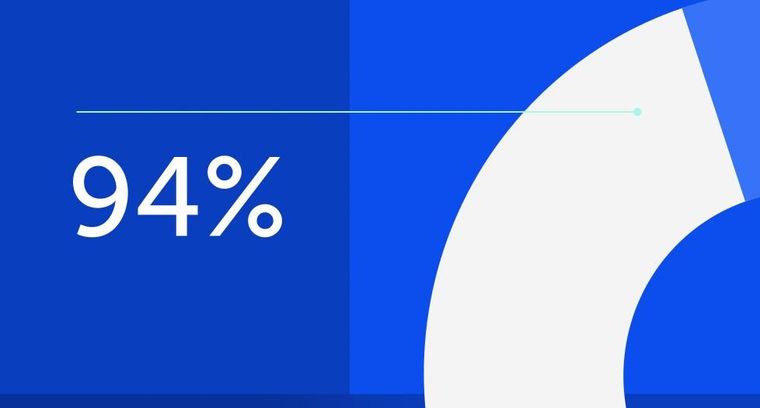
94% of researchers rate our articles as excellent or good
Learn more about the work of our research integrity team to safeguard the quality of each article we publish.
Find out more
ORIGINAL RESEARCH article
Front. Pharmacol.
Sec. Experimental Pharmacology and Drug Discovery
Volume 16 - 2025 | doi: 10.3389/fphar.2025.1585639
This article is part of the Research TopicAdvancing Glioma Treatment: Novel Drugs, Mechanisms of Resistance, and Therapeutic StrategiesView all 10 articles
The final, formatted version of the article will be published soon.
Select one of your emails
You have multiple emails registered with Frontiers:
Notify me on publication
Please enter your email address:
If you already have an account, please login
You don't have a Frontiers account ? You can register here
Gliomas, the most common and aggressive primary brain tumors, exhibit significant heterogeneity and poor prognosis. Despite advancements in treatment, patient outcomes remain unsatisfactory due to therapeutic resistance and tumor recurrence. Identifying novel molecular biomarkers is crucial for improving prognosis and developing more effective therapies. In this study, we constructed a solute carrier family prognostic signature (SLCFPS) for gliomas by applying univariate Cox regression and machine learning algorithms to five independent glioma cohorts. The prognostic performance of SLCFPS was assessed using Kaplan-Meier survival analysis, concordance index (C-index), and receiver operating characteristic (ROC) curve analysis. The immune landscape, immunotherapy response, and drug sensitivity were further explored through bioinformatics tools such as ESTIMATE, xCell, TIDE, and drug response correlation analysis. SLCFPS effectively stratified glioma patients into high-and low-risk groups, with higher scores correlating with poorer survival outcomes. The model demonstrated superior predictive performance compared to existing glioma prognostic models and was associated with an immunosuppressive tumor microenvironment, as well as upregulated immune checkpoints, suggesting implications for immunotherapy response. Furthermore, SLCFPS correlated with drug sensitivity, highlighting potential therapeutic options. Overall, SLCFPS is a robust biomarker for glioma prognosis and treatment response, offering insights into tumor immunity and facilitating personalized therapeutic strategies.
Keywords: Glioma, Slc family, machine learning, Immunotherapy, prognostic biomarker
Received: 01 Mar 2025; Accepted: 26 Mar 2025.
Copyright: © 2025 Liu. This is an open-access article distributed under the terms of the Creative Commons Attribution License (CC BY). The use, distribution or reproduction in other forums is permitted, provided the original author(s) or licensor are credited and that the original publication in this journal is cited, in accordance with accepted academic practice. No use, distribution or reproduction is permitted which does not comply with these terms.
* Correspondence: Yu'e Liu, Boston Children's Hospital, Harvard Medical School, Boston, United States
Disclaimer: All claims expressed in this article are solely those of the authors and do not necessarily represent those of their affiliated organizations, or those of the publisher, the editors and the reviewers. Any product that may be evaluated in this article or claim that may be made by its manufacturer is not guaranteed or endorsed by the publisher.
Supplementary Material
Research integrity at Frontiers
Learn more about the work of our research integrity team to safeguard the quality of each article we publish.