- Light and Health Research Center, Department of Population Health Science and Policy, Icahn School of Medicine at Mount Sinai, New York, NY, United States
Circadian disruption, a breakdown in the regularity of activity patterns across the 24-h day, can lead to a variety of maladies. Some individuals and organizations object to the twice-yearly, seasonal changes in local time because it contributes to circadian disruption. The number of days required to re-entrain the circadian system to the new local time following transitions to or from daylight saving time is not completely understood, but several simple rules of thumb (i.e., heuristics) have been offered to minimize the days to re-entrainment and, thus, circadian disruption (e.g., go for a morning walk). Recently, the authors developed a computational model for predicting circadian phase from calibrated light-dark exposure patterns, based largely on the pioneering work of Kronauer and colleagues. This model was used here to predict the days to re-entrainment of the circadian systems of “larks” and “owls” to a new local time if they were exposed to one of three specific light interventions. Simulations showed that the timing of a light intervention must account for chronotypes (e.g., timing of minimum core body temperature) and direction of shift (i.e., phase advance or delay) to achieve re-entrainment to the time change more quickly. Simple heuristics are not necessarily adequate for minimizing the days to re-entrainment.
1 Introduction
Models are developed to help us understand natural phenomena. Whether simple heuristics or complex algorithms, the value of any model depends upon its ability to accurately predict natural phenomena, both in terms of direction and magnitude. The present study employs a recently published computational model, the circadian stimulus (CS)-oscillator model (Rea et al., 2022), which is aimed at predicting light-induced phase changes, to accelerate re-entrainment following the shift from standard time to daylight saving time, and vice versa. Some of those predictions are counter to common heuristics (Tumin, 2023; Suni, 2024) for reducing circadian disruption.
Since the seminal publication of Circadian Clocks (Aschoff, 1965), much has been learned about the human master clock in our brain and how its timing is affected by light exposure on the retina. Ideally, the master clock will synchronize or entrain to the local, daily cycle of light and dark. Through entrainment, the master clock orchestrates the ideal timing for executing our physiological and behavioral functions over the entire 24-h daily cycle. Indeed, it is the ability of the master clock to anticipate what needs to happen, and when, that makes the circadian system so remarkable. Without a consistent, synchronizing 24-h cycle of light and dark, however, the master clock loses its ability to accurately anticipate and then control the best timing of physiology and behavior. As has been shown in innumerable studies, health and reproductive success (Miller and Takahashi, 2014; Swamy et al., 2018) are compromised by disruption of a regular circadian cycle resulting from disruption of a regular 24-h cycle of light and dark exposure on the retina.
The mountain of accumulated knowledge about the master clock in response to light can ideally be boiled down into simple heuristics for promoting circadian entrainment (e.g., UL Standards and Engagement, 2019) and thus, better health. By consistently exposing our retina to bright days and dark nights, the master clock can consistently influence what biologically needs to happen at what time(s) and thereby can orchestrate the entire circadian system for better chances of survival and reproduction. But we clearly do not always behave in accordance with that simple rubric. Rather, as a highly intelligent and highly social species, we find many ways to break the synchrony between the natural 24-h light-dark exposure pattern and the light-dark exposure profiles that we actually experience, thereby negatively impacting the timing of the master clock. For example, modern humans commonly travel rapidly across time zones, placing the timing of the master clock at odds with the new local times of sunrise and sunset (Meir, 2002). We also work and play into the night, limiting our exposure to bright light while we sleep during the day and extending exposure to dim light well into the night (Qin et al., 2003). Even without rapid, trans-meridian travel or shift work, nearly all of us living within the built environment (Cox-Ganser and Henneberger, 2021) experience insufficient light exposures during the day and prolonged light exposures after sunset (Reiter et al., 2007; Bonmati-Carrion et al., 2014; Smolensky et al., 2015).
Many people around the globe experience the sudden change in local clock time twice a year, forcing us to re-entrain our biological clock to the new local time. Therefore, our complicated modern lifestyles limit the predictive power of any simple heuristic. That being the case, we need to develop more-complex predictive models that consider the complicated sociological and technological environment in which we live. To do so, we need to integrate three conceptual domains into a more complete and thereby more accurate predictive model of circadian entrainment.
1.1 Retinal response to light
First, we must define light as it affects the master clock. Light is a biophysical construct that reflects the spectral and absolute sensitivities of the human retina to optical radiation. The photopic luminous efficiency function [V(λ)], or the “eye spectral sensitivity curve,” was developed by the International Commission on Illumination (CIE) in the 1920s (Commission Internationale de l'Éclairage, 1926) to support international commerce for the emerging electric lighting industry such that a lumen (visually effective radiant flux) was the same in one country as it was in another. V(λ) was created from empirical psychophysical experiments without the benefit of a clear understanding of how the human retina converts optical radiation into neural signals to the brain. Today we know that V(λ) represents the spectral sensitivity of two cone photoreceptors (L-cone and M-cone) in the retina as they feed one multi-neuron channel connecting the retina to the conscious brain. We also now know that the neural channel characterized by the V(λ) spectral sensitivity function does not function at low light levels, like starlight. The luminous efficiency of the so-called scotopic channel [V′(λ)] was established by the CIE in the 1950s (Jansen and Halbertsma, 1951) and is characterized by the rod photoreceptor action spectrum. A scotopic function was needed because the spectral and absolute sensitivities of rods are very different than those for the two-cone, photopic channel [V(λ)]. Indeed, we now describe the human eye as having a “duplex retina” (Barlow, 1972), one channel for daytime, photopic light levels, and one channel for nighttime, scotopic light levels. As the neuroscience has progressed since the 1920s and 1950s, we now know that there are five photoreceptors in the retina and, even more importantly, that they all participate in many neural channels that leave the eye to reach different parts of the brain. Light for each of these channels will be different because the photoreceptors and neurons that convert optical radiation into neural signals leaving the eye differ from light that stimulates the photopic or scotopic channels (Rea, 2012).
One of the multi-neuron channels leaving the retina, the retinohypothalamic tract (RHT) of the optic nerve formed by the axons of the intrinsically photosensitive retinal ganglion cells (ipRGCs), reaches the suprachiasmatic nuclei (SCN), the master biological clock in the brain. This light-sensitive channel drives the timing of the biological clock, where, hopefully, it sends neural signals to support a robust synchrony between the exogenous light-dark cycle and the endogenous diurnal-nocturnal cycles of physiology and behavior. Not surprisingly perhaps, the combinations of photoreceptors and neurons that form this neural channel are complex. A quantitative model of both the spectral sensitivity and the operating characteristics of the RHT neural channel has been developed (Rea et al., 2021a) that, importantly, is consistent with human retinal neural anatomy and physiology (Rea et al., 2021b).
Briefly, the spectral sensitivity of the RHT neural channel (Figure 1A) is characterized by what is termed circadian-effective light (CLA). All five photoreceptors in the retina (L-cone, M-cone, S-cone, rod, and ipRGC) contribute to CLA and their relative participation in spectral sensitivity changes with the amount of optical radiation incident on the retina. The operating characteristics of the RHT channel are modeled in terms of circadian stimulus (CS), which quantifies the magnitude of the neural signal generated by the retina from threshold to saturation (Figure 1B), typically following a sigmoid-like function (DeLean et al., 1978; Evans et al., 1993). Dark for this neural channel can be defined as CLA levels below CS threshold, and bright as CLA levels above CS saturation. For the RHT neural channel, CLA from full moonlight is below CS threshold but well above rod threshold, and CLA from daylight, even on a cloudy day, is above CS saturation but well below cone saturation. CLA levels within the indoor built environment are almost always between CS threshold and CS saturation as illustrated in Figure 1B.
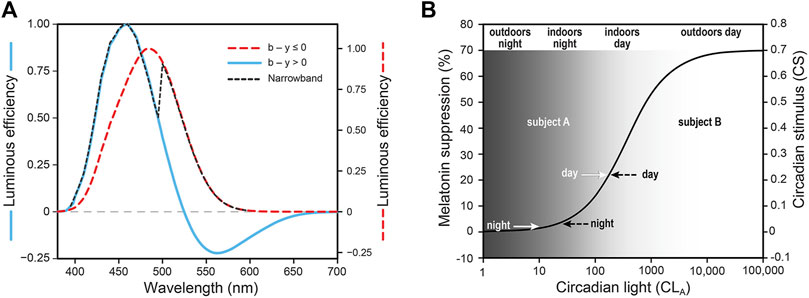
Figure 1. Spectral sensitivity of the RHT channel to monochromatic sources and to polychromatic “warm” (b (blue) – y (yellow) ≤ 0) and “cool” (b–y > 0) lights (A). Operating characteristic of the RHT channel from threshold to saturation (B); the value gradient illustrates CLA levels that would commonly be found in different locations and times of day. Also shown in panel (B) are the average CS levels measured during the day and during the night (before bedtime) for the two exemplar subjects in the present study (see Section 2.1).
1.2 Calibrated ambulatory light data
Second, we need to be able to capture representative profiles of calibrated light and dark exposures to the human retina as they might affect the timing of the master clock. Ambulatory light measurement devices must be used so that the timing, duration, and amount of the circadian-effective light exposures are recorded over the course of the 24-h days. These light measurement devices must be calibrated in terms of the RHT channel spectral and absolute sensitivities, CLA and CS, respectively. Ideally these devices should measure optical radiation near the person’s eyes, but this is often not practical or acceptable to the person, compromising the spatial accuracy of these measurement devices to various degrees (Figueiro et al., 2013). The first such calibrated circadian-effective light measurement device, the Daysimeter, was developed in 2005 (Bierman et al., 2005). The sensor was worn near the eyes and included three optical sensors (RGB). Through post-processing, the spectral sensitivity and the operating characteristics of the RHT neural channel were used to estimate the magnitudes of the circadian-effective light exposures every 3 min throughout the recording period, typically over seven consecutive days.
Several iterations of the Daysimeter have since been developed (Bierman et al., 2005; Miller et al., 2010; Rea et al., 2010; Figueiro et al., 2013). The accuracy of the calibration has improved as a better understanding of the spectral sensitivity (i.e., CLA 2.0) (Rea et al., 2021a) and operating characteristics (i.e., CS) of the channel has increased. However, again underscoring the complexity of human behavior, the spatial accuracy of the Daysimeter has been compromised to some degree because, as noted previously, subjects have been reluctant to wear the device near the eyes as it was originally designed (Figure 2A). Subjects were more compliant when the Daysimeter was worn as a pendant (Figure 2B) without, unlike wrist-worn devices, compromising accuracy compared to measurements at the eyes (Figueiro et al., 2013). Notwithstanding, continuous calibrated ambulatory light measurements across several days are essential for characterizing the 24-h light-dark exposure pattern that synchronizes, or disrupts, the biological clock with respect to a person’s local position on Earth. Without that information, it is impossible to characterize circadian entrainment or how a light intervention might affect entrainment. We have used the Daysimeter in Figure 2B successfully in several field studies (Figueiro et al., 2014; Rea et al., 2016).
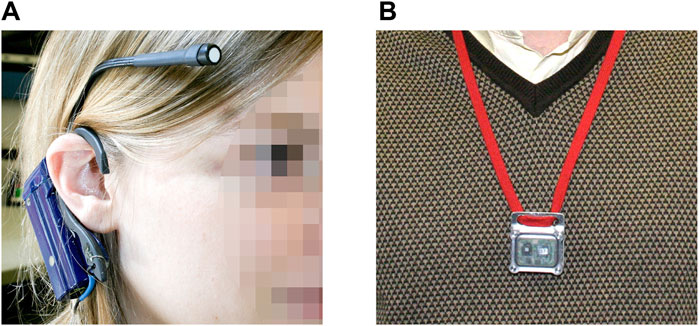
Figure 2. Examples of the Daysimeter worn near the eyes (A) and worn as a pendant (B). Images with permission of the Lighting Research Center.
1.3 Response of the biological clock to retinal input
Third, once the RHT neural channel signal to the SCN has been quantified, it is then necessary to model how the biological clock processes that signal for downstream communication of circadian phase to the many various systems that govern our physiology and behavior. Kronauer (1990) and colleagues (Kronauer et al., 1999) developed a van der Pol oscillator model of the SCN whereby its phase changed in response to photic input from the retina, measured in terms of photopic (two cone) illuminance. Several investigations have utilized this model, or variations on it, to predict light-induced phase changes quantified in terms of the predicted changes in clock time for the minimum core body temperature (CBTmin), a common marker of circadian phase (Refinetti, 2020). Many of these studies have been in a laboratory setting (St Hilaire et al., 2007; Mott et al., 2011) while others have used personal light measurement devices in the field (Woelders et al., 2017; Huang et al., 2021). All of these studies show a predictive accuracy of photopic (two cone) light-induced circadian phase changes no better than approximately 1 h.
The recently developed CS-oscillator model (Rea et al., 2022) retains the basic structure of the Kronauer et al. (1999) model, but the photic input is defined in terms of CS (Rea et al., 2021a; b). Four independent studies measuring circadian phase changes (Sharkey et al., 2011; Appleman et al., 2013; Figueiro et al., 2014; Rea et al., 2016), before and after a light intervention, were used to compare the predictive accuracies of the Kronauer et al. (1999) and CS-oscillator models. In these four studies light measurement devices (Daysimeter) calibrated in terms of photopic illuminance (lux) or CLA were used to continuously measure personal light exposures over 24 h for 1 week. The predicted phase changes in dim light melatonin onset, another measure of circadian phase, ranged from mean absolute error (MAE) between 0.91 h and 1.43 h using the Kronauer et al. (1999) model, with an average MAE of 1.07 h (1 h, 4 min). With the CS-oscillator model the range of MAE values was narrowed to between 0.59 h and 0.63 h with an average MAE of 0.61 h (37 min). To reach this level of accuracy from the CS-oscillator model, the initial circadian phase of an individual must be accurately estimated to properly assess the impact of a light intervention on altering circadian phase. It is also worth noting that the entire 24-h exposure pattern is needed for this level of accuracy, undermining the simple heuristic often repeated that only light exposures during the morning and the evening need to be considered to predict phase changes.
1.4 Goal of the present study
The present study utilizes information in all three aforementioned domains to predict how circadian phase is affected by personal light profiles experienced by two working adults, a typical “morning” (lark) type and a typical “evening” (owl) type (Lack et al., 2009), before and after the twice-yearly seasonal changes in local time at two geographically distant US cities (Boston and Detroit), but within the same (Eastern) time zone. We begin with exemplar, 7-day, 24-h, light-dark (and activity-rest) patterns obtained from calibrated Daysimeters that were worn as pendants (i.e., as in Figure 2B) by the two individuals. The Daysimeter records raw photic light levels from three channels, R, G, and B, and movement from three orthogonal accelerometer channels, x, y, and z. Through post-processing, calibrated light levels can be quantified in terms of photopic illuminance in lux, or circadian-effective light, at CLA 1.0 (Rea et al., 2010) or CLA 2.0 (Rea et al., 2021a; b) levels. The CLA 2.0 levels represent a refined version of the 1.0 version of the model used to characterize light for the circadian system, based on recent nocturnal melatonin suppression data collected to test predictions from the 1.0 version (Nagare et al., 2019b; Nagare et al., 2019c; d). From the processed CLA levels, CS levels can then be determined (Rea et al., 2021a; b). From the processed accelerometer data, an activity index (AI) is determined (Miller et al., 2010). AI is the root mean square (RMS) deviation in acceleration in the three (x, y, and z) accelerometer channels for each logging interval.
From the CS-calibrated, 7-day light-dark pattern, the CS-oscillator model was engaged to predict CBTmin with respect to local time before-and-after the transition times to standard time (ST) during the autumn (November) and to daylight saving time (DST) during the spring (March). From the two, lark and owl, before-and-after determinations of CBTmin, the days to re-entrainment (DTR) were then determined. DTR is defined as the number of days it takes for the internal biological clock of an individual to temporally re-align itself to local clock time following the autumn or the spring transition. The exemplary light-dark patterns were then virtually modified in several ways to illustrate how the CS-oscillator model could be used to predict DTR following those virtual light interventions. A goal of the present study was to determine how various, practical light interventions could be used by larks and owls to minimize DTR following the two seasonal changes in local clock time. Recognizing the infinite variations in behavior patterns, light exposure patterns, individual chronotypes, and geographical location, our general goal was to develop a more refined and accurate set of heuristics so that individuals could more rapidly adjust to the transition to and from DST.
2 Methods
2.1 Exemplar subject profile selections
Figures 3A,B show 7-day average daily levels of CS and AI (arbitrary units) for two employed subjects (denoted “A” and “B”) who participated in one of our Light and Health Institute online educational programs in September 2022. Subjects were permanent daytime workers and wore the Daysimeter as a pendant for a week at their home/work location prior to beginning the program. A variety of tabulated metrics derived from the Daysimeter data are shown in Table 1. Of particular note, CBTmin (see Section 2.2 for determining CBTmin), was calculated from the CS-oscillator model (Rea et al., 2022). Based upon that determination, subject A was classified as a “lark” (Figure 3A) and subject B was classified as an “owl” (Figure 3B) (Gale and Martyn, 1998; Roenneberg et al., 2003). The terms lark and owl are used here to characterize their relative CBTmin times, both of which lie near the center of the range for “morning types” and for “evening types,” respectively (Lack et al., 2009). In the context of exemplar subject selection for the present study, however, we wanted to not only select subjects who were different in terms of their predicted CBTmin, but we also wanted to select ones who were “typical” of individuals working and residing indoors most of the day, given that most Americans spend 90% of their time indoors (U.S. Environmental Protection Agency, 1989). It is noteworthy that their phasor magnitudes, indicating the strength of the synchronization between the 24-h light-dark and 24-h activity-rest rhythms (Rea et al., 2008), were very similar, indicating that both subjects were equally entrained to their personal light-dark exposure patterns and their activity-sleep schedules. Consistent with the similar phasor magnitudes, the difference between the CBTmin and wake time was about 3 h for both subjects, a value not unlike that found in other studies for entrained individuals (Carrier et al., 1999). Naturally, however, the phasor angles, which indicate the relative offset between the light-dark cycle and the activity-rest cycle (Rea et al., 2008), of the two subjects were quite different, consistent with their CBTmin values and their lark and owl categorizations.
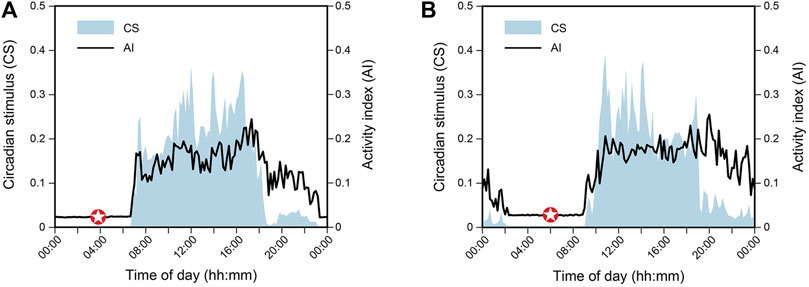
Figure 3. Temporal light-dark exposure (CS, shaded) and activity (AI, solid line) profiles, each averaged over 7 days, for subject A, the lark (A), and subject B, the owl (B). The star represents the time of CBTmin based upon the light-dark exposure profile.
Although phasor magnitudes and angles depend upon the synchrony between the 24-h light-dark and activity-rest patterns, irrespective of absolute levels of CS and AI, it is important to note that the average daily CS and AI levels are both quite similar for the two subjects. Further, the CS and AI levels for these two subjects are like those for subjects from other similar studies (e.g., Figueiro et al., 2012). Moreover, their average CS levels are typical of subjects who spend most of their active hours in indoor spaces (Figueiro et al., 2019; Figueiro et al., 2020). What is more, it is noteworthy that their daytime CS levels, presumably associated with commercial workspaces, are higher than those associated with their evening light levels, presumably associated with residences (Rea et al., 2020). Again, this difference between daytime and evening CS levels is typical of subjects from other studies. The grey-value gradient in Figure 1B illustrates CS levels that would likely be experienced outdoors at night, indoors at night, indoors during the day, and outdoors during the day. The average CS levels during daytime and during evening from Figures 3A,B are, as would be expected, consistent with the nominal categories illustrated in Figure 1B.
2.2 Days to re-entrainment (DTR)
As previously noted, the CS-oscillator model permits estimations of CBTmin clock time from a subject’s daily (24-h) personal light-dark exposure profiles (measured in terms of CS), like those shown in Figures 3A,B. CBTmin is both an input to the CS-oscillator model and an output from the model. To begin the process of estimating CBTmin, an initial estimated value of CBTmin is entered into the model along with the personal light-dark exposure pattern representing several (in this study, seven) 24-h days of time-series light exposure data for an individual. The CS-oscillator model calculates the individual’s new CBTmin clock time based on the light exposure profile, to a precision of 0.01 h. To identify an individual’s baseline CBTmin time, assuming a continuous 7-day weekly light exposure profile that repeats indefinitely, the CS-oscillator model is run iteratively using the calculated CBTmin time from the previous run as its input, until the resulting CBTmin time does not change within 0.01 h.
For the re-entrainment analyses, the initial CBTmin value was the asymptotic CBTmin for the baseline period, then shifted by 1 h to represent the immediate clock-time shift associated with the transition to or from DST. Several iterations of the model calculations were then conducted with the same 7-day light-dark exposure pattern. After each iteration, a new CBTmin value was output from the model, representing the CBTmin time resulting from the previous estimated CBTmin value and the light-dark exposure pattern. The resulting CBTmin is then entered into the model again and, using the same light-dark exposure pattern, the next CBTmin is output. Eventually, the estimated CBTmin would reach an asymptotic value.
As an example of this process, the solid line in Figure 4 shows how CBTmin would change during a series of model iterations when clock time had been advanced 1 h (Δ = 60 min) as would occur in the spring following the transition from ST to DST. To estimate the DTR following a time change in the spring and in the autumn, a criterion shift in CBTmin of 50 min (Δ = 50 min) was selected for all model iterations in the present study. This criterion was selected for three reasons; first, because as shown in Figure 4, a shift approaching 60 min can take as much as three times longer to achieve than a shift of 50 min. Second, the CS-oscillator model’s precision level of 0.01 h, while mathematically accurate, is not necessarily accurate in real-world conditions where other factors such as diet (Potter et al., 2016) or exercise (Youngstedt et al., 2019) can effect small changes in circadian phase. Third, as asymptotic model predictions can be unreliable (Sandberg et al., 2021), and a 50-min criterion shift is close to the inflection point of the re-entrainment curve in Figure 4 where it changes from very steep to nearly flat, using this criterion provides a more reliable estimate of the relative time needed to re-entrain following DST-related transitions. In the example shown in Figure 4, the DTR is equal to 9 days. The baseline, or initial, CBTmin for both subjects, based strictly upon their personal light-dark exposure pattern, is shown in Table 1.
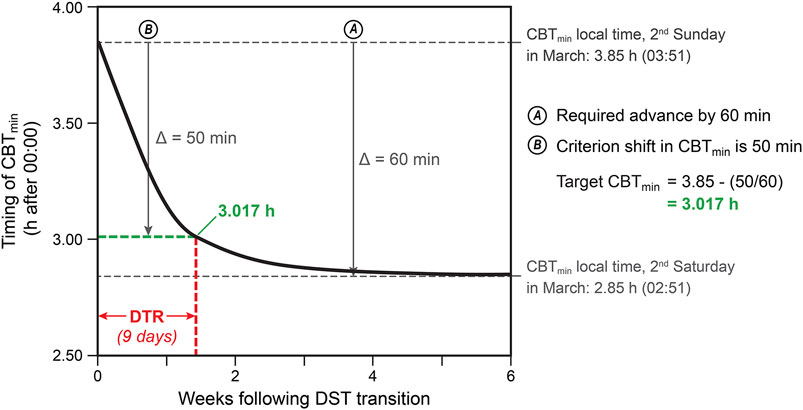
Figure 4. Illustration of the predicted change in CBTmin (Rea et al., 2022), represented by the heavy black line, for subject A following the transition to DST.
In estimating DTR, we explicitly assumed that the activity-rest pattern would be governed by the local clock time for the two working subjects without regard to a change in local time from ST to DST, and vice versa (e.g., people must get to work at the same clock time before and after a seasonal change in local time). Further, because these two subjects were (presumably) exposed only to indoor lighting the relationship between the activity-rest pattern and the light-dark pattern would always remain the same. Thus, in terms of local clock time, the relationship between their sleep time, including bedtime, midsleep, and wake time, and their personal light-dark exposure pattern would remain unchanged.
To determine the effects of the instantaneous change in local clock time from ST to DST and from DST to ST, we re-assigned the previous CBTmin value (e.g., 04:00) to the new CBTmin after the transition, which would be 1 h later (e.g., 05:00) in the spring and 1 h earlier (e.g., 03:00) in the autumn with respect to the local clock time prior to the clock time change. This places the baseline estimated CBTmin from the CS-oscillator model at odds with the new, shifted CBTmin. Again, keeping the relationship between light-dark pattern and the activity-rest pattern fixed before and after the shift in local clock time, we were then able to determine how many iterative cycles, or days, it would take for CBTmin to reach the 50-min criterion shift to the new local clock time. In other words, we were able to determine how long it would take to return to the same temporal difference between CBTmin and local clock wake time as before the change in local time; this difference was approximately 3 h for both subjects (Table 1).
For our analyses we further assumed that the two subjects either resided/worked in Boston, near the eastern border of the Eastern Time Zone, or in Detroit, at a similar latitude but near the western border of the Eastern Time Zone, where sunrise is 50 min later.
3 Results
3.1 DTR without light intervention
The baseline CBTmins prior to the change in local time and the CBTmins after the change in local time and the resulting predicted DTRs for Boston and for Detroit were determined for both subjects. Since these two subjects only experienced indoor lighting, the 50-min difference in sunrise had, as expected, little or no effect on DTR for both the lark and the owl. Given their personal light-dark exposure patterns were different, however, it would take the owl longer than the lark to re-entrain to the time changes, both in the spring and in the autumn (Table 2).
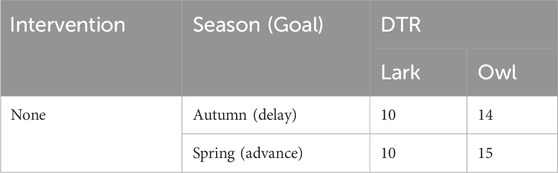
Table 2. Days to re-entrainment (DTR) for the lark and the owl following the transitions between DST and ST, with no change in light-dark exposure profiles relative to clock time.
As noted in Section 1.4, our primary goal in this study was to explore the impact of different practical light interventions that might be taken by larks and or owls following the change from ST to DST, and vice versa, to determine if and how DTR could be reduced, thereby minimizing the duration of circadian disruption due to the sudden change in local clock time. Three presumably practical calibrated light interventions were selected for modeling with the CS-oscillator model. Each light intervention was added to the light-dark profiles in Figures 3A,B, quantified in terms of the amount (CS) and duration (hours) and by the local time it was applied. It should be emphasized that these interventions are intended to be carried out after the transition to DST or ST and would be expected to cease once re-entrainment was established. An unchanged indoor behavioral profile before and after the transition will ensure continual entrainment of the stabilized circadian phase to the original, unchanged, light exposure profile.
3.2 DTR with light interventions
3.2.1 Self-luminous display
A recent meta-analysis investigating changes in screen time following the COVID-19 pandemic revealed that leisure (non-work/non-academic) screen time has increased by 0.7 h per day in adults (Trott et al., 2022). Several studies have characterized light exposures at the eye from self-luminous displays. For instance, Gringras et al. (2015) reported that smartphones (iPhone 5S, Apple Inc., Cupertino, CA, United States) can deliver a light level of 51 lx at the eye when operated from a typical reading distance of about 22.5 cm. For typical self-luminous spectra, this would translate into a CS value of 0.12. With regard to self-luminous displays, Wood et al. (2013) and Nagare et al. (2019a) have reported that iPads (iPad Air 2 and IPad 2, respectively, Apple Inc., Cupertino, CA, United States) deliver around 70 lx at the eye for an average viewing distance of 30.5 cm, or a CS of 0.13. For this virtual intervention, it was assumed that both the lark and the owl viewed a self-luminous display (CS = 0.13) for 30 min beginning at 19:00, around dinner time. For the two profiles under investigation in this paper, this clock time corresponds to the end of higher interior light level exposures (e.g., those from the workplace during the day) and the beginning of lower interior light level exposures (e.g., those from typical of a residence indoors) (Rea, 2000).
3.2.2 Trip to Florida
It is not uncommon for people in Boston and in Detroit, having approximately the same north latitude (42°N), to vacation in Miami, which is in the same time zone but much further south (28°N) during the colder months. In March during the change in local clock time, the day lengths in all three cities are approximately 12 h. In November, during this change, the day lengths are slightly shorter (10 h) in the northern cities than in Miami (11 h). One would expect the time spent outdoors in Miami would be longer than it would be in Boston and Detroit because of warmer weather, particularly when a person is on vacation. For this virtual intervention, it was assumed that the lark and the owl residing in Boston and the lark and the owl residing in Detroit both flew to Miami the Saturday evening of the clock change in spring and autumn. They then spent the next week outdoors in bright daylight. For this virtual intervention it was assumed that the lark and the owl kept the same activity-rest cycle they had exhibited in their respective cities. Because the owl would get up well past sunrise, however, the duration of their daylight exposure would naturally be less (9 h) than it would be for the lark (11 h). To simulate the light levels they would experience while outdoors in daylight, the recorded CLA light exposure profiles (for the daylight hours) in Figures 3A,B were all multiplied by a factor of 10, a representative multiplier for outdoor versus indoor light exposures for many individuals (Rea, 2000).
3.2.3 Morning walk
Recent statistics suggest that about 49 million people in the U.S. reported engaging in running and jogging activities in 2021 (Statista, 2024). An even greater number of people (115 million) reported engaging in walking for fitness activities during the same year. In fact, the physical activity guidelines from the American Heart Association recommend at least 150 min of moderate intensity aerobic activity per week (>30 min per weekday) to improve health and wellbeing (American Heart Association, 2024). It has been well-documented that even on cloudy days, daylight can deliver very high light levels at the eye (CS ≥ 0.5). For this virtual intervention, it was assumed that the lark and the owl went for an outdoor (CS = 0.5) morning walk for 45 min, 30 min after waking.
The results of the three intervention simulations are shown in Table 3. In some cases in Table 3, an asterisk (*) indicates when the intervention shifted the individual in the wrong direction for the DST-related transition in clock time (e.g., when an intervention caused a phase advance but the DST-related transition called for a phase delay). The DTRs in both Boston and Detroit were the same for these virtual light interventions. (A separate virtual light intervention comparing Boston and Detroit is discussed later to illustrate how a later sunrise in Detroit would affect predicted changes in circadian phase.) Of particular interest, the DTR values in Table 3 show that the same intervention can produce very different outcomes depending upon the season when the local time change occurred. As a prime example, a 45-min morning walk in the spring accelerates re-entrainment because it provides bright light exposure to advance circadian phase, but that same walk in the autumn prevents re-entrainment because it is counter to one wanting to delay circadian phase.
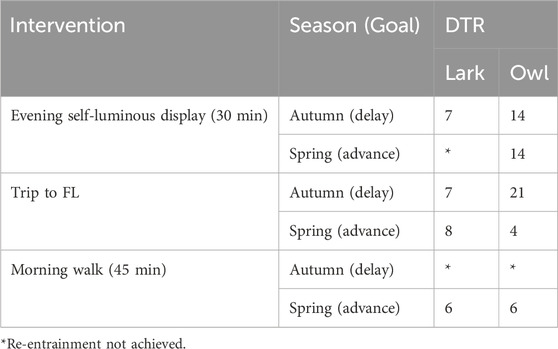
Table 3. Days to re-entrainment (DTR) for the lark and the owl for different post-transition interventions.
It is also interesting to consider light at night by the evening self-luminous display exposure. This has no effect on the owl because the timing of the light exposure is well before their CBTmin and outside both the delay and advance phase response to light (see Table 2). However, the same evening light exposure has a profound effect on the lark because, again, the timing of the light exposure is during the delay phase response to light and closer to their CBTmin.
As already noted, these virtual light interventions had little or no differential effect between Boston and Detroit for the morning walk or for the evening self-luminous display. The reason is that these two light interventions simulated here did not differentially influence DTR because they were not differentially exposed to daylight. The self-luminous display exposure in both Boston and Detroit was never accompanied by daylight because it was viewed indoors and the morning walkers in both Detroit and Boston were outside after sunrise. Suppose the morning walks were taken by the lark immediately after waking during the springtime, rather than 30 min after waking (which resulted in a DTR of 6 days according to Table 3). In this case, the earlier exposure to daylight during the walk in Boston would have advantaged the lark in Boston (reducing DTR from six to 5 days). However, because the sun would not have risen in Detroit at the same clock time, the earlier walk would disadvantage the lark (increasing DTR from 6 days to 9 days). For the owl, there is less difference because the sun has already risen by the time the owl wakes up, regardless of location within the time zone.
4 Discussion
There has been a great deal of discussion among politicians, bureaucrats, and the public about the wisdom of preserving or eliminating DST. Some people voice a preference for ST all year long while others prefer to have DST throughout the year. Others, perhaps the majority, like the fact that there are two yearly changes in local clock time so that, with respect to local clock time, they can commute to work in daylight during the winter and can enjoy daylight on the patio or in the backyard during the summer (Coogan et al., 2022).
Many chronobiologists do not like the seasonal changes in local time because most people live by local clock time, not biological time. This can create a sudden change in the light-dark exposure cycle relative to clock time which governs the activity-rest cycle. Chronobiologists know that this sudden disparity between clock time and biological time creates circadian disruption which has been linked to poor sleep (Harrison, 2013), accidents (Sullivan and Flannagan, 2002; Lahti et al., 2010), and even mortality (Poteser and Moshammer, 2020). As a result, many chronobiologists have argued for discontinuation of the seasonal changes in local time, often favoring ST over DST.
The analyses performed for this study suggest that DTR following a change in local time can be accelerated or prolonged indefinitely depending upon the light-dark exposure cycle. The heuristic that light can both delay and advance circadian phase is certainly not new, but what is new is the potential of the CS-oscillator model to quantitatively guide individuals experiencing a change in local time so that they can minimize DTR and thus minimize the duration of circadian disruption, based on the chronotype and lifestyle. It is important to consider, at a minimum, an individual’s chronotype (i.e., owl vs. lark) because the analyses here demonstrate that different chronotypes will respond differently to interventions like those discussed in this paper. Because of this, some advice given to the general population (e.g., “get daylight exposure in the morning” (Suni, 2024)) may not always be applicable or beneficial for re-entrainment after ST/DST transitions.
Directionally, everyone knows that the biological clock must, with respect to the new local clock time, advance in the spring and delay in the autumn. Everyone also knows that re-entrainment of the biological clock to the new clock time is not instantaneous. The exact number of days to re-entrain has not been clear; some have some have reported the time to re-entrain is 7 days (Monk and Folkard, 1976), but others say it can be accomplished in 2 days (Lahti et al., 2010). The present study suggests that “doing nothing different” after the local clock time change requires 10–15 days for re-entrainment.
Two things should be made clear about this exercise. First, the modeled quantitative predictions are just that, predictions. They are offered here based upon the latest science, but any and all predictions are and should be subject to empirical hypothesis testing. These predictions are only as good as the accuracy of information within the three domains described in Section 1 (the retinal response to light, ambulatory light data and the response of the biological clock). Without that information it would be difficult to predict the magnitude or even the direction that a change in the light-dark cycle might drive the biological clock.
Second, although we selected two real examples of daily light profiles and life-style activity patterns for analysis, the generalizability of the predictions for all people is limited. The two subjects that we selected were ones who had limited exposure to daylight. This seemed reasonable since the large majority of human activity is carried out indoors (Cox-Ganser and Henneberger, 2021). These data were also collected following the worldwide COVID-19 pandemic and may reflect a greater likelihood than before to stay indoors at home (Gold, 2023). We modified these two actual light-dark profiles to determine how additional, virtual, “practical” light interventions might affect predictions of the direction of circadian shift and the time to re-entrainment. We held lifestyle activity patterns constant assuming that people’s work and social activities are governed mainly by the local time, not biological time. There are literally an infinite number of daily light profiles and daily lifestyle activity patterns that are possible to model, including special ones like those experienced by farmers (bright light all day), computer analysts (dim light all day), flight attendants (non-24-h light exposures), and firefighters (shift workers). The two profiles we used in this paper could not possibly be indicative of the entire Western population, but they are typical of “morning” (lark) and “evening” (owl) types (Lack et al., 2009). Still, there is a great deal more “custom” work that needs to be undertaken to predict helpful, individualized light interventions, including better understanding the slower circadian adaptation of older adults (Costa, 2003) and those with large circadian rhythm amplitudes (Reinberg et al., 1978). We hope, however, that the CS-oscillator model can be a useful tool for enhancing our understanding of these and related factors.
Notwithstanding, and assuming that our two cases are not outliers, the guidance in Figure 5 is offered as “extended heuristics” to suggest light interventions that might help with the spring and autumn changes in local clock time. The green and red hues represent the direction of phase change induced by the light intervention with respect to the goal, either advance or delay. The hue saturation represents the magnitude of the direction induced by the light intervention. As previously mentioned, these three extended heuristic interventions are meant to be temporary, only occurring after the transition to DST or to ST until re-entrainment is achieved. Further, based on the underlying light exposure profiles we evaluated, the predictions described here apply to individuals who are primarily exposed to indoor lighting, a large fraction of the population.
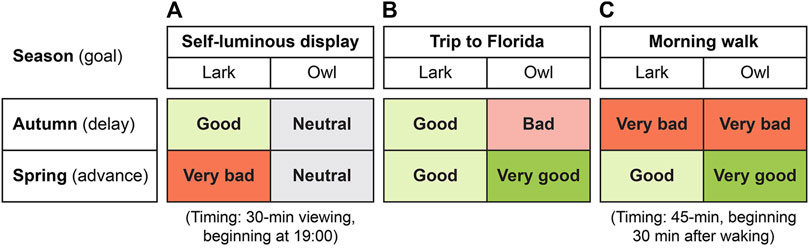
Figure 5. Qualitative impacts of the three interventions (with timing note, where applicable) for larks and owls immediately after the autumn and spring DST/ST transitions: self-luminous display (A), trip to Florida (B), morning walk (C).
5 Conclusion
The CS-oscillator model (Rea et al., 2022) used in the present study provides “extended heuristics” that probably can help people cope better with the twice-seasonal changes in local time associated with DST. Whereas these twice-seasonal changes in local time affect nearly everyone, their negative impact on the circadian system is probably small with respect to those induced by irregular light exposures experienced by individuals who engage in shift work or frequent air travel across multiple time zones. The CS-oscillator model is potentially important for developing strategies to minimize circadian disruption in these particularly vulnerable populations, but the model’s predictions need to be verified empirically and extended to more extreme chronotypes and to shift workers before formal implementation.
Data availability statement
The raw data supporting the conclusions of this article will be made available by the authors, without undue reservation.
Ethics statement
Ethical approval was not required for the study involving humans in accordance with the local legislation and institutional requirements. Written informed consent to participate in this study was not required from the participants or the participants’ legal guardians/next of kin in accordance with the national legislation and the institutional requirements.
Author contributions
MR: Conceptualization, Formal Analysis, Investigation, Methodology, Project administration, Supervision, Validation, Visualization, Writing–original draft, Writing–review and editing. RN: Conceptualization, Data curation, Formal Analysis, Investigation, Methodology, Resources, Software, Validation, Visualization, Writing–original draft, Writing–review and editing. JB: Formal Analysis, Investigation, Validation, Visualization, Writing–original draft, Writing–review and editing. MF: Conceptualization, Funding acquisition, Methodology, Project administration, Supervision, Validation, Writing–review and editing.
Funding
The author(s) declare that financial support was received for the research, authorship, and/or publication of this article. The authors declare financial support was received from National Institute on Aging grants 5R44AG060857 (MR, RN, JB, and MF) and 5R01AG034157 (MR, RN, JB, and MF) for the research, authorship, and publication of this article. The development of the CS-oscillator model used in this paper had been sponsored by the Army Research Office, contract W911NF2110183 (MR and MF).
Acknowledgments
The authors wish to acknowledge Andrew Bierman, who developed the basic code for the CS-oscillator model.
Conflict of interest
The authors declare that the research was conducted in the absence of any commercial or financial relationships that could be construed as a potential conflict of interest.
Publisher’s note
All claims expressed in this article are solely those of the authors and do not necessarily represent those of their affiliated organizations, or those of the publisher, the editors and the reviewers. Any product that may be evaluated in this article, or claim that may be made by its manufacturer, is not guaranteed or endorsed by the publisher.
Abbreviations
AI, Activity index; CBTmin, Core body temperature minimum; CIE, Commission Internationale de l’Éclairage; CLA, Circadian light; CS, Circadian stimulus; DST, Daylight saving time; DTR, Days to re-entrainment; ipRGC, Intrinsically photosensitive retinal ganglion cell; MAE, Mean absolute error; RHT, Retinohypothalamic tract; RMS, Root mean square; SCN, Suprachiasmatic nuclei; ST, Standard time.
References
American Heart Association (2024). American Heart association Recommendations for physical activity in adults and kids. Dallas, TX: American Heart Association. Available at: https://www.heart.org/en/healthy-living/fitness/fitness-basics/aha-recs-for-physical-activity-in-adults (Accessed February 8, 2024).
Appleman, K., Figueiro, M. G., and Rea, M. S. (2013). Controlling light-dark exposure patterns rather than sleep schedules determines circadian phase. Sleep. Med. 14, 456–461. doi:10.1016/j.sleep.2012.12.011
Aschoff, J. (1965). Circadian clocks: proceedings of the Feldafing summer School, 7-18 September 1964. Amsterdam: North-Holland Publishing Company.
Barlow, H. B. (1972) “Dark and Light Adaptation: Psychophysics,” in Visual Psychophysics. Editor M. Alpern, E. Aulhorn, H.B. Barlow, E. Baumgardt, H.R. Blackwell, and D.S. Blough Berlin, Heidelberg: Springer Berlin Heidelberg, 1–28.
Bierman, A., Klein, T. R., and Rea, M. S. (2005). The Daysimeter: a device for measuring optical radiation as a stimulus for the human circadian system. Meas. Sci. Technol. 16, 2292–2299. doi:10.1088/0957-0233/16/11/023
Bonmati-Carrion, M. A., Arguelles-Prieto, R., Martinez-Madrid, M. J., Reiter, R., Hardeland, R., Rol, M. A., et al. (2014). Protecting the melatonin rhythm through circadian healthy light exposure. Int. J. Mol. Sci. 15, 23448–23500. doi:10.3390/ijms151223448
Carrier, J., Monk, T. H., Reynolds, C. F., Buysse, D. J., and Kupfer, D. J. (1999). Are age differences in sleep due to phase differences in the output of the orcadian timing system? Chronobiol. Int. 16, 79–91. doi:10.3109/07420529908998714
Commission Internationale De L'éclairage (1926). Commission Internationale de l'Éclairage Proceedings, 1924. Vienna: Commission Internationale de l'Éclairage.
Coogan, A. N., Richardson, S., and Raman, S. (2022). A data-informed perspective on public preferences for retaining or abolishing biannual clock changes. J. Biol. Rhythms 37, 351–357. doi:10.1177/07487304221096390
Costa, G. (2003). Factors influencing health of workers and tolerance to shift work. Theor. Issues Ergon. Sci. 4, 263–288. doi:10.1080/14639220210158880
Cox-Ganser, J. M., and Henneberger, P. K. (2021). Occupations by proximity and indoor/outdoor work: relevance to COVID-19 in all workers and Black/Hispanic workers. Am. J. Prev. Med. 60, 621–628. doi:10.1016/j.amepre.2020.12.016
Delean, A., Munson, P. J., and Rodbard, D. (1978). Simultaneous analysis of families of sigmoidal curves: application to bioassay, radioligand assay, and physiological dose-response curves. Am. J. Physiol. 235, E97–E102. doi:10.1152/ajpendo.1978.235.2.E97
Evans, L. S., Peachey, N. S., and Marchese, A. L. (1993). Comparison of three methods of estimating the parameters of the Naka-Rushton equation. Doc. Ophthalmol. 84, 19–30. doi:10.1007/BF01203279
Figueiro, M. G., Hamner, R., Bierman, A., and Rea, M. S. (2013). Comparisons of three practical field devices used to measure personal light exposures and activity levels. Light. Res. Technol. 45, 421–434. doi:10.1177/1477153512450453
Figueiro, M. G., Kalsher, M., Steverson, B. C., Heerwagen, J., Kampschroer, K., and Rea, M. S. (2019). Circadian-effective light and its impact on alertness in office workers. Light. Res. Technol. 51, 171–183. doi:10.1177/1477153517750006
Figueiro, M. G., Plitnick, B., and Rea, M. S. (2014). The effects of chronotype, sleep schedule and light/dark pattern exposures on circadian phase. Sleep. Med. 15, 1554–1564. doi:10.1016/j.sleep.2014.07.009
Figueiro, M. G., Rea, M. S., and Hamner, R. (2012). “Calibrated personal light exposures as they might affect melatonin suppression in different populations,” in Proceedings of experiencing light 2012, eindhoven, NL, 12-13 November 2012 (Eindhoven, NL: Eindhoven University of Technology Library). Available at: http://www.2012.experiencinglight.nl/doc/9.pdf.
Figueiro, M. G., Steverson, B., Heerwagen, J., Yucel, R., Roohan, C., Sahin, L., et al. (2020). Light, entrainment and alertness: a case study in offices. Light. Res. Technol. 52, 736–750. doi:10.1177/1477153519885157
Gale, C., and Martyn, C. (1998). Larks and owls and health, wealth, and wisdom. BMJ 317, 1675–1677. doi:10.1136/bmj.317.7174.1675
Gold, J. (2023). Covid has turned many homeowners into Homebodies, New Survey Reveals. Forbes. Available at: https://www.forbes.com/sites/jamiegold/2023/04/25/covid-has-turned-many-homeowners-into-homebodies-new-survey-reveals/?sh=2da4a57b63d0 (Accessed February 8, 2024).
Gringras, P., Middleton, B., Skene, D. J., and Revell, V. L. (2015). Bigger, brighter, bluer-better? Current light-emitting devices - adverse sleep properties and preventative strategies. Front. Public Health 3, 233. doi:10.3389/fpubh.2015.00233
Harrison, Y. (2013). The impact of daylight saving time on sleep and related behaviours. Sleep. Med. Rev. 17, 285–292. doi:10.1016/j.smrv.2012.10.001
Huang, Y., Mayer, C., Cheng, P., Siddula, A., Burgess, H. J., Drake, C., et al. (2021). Predicting circadian phase across populations: a comparison of mathematical models and wearable devices. Sleep 44, zsab126. doi:10.1093/sleep/zsab126
Jansen, J., and Halbertsma, N. A. (1951). Collection of proceedings and report of sessions, twelfth session of the International Commission on llumination, Stockholm, June and July, 1951. New York: Bureau Central de la CIE.
Kronauer, R. E. (1990). “A quantitative model for the effects of light on the amplitude and phase of the deep circadian pacemaker, based on human data,” in Sleep ‘90, proceedings of the 10th ESRS congress. Editor J. Horne 1st edn. (Bochum, Germany: Pontenagel Press), 306–309.
Kronauer, R. E., Forger, D. B., and Jewett, M. E. (1999). Quantifying human circadian pacemaker response to brief, extended, and repeated light stimuli over the phototopic range. J. Biol. Rhythms 14, 501–516. doi:10.1177/074873049901400609
Lack, L., Bailey, M., Lovato, N., and Wright, H. (2009). Chronotype differences in circadian rhythms of temperature, melatonin, and sleepiness as measured in a modified constant routine protocol. Nat. Sci. Sleep. 1, 1–8. doi:10.2147/nss.s6234
Lahti, T., Nysten, E., Haukka, J., Sulander, P., and Partonen, T. (2010). Daylight saving time transitions and road traffic accidents. J. Environ. Public Health 2010, 1–3. doi:10.1155/2010/657167
Meir, R. (2002). Managing transmeridian travel: guidelines for minimizing the negative impact of international travel on performance. Strength Cond. J. 24, 28–34. doi:10.1519/1533-4295(2002)024<0028:mttgfm>2.0.co;2
Miller, B. H., and Takahashi, J. S. (2014). Central circadian control of female reproductive function. Front. Endocrinol. (Lausanne) 4, 195. doi:10.3389/fendo.2013.00195
Miller, D., Figueiro, M. G., Bierman, A., Schernhammer, E., and Rea, M. S. (2010). Ecological measurements of light exposure, activity and circadian disruption. Light. Res. Technol. 42, 271–284. doi:10.1177/1477153510367977
Monk, T. H., and Folkard, S. (1976). Adjusting to the changes to and from daylight saving time. Nature 261, 688–689. doi:10.1038/261688a0
Mott, C., Dumont, G., Boivin, D. B., and Mollicone, D. (2011). Model-based human circadian phase estimation using a particle filter. IEEE Trans. Biomed. Eng. 58, 1325–1336. doi:10.1109/TBME.2011.2107321
Nagare, R., Plitnick, B., and Figueiro, M. G. (2019a). Does the iPad Night Shift mode reduce melatonin suppression? Light. Res. Technol. 51, 373–383. doi:10.1177/1477153517748189
Nagare, R., Plitnick, B., and Figueiro, M. G. (2019b). Effect of exposure duration and light spectra on nighttime melatonin suppression in adolescents and adults. Light. Res. Technol. 51, 530–543. doi:10.1177/1477153518763003
Nagare, R., Rea, M. S., Plitnick, B., and Figueiro, M. G. (2019c). Effect of white light devoid of “cyan” spectrum radiation on nighttime melatonin suppression over a 1-h exposure duration. J. Biol. Rhythms 34, 195–204. doi:10.1177/0748730419830013
Nagare, R., Rea, M. S., Plitnick, B., and Figueiro, M. G. (2019d). Nocturnal melatonin suppression by adolescents and adults for different levels, spectra, and durations of light exposure. J. Biol. Rhythms 34, 178–194. doi:10.1177/0748730419828056
Poteser, M., and Moshammer, H. (2020). Daylight saving time transitions: impact on total mortality. Int. J. Env. Res. Public Health 17, 1611. doi:10.3390/ijerph17051611
Potter, G. D. M., Cade, J. E., Grant, P. J., and Hardie, L. J. (2016). Nutrition and the circadian system. Br. J. Nutr. 116, 434–442. doi:10.1017/S0007114516002117
Qin, L.-Q., Li, J., Wang, Y., Wang, J., Xu, J.-Y., and Kaneko, T. (2003). The effects of nocturnal life on endocrine circadian patterns in healthy adults. Life Sci. 73, 2467–2475. doi:10.1016/S0024-3205(03)00628-3
Rea, M. S. (2000). The IESNA lighting handbook: reference and application (New York: Illuminating Engineering Society).
Rea, M. S., Bierman, A., Figueiro, M. G., and Bullough, J. D. (2008). A new approach to understanding the impact of circadian disruption on human health. J. Circadian Rhythms 6, 7. doi:10.1186/1740-3391-6-7
Rea, M. S., Figueiro, M. G., Bierman, A., and Bullough, J. D. (2010). Circadian light. J. Circadian Rhythms 8, 2. doi:10.1186/1740-3391-8-2
Rea, M. S., Nagare, R., Bierman, A., and Figueiro, M. G. (2022). The circadian stimulus-oscillator model: improvements to Kronauer's model of the human circadian pacemaker. Front. Neurosci. 16, 965525. doi:10.3389/fnins.2022.965525
Rea, M. S., Nagare, R., and Figueiro, M. G. (2021a). Modeling circadian phototransduction: quantitative predictions of psychophysical data. Front. Neurosci. 15, 615322, doi:10.3389/fnins.2021.615322doi:10.3389/fnins.2021.615322
Rea, M. S., Nagare, R., and Figueiro, M. G. (2021b). Modeling circadian phototransduction: retinal neurophysiology and neuroanatomy. Front. Neurosci. 14, 615305, doi:10.3389/fnins.2020.615305doi:10.3389/fnins.2020.615305
Rea, M. S., Nagare, R. M., and Figueiro, M. G. (2020). Predictions of melatonin suppression during the early biological night and their implications for residential light exposures prior to sleeping. Sci. Rep. 10, 14114. doi:10.1038/s41598-020-70619-5
Rea, M. S., Plitnick, B., and Figueiro, M. G. (2016) “Effect of custom blue light intervention on dim light melatonin onset in healthy adults. Unpublished work, available from the Light and Health Research Center, Icahn School of Medicine at Mount Sinai, New York.
Refinetti, R. (2020). Circadian rhythmicity of body temperature and metabolism. Temperature 7, 321–362. doi:10.1080/23328940.2020.1743605
Reinberg, A., Vieux, N., Ghata, J., Chaumont, A. J., and Laporte, A. (1978). Is the rhythm amplitude related to the ability to phase-shift circadian rhythms of shift-workers? J. Physiol. Paris. 74, 405–409.
Reiter, R. J., Tan, D.-X., Korkmaz, A., Erren, T. C., Piekarski, C., Tamura, H., et al. (2007). Light at night, chronodisruption, melatonin suppression, and cancer risk: a review. Crit. Rev. Oncogen. 13, 303–328. doi:10.1615/critrevoncog.v13.i4.30
Roenneberg, T., Wirz-Justice, A., and Merrow, M. (2003). Life between clocks: daily temporal patterns of human chronotypes. J. Biol. Rhythms 18, 80–90. doi:10.1177/0748730402239679
Sandberg, A., Armstrong, S., Gorman, R., and England, R. (2021). Sigmoids behaving badly: why they usually cannot predict the future as well as they seem to promise. Rochester, NY: Social Science Research Network Elsevier. Available at: https://ssrn.com/abstract=3926169 (Accessed April 16, 2024).
Sharkey, K. M., Carskadon, M. A., Figueiro, M. G., Zhu, Y., and Rea, M. S. (2011). Effects of an advanced sleep schedule and morning short wavelength light exposure on circadian phase in young adults with late sleep schedules. Sleep. Med. 12, 685–692. doi:10.1016/j.sleep.2011.01.016
Smolensky, M. H., Sackett-Lundeen, L. L., and Portaluppi, F. (2015). Nocturnal light pollution and underexposure to daytime sunlight: complementary mechanisms of circadian disruption and related diseases. Chronobiol. Int. 32, 1029–1048. doi:10.3109/07420528.2015.1072002
Statista (2024). Running and jogging - statistics and facts Hamburg, DE: statista.com. Available at: https://www.statista.com/topics/1743/running-and-jogging/#topicOverview (Accessed 8 February 2024).
St Hilaire, M. A., Klerman, E. B., Khalsa, S. B., Wright, K. P., Czeisler, C. A., and Kronauer, R. E. (2007). Addition of a non-photic component to a light-based mathematical model of the human circadian pacemaker. J. Theor. Biol. 247, 583–599. doi:10.1016/j.jtbi.2007.04.001
Sullivan, J. M., and Flannagan, M. J. (2002). The role of ambient light level in fatal crashes: inferences from daylight saving time transitions. Accid. Anal. Prev. 34, 487–498. doi:10.1016/S0001-4575(01)00046-X
Suni, E. (2024). How to prepare for the start and end of daylight saving time. Sleep Foundation. Available at: https://www.sleepfoundation.org/circadian-rhythm/how-to-prepare-for-daylight-saving-time (Accessed February 6, 2024).
Swamy, S., Xie, X., Kukino, A., Calcagno, H. E., Lasarev, M. R., Park, J. H., et al. (2018). Circadian disruption of food availability significantly reduces reproductive success in mice. Horm. Behav. 105, 177–184. doi:10.1016/j.yhbeh.2018.07.006
Trott, M., Driscoll, R., Iraldo, E., and Pardhan, S. (2022). Changes and correlates of screen time in adults and children during the COVID-19 pandemic: a systematic review and meta-analysis. eClinicalMedicine 48, 101452. doi:10.1016/j.eclinm.2022.101452
Tumin, R. (2023). How to make the most of the morning light. New York Times. Available at: https://www.nytimes.com/2023/11/03/well/daylight-saving-tips.html (Accessed February 7, 2024).
Ul Standards and Engagement (2019). Design guideline for promoting circadian entrainment with light for day-active people, design guideline 24480. 1st Edn. Northbrook, IL: Underwriters Laboratories.
U.S. Environmental Protection Agency (1989) “Assessment and control of indoor air pollution,” in Report to congress on indoor air quality, 2. Washington, DC: U.S. Environmental Protection Agency. Report number PB-90-167396/XAB; EPA-400/1-89/001C.
Woelders, T., Beersma, D. G. M., Gordijn, M. C. M., Hut, R. A., and Wams, E. J. (2017). Daily light exposure patterns reveal phase and period of the human circadian clock. J. Biol. Rhythms 32, 274–286. doi:10.1177/0748730417696787
Wood, B., Rea, M. S., Plitnick, B., and Figueiro, M. G. (2013). Light level and duration of exposure determine the impact of self-luminous tablets on melatonin suppression. Appl. Ergon. 44, 237–240. doi:10.1016/j.apergo.2012.07.008
Keywords: circadian disruption, circadian entrainment, CS-oscillator, daylight saving time, light interventions, retinal light exposures
Citation: Rea MS, Nagare R, Bullough JD and Figueiro MG (2024) Days to re-entrainment following the spring and autumn changes in local clock time: beyond simple heuristics. Front. Photonics 5:1386703. doi: 10.3389/fphot.2024.1386703
Received: 15 February 2024; Accepted: 27 June 2024;
Published: 22 July 2024.
Edited by:
Bahman Anvari, University of California, Riverside, United StatesReviewed by:
Ziquan Guo, Xiamen University, ChinaMichael Smolensky, The University of Texas at Austin, United States
Copyright © 2024 Rea, Nagare, Bullough and Figueiro. This is an open-access article distributed under the terms of the Creative Commons Attribution License (CC BY). The use, distribution or reproduction in other forums is permitted, provided the original author(s) and the copyright owner(s) are credited and that the original publication in this journal is cited, in accordance with accepted academic practice. No use, distribution or reproduction is permitted which does not comply with these terms.
*Correspondence: Mark S. Rea, mark.rea@mountsinai.org