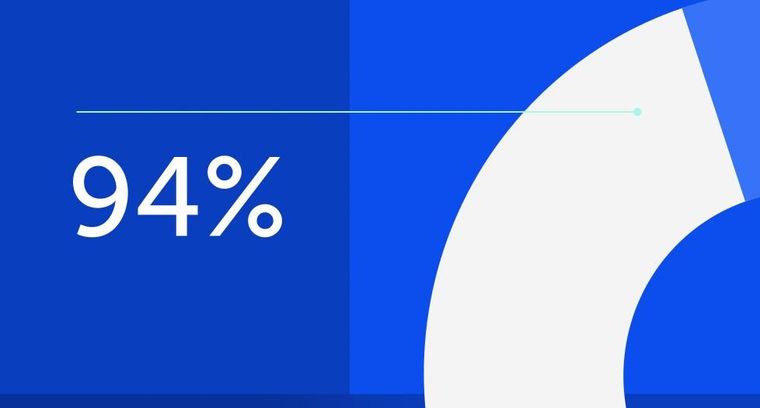
94% of researchers rate our articles as excellent or good
Learn more about the work of our research integrity team to safeguard the quality of each article we publish.
Find out more
ORIGINAL RESEARCH article
Front. Pharmacol.
Sec. Ethnopharmacology
Volume 16 - 2025 | doi: 10.3389/fphar.2025.1594186
This article is part of the Research TopicNatural Medicines for Metabolic Diseases – Computational and Pharmacological Approaches, Volume IIView all articles
The final, formatted version of the article will be published soon.
Select one of your emails
You have multiple emails registered with Frontiers:
Notify me on publication
Please enter your email address:
If you already have an account, please login
You don't have a Frontiers account ? You can register here
Metabolic diseases, such as diabetes, pose significant risks to human health due to their complex pathogenic mechanisms, complicating the use of combination drug therapies. Natural medicines, which contain multiple bioactive components and exhibit fewer side effects, offer promising therapeutic potential. Metabolite imbalances are often closely associated with the pathogenesis of metabolic diseases. Therefore, metabolite detection not only aids in disease diagnosis but also provides insights into how natural medicines regulate metabolism, thereby supporting the development of preventive and therapeutic strategies. Deep learning has shown remarkable efficacy and precision across multiple domains, particularly in drug discovery applications. Building on this, We developed an innovative framework combining graph autoencoders (GAEs) with non-negative matrix factorization (NMF) to investigate metabolic disease pathogenesis via metabolite-disease association analysis. First, we applied NMF to extract discriminative features from established metabolite-disease associations. These features were subsequently integrated with known relationships and processed through a GAE to identify potential disease mechanisms. Comprehensive evaluations demonstrate our method's superior performance, while case studies validate its capability to reveal pathological mechanisms in metabolic disorders including diabetes. This approach may facilitate the development of natural medicine-based interventions.
Keywords: Metabolic Diseases, Natural medicines, Drug Discovery, Graph autoencoder, Metabolite-disease associations
Received: 15 Mar 2025; Accepted: 08 Apr 2025.
Copyright: © 2025 Liao, Zhao, Wang, Xu, Yang, Liu and Zhang. This is an open-access article distributed under the terms of the Creative Commons Attribution License (CC BY). The use, distribution or reproduction in other forums is permitted, provided the original author(s) or licensor are credited and that the original publication in this journal is cited, in accordance with accepted academic practice. No use, distribution or reproduction is permitted which does not comply with these terms.
* Correspondence: Qingquan Liao, Hunan Police Academy, Changsha, 410138, Hunan Province, China
Disclaimer: All claims expressed in this article are solely those of the authors and do not necessarily represent those of their affiliated organizations, or those of the publisher, the editors and the reviewers. Any product that may be evaluated in this article or claim that may be made by its manufacturer is not guaranteed or endorsed by the publisher.
Supplementary Material
Research integrity at Frontiers
Learn more about the work of our research integrity team to safeguard the quality of each article we publish.