields ρ(x, t) and
where 〈x(t)〉 = 0, assuming the symmetry ρ(−x, t) = ρ(x, t). For simplicity, we have considered the motion one-dimensional and set all particles at the origin at time t = 0. Generalization to two and three dimensions is straightforward. He then considered the molecules as single non-interacting spherical particles with radius a and mass m, and subject to a friction γ when moving in the liquid [1, 18, 19].
Finally, he deduced the famous Einstein-Stokes relation [19] for the diffusion constant
where Na is Avogadro's number, fundamental for atomic theory, but unknown at the time, R is the gas constant, μ the mobility, η the viscosity, and T the temperature.
The scientific community became very excited and, in the years following Einstein's papers, some dedicated experiments helped to verify Equation (3). Diffusion constants were measured and it became possible to estimate Avogadro's number from different experiments. Moreover, it was possible to estimate the radius of molecules with few hundreds of atoms. Einstein was successful in demonstrating that atoms and molecules were not mere illusions as critics used to suggest. Finally, the theory of Brownian motion started to set a firm ground. For instance, it became possible to associate diffusion with conductivity in the case of a gas of charged particles. Supposing each has the same charge e and is subjected to a time dependent electric field , the conductivity can be defined by , where is the current. Now, it was possible to relate the diffusivity with by the relation [1, 20, 21]
where is n the carrier density. From that it was obvious that connections between a diversity of response functions could be obtained.
Two major achievements in the theory of stochastic motion were due to Smoluchowski. As expressed by Novak et al. [22], “One was the Smoluchowski equation describing the motion of a diffusive particle in an external force field, known in the Western literature as the Fokker-Planck equation [23, 24]”
where
and
Here P(v′, δt, v) is the transition probability between two states with different velocities. The second one, a fundamental cornerstone of molecular physical chemistry and of cellular biochemistry [22, 25] is “Smoluchowski's theory of diffusion limited coagulation of two colloidal particles.” Unfortunately, due to his premature death, the Nobel prize was not awarded to Smoluchowski. However, the scientific community pays tribute to him [26].
The last of the three giants was Langevin, who considered Newton's second law of motion for a particle as [27]
dividing the environment's (thermal bath) influence into two parts: a slow dissipative force, −mγv, with time scale τ = γ−1, and a fast random force f(t), which changes in a time scale Δt≪τ, subject to the conditions
and
If we solve Equation (9) and, using the equipartition theorem, impose , where kB = R/Na is the Boltzmann constant, we obtain Λ = 2mγkBT and write
This last equation establishes a relation between the fluctuation and the dissipation in the system reconnecting the useful, although artificial, separation of the two forces. This relation has been named the fluctuation-dissipation theorem (FDT) and is one the most important theorems of statistical physics. Equations (10, 11, 13) yield the velocity-velocity correlation function that reads [28]
the mean square displacement
and
known as the Kubo formula. This bring us back to Einstein's results for diffusion, Equation (4).
The simplification introduced by the Langevin formalism makes it easy to carry out analytical calculations and computer simulations. Consequently, the Langevin equation, and its generalization (Section 3), has been applied successfully to the study of many different systems such as chain dynamics [29–34], liquids [35, 36], ratchets [37, 38], and synchronization [39, 40]. However, its major importance was to relate fluctuation with dissipation.
The Fokker-Planck Equation (6) with the Langevin choices becomes
known as the Ornstein-Uhlenbeck equation. It obviously yields the same result as that of Einstein and Langevin. This completes the tale of the three giants. Their work established the bases of non-equilibrium statistical mechanics opening a new field in research for the next decades. For example, it was demonstrated that hydrodynamics could be obtained from the Boltzmann equation in particular situations [41], so that the physics community could appreciate the work of yet another giant.
2. Breakdown of the Normal Diffusive Regime
2.1. The Different Facets of the Anomaly: Subdiffusion and Superdiffusion
Inspection of different supposedly diffusive processes such as enhanced diffusion in the intracellular medium [42], cell migration in monolayers [43], Levy flight search on a polymeric DNA [44], or the Brownian motion in an inhomogeneous medium [45] reveals that the previous framework is not always fulfilled. Instead, in these and other similar examples the mean square displacement deviates from the linear temporal evolution. One of the most common anomalous behaviours is given by
where α ≠ 1 is a real positive number [4–6, 46].
The origin of this discrepancy is the tacit assumption made in the derivation of (1) that the Brownian particle moves in an infinite structureless medium acting as a heat bath. This assumption is generally incorrect when the Brownian motion takes place in a complex medium, as is the case of the previously mentioned examples. We illustrate in Figure 1 the mean square displacement 〈x2(t)〉 as a function of t for three distinct Brownian motions in one dimension. From the upper curve downwards, we have α = 1.5 (superdiffusion), α = 1 (normal diffusion), and α = 0.5 (subdiffusion).
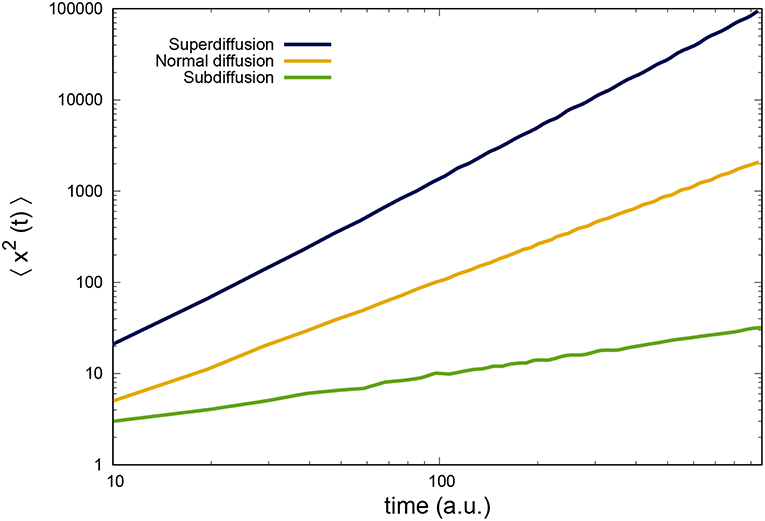
Figure 1. (Color) Time evolution of the mean square displacement (in arbitrary units) for normal and anomalous diffusion. For the upper curve α = 1.5 (superdiffusion), for the middle curve α = 1 (normal diffusion) and for the lower curve α = 0.5 (subdiffusion). From Lapas et al. [9], with permission from Physical Review Letters.
The recent interest in the study of complex systems has led to an increased focus on anomalous diffusion [4–7, 36, 42, 45], which has been described in biological systems [42–44], protostellar birth [47], complex fluids [48–53], electronic transportation [54–59], porous media and infiltration [60–62], drug delivery [63–66], fractal structures and networks [67–71], and in water's anomalous behavior [72–74], phase transitions in synchronizing oscillators [39, 40, 75–77], to name a few.
There are many formalisms that describe anomalous diffusion, ranging from thermodynamics [78–80], and fractional derivatives [4, 6] to generalized Langevin equations (GLE) [81–83]. The goal of this short review is to call attention to relevant research within the GLE framework and to some fundamental theorems in statistical mechanics.
3. The Generalized Langevin Equation Approach
3.1. Non-markovian Processes
The Langevin formalism within its classical description has some restrictions:
1. It has a relaxation time τ = γ−1, and an unspecified Δt ≪ τ, while a diffusive process in a real system can present several time scales;
2. For short times t < Δt, predictions are unrealistic; for example, the derivative of Cv(t) is zero [84] at t = 0, while in Langevin's formalism Cv(t) = exp(−γ|t|), exhibiting a discontinuity.
3. If no external field is applied it cannot predict anomalous diffusion.
Up to now we have used time and ensemble averages without distinction, i.e. we implicitly used the Boltzmann Ergodic hypothesis (EH). The ensemble average for a variable B(t) is defined by
where Ω(E, B) is the number of states for a given energy E. For the time average we have
For times τ0 ≫ τ, the Ergodic Hypothesis (EH) reads
which means that the system should be able to reach every accessible state in configuration space given enough time. This is expected to be true for equilibrated macroscopic systems and also for systems that suffer small perturbations close to equilibrium.
In this section, we generalize Langevin‘s equation and study some of its consequence for diffusion. The first correction to the FDT was done by Nyquist [85] who formulated a quantum version of the FDT. Later on Mori [86, 87], and Kubo [88] used a projection operator method to obtain the equation of motion, the Generalized Langevin equation (GLE),
for a dynamical operator A(t), where Π(t) is a non-Markovian memory and F(t) is a random variable subject to
1. ,
2. , and,
3. the Kubo fluctuation-dissipation theorem (FDT) [89, 90]
In this way, it is clear that time translational invariance holds in the Kubo formalism. An alternative to the projection operators, the recurrence relation method, was derived by Lee [84, 91, 92], and Lee and Hong [93].
It is easy to show that Equation (22) can give rise both to normal and to anomalous diffusion [1]. Let us consider two limiting case examples: first, when Π(t) = 2γδ(t), we recover the normal Langevin equation (9) with normal diffusion (α = 1); second, for a constant memory Π(t) = K, Equation (22) becomes
where
Equation (24) is the equation of motion for a harmonic oscillator with zero diffusion constant (D = 0) and with exponent α = 0. Consequently, we may have different classes of diffusion for distinct memories.
Now, we can study the asymptotic behavior of Equation (25) or of its second moment, Equation (18), to characterize the type of diffusion presented by the system for any memory Π(t). Much information about the system's relaxation properties can be obtained by studying the correlation function
The existence of stationary states warrants time-translation invariance so that the the two-time correlation function becomes a function only of the difference between two times, such as in the Kubo FDT above. We shall return to this point later. The main equation we are interested in is Equation (1), where for anomalous diffusion, D should be replaced by D(t) now defined by then
From here onwards, the tilde over the function stands for the Laplace transform. For the last equality, we use the final-value theorem for Laplace transforms [94], which states that if f(t) is bounded on (0, ∞) and has a finite limit, then . Also note that the Laplace transform of the integral of a function is the Laplace transform of the function divided by z, then . Using z ∝ 1/t, we obtain the asymptotic behavior of D(t). In order to do that, we multiply Equation (22) by A(0), take the average and use the conditions (1) and (2) above for the noise to obtain the self-consistent equation
where for simplicity we have defined
Note that from Equation (22), we need to average from a large number of stochastic trajectories, while to obtain Equation (29), it is only necessary to solve a single equation, i.e., Equation (28). Further insight can be gained by analyzing the Laplace transformed version of Equation (28)
From here, it is clear that the knowledge of in the limit z → 0 completely defines the asymptotic dynamics. For instance, if then Equation (27) becomes
and consequently [5]
where
We see that we have a cutoff limit for the exponent β and, therefore, also for α.
Due to the existence of correlations in the GLE that arise through hydrodynamical interactions [28], it has been proposed [5, 46, 95] to establish a connection between the random force F(t) and the noise density of states ρ(ω) of the surrounding media, modeled as a thermal bath of harmonic oscillators [5, 81] of the form
where 0 < ϕ < π are random phases. Now, using the Kubo FDT, Equation (23), and time averaging over the cosines, we obtain the memory as [5, 8]
which is an even function independent of the noise distribution. Here, .
The consequences of considering a colored noise given by a generalization of the Debye spectrum
with ωs as a Debye cutoff frequency were analyzed in detail in Vainstein et al. [96]. The reason for the choice of this functional form for the noise density of states is that it was previously shown in Morgado et al. [5] and Costa et al. [8] that if as z → 0, then the same restriction as in Equation (33), with ν = μ, applies and the diffusion exponent [5] is given by Equation (32).
Later, this problem was revisited by Ferreira et al. [97] in which a generalized version of Equation (18) was considered, namely
Most authors [4–6, 46] have reported the cases of anomalous diffusion where, n = 0 and α ≠ 1. However, some authors such as for example, Srokowksi [98, 99] reports situations were for t → ∞, the dispersion behaves as
i.e., a weak subdiffusive behavior for which we can say that α = 1−. In this way Ferreira et al. [97] generalizes the concept of α, to associate with Equation (37), the α± exponents, which arise analogously to the critical exponents of a phase transition [100–102]. For example, in magnetic systems with temperatures T close to the transition temperature Tc, the specific heat at zero field, H = 0, exhibits the power law behavior , where α is the critical exponent. However, for the two-dimensional Ising model [100] the critical exponent can be considered α = 0+, since the specific heat behaves logarithmically, CH = 0 ∝ ln |T − Tc|, instead. Logarithmic corrections [102] to scaling have also been applied to the diluted Ising model in two dimensions in Kenna and Ruiz-Lorenzo [103]. This generalized nomenclature is pertinent because there are many possible combinations of both logarithmic and power law behaviors. This result highlights the existence of different types of diffusion.
In this way, for the density of states (36), the generalized α becomes
In Equation (36), we choose the constant such that for normal diffusion . The exponent for Ballistic diffusion (BD), α = 2, is the maximum for diffusion in the absence of an external field. The slow ballistic motion α = 2− has properties that differ markedly from the ballistic case, see sections (3.3 and 3.4).
3.2. Non-exponential Relaxation
Besides the importance of the asymptotic behavior, the study of the correlation R(t) for finite times is also obviously significant, and there exists a vast literature describing non-exponential behavior of correlation functions in systems ranging from plasmas to hydrated proteins [104–112], since the pioneering works of Rudolph Kohlrausch [113] who described charge relaxation in Leyden jars using stretched exponentials, R(t) ≈ exp [−(t/τ)β] with 0 < β < 1, and his son Friedrich Kohlrausch [114], who observed two universal behaviors: the stretched exponential and the power law. Since many features are shared among such systems and those that present anomalous diffusion [95, 115], it is natural that similar methods of analysis can be applied to both. For example, from Equation (28), must be zero at t = 0, which is at odds with the result R(t) = exp(−γ|t|) of the memoryless Langevin equation. Nevertheless, we know that the exponential can be a reasonable approximation in some cases: Vainstein et al. [96] have presented a large diversity of correlation functions that can be obtained from Equation (28) once Π(t) is known. Since, from Equation (35), Π(t) is an even function then we can write
From Equation (28), they proved that R(t) must also be an even function, therefore
with a0 = R(0) = 1. We insert Equations (40) and (41) into Equation (28) to obtain the recurrence relation [96]
which shows the richness and complexity of behavior that can arise from a non-Markovian model. The above defined convergent power series represents a large class of functions, including the Mittag-Leffler function [116] which behaves as a stretched exponential for short times and as an inverse power law in the long time scale. Note that even for the simplest case of normal diffusion, ν = 0, R(t) is not an exponential since at the origin its derivative is zero; however, for broad-band noise ωs ≫ γ, i.e., in the limit of white noise it approaches the exponential R(t) = exp(−γt), for times larger than .
In Figure 2, from Vainstein et al. [96], we display the rich behavior of the correlation function R(t) for the case of normal diffusion. Here, γ = 1 and ωs = 2 and 20, for curves a and b, respectively. Curve c is the plot of exp(−γt). In the inset, we highlight that although curve b and the exponential approach one another for long times, for short times they differ appreciably. Also plotted is cos(ω0t), with .
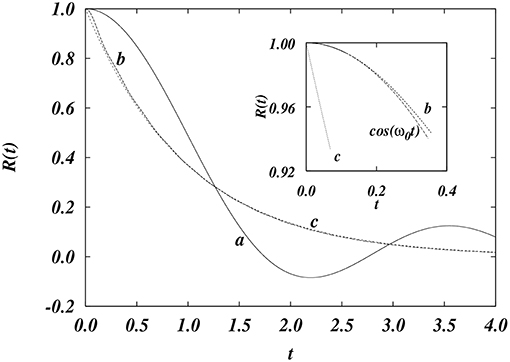
Figure 2. Plot of the correlation function R(t) for normal diffusion (ν = 0). Here, γ = 1 and ωs = 2 and 20, for curves a and b, respectively. Curve c is the plot of exp(−γt). Note that curves b and c are indistinguishable, except near the origin (see inset). Inset: Plot of curves b, c and cos(ω0t), near the origin. From Vainstein et al. [1], with permission from Europhysics Letters.
3.3. Basic Theorems of Statistical Mechanics
3.3.1. The Decay Toward an Equilibrium State
One of the most important aspects of dynamics is to observe the asymptotic behavior of a system or how it approaches equilibrium (or not). Observe that a direct solution for Equation (22) is
Given the initial states A(0), it is possible to average over many trajectories to obtain the temporal evolution of the moments , with n = 1, 2, …. The first moment arises directly from an average of Equation (43),
Taking the square of Equation (43) and averaging, we obtain
The skewness is defined as a measure of the degree of asymmetry of the distribution of A(t), and is given by [83]
where . We also obtain the non-Gaussian factor [83]
Consequently, we see that R(t) completely determines these averages. One can note that if the system is originally at equilibrium and , then the system remains in equilibrium. If the system is not in equilibrium, then
drives the system toward equilibrium. The condition stated in Equation (48) is called the mixing condition (MC) and is a fundamental concept in statistical mechanics, which asserts that after a long time the system reaches equilibrium and forgets all initial conditions.
Note that parity is conserved as well: if the initial skewness is null, ζ(0) = 0 in Equation (46), it will remain null during the whole evolution; the same holds for the nongaussian factor.
3.3.2. The Kinchin Theorem and Ergodicity
The Khinchin theorem (KT) [9, 117] states that if the the MC holds, then ergodicity holds. As shown below, anomalous diffusion is a good field for testing ergodicity breaking [9, 10, 83]. For a situation where the MC is violated, we have
where κ is the nonergodic factor [8, 9, 38, 118]. For example for , α = 2, and
I.e., the MC is violated in the ballistic motion, and consequently ergodicity is violated, but not the KT. Note that for α = 2−, where R(t → ∞) → 1/ln(t) → 0, the MC is not violated. In this way the MC is satisfied for all diffusive processes in the range 0 < α < 2− [97].
It is interesting to observe that Equation (49) for long time behavior is equivalent to the condition
for systems with long range interactions [119, 120], where is the potential between the particles and the integration is performed over all space.
3.3.3. Gaussianization
As an illustration of the analytical results, we numerically integrated the GLE, Equation (22), to approximate the particle velocity distribution function, using Equations (35, 36) to generate the memory for ν = −0.5, 0, and 0.5, which by Equation (39) give α = 0.5, 1, and 1.5, respectively. The results are exhibited in Figure 3, from Lapas et al. [9], where we show the probability distribution functions as function of the momentum p. From left to right, we have subdiffusion (ν = −0.5), normal diffusion (ν = 0), and superdiffusion (ν = 0.5) for the values . A value ωs = 0.5 was used for normal and superdiffusion. In the case of subdiffusion, a broader noise ωs = 2 is needed for it to arrive at the stationary state. It is expected that R(t → ∞) = 0 in all cases, and that the EH will be valid even for the subdiffusion (superdiffusion). It should be noted that despite large fluctuations in the time average, there is a good agreement between the ensemble and time distributions, in agreement with Equations (44–48), indicating the validity of the EH. In all cases, the distributions converge to the expected Maxwell-Boltzmann distribution, in accordance with analytical results [83].
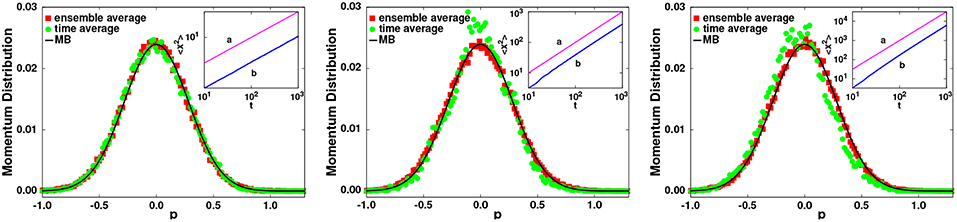
Figure 3. (Color online) Numerical results for the probability distribution function for subdiffusion (left, α = 0.5), normal diffusion (middle, α = 1) and superdiffusion (right, α = 1.5). The time averages (circles) are obtained by following one particle trajectory and calculating the histogram for times from t = 100 to t = 5000. For the ensemble averages (squares), we calculate the histogram using 5 · 104 particles, at time t = 1000. The continuous line is the Maxwell-Boltzmann distribution. Insets: Curves a correspond to the functions tα and curves b to the simulated mean square displacements. From Lapas et al. [9], with permission from Physical Review Letters.
In Figure 4 we show the evolution of the nongaussian factor. We see that for the Ballistic diffusion (BD), it does not reach a null value, but it evolves toward it. Note that even for a situation where κ ≠ 0, the nongaussian factor will be very small and the probability of it being non-zero in simulations after a long time is very small. For example for κ = 0.1, there will a factor of 10−4 in relation (47).
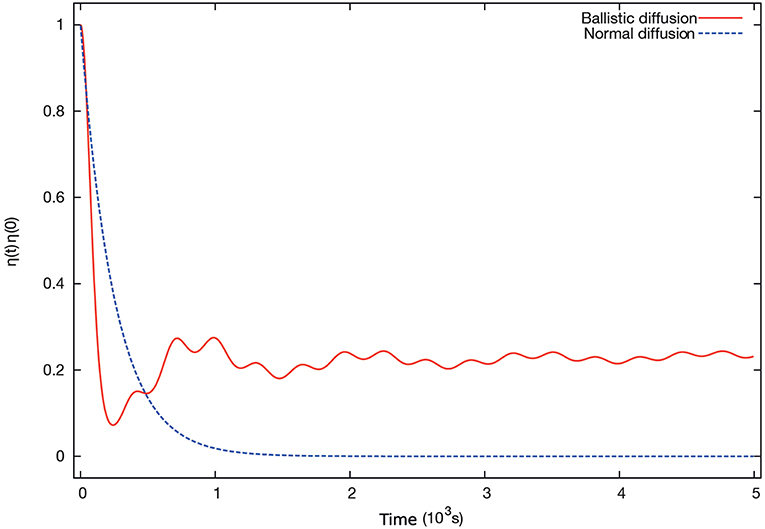
Figure 4. (Color online) Time evolution of the normalized non-Gaussian factor η(t)/η(0) for normal and ballistic diffusion. In both cases, we consider the initial PDF as a Laplace distribution with unit mean and unit variance. The functions R(t) are as in Figure 5. From Lapas et al. [83], with permission from Europhysics Letters.
In Figure 5, from Lapas et al. [83], we show the evolution of the kinetic temperature of the system by taking A2(t), with A = P, the momentum of the particles. In this case we should have [83] and the temperature evolution can be obtained from Equation (45). We consider both normal and ballistic diffusion (BD) in a reservoir characterized by T = 1.0 with initial high and low temperatures T0 = 1.5 and T0 = 0.5, respectively. For normal diffusion (dashed curve), we have R(t) = exp(−γt), with γ = 10−3. For BD, R(t) is calculated numerically in Lapas et al. [83] with γ = 1. Since BD's relaxation is slow, we take a “friction” γ a thousand times larger than that of normal diffusion for comparison. As expected, in the case of normal diffusion the system's temperature always relaxes to that of the reservoir, while for BD the temperature approaches that of the reservoir without reaching it Lapas et al. [83, 115]. Figure 4 displays the normalized non-Gaussian factor, Equation (47), as a function of time [83] for the cases in the previous figure, with the same convention for the labeling. For normal diffusion, the system's probability distribution evolves toward a Gaussian, which is not the case for BD. In the latter case, R(t) oscillates around the value predicted by Equation (50), even for long times, In both cases, the initial probability distribution function was the Laplace distribution, with 〈A2(0)〉 = 1 and 〈A(0)〉 = 0.
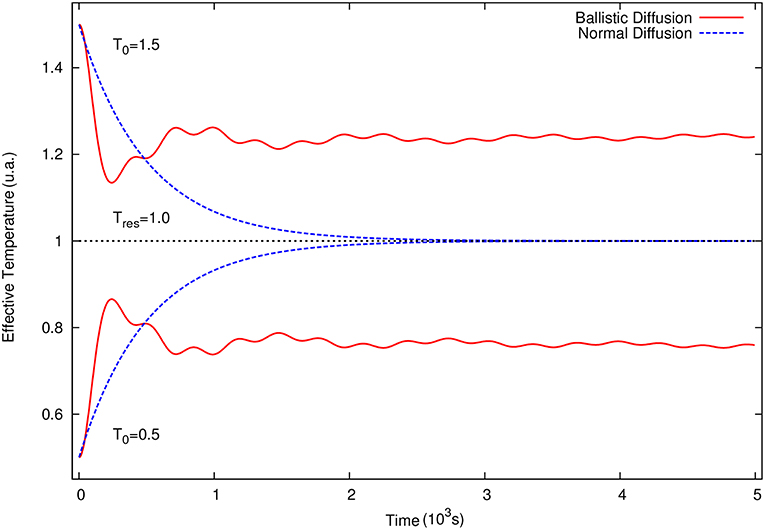
Figure 5. (Color) Time evolution of the effective kinetic temperature (in arbitrary units) for normal and ballistic diffusion. For BD we use the noise distribution ρ(ω) = 2γ/π for 1 < ω < 4 and ρ(ω) = 0, otherwise, and we found R(t) numerically (solid curve). Since BD converges very slowly, we use γ = 1 for BD and γ = 10−3 for normal diffusion with R(t) = exp(−γt), so we can compare both. We choose T0 = 1.5 and T0 = 0.5 for the initial temperatures. In both cases we used T = 1 for the reservoir temperature. From Lapas et al. [83], with permission from Europhysics Letters.
3.3.4. The Fluctuation-Dissipation Theorem
Figures 3, 4 are just illustrations of the results that can be obtained analytically from Equations (21–48). Lapas et al. [9, 83] have shown that the KT is valid for all forms of diffusion, and that the ballistic diffusion violates the EH, but not the KT.
Although it is expected that a system in contact with a heat reservoir will be driven to equilibrium by fluctuations, Figures 4, 5 show that it is not always the case and in some far from equilibrium situation it may not happen. The concept of “far from equilibrium” is itself sometimes misleading, since it depends not only on the initial conditions, but also on the possible trajectories the system may follow [8, 104, 105]. It was a known fact that the FDT can be violated in many slow relaxation processes [1, 121, 122], however, it was a surprise that it could occur in a GLE without disorder [123] or an external field [1, 8, 20]. Finally, Costa et al. [8] have called attention to the fact that if after a long time the fluctuations are not enough to drive the system to equilibrium then the fluctuation-dissipation theorem is violated. For the dissipation relation to be fulfilled, the MC condition, Equation (48), must be valid.
4. Beyond the Basics…and More Basic
In the last sections we have discussed some basic results for anomalous diffusion under the point of view of the formalism of the generalized Langevin equation which yield two main features: simplicity and exact results. Obviously, this approach does not exhaust the subject, since diffusion is a basic phenomenon in physics it is a starting point to many different formalisms which we shall briefly discuss.
4.1. Fractional Fokker-Planck Equation
We have seen that, in principle, all kinds of anomalous diffusion can be described by the GLE formalism, which is itself well established from the Mori method. Since normal diffusion can be studied both from Langevin equations and from Fokker-Planck equations, we would expect to obtain a generalized Fokker-Planck formalism for anomalous diffusion. Indeed, fractal formulations of the Fokker-Planck equation (FFPE) have been widely used in the literature in the last decades, in which the evolution of the probability distribution function P(x, t) reads [3, 4, 6]
where on the left-hand-side a fractional Rieman-Liouville time-derivative is defined as [124]
with 0 < α < 1. Note that the definition of fractional derivatives is not unique [124, 125], with a variety of possibilities, physically (almost) totally unexplored. We notice here that the nonlocal character of the fractional Fokker-Planck equation is similar to the memory kernel in the GLE. On the right hand side, γα is a generalized friction, U(x) is the potential, while Dα is generalized Einstein-Stokes relation
The major result from Equation (52) for a force-free diffusion is the asymptotic solution
Again, for α ≠ 1, we reach an anomalous diffusion regime [2, 4, 6, 126].
4.2. Interface Growth
Models for interface growth generally consider the random deposition of particles that diffuse to a surface and, as such, have been studied with Langevin equations and modified diffusion equations. Since diffusion with subsequent deposition is ubiquitous, rough surfaces at the interface of two media are very common in nature [14, 127–131] and the description of interface evolution is a very interesting problem in statistical physics. The major objective here is to describe the temporal evolution of the height of the interface between two substrates [14, 127], where is the d dimensional position vector and t is the time. We outline the evolution of h in Figure 6. In (a), we show a real forest fire propagation, a very complex situation. However, we can focus on the interface between the burnt and unburned regions. In (b), we provide a snapshot at a fixed time t of the height for a microscopic growth process in which the green medium is penetrating the blue one with arbitrary units. These types of dynamics in d + 1 dimensional space are easy to understand, but not so simple to solve analytically. Experiments can be done for d = 1, 2, 3; however, they present hard theoretical problems for any d.
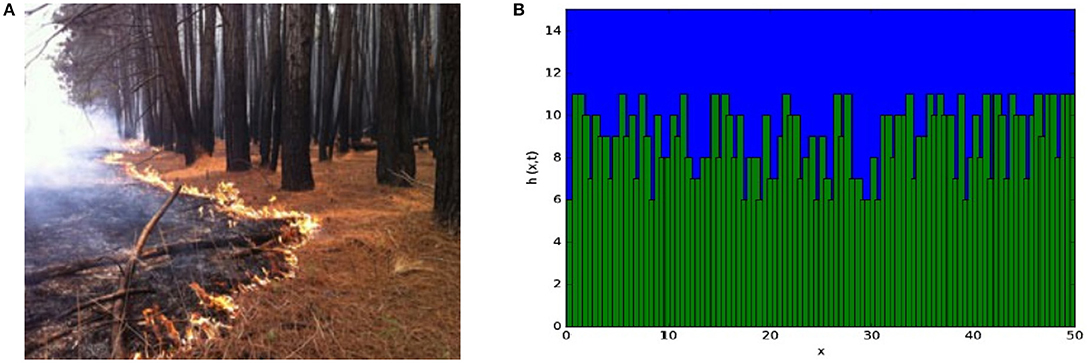
Figure 6. (Color online) (A) Fire propagation in the Amazon rainforest. Source: http://g1.globo.com/am/amazonas/noticia/2013/01/florestas-no-sul-do-am-estao-mais-vulneraveis-incendio-aponta-estudo.html; (B) Schematic representation on a microscopic scale of the height evolution , defining the interface between two media, as function of the position for a given time t.
The two main quantities of interest are the average height
where V is the sample volume, and the standard deviation
often called the surface width w(t), or roughness. Not surprisingly, this height fluctuation has a lot of information about the physical processes governing the system. The evolution of w(t) observed through experiments, computer simulations, and a few analytical results gives us some general features of growth dynamics.
Starting with a flat surface, w(0) = 0, the evolution exhibits four distinct regions: (a) for a very short period 0 < t < t0, during which correlations are negligible, the process is a random deposition w(t) ~ t1/2; (b) for t0 < t < t× we have w(t) ~ tβ. Here the t× follows a power law of the form , where L is the size of the sample, z is the dynamic exponent, and β the growth exponent; (c) there is a transition region for t ~ t×; (d) finally, for t ≫ t×, the dynamic equilibrium leads to surface width saturation, ws, which also follows a power law , where α is the roughness exponent. The crossing of the curves w(t) ~ tβ and w ~ ws yields the universal relation
It should be pointed out that these exponents are not related with those of the previous section.
To obtain w(t), we need to know and there are two major theoretical ways to attack this problem: (1) Continuous growth equations; (2) Discrete growth models. Figure 6A is an example of the first, while Figure 6B is an example of the second.
4.2.1. Equation of Motion and Symmetries
The growth, in general, is due to the number of particles per unit of time arriving on the surface at the position and time t. The particle flux is not uniform since the particles are deposited at random positions [14]. Therefore, the evolution of can be described by
where the first term F is the average number of particles arriving at site . The second term, , reflects the random fluctuations and satisfies
where, Dg measures the degree of growth randomness. The deterministic flux F must satisfy certain symmetry requirements, such as invariance under translation of position, , height, h → h + h0 and time t → t + t0. To satisfy these conditions, it must depend only on derivatives, which, again by the symmetries and h → −h, must be of even order [14, 127]. Besides this, considering that symmetrically possible terms such as , for n = 2, 3, …, are irrelevant in the long wavelength limit, since they go to zero faster than , we obtain, at the lowest-order in the derivatives, [127]
known as Edwards-Wilkinson equation (EW). Note that it is basically a diffusion equation, as (2), plus a noise, where we have a surface tension ν associated with the Laplacian smoothening mechanism. The random deposition model with surface relaxation is in the same universality class as the EW model [132], i.e., they share the same exponents.
Because there are, however, a large class of growth phenomena which are not described by the EW equation, new formulations become necessary. The recently proposed Arcetri models [133] allow the study of more rigid interfaces than the EW model and still allow for exact solutions in any dimension d. Depending on the initial conditions, both growing interfaces and particle motion on the lattice can be modeled. Another classical model was proposed by Kardar et al. [128], inspired by the stochastic Burgers equation. They observed that lateral growth could be added to this equation via the nonlinear term of the Burgers equation so that Equation (62) becomes
Since its formulation, the Kardar-Parisi-Zhang equation (KPZ) has been a prime model in the description of growth dynamics. The nonlinear term includes a new constant λ associated with the tilt mechanism and breaks down the symmetry h → −h. Consequently, the universality class of KPZ is different from that of EW.
Equation (63) is the simplest nonlinear equation that can describe a large number of growth processes [14, 128]. However, the apparent simplicity of this equation is misleading, since the nonlinear gradient term combined with the noise makes it one of the toughest problems in modern mathematical physics [134, 135]. On the other hand, its complexity is compensated for its generality. It is connected to a large number of stochastic processes, such as the direct polymer model [136], the weakly asymmetric simple exclusion process [137], the totally asymmetric exclusion process [138], direct d-mer diffusion [139], fire propagation [140–142], atomic deposition [143], evolution of bacterial colonies [144, 145], turbulent liquid-crystals [146–148], polymer deposition in semiconductors [149], and etching [150–161].
This problem in non-equilibrium statistical physics is analogous to the Ising model for equilibrium statistical physics, which is used as a basic model for understanding a large class of phenomena. The search for exact solutions to this equation in the d + 1 dimensional space has resulted in important contributions to mathematics; however, to date, they have been obtained only for specific situations and are limited to 1 + 1 dimensions [128, 135].
4.2.2. Scaling Invariance
In this small subsection we limit ourselves to scaling symmetries only. However, in interface growth, the shape of single-time and two-time responses and height correlation functions can be derived from the assumption of a local time-dependent scale-invariance [162]. For the KPZ equation, a systematic test of aging scaling was performed, showing the scaling relations for the two-time spatio-temporal autocorrelator and for the time-integrated response function [152, 163, 164].
The scaling invariance can be investigated through the transformation [14] , h → bα and t → tz which yields, for the KPZ equation, (63)
and
Scaling invariance demands that the exponents in Equations (64–66) must be zero. However, a simple inspection shows that they are inconsistent. The renormalization group approach of Kardar et al. [128], uses the nonlinear term as a perturbation, and their result shows that only Equation (66) remains invariant, yielding the famous Galilean invariance
Equations (64, 65) are corrected by the renormalization, and the final result yields α = 1/2, β = 1/3, and z = 3/2, for 1 + 1 dimensions. Since the KPZ renormalization approach is valid only for 1 + 1 dimensions, questions about the validity of the Galilean invariance [165, 166] for d > 1 and the existence of an upper critical dimension for KPZ [167, 168] have been raised.
For d > 1, the numerical simulation of the KPZ equation is not an easy task [165, 166, 169–172], and the use of cellular automata models [150–161, 173–176] has become increasingly common for growth simulations. Polynuclear growth (PNG), is a typical example of a discrete model that has received a lot of attention, and the outstanding works of Prähofer and Spohn [177] and Johansson [178] drive the way to the exact solution of the distributions of the heights fluctuations f(h, t) in the KPZ equation for 1 + 1 dimensions [135]. By construction, h → h − 〈h〉, then f(h, t) has zero mean, so its skewness and kurtosis are the most important quantities to observe [148, 179–182]. In addition, Langevin equations for growth models have been discussed by some authors [183–185]. Several works have been done in the weakly asymmetric simple exclusion process [137], the totally asymmetric exclusion process [138, 186], and the direct d-mer diffusion model [139]: for a review see [171, 182, 187, 188]. More experimentally measured exponents for growing interfaces in four universality classes (KPZ, quenched KPZ, EW and Arcetri) can be found in Henkel and Durang [133] and references therein.
4.2.3. Cellular Automata Growth Models
Cellular automata are described by simple rules, which allow us to inquire about relevant properties of a complex dynamical system. For a given growth model, the first question to be answered is if the model belongs to the same universality class as KPZ. In this context, we expose here the etching model [150], which has attracted considerable attention in recent years [151–161]. These studies suggest a close relation between the etching model and KPZ. For example, for 1 + 1 dimensions Alves et al. [159] have proven that α = 1/2 exactly. Unfortunately, their method does not allow to obtain β or z.
The etching model describes the mechanism of an acid eroding a surface. The detailed description of the model can be found in Mello et al. [150]. For simplicity, let us consider a site i in a hypercube of side L and volume V = Ld in space Ψ of dimension d, and look at one of its nearest neighbors, j. If hj > hi, then it becomes equal to hi. We then define the etching model following the steps:
1. At time t we randomly choose a site i ∈ V.
2. If hj(t) > hi(t), do hj(t + Δt) = hi(t).
3. Do hi(t + Δt) = hi(t) − 1.
In Figure 7, we show the mechanism of the etching model in one dimension: (a) step 1, we randomly select a site at time t, here i = 2 shown as green in the figure; step 2, the site i = 2 interacts with its neighbors j = 1, 3. Then in Figure 7 h1(t + Δt) = h2(t), meaning that it is strongly affected, and h3(t + Δt) = h3(t), meaning it is not affected; (c) step 3, h2(t + Δt) = h2(t) − 1. We describe here a process of strong interactions between the site and its neighbors.
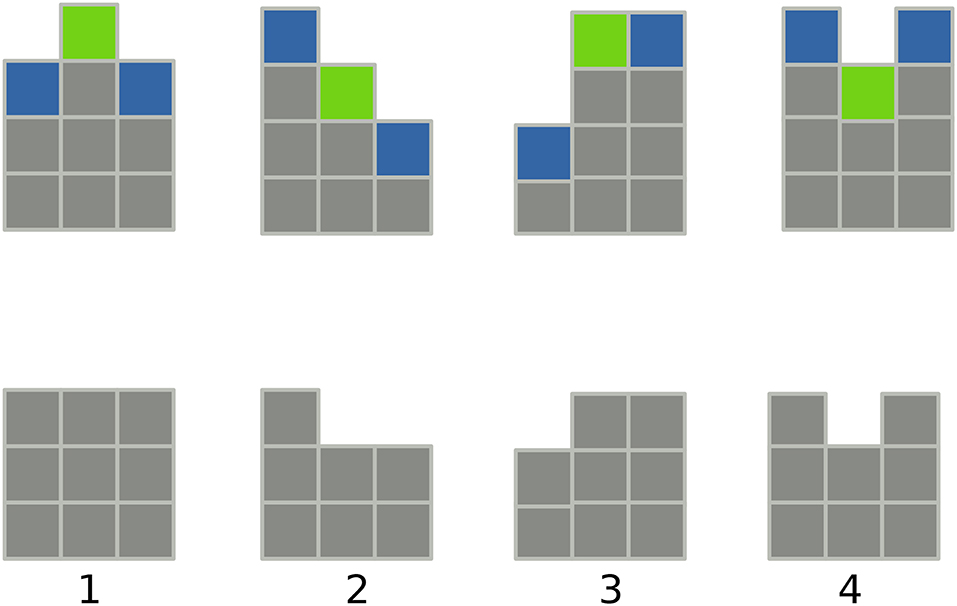
Figure 7. The etching mechanism in one dimension. The figures are the four situations in which the dynamics of the etching model can be divided. The initial configuration is at the top, where the selected site, in column 2, is green, and its first neighbors, in columns 1 and 3, are shown in dark blue. After the corrosion process the final situation is exhibited at the bottom. The steps are: step 1, site selection, green; step 2, interaction with nearest neighbors; step 3, decrease of height by one. From Alves et al. [159], with permission from Physics Review E.
Using these rules and averaging over Ne numerical experiments, it is possible to obtain the exponents α, β, and z [150–161]. Finally, the upper critical limit for the etching model (KPZ) was recently discussed by Rodrigues et al. [157], where it was shown numerically that there is no upper critical dimension for the etching model up to 6 + 1 dimensions. Thus, we have established a lower limit for the KPZ critical dimension, i.e., if it exists then dc > 6, in agreement with other authors [139]. They have shown that the Galilean invariance (67) remains valid for d ≤ 6 as well. These problems, however, still lack an exact solution.
4.3. Reaction-Diffusion Processes
One could not complete a work on diffusion without a brief discussion of reaction-diffusion processes [186, 189–191]. Exactly solvable reaction-diffusion models consist largely of single species reactions in one dimension, e.g., variations of the coalescence process, A + A → A + S [192–194] and the annihilation process A + A → S + S [193, 194], where A and S denote occupied and empty sites, respectively. These simple reactions display a wide range of behavior characteristic of non-equilibrium kinetics, such as self organization, pattern formation, and kinetic phase transitions. Interval methods have provided many exact solutions for one-dimensional coalescence and annihilation models. The method of empty intervals, applicable to coalescence models, requires solution of an infinite hierarchy of differential difference equations for the probabilities En of finding n consecutive lattice sites simultaneously empty. For annihilation models, the method of parity intervals similarly requires determination of Gn, the probability of n consecutive lattice sites containing an even number of particles [190]. In the continuous limit, such models can be described exactly in terms of the probability E(t, x) of finding an empty interval of size x at time t. Under fairly weak conditions, the equation of motion for E(t, x) is a diffusion equation, albeit with the unusual boundary condition E(t, 0) = 1. In several cases, the exact and fluctuation-dominated behavior in d = 1 has been seen experimentally, since the 1990s. This is one of the rare cases where theoretical statistical mechanics can be compared with experiments and also shows that simple mean-field schemes are not enough. For an introduction see Ben-Avraham and Havlin [189], and for up to date references see Shapoval et al. [191]. Another important new development in diffusion concerns stochastic resets, as they were introduced by Evans and Majumdar (see [195–197] and references therein). This leads to important modifications of the stationary state and raises the question how to consider the status of the general theorem described in the present review.
5. Conclusions
In this short review we address diffusion, as a modern and important topic of statistical physics, with broad applications [4–6, 8, 9, 40, 42]. We emphasize the study of systems with memory, for which a generalized Langevin equation applies and describe how the diffusion exponent is obtained from the memory [5, 97]. We highlight the properties of response functions [1, 83] for the processes of anomalous relaxation [1, 115], Gaussianization and ergodicity [9, 83]. Moreover, we have established a hierarchy: the mixing condition is stronger than the ergodic hypothesis, which is itself stronger than the fluctuation-dissipation theorem. It is important to call attention again to Costa et al. [8], where it was observed that in the ballistic diffusion the fluctuation-dissipation theorem fails. Indeed, for the ballistic motion which, on average, behaves as a Newtonian particle with constant velocity, the fluctuations are not enough to bring the system to equilibrium, i.e., the dissipation, which for large times decays as 1/t, does not balance the fluctuations. This work points out that the violation of the mixing condition breaks down ergodicity, as required by the Khinchin theorem and the fluctuation dissipation theorem.
In conclusion, since the mechanism of diffusion is present in most nonequilibrium processes, diffusion is an exhaustive subject, and we have only called attention to some of its aspects. Consequently, we apologize to the authors of important works not mentioned here, such as aging [123, 152, 163, 164, 198] for example.
Author Contributions
FO suggested the work, and wrote some sections. RF was responsible for the section on non-Markovian processes and selected the figures. LL wrote the ergodicity and decay toward equilibrium sections. MV collaborated on the section on the generalized Langevin equation and reedited the text.
Funding
This study was financed in part by the Coordenação de Aperfeiçoamento de Pessoal de Nível Superior - Brasil (CAPES) - Finance Code 001, CNPq and FAPDF. MV was supported by a Senior fellowship (88881.119772/2016-01) from Coordenação de Aperfeiçoamento de Pessoal de Nível Superior (CAPES) at MIT. MV would like to thank Prof. Jeff Gore for the hospitality at Physics of Living Systems, MIT.
Conflict of Interest Statement
The authors declare that the research was conducted in the absence of any commercial or financial relationships that could be construed as a potential conflict of interest.
References
1. Vainstein MH, Costa IVL, Oliveira FA. Mixing, ergodicity and the Fluctuation-dissipation Theorem in complex Systems. In: Miguel MC, Rubí M, editors. Jamming, Yielding, and Irreversible Deformation in Condensed Matter. Vol. 688 of Lecture Notes in Physics. Berlin;Heidelberg: Springer (2006). pp. 159–188.
2. Shlesinger MF, Zaslavsky GM, Klafter J. Strange kinetics. Nature (1993) 363:31. doi: 10.1038/363031a0
3. Metzler R, Barkai E, Klafter J. Anomalous diffusion and relaxation close to thermal equilibrium: a fractional Fokker-Planck equation approach. Phys Rev Lett. (1999) 82:3563. doi: 10.1103/PhysRevLett.82.3563
4. Metzler R, Klafter J. The random walk's guide to anomalous diffusion: a fractional dynamics approach. Phys Rep. (2000) 339:1. doi: 10.1016/S0370-1573(00)00070-3
5. Morgado R, Oliveira FA, Batrouni GG, Hansen A. Relation between anomalous and normal diffusion in systems with memory. Phys Rev Lett (2002) 89:100601. doi: 10.1103/PhysRevLett.89.100601
6. Metzler R, Klafter J. The restaurant at the end of the random walk: recent developments in the description of anomalous transport by fractional dynamics. J Phys A. (2004) 37:161. doi: 10.1088/0305-4470/37/31/R01
7. Sancho JM, Lacasta AM, Lindenberg K, Sokolov IM, Romero AH. Diffusion on a solid surface: anomalous is normal. Phys Rev Lett. (2004) 92:250601. doi: 10.1103/PhysRevLett.92.250601
8. Costa IVL, Morgado R, Lima MVBT, Oliveira FA. The Fluctuation-Dissipation Theorem fails for fast superdiffusion. Europhys Lett. (2003) 63:173. doi: 10.1209/epl/i2003-00514-3
9. Lapas LC, Morgado R, Vainstein MH, Rubí JM, Oliveira FA. Khinchin Theorem and anomalous diffusion. Phys Rev Lett. (2008) 101:230602. doi: 10.1103/PhysRevLett.101.230602
10. Weron A, Magdziarz M. Generalization of the Khinchin Theorem to Lévy flights. Phys Rev Lett. (2010) 105:260603. doi: 10.1103/PhysRevLett.105.260603
11. Thiel F, Flegel F, Sokolov IM. Disentangling sources of anomalous diffusion. Phys Rev Lett. (2013) 111:010601. doi: 10.1103/PhysRevLett.111.010601
12. McKinley S, Nguyen H. Anomalous diffusion and the generalized Langevin equation. SIAM J. Math. Anal. (2018) 50, 5119–160. doi: 10.1137/17M115517X
13. Flekkøy EG. Minimal model for anomalous diffusion. Phys Rev E. (2017) 95:012139. doi: 10.1103/PhysRevE.95.012139
14. Barabási AL, Stanley HE. Fractal Concepts in Surface Growth. Cambridge: Cambridge University Press (1995).
15. Murray JD. Mathematical Biology I. An Introduction. Vol. 17 of Interdisciplinary Applied Mathematics. 3rd edition. New York: Springer (2002).
16. Brown R. A brief account of microscopical observations made in the Months on June, July, and August, 1827, on the particles contained in the pollen of plants; and on the general existence of active molecules in Organic and Inorganic Bodies. Phil Mag. (1828) 4:161. doi: 10.1080/14786442808674769
17. Brown R. Mikroskopische Beobachtungen ber die im Pollen der Pflanzen enthaltenen Partikeln, und ber das allgemeine Vorkommen activer Molecle in organischen und unorganischen Kpern. Annalen der Physik (1828) 90:294–313. doi: 10.1002/andp.18280901016
18. Einstein A. ber die von der molekularkinetischen Theorie der Wrme geforderte Bewegung von in ruhenden Flssigkeiten suspendierten Teilchen. Annalen der Physik. (1905) 322:549–60. doi: 10.1002/andp.19053220806
20. Dyre JC, Schrøder TB. Universality of AC conduction in disordered solids. Rev Mod Phys. (2000) 72:873–92. doi: 10.1103/RevModPhys.72.873
21. Oliveira FA, Morgado R, Hansen A, Rubí JM. Superdiffusive conduction: AC conductivity with correlated noise. Physica A. (2005) 357:115–21. doi: 10.1016/j.physa.2005.05.056
22. Gudowska-Nowak E, Lindenberg K, Metzler R. Preface: Marian Smoluchowski's 1916 paper a century of inspiration. J Phys A. (2017) 50:380301. doi: 10.1088/1751-8121/aa8529
25. Gadomski A, Kruszewska N, Bełdowski P, Lent B, Ausloos M. A tribute to Marian Smoluchowski's legacy on soft grains assembly and hydrogel formation. Acta Phys. Polon. B (2018) 49:993–1005. doi: 10.5506/APhysPolB.49.993
26. On the Uniformity of Laws of Nature. XXX Marian Smoluchowski Symposium. 100th Anniversary of Smoluchowski's Death. Krakow (2017). Available online at: http://www.smoluchowski.if.uj.edu.pl/smoluchowski-2017
29. Toussaint R, Helgesen G, Flekkøy EG. Dynamic roughening and fluctuations of dipolar chains. Phys Rev Lett (2004) 93:108304. doi: 10.1103/PhysRevLett.93.108304
30. Oliveira FA, Taylor PL. Breaking in polymer chains. II. The Lennard Jones chain. J Chem Phys. (1994) 101:10118–125. doi: 10.1063/1.468000
31. Oliveira FA, Gonzalez JA. Bond-stability criterion in chain dynamics. Phys Rev B. (1996) 54:3954–8. doi: 10.1103/PhysRevB.54.3954
32. Oliveira FA. Transition-state analysis for fracture nucleation in polymers: the Lennard-Jones chain. Phys Rev B. (1998) 57:10576–82. doi: 10.1103/PhysRevB.57.10576
33. Maroja AM, Oliveira FA, Cieśla M, Longa L. Polymer fragmentation in extensional flow. Phys Rev E. (2001) 63:061801. doi: 10.1103/PhysRevE.63.061801
34. Dias CL, Dubé M, Oliveira FA, Grant M. Scaling in force spectroscopy of macromolecules. Phys Rev E. (2005) 72:011918. doi: 10.1103/PhysRevE.72.011918
35. Rahman A, Singwi KS, Sjölander A. Stochastic Model of a Liquid and Cold Neutron Scattering. II. Phys Rev. (1962) 126:997–1004. doi: 10.1103/PhysRev.126.997
36. Yulmetyev RM, Mokshin AV, Hänggi P. Diffusion time-scale invariance, randomization processes, and memory effects in Lennard-Jones liquids. Phys Rev E. (2003) 68:051201. doi: 10.1103/PhysRevE.68.051201
37. Bao JD. Transport in a flashing ratchet in the presence of anomalous diffusion. Phys Lett A. (2003) 314:203–8. doi: 10.1016/S0375-9601(03)00910-1
38. Bao JD, Zhuo YZ, Oliveira FA, Hänggi P. Intermediate dynamics between Newton and Langevin. Phys Rev E. (2006) 74:061111. doi: 10.1103/PhysRevE.74.061111
39. Longa L, Curado EMF, Oliveira FA. Roundoff-induced coalescence of chaotic trajectories. Phys Rev E. (1996) 54:R2201. doi: 10.1103/PhysRevE.54.R2201
40. Cieśla M, Dias SP, Longa L, Oliveira FA. Synchronization induced by Langevin dynamics. Phys Rev E. (2001) 63:065202. doi: 10.1103/PhysRevE.63.065202
42. Santamar-a-Holek I, Vainstein MH, Rubí JM, Oliveira FA. Protein motors induced enhanced diffusion in intracellular transport. Physica A. (2009) 388:1515–20. doi: 10.1016/j.physa.2009.01.013
43. Palmieri B, Bresler Y, Wirtz D, Grant M. Multiple scale model for cell migration in monolayers: elastic mismatch between cells enhances motility. Sci Rep. (2015) 5:11745. doi: 10.1038/srep11745
44. Lomholt MA, Ambjörnsson T, Metzler R. Optimal target search on a fast-folding polymer chain with volume exchange. Phys Rev Lett. (2005) 95:260603. doi: 10.1103/PhysRevLett.95.260603
45. Durang X, Kwon C, Park H. Overdamped limit and inverse-friction expansion for Brownian motion in an inhomogeneous medium. Phys Rev E. (2015) 91:062118. doi: 10.1103/PhysRevE.91.062118
46. Morgado R, Costa IVL, Oliveira FA. Normal and anomalous diffusion: ergodicity and fluctuation-dissipation theorem. Acta Phys Polon B. (2004) 35:1359. Available online at: http://www.actaphys.uj.edu.pl/fulltext?series=Reg&vol=35&page=1365
47. Vaytet N, Commerçon B, Masson J, González M, Chabrier G. Protostellar birth with ambipolar and ohmic diffusion. A A. (2018) 615:A5.
48. Mason TG, Weitz D. Optical measurements of frequency-dependent linear viscoelastic moduli of complex fluids. Phys Rev Lett. (1995) 74:1250. doi: 10.1103/PhysRevLett.74.1250
49. Grmela M, Öttinger HC. Dynamics and thermodynamics of complex fluids. I. Development of a general formalism. Phys Rev E. (1997) 56:6620. doi: 10.1103/PhysRevE.56.6620
50. Bakk A, Fossum JO, da Silva GJ, Adland HM, Mikkelsen A, Elgsaeter A. Viscosity and transient electric birefringence study of clay colloidal aggregation. Phys Rev E. (2002) 65:021407. doi: 10.1103/PhysRevE.65.021407
51. Sehnem AL, Aquino R, Campos AFC, Tourinho FA, Depeyrot J, Neto AMF. Thermodiffusion in positively charged magnetic colloids: influence of the particle diameter. Phys Rev E. (2014) 89:032308. doi: 10.1103/PhysRevE.89.032308
52. Sehnem AL, Neto AMF, Aquino R, Campos AFC, Tourinho FA, Depeyrot J. Temperature dependence of the Soret coefficient of ionic colloids. Phys Rev E. (2015) 92:042311. doi: 10.1103/PhysRevE.92.042311
53. Cabreira Gomes R, Ferreira da Silva A, Kouyat M, Demouchy G, Mriguet G, Aquino R, et al. Thermodiffusion of repulsive charged nanoparticles–the interplay between single-particle and thermoelectric contributions. Phys Chem Chem Phys. (2018) 20:16402–13. doi: 10.1039/C8CP02558D
54. de Brito PE, da Silva CAA, Nazareno HN. Field-induced localization in Fibonacci and Thue-Morse lattices. Phys Rev B. (1995) 51:6096–9. doi: 10.1103/PhysRevB.51.6096
55. Monte AFG, da Silva SW, Cruz JMR, Morais PC, Chaves AS, Cox HM. Symmetric and asymmetric fractal diffusion of electron-hole plasmas in semiconductor quantum wells. Phys Lett A. (2000) 268:430–5. doi: 10.1016/S0375-9601(00)00193-6
56. Monte AFG, da Silva SW, Cruz JMR, Morais PC, Chaves AS. Experimental evidence of asymmetric carrier transport in InGaAs quantum wells and wires grown on tilted InP substrates. Appl Phys Lett. (2002) 81:2460–2. doi: 10.1063/1.1507619
57. Kumakura K, Makimoto T, Kobayashi N, Hashizume T, Fukui T, Hasegawa H. Minority carrier diffusion length in GaN: dislocation density and doping concentration dependence. Appl Phys Lett. (2005) 86:052105. doi: 10.1063/1.1861116
58. Borges JB, da Silva SW, Morais PC, Monte AFG. Optical signatures of asymmetric fractal diffusion of electron-hole plasma in semiconductor quantum wells. Appl Phys Lett. (2006) 89:142103. doi: 10.1063/1.2358842
59. Gudowska-Nowak E, Bochenek K, Jurlewicz A, Weron K. Hopping models of charge transfer in a complex environment: coupled memory continuous-time random walk approach. Phys Rev E. (2005) 72:061101. doi: 10.1103/PhysRevE.72.061101
60. Filipovitch N, Hill K, Longjas A, Voller V. Infiltration experiments demonstrate an explicit connection between heterogeneity and anomalous diffusion behavior. Water Resour Res. (2016) 52:5167–78. doi: 10.1002/2016WR018667
61. Aarão Reis FDA. Scaling relations in the diffusive infiltration in fractals. Phys Rev E. (2016) 94:052124. doi: 10.1103/PhysRevE.94.052124
62. Aarão Reis FDA, Bolster D, Voller VR. Anomalous behaviors during infiltration into heterogeneous porous media. Adv Water Resour. (2018) 113:180–8. doi: 10.1016/j.advwatres.2018.01.010
63. Gomes Filho MS, Oliveira FA, Barbosa MAA. A statistical mechanical model for drug release: investigations on size and porosity dependence. Physica A. (2016) 460:29–37. doi: 10.1016/j.physa.2016.04.040
64. Ignacio M, Chubynsky MV, Slater GW. Interpreting the Weibull fitting parameters for diffusion-controlled release data. Physica A. (2017) 486:486–96. doi: 10.1016/j.physa.2017.05.033
65. Gun L, Kaikai Z, Rui G. Simulation on Drug Molecules Permeability of the Blood-Brain-Barrier. Am J Biol Life Sci. (2017) 5:30.
66. Soares JMD, Almeida JRGS, de Oliveira HP. Controlled release of extract of Morus nigra from Eudragit L-100 electrospun fibers: toxicity and in vitro release evaluation. Curr Trad Med. (2017) 3:146–54. doi: 10.2174/2215083803666170309123836
69. Cristea LL, Steinsky B. On totally disconnected generalised Sierpiński carpets. B Math Soc Sci Math. (2014) 57:27–34.
70. Balankin AS. The topological Hausdorff dimension and transport properties of Sierpiński carpets. Phys Lett A. (2017) 381:2801–8. doi: 10.1016/j.physleta.2017.06.049
71. Balankin AS. Mapping physical problems on fractals onto boundary value problems within continuum framework. Phys Lett A. (2018) 382:141–6. doi: 10.1016/j.physleta.2017.11.005
72. Barbosa MAA, Barbosa FV, Oliveira FA. Thermodynamic and dynamic anomalies in a one-dimensional lattice model of liquid water. J Chem Phys. (2011) 134:024511. doi: 10.1063/1.3522772
73. Bertolazzo AA, Kumar A, Chakravarty C, Molinero V. Water-like anomalies and phase behavior of a pair potential that stabilizes diamond. J Phys Chem B. (2015) 120:1649–59. doi: 10.1021/acs.jpcb.5b08432
74. da Silva FBV, Oliveira FA, Barbosa MAA. Residual entropy and waterlike anomalies in the repulsive one dimensional lattice gas. J Chem Phys. (2015) 142:144506. doi: 10.1063/1.4916905
75. Bier M, Lisowski B, Gudowska-Nowak E. Phase transitions and entropies for synchronizing oscillators. Phys Rev E. (2016) 93:012143. doi: 10.1103/PhysRevE.93.012143
76. Pinto PD, Oliveira FA, Penna ALA. Thermodynamics aspects of noise-induced phase synchronization. Phys Rev E. (2016) 93:052220. doi: 10.1103/PhysRevE.93.052220
77. Pinto PD, Penna AL, Oliveira FA. Critical behavior of noise-induced phase synchronization. Europhys Lett. (2017) 117:50009. doi: 10.1209/0295-5075/117/50009
78. Pérez-Madrid A. Gibbs entropy and irreversibility. Physica A. (2004) 339:339. doi: 10.1016/j.physa.2004.04.106
79. Rubí JM, Bedeaux D. Brownian motion in a fluid in elongational flow. J Stat Phys. (1988) 53:125. doi: 10.1007/BF01011549
80. Kuśmierz Ł, Dybiec B, Gudowska-Nowak E. Thermodynamics of superdiffusion generated by lévy–wiener fluctuating forces. Entropy (2018) 20:658. doi: 10.3390/e20090658
81. Hänggi P, Talkner P, Borkovec M. Reaction-rate theory: fifty years after kramers. Rev Mod Phys. (1990) 62:251–341. doi: 10.1103/RevModPhys.62.251
82. Morgado R, Cieśla M, Longa L, Oliveira FA. Synchronization in the presence of memory. Europhys Lett. (2007) 79:10002. doi: 10.1209/0295-5075/79/10002
83. Lapas LC, Costa IVL, Vainstein MH, Oliveira FA. Entropy, non-ergodicity and non-Gaussian behaviour in ballistic transport. Europhys Lett. (2007) 77:37004. doi: 10.1209/0295-5075/77/37004
84. Lee MH. Can the velocity autocorrelation function decay exponentially? Phys Rev Lett. (1983) 51:1227–30. doi: 10.1103/PhysRevLett.51.1227
85. Nyquist H. Thermal agitation of electric charge in conductors. Phys Rev. (1928) 32:110–13. doi: 10.1103/PhysRev.32.110
86. Mori H. Transport, collective motion, and Brownian motion. Prog Theor Phys. (1965) 33:423. doi: 10.1143/PTP.33.423
87. Mori H. A continued-fraction representation of the time-correlation function. Prog Theor Phys. (1965) 34:399. doi: 10.1143/PTP.34.399
88. Kubo R. Response, relaxation and fluctuation. In: Kirczenow G, Marro J, editors. Transport Phenomena. Lecture Notes in Physics. Vol. 31. Berlin; Heidelberg: Springer-Verlag (1974). p. 74-124.
90. Kubo R. Fluctuation-dissipation theorem. Prog Phys. (1966) 29:255. doi: 10.1088/0034-4885/29/1/306
91. Lee MH. Solutions of the generalized Langevin equation by a method of recurrence relations. Phys Rev B. (1982) 26:2547. doi: 10.1103/PhysRevB.26.2547
92. Lee MH. Derivation of the generalized Langevin equation by a method of recurrence relations. J Math Phys. (1983) 24:512. doi: 10.1063/1.525628
93. Lee MH, Hong J. Transport behavior of dense protons in a slab. Phys Rev B. (1984) 30:6756. doi: 10.1103/PhysRevB.30.6756
94. Gluskin E. Let us teach this generalization of the final-value theorem. Eur J Phys. (2003) 24:591. doi: 10.1088/0143-0807/24/6/005
95. Vainstein MH, Morgado R, Oliveira FA, de Moura FABF, Coutinho-Filho MD. Stochastic description of the dynamics of the random-exchange Heisenberg chain. Phys Lett A. (2005) 339:33–8. doi: 10.1016/j.physleta.2005.02.059
96. Vainstein MH, Costa IVL, Morgado R, Oliveira FA. Non-exponential relaxation for anomalous diffusion. Europhys Lett. (2006) 73:726–732. doi: 10.1209/epl/i2005-10455-9
97. Ferreira RMS, Santos MVS, Donato CC, Andrade JS, Oliveira FA. Analytical results for long-time behavior in anomalous diffusion. Phys Rev E. (2012) 86:021121. doi: 10.1103/PhysRevE.86.021121
98. Srokowski T. Nonstationarity induced by long-time noise correlations in the Langevin Equation. Phys Rev Lett. (2000) 85:2232. doi: 10.1103/PhysRevLett.85.2232
99. Srokowski T. Fluctuations in multiplicative systems with jumps. Phys Rev E. (2013) 87:032104. doi: 10.1103/PhysRevE.87.032104
100. Kadanoff LP, Götze W, Hamblen D, Hecht R, Lewis E, Palciauskas VV, et al. Static phenomena near critical points: theory and experiment. Rev Mod Phys. (1967) 39:395. doi: 10.1103/RevModPhys.39.395
101. Kadanoff LP. Statistical Physics: Statics, Dynamics and Renormalization. Singapore: World Scientific Publishing Company (2000).
102. Kenna R, Johnston DA, Janke W. Self-consistent scaling theory for logarithmic-correction exponents. Phys Rev Lett. (2006) 97:155702. doi: 10.1103/PhysRevLett.97.155702
103. Kenna R, Ruiz-Lorenzo JJ. Scaling analysis of the site-diluted Ising model in two dimensions. Phys Rev E. (2008) 78:031134. doi: 10.1103/PhysRevE.78.031134
104. Rubí JM, Santamaría-Holek I, Pérez-Madrid A. Slow dynamics and local quasi-equilibrium—relaxation in supercooled colloidal systems. J Phys. (2004) 16:S2047. doi: 10.1088/0953-8984/16/22/002
105. Santamaría-Holek I, Pérez-Madrid A, Rubí JM. Local quasi-equilibrium description of slow relaxation systems. J Chem Phys. (2004) 120:2818. doi: 10.1063/1.1640346
106. Vainstein MH, Stariolo DA, Arenzon JJ. Heterogeneities in systems with quenched disorder. J Phys A. (2003) 36:10907–19. doi: 10.1088/0305-4470/36/43/016
107. Santos MBL, Oliveira EA, Neto AMF. Rayleigh scattering of a new lyotropic nematic liquid crystal system: crossover of propagative and diffusive behavior. Liq Cryst. (2000) 27:1485. doi: 10.1080/026782900750018654
108. Benmouna F, Peng B, Gapinski J, Patkowski A, Ruhe J, Johannsmann D. Dynamic light scattering from liquid crystal polymer brushes swollen in a nematic solvent. Liq Cryst. (2001) 28:1353. doi: 10.1080/02678290110061395
109. Peyrard M. Glass transition in protein hydration water. Phys Rev E. (2001) 64:011109. doi: 10.1103/PhysRevE.64.011109
110. Colaiori F, Moore MA. Stretched exponential relaxation in the mode-coupling theory for the Kardar-Parisi-Zhang equation. Phys Rev E. (2001) 63:057103. doi: 10.1103/PhysRevE.63.057103
111. Ferreira JL, Ludwig GO, Montes A. Experimental investigations of ion-acoustic double-layers in the electron flow across multidipole magnetic fields. Plasma Phys Control Fusion. (1991) 33:297–311. doi: 10.1088/0741-3335/33/4/003
112. Bouchaud JP, Mézard M, Yedidia JS. Variational theory for disordered vortex lattices. Phys Rev Lett. (1991) 67:3840. doi: 10.1103/PhysRevLett.67.3840
113. Kohlrausch R. Theorie des elektrischen Rckstandes in der Leidener Flasche. Annalen der Physik. (1854) 167:56–82. doi: 10.1002/andp.18541670103
114. Kohlrausch F. ber die elastische Nachwirkung bei der Torsion. Annalen der Physik. (1863) 195:337. doi: 10.1002/andp.18631950702
115. Lapas LC, Ferreira RM, Rubí JM, Oliveira FA. Anomalous law of cooling. J Chem Phys. (2015) 142:104106. doi: 10.1063/1.4914872
116. Mittag-Leffler GM. Sur la reprsentation analytique d'une branche uniforme d'une fonction monogne. Acta Math. (1905) 29:101. doi: 10.1007/BF02403200
118. Bao JD, Hänggi P, Zhuo YZ. Non-Markovian Brownian dynamics and nonergodicity. Phys Rev E. (2005) 72:061107. doi: 10.1103/PhysRevE.72.061107
119. Silvestre C, Rocha Filho T. Ergodicity in a two-dimensional self-gravitating many-body system. Phys Lett A. (2016) 380:337–48. doi: 10.1016/j.physleta.2015.10.042
120. Campa A, Dauxois T, Ruffo S. Statistical mechanics and dynamics of solvable models with long-range interactions. Phys Rep. (2009) 480:57–159. doi: 10.1016/j.physrep.2009.07.001
121. Parisi G. Off-equilibrium fluctuation-dissipation relation in fragile glasses. Phys Rev Lett. (1997) 79:3660–3. doi: 10.1103/PhysRevLett.79.3660
122. Dybiec B, Parrondo JMR, Gudowska-Nowak E. Fluctuation-dissipation relations under Lvy noises. EPL (2012) 98:50006. doi: 10.1209/0295-5075/98/50006
123. Cugliandolo L, Kurchan J, Parisi G. Off equilibrium dynamics and aging in unfrustrated systems. J de Physique I. (1994) 4:1641–56. doi: 10.1051/jp1:1994212
124. Oldham K, Spanier J. The Fractional Calculus Theory and Applications of Differentiation and Integration to Arbitrary Order. Vol. 111. San Diego, CA: Elsevier (1974).
125. Kilbas AAA, Srivastava HM, Trujillo JJ. Theory and Applications of Fractional Differential Equations. Vol. 204. Amsterdam: Elsevier Science Limited (2006).
126. Klafter J, Shlesinger MF, Zumofen G. Beyond Brownian Motion. Phys Today (1996) 49:33. doi: 10.1063/1.881487
127. Edwards SF, Wilkinson DR. The surface statistics of a granular aggregate. Proc R Soc Lond A. (1982) 381:17–31. doi: 10.1098/rspa.1982.0056
128. Kardar M, Parisi G, Zhang YC. Dynamic Scaling of Growing Interfaces. Phys Rev Lett. (1986) 56:889–92. doi: 10.1103/PhysRevLett.56.889
129. Hansen A, Schmittbuhl J, Batrouni GG, de Oliveira FA. Normal stress distribution of rough surfaces in contact. Geophys Res Lett. (2000) 27:3639–42. doi: 10.1029/2000GL011757
130. Cordeiro JA, Lima MVBT, Dias RM, Oliveira FA. Morphology of growth by random walk deposition. Phys A. (2001) 295:209. doi: 10.1016/S0378-4371(01)00075-9
131. Schmittbuhl J, Chambon G, Hansen A, Bouchon M. Are stress distributions along faults the signature of asperity squeeze? Geophys Res Lett. (2006) 33:L13307. doi: 10.1029/2006GL025952
132. Horowitz CM, Monetti RA, Albano EV. Competitive growth model involving random deposition and random deposition with surface relaxation. Phys Rev E. (2001) 63:066132. doi: 10.1103/PhysRevE.63.066132
133. Henkel M, Durang X. Spherical model of growing interfaces. J Stat Mech Theory Exp. (2015) 2015:P05022. doi: 10.1088/1742-5468/2015/05/p05022
134. Hairer M. Solving the KPZ equation. Ann Math. (2013) 178:559–664. doi: 10.4007/annals.2013.178.2.4
135. Sasamoto T, Spohn H. One-dimensional kardar-parisi-zhang Equation: an exact solution and its Universality. Phys Rev Lett. (2010) 104:230602. doi: 10.1103/PhysRevLett.104.230602
136. Kardar M. (1985). Roughening by impurities at finite temperatures. Phys. Rev. Lett. 55:2923. doi: 10.1103/PhysRevLett.55.2923
137. Bertini L, Giacomin G. Stochastic Burgers and KPZ equations from particle systems. Commun Math Phys. (1997) 183:571–607. doi: 10.1007/s002200050044
138. Spitzer F. Interaction of Markov processes. Adv Math. (1970) 5:246–90. doi: 10.1016/0001-8708(70)90034-4
139. Ódor G, Liedke B, Heinig KH. Directed d-mer diffusion describing the Kardar-Parisi-Zhang-type surface growth. Phys Rev E. (2010) 81:031112. doi: 10.1103/PhysRevE.81.031112
140. Myllys M, Maunuksela J, Alava M, Ala-Nissila T, Merikoski J, Timonen J. Kinetic roughening in slow combustion of paper. Phys Rev E. (2001) 64:036101. doi: 10.1103/PhysRevE.64.036101
141. Myllys M, Maunuksela J, Merikoski J, Timonen J, Horvath V, Ha M, et al. Effect of a columnar defect on the shape of slow-combustion fronts. Phys Rev E. (2003) 68:051103. doi: 10.1103/PhysRevE.68.051103
142. Merikoski J, Maunuksela J, Myllys M, Timonen J, Alava MJ. Temporal and spatial persistence of combustion fronts in paper. Phys Rev Lett. (2003) 90:24501. doi: 10.1103/PhysRevLett.90.024501
143. Csahók Z, Vicsek T. Kinetic roughening in a model of sedimentation of granular materials. Phys Rev A. (1992) 46:4577–81. doi: 10.1103/PhysRevA.46.4577
144. Ben-Jacob E, Schochet O, Tenenbaum A, Cohen I, Czirk A, Vicsek T. Generic modelling of cooperative growth patterns in bacterial colonies. Nature (1994) 368:46–9. doi: 10.1038/368046a0
145. Matsushita M, Fujikawa H. Diffusion-limited growth in bacterial colony formation. Physica A. (1990) 168:498–506. doi: 10.1016/0378-4371(90)90402-E
146. Takeuchi KA, Sano M, Sasamoto T, Spohn H. Growing interfaces uncover universal fluctuations behind scale invariance. Sci Rep. (2011) 1:34. doi: 10.1038/srep00034
147. Takeuchi KA, Sano M. Evidence for geometry-dependent universal fluctuations of the Kardar-Parisi-Zhang interfaces in liquid-crystal turbulence. J Stat Phys. (2012) 147:853–90. doi: 10.1007/s10955-012-0503-0
148. Takeuchi KA. Crossover from growing to stationary interfaces in the Kardar-Parisi-Zhang class. Phys Rev Lett. (2013) 110:210604. doi: 10.1103/PhysRevLett.110.210604
149. Almeida RAL, Ferreira SO, Ferraz I, Oliveira TJ. Initial pseudo-steady state & asymptotic KPZ universality in semiconductor on polymer deposition. Sci Rep. (2017) 7:3773. doi: 10.1038/s41598-017-03843-1
150. Mello BA, Chaves AS, Oliveira FA. Discrete atomistic model to simulate etching of a crystalline solid. Phys Rev E. (2001) 63:041113. doi: 10.1103/PhysRevE.63.041113
151. Aarão Reis F. Dynamic transition in etching with poisoning. Phys Rev E. (2003) 68:041602. doi: 10.1103/PhysRevE.68.041602
152. Aarão Reis F. Universality in two-dimensional Kardar-Parisi-Zhang growth. Phys Rev E. (2004) 69:021610. doi: 10.1103/PhysRevE.69.021610
153. Aarão Reis FDA. Numerical study of roughness distributions in nonlinear models of interface growth. Phys Rev E. (2005) 72:032601. doi: 10.1103/PhysRevE.72.032601
154. Oliveira TJ, Aarão Reis FDA. Maximal- and minimal-height distributions of fluctuating interfaces. Phys Rev E. (2008) 77:041605. doi: 10.1103/PhysRevE.77.041605
155. Tang G, Xun Z, Wen R, Han K, Xia H, Hao D, et al. Discrete growth models on deterministic fractal substrate. Phys A. (2010) 389:4552–7. doi: 10.1016/j.physa.2010.06.041
156. Xun Z, Zhang Y, Li Y, Xia H, Hao D, Tang G. Dynamic scaling behaviors of the discrete growth models on fractal substrates. J Stat Mech Theory Exp. (2012) 2012:P10014. doi: 10.1088/1742-5468/2012/10/P10014
157. Rodrigues EA, Mello BA, Oliveira FA. Growth exponents of the etching model in high dimensions. J Phys A. (2015) 48:35001–12. doi: 10.1088/1751-8113/48/3/035001
158. Mello BA. A random rule model of surface growth. Physica A. (2015) 419:762–7. doi: 10.1016/j.physa.2014.10.064
159. Alves WS, Rodrigues EA, Fernandes HA, Mello BA, Oliveira FA, Costa IVL. Analysis of etching at a solid-solid interface. Phys Rev E. (2016) 94:042119. doi: 10.1103/PhysRevE.94.042119
160. Carrasco ISS, Oliveira TJ. Universality and dependence on initial conditions in the class of the nonlinear molecular beam epitaxy equation. Phys Rev E. (2016) 94:050801. doi: 10.1103/PhysRevE.94.050801
161. Carrasco ISS, Oliveira TJ. Kardar-Parisi-Zhang growth on one-dimensional decreasing substrates. Phys Rev E. (2018) 98:010102. doi: 10.1103/PhysRevE.98.010102
162. Henkel M. From dynamical scaling to local scale-invariance: a tutorial. Eur Phys J Spec Top. (2017) 226:605–25. doi: 10.1140/epjst/e2016-60336-5
163. Henkel M, Noh JD, Pleimling M. Phenomenology of aging in the Kardar-Parisi-Zhang equation. Phys Rev E. (2012) 85:030102. doi: 10.1103/PhysRevE.85.030102
164. Kelling J, Ódor G, Gemming S. Local scale-invariance of the 2+1 dimensional Kardar–Parisi–Zhang model. J Phys A. (2017) 50:12LT01. doi: 10.1088/1751-8121/aa5d87
165. Wio HS, Revelli JA, Deza RR, Escudero C, de La Lama MS. Discretization-related issues in the Kardar-Parisi-Zhang equation: consistency, Galilean-invariance violation, and fluctuation-dissipation relation. Phys Rev E. (2010) 81:066706. doi: 10.1103/PhysRevE.81.066706
166. Wio HS, Rodríguez MA, Gallego R, Revelli JA, Alés A, Deza RR. d-Dimensional KPZ equation as a stochastic gradient flow in an evolving landscape: interpretation and time evolution of its generating functional. Front Phys. (2017) 4:52. doi: 10.3389/fphy.2016.00052
167. Colaiori F, Moore M. Upper critical dimension, dynamic exponent, and scaling functions in the mode-coupling theory for the Kardar-Parisi-Zhang equation. Phys Rev Lett. (2001) 86:3946. doi: 10.1103/PhysRevLett.86.3946
168. Schwartz M, Perlsman E. Upper critical dimension of the Kardar-Parisi-Zhang equation. Phys Rev E. (2012) 85:050103. doi: 10.1103/PhysRevE.85.050103
169. Lam CH, Shin FG. Improved discretization of the Kardar-Parisi-Zhang equation. Phys Rev E. (1998) 58:5592. doi: 10.1103/PhysRevE.58.5592
170. Xu Z, Han H, Wu X. Numerical method for the deterministic Kardar-Parisi-Zhang equation in unbounded domains. Commun Comput Phys. (2006) 1:479–93.
171. Halpin-Healy T, Takeuchi KA. A KPZ cocktail-shaken, not stirred… J Stat Phys. (2015) 160:794–814. doi: 10.1007/s10955-015-1282-1
172. Torres MF, Buceta RC. Numerical integration of KPZ equation with restrictions. JStat Mech Theory Exp. (2018) 2018:033208. doi: 10.1088/1742-5468/aab1b3
173. Kelling J, Ódor G, Gemming S. Dynamical universality classes of simple growth and lattice gas models. J Phys A. (2017) 51:035003. doi: 10.1088/1751-8121/aa97f3
174. Předota M, Kotrla M. Stochastic equations for simple discrete models of epitaxial growth. Phys Rev E. (1996) 54:3933–42. doi: 10.1103/PhysRevE.54.3933
175. Chua ALS, Haselwandter CA, Baggio C, Vvedensky DD. Langevin equations for fluctuating surfaces. Phys Rev E. (2005) 72:051103. doi: 10.1103/PhysRevE.72.051103
176. Buceta RC, Hansmann D, von Haeften B. Revisiting random deposition with surface relaxation: approaches from growth rules to the Edwards-Wilkinson equation. J Stat Mech Theory Exp. (2014) 2014:p12028. doi: 10.1088/1742-5468/2014/12/P12028
177. Prähofer M, Spohn H. Universal distributions for growth processes in 1 + 1 dimensions and random matrices. Phys Rev Lett. (2000) 84:4882–5. doi: 10.1103/PhysRevLett.84.4882
178. Johansson K. Shape fluctuations and random matrices. Commun Math Phys. (2000) 209:437–76. doi: 10.1007/s002200050027
179. Oliveira TJ, Alves SG, Ferreira SC. Kardar-Parisi-Zhang universality class in (2+1) dimensions: universal geometry-dependent distributions and finite-time corrections. Phys Rev E. (2013) 87:040102. doi: 10.1103/PhysRevE.87.040102
180. Alves SG, Oliveira TJ, Ferreira SC. Non-universal parameters, corrections and universality in Kardar–Parisi–Zhang growth. J Stat Mech Theory Exp. (2013) 2013:P05007. doi: 10.1088/1742-5468/2013/05/P05007
181. Almeida RAL, Ferreira SO, Oliveira TJ, Aarão Reis FDA. Universal fluctuations in the growth of semiconductor thin films. Phys Rev B. (2014) 89:045309. doi: 10.1103/PhysRevB.89.045309
182. Halpin-Healy T, Zhang YC. Kinetic roughening phenomena, stochastic growth, directed polymers and all that. Aspects of multidisciplinary statistical mechanics. Phys Rep. (1995) 254:215–414. doi: 10.1016/0370-1573(94)00087-J
183. Haselwandter CA, Vvedensky DD. Scaling of ballistic deposition from a Langevin equation. Phys Rev E. (2006) 73:040101. doi: 10.1103/PhysRevE.73.040101
184. Haselwandter CA, Vvedensky DD. Renormalization of stochastic lattice models: Epitaxial surfaces. Phys Rev E. (2008) 77:061129. doi: 10.1103/PhysRevE.77.061129
185. Silveira FA, Aarão Reis FDA. Langevin equations for competitive growth models. Phys Rev E. (2012) 85:011601. doi: 10.1103/PhysRevE.85.011601
186. Alcaraz FC, Bariev RZ. Exact solution of the asymmetric exclusion model with particles of arbitrary size. Phys Rev E. (1999) 60:79. doi: 10.1103/PhysRevE.60.79
187. Meakin P. The growth of rough surfaces and interfaces. Phys Rep. (1993) 235:189–289. doi: 10.1016/0370-1573(93)90047-H
188. Krug J. Origins of scale invariance in growth processes. Adv Phys. (1997) 46:139–282. doi: 10.1080/00018739700101498
189. Ben-Avraham D, Havlin S. Diffusion and Reactions in Fractals and Disordered Systems. Cambridge, MA: Cambridge University Press (2000).
190. Abad E, Masser T, Ben-Avraham D. Lattice kinetics of diffusion-limited coalescence and annihilation with sources. J Phys A Math Gen. (2002) 35:1483. doi: 10.1088/0305-4470/35/7/301
191. Shapoval D, Dudka M, Durang X, Henkel M. Cross-over between diffusion-limited and reaction-limited regimes in the coagulation-diffusion process. J Phys A Math Theor. (2018) 51:425002. doi: 10.1088/1751-8121/aadd53
192. Doering CR, Ben-Avraham D. Diffusion-limited coagulation in the presence of particle input: exact results in one dimension. Phys Rev Lett. (1989) 62:2563. doi: 10.1103/PhysRevLett.62.2563
193. Krebs K, Pfannmüller MP, Wehefritz B, Hinrichsen H. Finite-size scaling studies of one-dimensional reaction-diffusion systems. Part I. Analytical results. J Stat Phys. (1995) 78:1429–70. doi: 10.1007/BF02180138
194. Simon H. Concentration for one and two-species one-dimensional reaction-diffusion systems. J Phys A Math Gen. (1995) 28:6585. doi: 10.1088/0305-4470/28/23/013
195. Evans MR, Majumdar SN. Diffusion with stochastic resetting. Phys Rev Lett. (2011) 106:160601. doi: 10.1103/PhysRevLett.106.160601
196. Evans MR, Majumdar SN. Diffusion with resetting in arbitrary spatial dimension. J Phys A Math Theor. (2014) 47:285001. doi: 10.1088/1751-8113/47/28/285001
197. Durang X, Henkel M, Park H. The statistical mechanics of the coagulation–diffusion process with a stochastic reset. J Phys A Math Theor. (2014) 47:045002. doi: 10.1088/1751-8113/47/4/045002
Keywords: anomalous diffusion, fluctuation-dissipation theorem, generalized Langevin equation, Khinchin theorem, correlation functions, Fokker-Planck equation, growth processes, ergodicity breaking
Citation: Oliveira FA, Ferreira RMS, Lapas LC and Vainstein MH (2019) Anomalous Diffusion: A Basic Mechanism for the Evolution of Inhomogeneous Systems. Front. Phys. 7:18. doi: 10.3389/fphy.2019.00018
Received: 31 August 2018; Accepted: 28 January 2019;
Published: 19 February 2019.
Edited by:
Michel Droz, Universitè de Genèe, SwitzerlandReviewed by:
Adam Lipowski, Adam Mickiewicz University in Poznań, PolandMalte Henkel, University of Lorraine, France
Copyright © 2019 Oliveira, Ferreira, Lapas and Vainstein. This is an open-access article distributed under the terms of the Creative Commons Attribution License (CC BY). The use, distribution or reproduction in other forums is permitted, provided the original author(s) and the copyright owner(s) are credited and that the original publication in this journal is cited, in accordance with accepted academic practice. No use, distribution or reproduction is permitted which does not comply with these terms.
*Correspondence: Fernando A. Oliveira, ZmFvQGZpcy51bmIuYnI=