- 1University of Strasbourg, ICube Laboratory, Illkirch, France
- 2Research Institute Against Digestive Cancer (IRCAD), Strasbourg, Alsace, France
- 3Department of General and Pancreatic Surgery—The Pancreas Institute, University of Verona, Verona, Italy
- 4Department of Gastrointestinal Surgery, San Raffaele Hospital IRCCS, Milan, Italy
- 5University of CA-Davis Medical Center, Department of Surgery, Sacramento, CA, United States
- 6INSA Strasbourg, Strasbourg, Alsace, France
- 7Intuitive Surgical, Aubonne, Switzerland
Despite recent technological progress in surgical guidance, current intraoperative assessment of tissue that should be removed (e.g., cancer) or avoided (e.g., nerves) is still performed subjectively. Optical imaging is a non-contact, non-invasive modality that has the potential to provide feedback regarding the condition of living tissues by imaging either an exogenously administered contrast agent or endogenous constituents such as hemoglobin, water, and lipids. As such, optical imaging is an attractive modality to provide physiologically and structurally relevant information for decision-making in real-time during surgery. The Trident imaging platform has been designed for real-time surgical guidance using state-of-the-art optical imaging. This platform is capable of dual exogenous and endogenous imaging owing to a unique filter and source combination, allowing to take advantage of both imaging modalities. This platform makes use of a real-time and quantitative imaging method working in the spatial frequency domain, called Single Snapshot imaging of Optical Properties (SSOP). The Trident imaging platform is designed to comply with all relevant standards for clinical use. In this manuscript, we first introduce the rationale for developing the Trident imaging platform. We then describe fluorescence and endogenous imaging modalities where we present the details of the design, assess the performance of the platform on the bench. Finally, we perform the validation of the platform during an in vivo preclinical experiment. Altogether, this work lays the foundation for translating state-of-the-art optical imaging technology to the clinic.
1 Introduction
The capabilities of near-infrared (NIR) optical imaging greatly expanded over the past few years, providing feedback for tissue status assessment during surgery, and particularly blood perfusion assessment. In the context of this work, several intraoperative optical imaging technologies allowing non-contact, large field-of-view macroscopic (i.e., greater than 100 cm2) imaging of the surgical field have been developed all the way from concept to clinical trials testing. These technologies differ from microscopic imaging technologies having smaller fields of view and requiring the instrument to be in contact or very close to the specimen. In this context, the development of fluorescence imaging for surgical guidance paved the way with the growing number of available contrast agents and the development of new macroscopic imaging technologies (Gioux et al., 2010; Gibbs, 2012; Vahrmeijer et al., 2013). However, the lack of quantitative capabilities leads to subjective assessment of fluorescence images, introducing variability in the surgical outcome (Pogue et al., 2018a; Pogue et al., 2018b; Mieog et al., 2022). Alternatively, the ability of multispectral and hyperspectral endogenous imaging to extract functional parameters for clinical applications has been reported for surgical guidance (Lu and Fei, 2014; Shapey et al., 2019; Clancy et al., 2020). Yet, it suffers from the lack of quantitative extraction of tissue constituents’ concentrations and cannot provide real-time feedback intraoperatively.
Spatial Frequency Domain Imaging (SFDI) is a relatively recent optical imaging method that has the capability to perform quantitative imaging of hemoglobin concentrations, and in turn of tissue oxygenation over large fields of view (>100 cm2) (Dognitz and Wagnieres, 1998; Cuccia et al., 2009; Gioux et al., 2019). Recent developments in acquisition and processing led to a real-time implementation of SFDI–namely Single Snapshot imaging of Optical Properties (SSOP) (Vervandier and Gioux, 2013; van de Giessen et al., 2015). The most advanced form of SSOP makes use of deep learning-based processing, leading to high-quality images while preserving the real-time quantitative capabilities of the method (Aguenounon et al., 2020; Smith et al., 2022).
To overcome the lack of tools capable of quantitative endogenous imaging for tissue assessment in clinical settings, we propose a novel imaging platform, called Trident, integrating the latest SSOP developments for performing real-time oxygenation imaging as well as fluorescence imaging. Combining these state-of-the-art imaging modalities in a single imaging platform enables the comparison of their respective performances as well as the potential for their combination.
In this manuscript, following a background section on optical imaging for surgery, the Trident imaging platform is described in detail, as well as the fluorescence and oxygenation imaging methods used. Next, bench characterization experiments are presented, showing the performances of the platform for optical properties imaging and fluorescence detection. Finally, in vivo validation demonstrates the ability of the imaging platform to dynamically detect ischemia in the small bowel by measuring tissue oxygenation and fluorescence in real-time.
2 Background
2.1 Quantitative optical imaging for surgery
Several implementations of either fluorescence imaging or oxygenation wide-field (>100 cm2) imaging exist in the literature (DSouza et al., 2016; Shapey et al., 2019; Clancy et al., 2020). Depending on the imaging methodology used, these implementations greatly vary in their capability to quantify the measured signal. Without trying to provide an extensive review, it is worth mentioning that the most common form of optical imaging for either fluorescence or oxygenation consists of using temporally and spatially constant illuminations (Bigio and Fantini, 2016). These methods are inherently limited in their capacity to quantify signals since they are unable to separate scattering from absorption, creating a crosstalk between the two sources of contrast. By contrast, methods using modulations in either time or space allow for the separation of scattering and absorption, thereby allowing a more accurate quantification of the signals.
In this work, we use a method called Spatial Frequency Domain Imaging (SFDI). A recent review has been published in the literature, explaining in detail the principles, acquisition and processing methods as well as applications (Gioux et al., 2019). In a nutshell, SFDI relies on the projection of a spatially modulated illumination pattern (also called structured illumination, typically a sinusoidally modulated pattern) and the quantification of the amplitude dampening of the reflectance pattern as a function of spatial frequency. A light propagation model is then used (typically Monte Carlo) to relate the dampening of the amplitude of the reflectance pattern to the optical properties of the medium (absorption coefficient and reduced scattering coefficient). Our group developed a real-time implementation of SFDI, called Single Snapshot of Optical Properties (SSOP) capable of capturing absorption and reduced scattering coefficients as well as profile information (for 3D profile correction) of a sample with only one image (Vervandier and Gioux, 2013; van de Giessen et al., 2015; Angelo et al., 2017). The latest implementation makes use of GPU, fast lookup tables and deep learning to allow accurate and high image-quality imaging with profile correction in real-time (Angelo et al., 2016; Panigrahi and Gioux, 2018; Schmidt et al., 2019; Aguenounon et al., 2020). Resources regarding the instrumental and processing aspects of SFDI and SSOP, including material references and processing code, are freely available in OpenSFDI (Applegate et al., 2020).
2.2 Fluorescence imaging
The most common form of fluorescence imaging for image-guided surgery relies on the injection of an exogenous contrast agent to highlight a particular structure (e.g., lymph node, vessels, tumor) (Frangioni, 2003; Ntziachristos, 2006; Gioux et al., 2010; Gibbs, 2012; Vahrmeijer et al., 2013; Mieog et al., 2022). A dedicated imaging system that matches the optical properties of the contrast agent (namely absorption and emission spectra) is then used to highlight the presence of the contrast agent in real-time during the surgery. Current implementations used in the clinic (many being commercial products) are qualitative in nature, meaning the fluorescence signal measured by the imaging system is not directly related to the local amount of contrast agent (i.e., its concentration). The fluorescence signal is dependent on multiple external and internal factors such as the illumination homogeneity, the distance between the imaging system and the surgical field, the local tissue optical properties (absorption and scattering properties), the variation of the fluorescence properties due to the dye environment, or the quantum yield of the molecule. Recent effort has tried to address these challenges (Pogue et al., 2018a; Pogue et al., 2018b; Mieog et al., 2022). Some current commercial systems allow to correct for the distance between the imaging system and the surgical field, and some even correct for the illumination inhomogeneities. Research prototypes have been designed to further correct not only distance and field homogeneity, but also optical properties, even in real-time. Finally, the temporal behavior of the fluorescence signal is being investigated as a source of contrast, notably for the imaging of blood perfusion (Matsui et al., 2009; Diana et al., 2015; Meijer et al., 2021). This method referred to as fluorescence dynamics is less dependent on several external and internal factors.
In the implementation described in this work, we chose to enable three modes of fluorescence imaging in the same prototype. The first mode consists of illuminating the field with a temporally constant (called continuous wave, CW) near infrared illumination. CW fluorescence imaging is the most common implementation both commercially and in research (Frangioni, 2003; Gioux et al., 2010). The second mode consists of capturing both the fluorescence image and the excitation illumination. One then divides the fluorescence image by the excitation image to automatically correct for distance, illumination inhomogeneity and partially for optical properties (Ntziachristos et al., 2005; Themelis et al., 2009). The third mode consists of using SFDI or SSOP to extract the optical properties of the surgical field at both the excitation and emission wavelength and use this information to correct for distance, sample profile, illumination inhomogeneity and optical properties. The method used to obtain the so-called quantitative fluorescence images have been previously described in the literature (Sibai et al., 2019; Valdes et al., 2019). We use our own method called qF-SSOP (Valdes et al., 2017). Note that for fluorescence imaging, the excitation wavelength and filter used to detect the signal are dependent on the contrast agent imaged. In our case, we intend to image indocyanine green (ICG), and from prior work, we use an excitation at 760 nm and collection through filters starting at 780 nm. More details about our filtration strategy are described in section 2.4.2.
2.3 Oxygenation imaging
Oxygenation imaging has gained increasing interest for surgical applications (DSouza et al., 2016; Kohler et al., 2019; Shapey et al., 2019; Clancy et al., 2020; Felli et al., 2020; Felli et al., 2021). Oxygen saturation is computed as the ratio of the concentration of oxy-hemoglobin to the concentration of total hemoglobin (oxy-hemoglobin + deoxy-hemoglobin). Note that oxygen saturation here refers to a mix of arterial and venous blood, as opposed to pulse oximetry that only measures the amount of oxygen saturation in arterial blood. Most methods used for oxygenation imaging rely on reflectance imaging at several wavelengths, also called multispectral imaging, or hyperspectral imaging in cases where a large number of wavelengths are used (e.g., > 10 wavelengths). These methods rely on CW illumination and typically fit the measured reflectance spectrum to a theoretical spectrum and extract an oxygenation value. In the case of oxygenation imaging, because it is a ratio (oxy-hemoglobin to total hemoglobin), the influence of scattering and distance between the sample and the imaging system can be managed. Several authors demonstrate the use of these CW multispectral and hyperspectral methods in surgery.
Another approach for oxygenation imaging consists of properly separating scattering and absorption on the measured signal by using a quantitative optical imaging method, such as SFDI (Gioux et al., 2011; Ponticorvo et al., 2013). In this case, the absorption coefficient at a minimum of two wavelengths is then used in conjunction with Beer’s law to directly quantify the concentration of oxy-hemoglobin and deoxy-hemoglobin. These values are then used to compute the oxygen saturation of the sample. In our case, prior work in SFDI determined that optimal wavelengths for oxygenation imaging were close to 665 nm and 860 nm (Mazhar et al., 2010). Our group and others have translated similar technology to preclinical and clinical experiments (Gioux et al., 2011; Nadeau et al., 2013; Ponticorvo et al., 2013; Ghijsen et al., 2018; Schmidt et al., 2019; Weinkauf et al., 2019; Chen and Durr, 2020; Ren et al., 2020; Zhao et al., 2021; Lyu et al., 2022). However, none of the prior work includes a real-time methodology and combines the measurement of oxygenation with the measurement of fluorescence. The Trident imaging platform enables such feature to understand potential clinical use of either or both technologies.
3 Materials and methods
3.1 System design
This section describes the design of the imaging platform for each subsystem. An overview of the complete system is given in Figure 1A. This medical cart-based platform features a three channel-imaging head, fiber-coupled white light sources and laser sources, and a workstation.
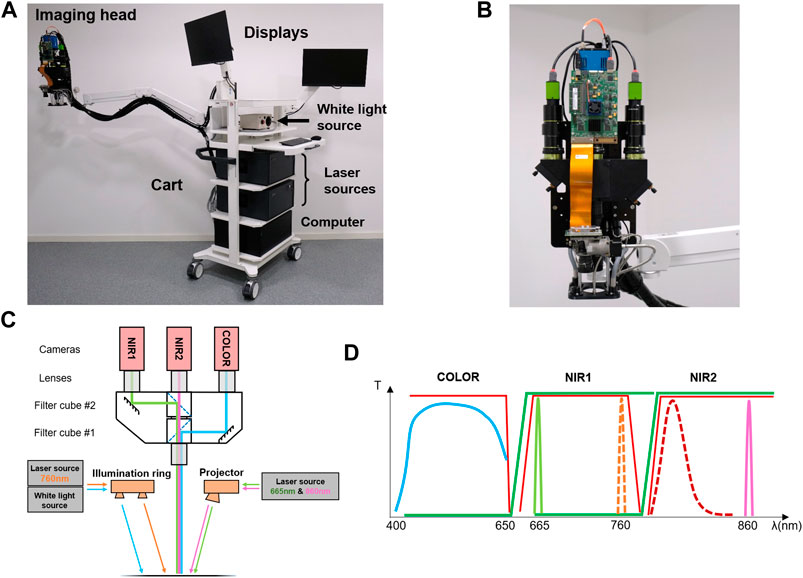
FIGURE 1. Trident imaging platform: (A) Picture of the entire imaging platform, including the medical-grade tart, the articulated arm, the imaging head, the light sources (white light and lasers), the computer and the displays; (B) picture of the imaging head at the end of the articulated arm including the cameras, the Dptomechanical coupling system, the projector and the optical lenses; (C) detailed schematics of the imaging head including the cameras, the optomechanical coupling system, the optical lenses, the dichroic mirrors, the light sources, the illumination ring, the projector and the light paths; (D) details of the filters design of the imaging system: note the separation between the color, NIR1 and NIR2 cameras in combination with the light sources allowing the imaging of the surgical field in color, oxygenation or fluorescence.
3.1.1 Cart and arm
The platform is built upon a custom medical-grade cart (Symbio cart, ITD, Neubiberg, Germany). The wheeled base is equipped with an isolation transformer to regulate power supply to the device and counterweights to safely balance the assembly (compliant with IEC60601). Two articulated arms bear display screens both for the surgeons and the operator. A third articulated arm (Elite 5220, ICW, Medford, OR, United States) is fixed to the central column to accommodate the imaging head. Its large reach enables a user to operate the system while keeping distance from the sterile field. Cables can be guided from the devices through the central column and cable retainer on the articulated arm. Power is supplied to the imaging head using a 24 V AC/DC converter (AKM90PS24, XP Power, Singapore). All three articulated arms conveniently fold for storage. The wide castors and large handle provide good mobility when deploying or rolling out the platform.
3.1.2 Imaging head and filters design
The imaging head is depicted in Figures 1B,C. The optomechanical design features three independent imaging channels: one visible light channel (COLOR) and two NIR channels (NIR1 and NIR2). These channels are co-registered using tilting mirrors, producing a field of view of 12 cm × 15 cm. The detection optics enable fine focusing and individual aperture control. An RGB camera (GO-5000C-USB, JAI Ltd., Kanagawa, Japan) and two monochrome cameras (GO-5000M-USB, JAI Ltd., Kanagawa, Japan; Edge 4.2, Excelitas PCO GmbH, Kelheim, Germany) are used for the detection in the COLOR, NIR1 and NIR2 channels, respectively.
Custom filter sets (Chroma Inc., Bellows Falls, VT, United States) provide wavebands selection for optical properties and fluorescence imaging with minimized crosstalk between channels. Two filter cubes (Axio cubes, Zeiss, Oberkochen, Germany) are composed of two emission filters and a dichroic mirror. These filters can be swapped easily with minimal re-alignment depending on the desired imaging configuration. Figure 1D depicts the filter design in combination with the sources for the presented configuration of oxygenation imaging and 800 nm fluorescence imaging. A first filter cube allows for the isolation of the COLOR channel (400–650 nm). A second filter cube separates the NIR1 and NIR2 channels. The NIR1 channel in the presented configuration allows for the detection of either the 665 nm for oxygenation imaging or 760 nm for fluorescence imaging. The NIR2 channel in the presented configuration allows for the detection of either the emission fluorescence signal from ICG above 780 nm or 860 nm for oxygenation imaging. Note that the dichroic filters optical density is around 2 and the channels emission filters optical densities are around 6–7. This configuration allows appropriate separation of the visible anatomical information in the COLOR channel, concurrently with either oxygenation imaging or fluorescence in NIR1 and NIR2 channels.
3.1.3 White light source
Light emitting diodes (LEDs) based lamps (LO-35, Fiberoptics Technologies Inc., Pomfret, CT, United States) with custom 650 nm short-pass filters and fiber bundles provide a bright illumination of the surgical field. Though the LEDs emit low amounts of infrared light, the additional filter ensures an optimal visible illumination while preserving the NIR channels from background noise. The two fiber bundles are split in two outputs each to produce a multi-angle illumination from the imaging head over a large area over 50 cm diameter at a 45 cm working distance. An illumination ring with custom lensing allows to illuminate the field in the most homogenous manner.
3.1.4 Laser source
Custom laser sources were designed to equip the platform. A first source is dedicated to the projector illumination to collect reflectance data for optical properties imaging and a second source is dedicated to fluorescence imaging. The two sources are referred to as reflectance source and fluorescence source, respectively to their purpose. Figures 2A,B presents one complete source closed and open (top-view) respectively.
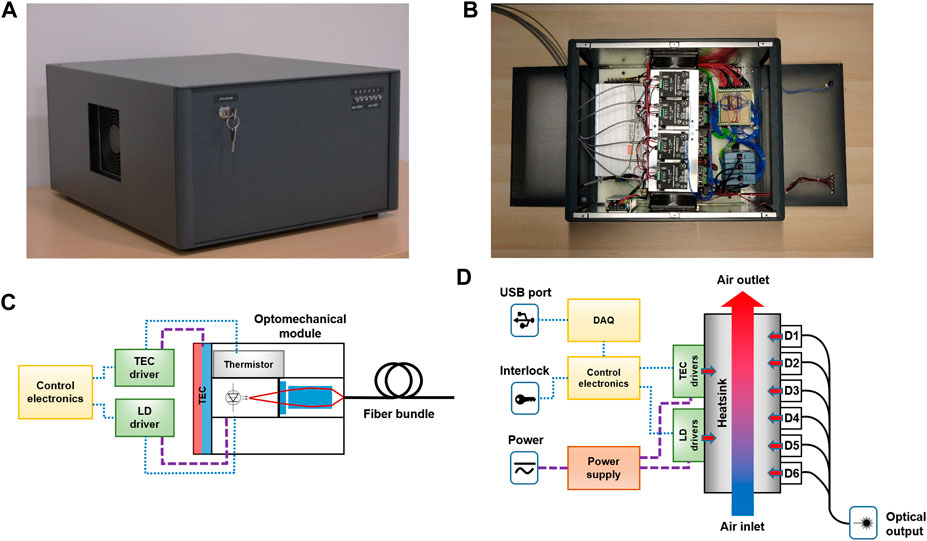
FIGURE 2. Laser light source: (A) Picture of the light source closed; (B) picture of the light source opened (top view); (C) detailed schematics of a single laser diode unit including the laser diode (LD) and its driver, the thermoelectric cooler (TEC) and its driver, the thermistor, the optomechanical module and the output fiber; (D) details of the cooling design of the source: note that the single laser diode units (D1 to D6) are assembled onto a unique heatsink with forced airflow (in and out) for more efficient cooling while maintaining a compact design.
A single source houses six independent modules that can be loaded with different laser diodes (LDX Optronics, Maryville, TN, United States) to achieve multispectral imaging, or to match the excitation of fluorophores of interest. Identical laser diodes can also be combined to provide higher illumination power. For real-time oxygenation imaging, a combination of four 665 nm (2 W each) and two 860 nm (5 W each) laser diodes equip the reflectance source. In order to excite 800 nm fluorophores–especially indocyanine green (ICG)—six 760 nm (6 W each) laser diodes are mounted in the fluorescence source.
Each diode is thermally regulated by a thermoelectric cooler (TEC) (TEC-15.4-3.9-33.4-72-40-CH4,7, Arctic TEC Technologies, Dortmund, Germany) and a thermistor placed on the laser diode chassis (TH10K, Thorlabs, Newton, NJ, United States). Individual TEC drivers (PTC5K-CH, Wavelength electronics, Bozeman, MT, United States) enable temperature regulation and monitoring of the diodes. Similarly, laser diode drivers (LD10CHA, wavelength electronics, Bozeman, MT, United States) control the laser output power, and monitoring of the integrated photodiode feedback. A custom optomechanical assembly featuring optical filters (Chroma Inc., Bellows Falls, VT, United States) and graded-index lenses (Newport, Irvine, CA, United States) performs the laser output coupling into a dedicated fiber bundle (CeramOptec, Bonn, Germany). In the case of the reflectance source, the modules are coupled into a 6-legged fiber bundle combined in a single output for coupling in the projector. In the fluorescence source, the 6 legs of the bundle contain multiple fibers which are randomized and redistributed into 6 legs that are plugged in an illumination ring. In addition, each module is interfaced with USB analog input/ouput modules (USB-202, Measurement Computing, Norton, MA, United States) and custom electronics to control the modules and record feedback signals from a computer. The interfacing of a single module is depicted in Figure 2C.
As shown in Figure 2D, in order to dissipate the great amounts of heat generated by the laser diode cooling and different drivers, all the heating modules components are mounted on a tunnel heatsink equipped will push-pull fans. This design produces a compact assembly and while limiting noise due to the limited number of fans and their large diameter.
3.1.5 Illumination
Two independent illuminations can be used with the Trident imaging platform. The first illumination is a single illumination ring containing four lensed outputs for the white light (from the LED white light source), and six SMA outputs for the CW fluorescence illumination (from the fluorescence laser diode source). The pattern and lensing have been optimized to the best of our effort to provide the most homogeneous illumination possible.
A projector based on a digital micromirror device equipped with a NIR compatible projection optics (V7000 HiSpeed and STAR-CORE, Vialux GmbH, Chemnitz, Germany) and a custom fiber coupling adapter enables the projection of patterns at a 45 cm working distance for optical properties imaging using SFDI. Linear polarizers (RCV8N2EC, Moxtek, Orem, UT, United States) are cross-polarized in front of the projection and detection paths to reject specular reflections. One 5 V DC/DC converter (PYB30-Q24-S5-U, CUI Inc., Tualatin, OR, United States) provides power supply to the digital micromirror device (DMD) board.
3.1.6 Workstation
A high-performance workstation has been assembled to achieve the imaging device control, data processing, data storage management and visualization. A CameraLink HS frame grabber (ME5 Marathon AF2, Silicon Software, Mannheim, Germany) and an additional USB3.0 controller ensure the communication with the imaging head. Powerful CPU (Intel Core i5-10600K, Intel, Santa Clara, CA, United States) and GPU (Quadro RTX 4000, NVIDIA, Santa Clara, CA, United States) and 64 GB of high-speed RAM can perform intensive data processing and visualization on multiple displays. Finally, 5 TB of fast SSD storage are installed for data stream management up to 1 GB/s.
3.2 System testing
3.2.1 Optical performances
A USAF1951 resolution chart (RES-2, Newport, Irvine, CA, United States) was placed under the imaging head at 45 cm working distance and imaged with each channel: COLOR, NIR1 and NIR2. Optical properties extraction was characterized by imaging an array of 12 tissue-mimicking phantoms of 6 × 6 × 2.5 cm. These cured silicone-base Polydimethylsiloxane (PDMS) samples were fabricated using varying amounts of alcohol-soluble nigrosine and Titanium dioxide (TiO2) to tune the absorption and scattering of the material, respectively, spanning a large range of optical properties to be measured: µa ranging from 0.013 to 0.08 mm−1 and µs’ ranging from 0.75 to 1.8 mm−1 at 665 nm. These samples were imaged at 665 nm and 860 nm under a 0.2 mm−1 spatial frequency illumination and processed using the deep learning-based implementation of SSOP. The measurements were compared to those obtained with a reference benchtop imaging system using 7 phase-SFDI. That reference SFDI system was validated in a multi-laboratory performance assessment of diffuse optics instruments (BitMap exercise) (Lanka et al., 2022) and used to obtain the reference optical property values for this work.
3.2.2 Illumination
Peak fluence rates were measured at 45 cm working distance in the center of the field of view using a digital handheld power meter (PM100D, Thorlabs, Newton, NJ, United States) at 665 nm and 860 nm while projecting a continuous wave pattern, and at 760 nm by fiber illumination.
3.2.3 Sensitivity and noise
The sensitivity of the NIR2 channel for fluorescence detection was assessed by imaging eight different dilutions of ICG diluted in dimethyl sulfoxide (DMSO) at concentrations ranging from 25 nM up to 800 nM. The vials were placed at 45 cm from the imaging head and imaged with varying exposure settings successively onto two different background materials: a large tissue-mimicking phantom with µa = 0.012 mm−1 and µs’ = 1.08 mm−1 at 665 nm and a sheet of white paper. Average fluorescence signals were extracted and compared to the noise floor level for sensitivity assessment. Sensitivity was defined as the concentration producing an intensity twice as high as the noise floor level.
In order to quantify the background noise in the fluorescence images, the contributions from dark noise and both white light and excitation light leakage were measured. Images of the previously mentioned background materials from the NIR2 channel were acquired with varying exposure settings–exposure time, sensor binning–with successively all lights switched off, and white light and excitation laser light switched on.
3.3 Preclinical experiments
3.3.1 Bowel ischemia model
One large swine was involved in this non-survival study, which received full approval from the local Ethical Committee on Animal Experimentation (ICOMETH No. 038.2019.01.121) and by the French Ministry of Superior Education and Research (MESR) under the following reference: APAFIS #20819-2019052411591088 v3. After performing a midline laparotomy, a 20 cm small bowel loop was exposed. A central region was delineated, and corresponding arcade branches were isolated. A clamp was used to create an occlusion on these vessels, causing ischemia on a limited portion of the bowel while the rest of the loop remained perfused. The occlusion was maintained during 14 min until release.
3.3.2 Fluorescence imaging
A dose of 4 mg of ICG powder diluted in 1.6 ml of 5% glucose solution (Infracyanine, SERB, Paris, France) was injected intravenously 2 min before occlusion release. Color images from the COLOR channel and fluorescence images from the NIR2 channel were acquired starting from the injection timepoint and for 2 min and 30 s. The imaging working distance was maintained at 45 cm. Fluorescence excitation was produced using 760 nm laser light.
3.3.3 Oxygenation imaging
A sinusoidal pattern with spatial frequency of 0.2 mm−1 was projected at both 665 nm and 860 nm wavelengths using the DMD projector. Reflectance images from the NIR1 and NIR2 channels were acquired, as well as color images from the COLOR channel for anatomical reference. Images were recorded starting 2 min before occlusion to establish the oxygen saturation baseline, and during 12 min after occlusion. After performing fluorescence imaging, oxygenation imaging resumed to record for 3 min during the end of the reperfusion phase.
4 Results
4.1 System testing
4.1.1 Optical performances
At 45 cm working distance and performing 2 × 2 binning on the camera sensor (for increased sensitivity), the optical system produces images of a 12 × 15 cm field-of-view, with a resolution of 1024 × 1280 pixels. The measured lateral resolution with fully open apertures for channels COLOR and NIR2 was 5.04 lp/mm and 5.66 lp/mm for the NIR1 channel.
A set of 12 tissue-mimicking phantoms was imaged first with a benchtop imaging system using 7 phase-SFDI as reference. The images produced by the clinical platform were processed using deep learning-based SSOP. High image quality and good accuracy with respect to optical properties extraction were achieved. Figure 3 presents the extracted optical properties maps and error estimation in comparison to standard SFDI. Errors in µa and µs’ measurements were respectively below 2.7% and 2.1% for both 665 nm and 860 nm oxygenation imaging wavelengths.
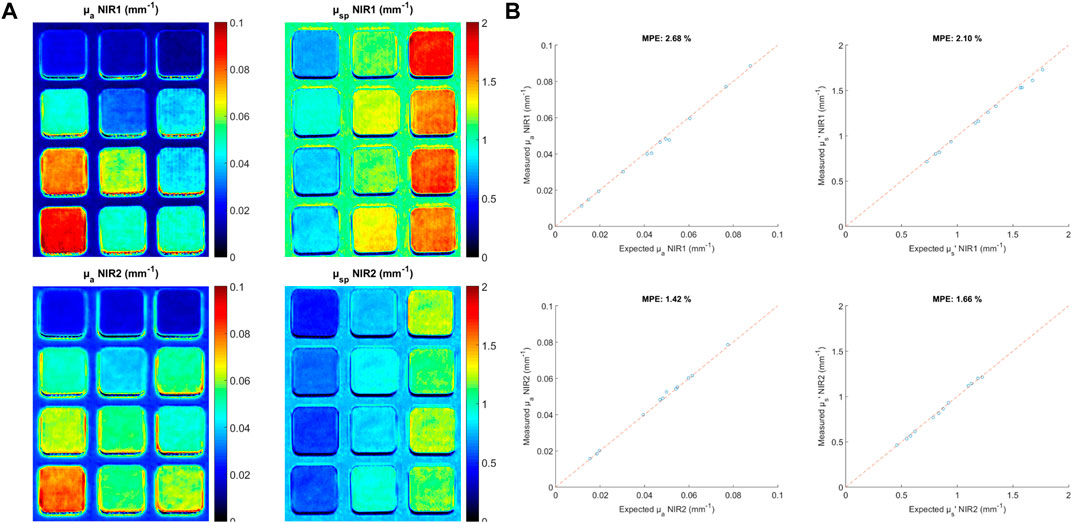
FIGURE 3. Bench results: endogenous imaging: (A) Tissue mimicking phantoms having different absorption and reduced scattering properties imaged with the Trident imaging platform: optical properties are imaged on all phantoms using the NIR1 (665 nm) and NIR2 (860 nm) cameras; (B) plots comparing the results obtained using a referencing SFDI acquisition method on a bench system compared with the results obtained with the SSOP acquisition method on the Trident platform: note the good agreement in absorption and reduced scattering (errors lower than 2.7% and 2.1%, respectively).
4.1.2 Illumination
A continuous-wave illumination was produced using the DMD projector at both 665 nm and 860 nm oxygenation imaging wavelengths. The projector has a small Gaussian-like variation of intensity across the projected field (55% maximum). This inhomogeneity does not affect the performance of the system as the signal-to-noise ratio allows proper processing of the data at all locations of the image. At 45 cm working distance, a power meter probe was placed in the center of the field-of-view. Fluence rates of 0.93 mW/cm2 at 665 nm and 1.51 mW/cm2 at 860 nm were measured and classified as laser Class 1 as per IEC60825. The fluorescence excitation field at 760 nm showed a peak fluence rate of 31.51 mW/cm2 and classified as laser Class 3 R as per IEC60825.
4.1.3 Sensitivity and noise
A set of 8 ICG dilutions with concentrations ranging from 25 nM to 800 nM was imaged in the same previously stated conditions. Figure 4A shows the images from the COLOR and NIR2 channels as well as an overlay. Figure 4B shows the evolution of intensity in the NIR2 images for each dilution at various exposure times with fixed 2 × 2 sensor binning as well as using a silicone phantom as background. The intensity increases linearly with the exposure time until reaching saturation. From the entire dilution series, the sensitivity was estimated by measuring the concentration corresponding to an intensity 2 times higher than the noise floor level, yielding a signal-to-background ratio of 2. At 40 ms exposure time and 2 × 2 binning factor, the sensitivity was estimated at 18.8 nM.
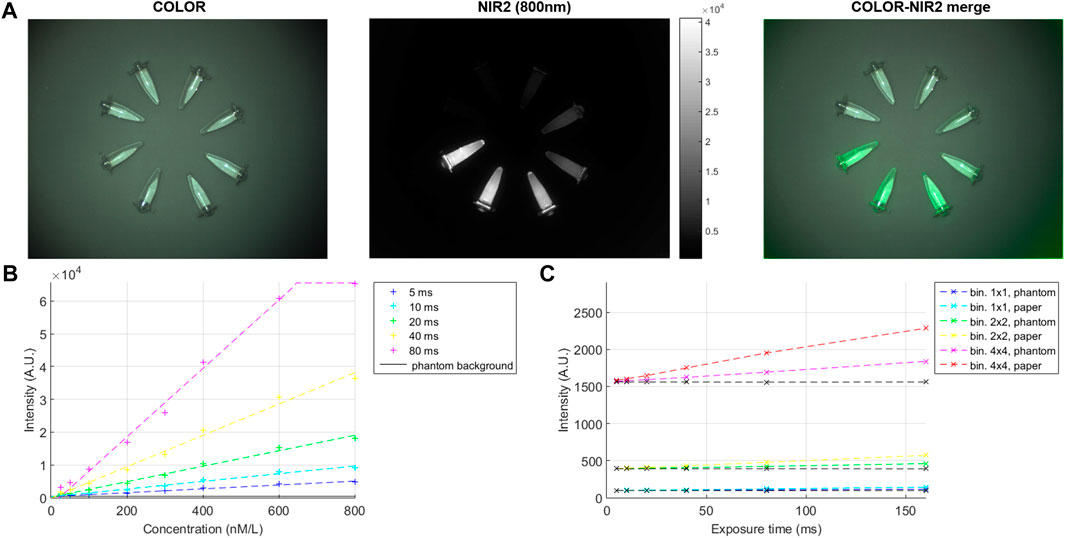
FIGURE 4. Bench results: fluorescence imaging: (A) Results obtained with the Trident imaging platform from a dilution series of indocyanine green (ICG) diluted in dimethyl sulfoxide (DMSO) at concentrations ranging from 25 nM to 800 nM in color (left), NIR2 fluorescence (middle) and a merged image of the color with the fluorescence (right); (B) plot showing the dilution series and a phantom background imaged at various exposure times from 5 to 80 ms; (C) plot showing the dark noise and leakage with different binning, exposure settings and background materials.
Background noise in the NIR2 channel was measured using successively a tissue-mimicking phantom and a sheet of white paper placed at 45 cm working distance. Figure 4C shows the evolution of dark noise and leakage with exposure settings and background materials. Dark noise was first measured with exposure times ranging from 5 ms to 160 ms and sensor binning factors of 1 × 1, 2 × 2, and 4 × 4. Noise levels representing 0.1%, 0.6%, and 2.4% of the dynamic range of the NIR2 camera were measured respectively with 1 × 1, 2 × 2, and 4 × 4 binning factors. The same set of images was acquired with fully open aperture, and both white light and 760 nm laser excitation light switched on. Noise levels were on average 17% and 46% higher than the dark noise using a phantom background or a paper background, corresponding mostly to excitation light leakage into the NIR2 channel.
4.2 Preclinical experiments
Figures 5A,B shows the results of the preclinical experiments in COLOR, fluorescence and oxygenation. A comparison of fluorescence and oxygenation imaging in given at three timepoints: during the baseline phase, after 12 min of occlusion and 1 min after reperfusion.
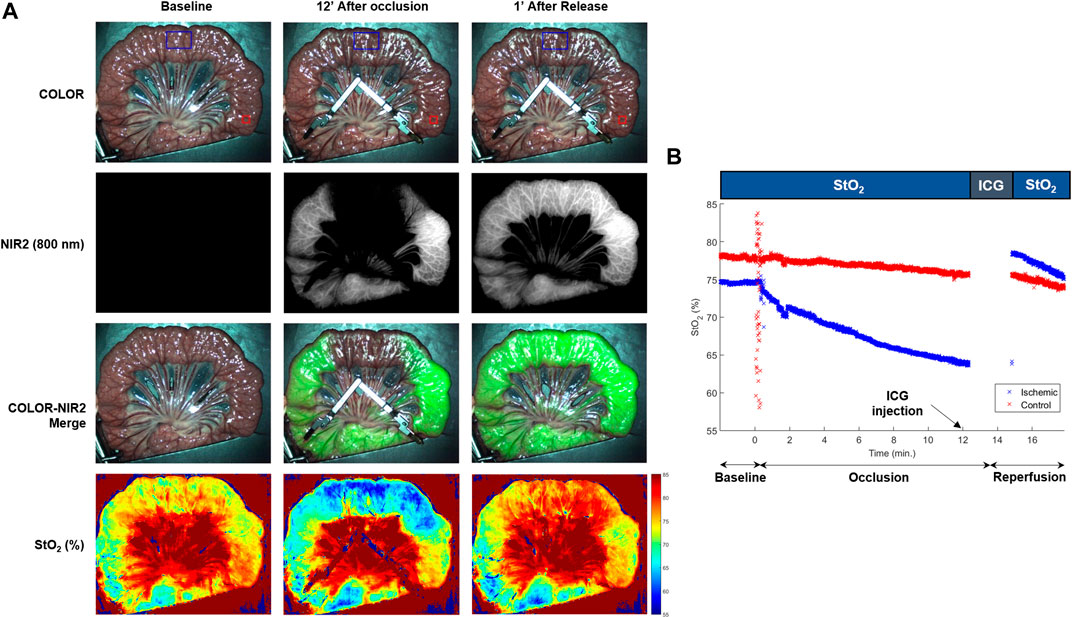
FIGURE 5. In-vivo results: Fluorescence and oxygenation imaging: (A) Images obtained with the Trident imaging platform during the baseline (left column), 12 min after occlusion (middle column) and 1 min after release (right column). Note the influence of the occlusion and release compared with baseline for the color images (first row), fluorescence images (second row), color and fluorescence merge (third row) and oxygenation (fourth row). (B) Time plot of the oxygenation results over the course of the experiment, including one region that is occluded (ischemic, blue ROI and curve) and one region that is not occluded (control, red ROI and curve). Regions of interest (ROls) are indicated on the color images in Figure 5A.
4.2.1 Fluorescence imaging
At 12 min into the occlusion phase, a dose of ICG was intravenously injected. Images from the NIR2 and COLOR channels were recorded at 12 frames per second with 50 ms exposure time and 2 × 2 sensor binning while illuminating the surgical field with 760 nm laser light. Fluorescence intensity was monitored in a 120 × 180 pixels region of interest (ROI) located in the ischemic area and in a 50 × 50 pixels ROI located in a perfused area. The fluorescence signal reached its peak in the perfused area 35 s after injection before decreasing and stabilizing at 58% of the peak intensity 1 min later.
After 14 min of occlusion, the clamp was released. The fluorescence intensity immediately increases in the ischemic area, as the perfusion resumes. When stabilizing, the intensity reaches 72% of the intensity in the control area.
4.2.2 Oxygenation imaging
Oxygenation imaging was achieved at 12 frames per second using structured illumination at 0.2 mm−1 spatial frequency at 665 nm and 860 nm. Figure 5B shows the oxygen saturation trends for the two ROIs over time. During the baseline phase, both ROIs show stable StO2 rates of 77.9% and 74.6% respectively in the control and ischemic areas. While the perfused area remains 4% of its baseline value of StO2, oxygenation in the ischemic area decreases until reaching 63.8% after 12 min of occlusion. From 2 min to 30 s after the occlusion release, the StO2 rate decreases back its baseline state, reaching 75% after 5 min and 30 s of reperfusion.
4.2.3 Video
A video is included in the supplementary material. It presents the merged of the oxygenation and fluorescence results over the course of the experiment. In vivo oxygenation and fluorescence imaging. An occlusion of a portion of a bowel loop was performed for 14 min. Oxygenation was monitored prior to clamping and during the 12 first minutes of occlusion. Fluorescence images were recorded from the ICG intravenous injection until 30 s after release. Oxygenation imaging resumed for the 3 min of the reperfusion phase. The absence of fluorescence signal in the ischemic area correlates with the low StO2 rates observed. After reperfusion, this area shows fluorescence signal levels and StO2 rates close to those of the perfused areas. Oxygenation rates are steady in the control area whereas ischemia could be detected and quantified in the occluded area.
5 Discussion
We presented the design and testing of a clinically-compatible imaging platform for real-time quantitative optical imaging during surgery. The platform is designed to image either fluorescence in three modes (CW, semi-quantitative and quantitative) or oxygenation. The platform imaging modality can be changed on the fly during the surgery allowing to compare both fluorescence and oxygenation modalities.
The bench characterization of the platform demonstrates its performances for optical properties imaging and fluorescence imaging. First, the comparison between tissue-mimicking phantom optical properties obtained from standard profile corrected SFDI and state-of-the-art deep learning-based profile corrected SSOP shows good agreement for both absorption and reduced scattering, at 665 nm and 860 nm, as expected from previous published validation of SSOP. Average error below 2.7% in absorption and 2.1% in reduced scattering were observed. The considered optical properties were matching the typical range of those of biological tissues, ensuring similar performances for in vivo imaging. Second, the characterization studies demonstrate sensitivity for ICG detection at concentrations as low as 18.8 nM with 40 ms exposure time and 2 × 2 binning factor, corresponding to ideal exposure parameters to produce real-time fluorescence imaging (25 fps) with high-resolution (1 mega-pixel images). Relatively low noise levels were measured, validating the performances of the implemented optical filtering, and ensuring high signal to background ratios.
Next, results from an in vivo preclinical trial on a small bowel model demonstrate the ability of the platform to produce oxygenation rate mapping in real-time, yielding the monitoring of tissue ischemia. More precisely, the oxygenation dynamics could be observed during the ischemic phase, and during the reperfusion phase. These results are in agreement with the outcome of ICG fluorescence, confirming the suitability of StO2 as a metric for blood perfusion monitoring. However, concurrent oxygenation and fluorescence imaging is currently not possible. However, a mode where both oxygenation and fluorescence imaging are required concurrently has not been investigated, and display of both data or their combination might be challenging.
Several other preclinical studies are being conducted to understand better the interest of oxygenation imaging for different surgical procedures (Felli et al., 2020; Cinelli et al., 2021; Felli et al., 2021; Jansen-Winkeln et al., 2021). Particularly, this platform provided value for perfusion assessment during gastric conduit creation for esophagectomy, for liver viability assessment, and for bowel ischemia monitoring during colorectal procedures.
Overall, the design of the imaging platform ensured minimal interference with the surgical workflow by maintaining a long 45 cm working distance, providing bright white light illumination of the surgical field, and by featuring ergonomic handling of the device. The platform is currently undergoing clinical translation for first-in-human use during colorectal anastomosis procedures using oxygenation imaging. This study lays the foundation for translating state-of-the-art optical imaging technology to the clinic and better understand its potential clinical value.
6 Conclusion
In conclusion, we presented a novel imaging platform called Trident. We detailed the platform design, characterization and validation for oxygenation and 800 nm fluorescence imaging in real-time in a clinical environment. The validation of the imaging device was performed on the bench before demonstrating its performances in vivo during a preclinical study for blood perfusion monitoring in the small bowel. Several other preclinical studies are ongoing in various surgical procedures (liver, stomach, bowel, colon, kidney). The platform is currently undergoing clinical translation for first-in-human use during colorectal anastomosis procedures using oxygenation imaging. This study lays the foundation for translating state-of-the-art optical imaging technology to the clinic and better understand its potential clinical value.
Data availability statement
The raw data supporting the conclusion of this article will be made available by the authors, without undue reservation.
Ethics statement
The animal study was reviewed and approved by local Ethical Committee on Animal Experimentation (ICOMETH No. 038.2019.01.121) and by the French Ministry of Superior Education and Research (MESR) under the following reference: APAFIS #20819-2019052411591088 v3.
Author contributions
SS, LB, and SG designed and fabricated the Trident imaging system along with its acquisition and processing software. SG and MD designed the study. SS, LB, EF, EB, LC, MR-L, NO, DK, SG, and MD, conducted the experiments and analysed the data. SS and SG drafted the manuscript. MD, MM, SL, and SG revised the manuscript for important intellectual content. All authors approved the final version of the manuscript.
Funding
This research was funded by the European Research Council (ERC) under the European Union’s Horizon 2020 Research and Innovation Program under Grant Agreement No. 715737 (QuantSURG; SG), Agence Nationale de la Recherche (LiverSURG; ANR-18-CE19-0026), France Life Imaging WP3 (SG), the French program “Investissement d’Avenir” run by the “Agence Nationale de la Recherche” under Grant Agreement No. ANR-11-INBS-006 (SG), the University of Strasbourg IdEx (SG) and the European Union’s Horizon 2020 Research and Innovation Program under the Marie Skłodowska-Curie Grant Agreement No 857894—CAST (SG and MD).
Conflict of interest
Author SG is employed by the company Intuitive Surgical.
The remaining authors declare that the research was conducted in the absence of any commercial or financial relationships that could be construed as a potential conflict of interest.
Publisher’s note
All claims expressed in this article are solely those of the authors and do not necessarily represent those of their affiliated organizations, or those of the publisher, the editors and the reviewers. Any product that may be evaluated in this article, or claim that may be made by its manufacturer, is not guaranteed or endorsed by the publisher.
Supplementary material
The Supplementary Material for this article can be found online at: https://www.frontiersin.org/articles/10.3389/fphot.2022.1032776/full#supplementary-material
References
Aguenounon, E., Smith, J. T., Al-Taher, M., Diana, M., Intes, X., and Gioux, S. (2020). Real-time, wide-field and high-quality single snapshot imaging of optical properties with profile correction using deep learning. Biomed. Opt. Express 11 (10), 5701–5716. doi:10.1364/boe.397681
Angelo, J., Vargas, C. R., Lee, B. T., Bigio, I. J., and Gioux, S. (2016). Ultrafast optical property map generation using lookup tables. J. Biomed. Opt. 21 (11), 110501. doi:10.1117/1.jbo.21.11.110501
Angelo, J. P., van de Giessen, M., and Gioux, S. (2017). Real-time endoscopic optical properties imaging. Biomed. Opt. Express 8 (11), 5113–5126. doi:10.1364/boe.8.005113
Applegate, M., Karrobi, K., Angelo, J., Austin, W., Tabassum, S., Aguenounon, E., et al. (2020). OpenSFDI: an open-source guide for constructing a spatial frequency domain imaging system. J. Biomed. Opt. 25 (1), 1–13. doi:10.1117/1.jbo.25.1.016002
Bigio, I. J., and Fantini, S. (2016). Quantitative biomedical optics: theory, methods, and applications. Cambridge: Cambridge University Press.
Chen, M. T., and Durr, N. J. (2020). Rapid tissue oxygenation mapping from snapshot structured-light images with adversarial deep learning. J. Biomed. Opt. 25 (11). doi:10.1117/1.jbo.25.11.112907
Cinelli, L., Felli, E., Baratelli, L., Segaud, S., Baiocchini, A., Okamoto, N., et al. (2021). Single snapshot imaging of optical properties (SSOP) for perfusion assessment during gastric conduit creation for esophagectomy: an experimental study on pigs. Cancers (Basel). 13 (23), 6079. doi:10.3390/cancers13236079
Clancy, N. T., Jones, G., Maier-Hein, L., Elson, D. S., and Stoyanov, D. (2020). Surgical spectral imaging. Med. Image Anal. 63, 101699. doi:10.1016/j.media.2020.101699
Cuccia, D. J., Bevilacqua, F., Durkin, A. J., Ayers, F. R., and Tromberg, B. J. (2009). Quantitation and mapping of tissue optical properties using modulated imaging. J. Biomed. Opt. 14 (2), 024012. doi:10.1117/1.3088140
Diana, M., Agnus, V., Halvax, P., Liu, Y. Y., Dallemagne, B., Schlagowski, A. I., et al. (2015). Intraoperative fluorescence-based enhanced reality laparoscopic real-time imaging to assess bowel perfusion at the anastomotic site in an experimental model. Br. J. Surg. 102 (2), e169–e176. doi:10.1002/bjs.9725
Dognitz, N., and Wagnieres, G. (1998). Determination of tissue optical properties by steady-state spatial frequency-domain reflectometry. Lasers Med. Sci. 13 (1), 55–65. doi:10.1007/bf00592960
DSouza, A. V., Lin, H., Henderson, E. R., Samkoe, K. S., and Pogue, B. W. (2016). Review of fluorescence guided surgery systems: identification of key performance capabilities beyond indocyanine green imaging. J. Biomed. Opt. 21 (8), 080901. doi:10.1117/1.jbo.21.8.080901
Felli, E., Al-Taher, M., Collins, T., Baiocchini, A., Felli, E., Barberio, M., et al. (2020). Hyperspectral evaluation of hepatic oxygenation in a model of total vs. arterial liver ischaemia. Sci. Rep. 10 (1), 15441. doi:10.1038/s41598-020-72915-6
Felli, E., Al-Taher, M., Collins, T., Nkusi, R., Felli, E., Baiocchini, A., et al. (2021). Automatic liver viability scoring with deep learning and hyperspectral imaging. Diagn. (Basel) 11 (9), 1527. doi:10.3390/diagnostics11091527
Frangioni, J. V. (2003). In vivo near-infrared fluorescence imaging. Curr. Opin. Chem. Biol. 7 (5), 626–634. doi:10.1016/j.cbpa.2003.08.007
Ghijsen, M., Lentsch, G. R., Gioux, S., Brenner, M., Durkin, A. J., Choi, B., et al. (2018). Quantitative real-time optical imaging of the tissue metabolic rate of oxygen consumption. J. Biomed. Opt. 23 (3), 1–12. doi:10.1117/1.jbo.23.3.036013
Gibbs, S. L. (2012). Near infrared fluorescence for image-guided surgery. Quant. Imaging Med. Surg. 2 (3), 177–187. doi:10.3978/j.issn.2223-4292.2012.09.04
Gioux, S., Choi, H. S., and Frangioni, J. V. (2010). Image-guided surgery using invisible near-infrared light: fundamentals of clinical translation. Mol. Imaging 9 (5), 7290.2010.00034–55. doi:10.2310/7290.2010.00034
Gioux, S., Mazhar, A., and Cuccia, D. J. (2019). Spatial frequency domain imaging in 2019: principles, applications, and perspectives. J. Biomed. Opt. 24 (7), 1–18. doi:10.1117/1.jbo.24.7.071613
Gioux, S., Mazhar, A., Lee, B. T., Lin, S. J., Tobias, A. M., Cuccia, D. J., et al. (2011). First-in-human pilot study of a spatial frequency domain oxygenation imaging system. J. Biomed. Opt. 16 (8), 1. doi:10.1117/1.3614566
Jansen-Winkeln, B., Germann, I., Kohler, H., Mehdorn, M., Maktabi, M., Sucher, R., et al. (2021). Comparison of hyperspectral imaging and fluorescence angiography for the determination of the transection margin in colorectal resections-a comparative study. Int. J. Colorectal Dis. 36 (2), 283–291. doi:10.1007/s00384-020-03755-z
Kohler, H., Jansen-Winkeln, B., Maktabi, M., Barberio, M., Takoh, J., Holfert, N., et al. (2019). Evaluation of hyperspectral imaging (HSI) for the measurement of ischemic conditioning effects of the gastric conduit during esophagectomy. Surg. Endosc. 33 (11), 3775–3782. doi:10.1007/s00464-019-06675-4
Lanka, P., Yang, L., Orive-Miguel, D., Veesa, J. D., Tagliabue, S., Sudakou, A., et al. (2022). Multi-laboratory performance assessment of diffuse optics instruments: the BitMap exercise. J. Biomed. Opt. 27 (7). doi:10.1117/1.jbo.27.7.074716
Lu, G., and Fei, B. (2014). Medical hyperspectral imaging: a review. J. Biomed. Opt. 19 (1), 010901. doi:10.1117/1.jbo.19.1.010901
Lyu, L., Kim, H., Bae, J. S., Hua, C., Kim, J. H., Kim, E. H., et al. (2022). The application of SFDI and LSI system to evaluate the blood perfusion in skin flap mouse model. Lasers Med. Sci. 37 (2), 1069–1079. doi:10.1007/s10103-021-03354-6
Matsui, A., Lee, B. T., Winer, J. H., Laurence, R. G., and Frangioni, J. V. (2009). Quantitative assessment of perfusion and vascular compromise in perforator flaps using a near-infrared fluorescence-guided imaging system. Plastic Reconstr. Surg. 124 (2), 451–460. doi:10.1097/prs.0b013e3181adcf7d
Mazhar, A., Dell, S., Cuccia, D. J., Gioux, S., Durkin, A. J., Frangioni, J. V., et al. (2010). Wavelength optimization for rapid chromophore mapping using spatial frequency domain imaging. J. Biomed. Opt. 15 (6), 1. doi:10.1117/1.3523373
Meijer, R. P. J., van Manen, L., Hartgrink, H. H., Burggraaf, J., Gioux, S., Vahrmeijer, A. L., et al. (2021). Quantitative dynamic near-infrared fluorescence imaging using indocyanine green for analysis of bowel perfusion after mesenteric resection. J. Biomed. Opt. 26 (6). doi:10.1117/1.jbo.26.6.060501
Mieog, J. S. D., Achterberg, F. B., Zlitni, A., Hutteman, M., Burggraaf, J., Swijnenburg, R. J., et al. (2022). Fundamentals and developments in fluorescence-guided cancer surgery. Nat. Rev. Clin. Oncol. 19 (1), 9–22. doi:10.1038/s41571-021-00548-3
Nadeau, K. P., Ponticorvo, A., Lee, H. J., Lu, D., Durkin, A. J., and Tromberg, B. J. (2013). Quantitative assessment of renal arterial occlusion in a porcine model using spatial frequency domain imaging. Opt. Lett. 38 (18), 3566–3569. doi:10.1364/ol.38.003566
Ntziachristos, V. (2006). Fluorescence molecular imaging. Annu. Rev. Biomed. Eng. 8, 1–33. doi:10.1146/annurev.bioeng.8.061505.095831
Ntziachristos, V., Turner, G., Dunham, J., Windsor, S., Soubret, A., Ripoll, J., et al. (2005). Planar fluorescence imaging using normalized data. J. Biomed. Opt. 10 (6), 064007. doi:10.1117/1.2136148
Panigrahi, S., and Gioux, S. (2018). Machine learning approach for rapid and accurate estimation of optical properties using spatial frequency domain imaging. J. Biomed. Opt. 24 (7), 1. doi:10.1117/1.jbo.24.7.071606
Pogue, B. W., Rosenthal, E. L., Achilefu, S., and van Dam, G. M. (2018). Perspective review of what is needed for molecular-specific fluorescence-guided surgery. J. Biomed. Opt. 23 (10), 1–9. doi:10.1117/1.jbo.23.10.100601
Pogue, B. W., Zhu, T. C., Ntziachristos, V., Paulsen, K. D., Wilson, B. C., Pfefer, J., et al. (2018). Fluorescence-guided surgery and intervention - an AAPM emerging technology blue paper. Med. Phys. 45 (6), 2681–2688. doi:10.1002/mp.12909
Ponticorvo, A., Taydas, E., Mazhar, A., Scholz, T., Kim, H. S., Rimler, J., et al. (2013). Quantitative assessment of partial vascular occlusions in a swine pedicle flap model using spatial frequency domain imaging. Biomed. Opt. Express 4 (2), 298–306. doi:10.1364/boe.4.000298
Ren, J., Ramirez, G. A., Proctor, A. R., Wu, T. T., Benoit, D. S. W., and Choe, R. (2020). Spatial frequency domain imaging for the longitudinal monitoring of vascularization during mouse femoral graft healing. Biomed. Opt. Express 11 (10), 5442–5455. doi:10.1364/boe.401472
Schmidt, M., Aguenounon, E., Nahas, A., Torregrossa, M., Tromberg, B. J., Uhring, W., et al. (2019). Real-time, wide-field, and quantitative oxygenation imaging using spatiotemporal modulation of light. J. Biomed. Opt. 24 (7), 1–7. doi:10.1117/1.jbo.24.7.071610
Shapey, J., Xie, Y., Nabavi, E., Bradford, R., Saeed, S. R., Ourselin, S., et al. (2019). Intraoperative multispectral and hyperspectral label-free imaging: a systematic review of in vivo clinical studies. J. Biophot. 12 (9), e201800455. doi:10.1002/jbio.201800455
Sibai, M., Wirth, D. J., Leblond, F., Roberts, D. W., Paulsen, K. D., and Wilson, B. C. (2019). Quantitative subsurface spatial frequency-domain fluorescence imaging for enhanced glioma resection. J. Biophot. 12 (5), e201800271. doi:10.1002/jbio.201800271
Smith, J. T., Ochoa, M., Faulkner, D., Haskins, G., and Intes, X. (2022). Deep learning in macroscopic diffuse optical imaging. J. Biomed. Opt. 27 (2). doi:10.1117/1.jbo.27.2.020901
Themelis, G., Yoo, J. S., Soh, K., Schulz, R., and Ntziachristos, V. (2009). Real-time intraoperative fluorescence imaging system using light-absorption correction. J. Biomed. Opt. 14 (6), 064012. doi:10.1117/1.3259362
Vahrmeijer, A. L., Hutteman, M., van der Vorst, J. R., van de Velde, C. J., and Frangioni, J. V. (2013). Image-guided cancer surgery using near-infrared fluorescence. Nat. Rev. Clin. Oncol. 10 (9), 507–518. doi:10.1038/nrclinonc.2013.123
Valdes, P. A., Angelo, J. P., Choi, H. S., and Gioux, S. (2017). qF-SSOP: real-time optical property corrected fluorescence imaging. Biomed. Opt. Express 8 (8), 3597–3605. doi:10.1364/boe.8.003597
Valdes, P. A., Juvekar, P., Agar, N. Y. R., Gioux, S., and Golby, A. J. (2019). Quantitative wide-field imaging techniques for fluorescence guided neurosurgery. Front. Surg. 6, 31. doi:10.3389/fsurg.2019.00031
van de Giessen, M., Angelo, J. P., and Gioux, S. (2015). Real-time, profile-corrected single snapshot imaging of optical properties. Biomed. Opt. Express 6 (10), 4051–4062. doi:10.1364/boe.6.004051
Vervandier, J., and Gioux, S. (2013). Single snapshot imaging of optical properties. Biomed. Opt. Express 4 (12), 2938–2944. doi:10.1364/boe.4.002938
Weinkauf, C., Mazhar, A., Vaishnav, K., Hamadani, A. A., Cuccia, D. J., and Armstrong, D. G. (2019). Near-instant noninvasive optical imaging of tissue perfusion for vascular assessment. J. Vasc. Surg. 69 (2), 555–562. doi:10.1016/j.jvs.2018.06.202
Keywords: optical imaging, oxygenation imaging, fluorescence imaging, surgical guidance/navigation, clinical translation
Citation: Ségaud S, Baratelli L, Felli E, Bannone E, Cinelli L, Rodríguez-Luna MR, Okamoto N, Keller DS, de Mathelin M, Lecler S, Diana M and Gioux S (2022) Trident: A dual oxygenation and fluorescence imaging platform for real-time and quantitative surgical guidance. Front. Photonics 3:1032776. doi: 10.3389/fphot.2022.1032776
Received: 31 August 2022; Accepted: 18 October 2022;
Published: 09 November 2022.
Edited by:
Kristen M. Meiburger, Politecnico di Torino, ItalyReviewed by:
Mengyang Liu, Medical University of Vienna, AustriaDimitris Gorpas, Helmholtz Association of German Research Centres (HZ), Germany
Copyright © 2022 Ségaud, Baratelli, Felli, Bannone, Cinelli, Rodríguez-Luna, Okamoto, Keller, de Mathelin, Lecler, Diana and Gioux. This is an open-access article distributed under the terms of the Creative Commons Attribution License (CC BY). The use, distribution or reproduction in other forums is permitted, provided the original author(s) and the copyright owner(s) are credited and that the original publication in this journal is cited, in accordance with accepted academic practice. No use, distribution or reproduction is permitted which does not comply with these terms.
*Correspondence: Sylvain Gioux, c3lsdmFpbi5naW91eEBpbnR1c3VyZy5jb20=