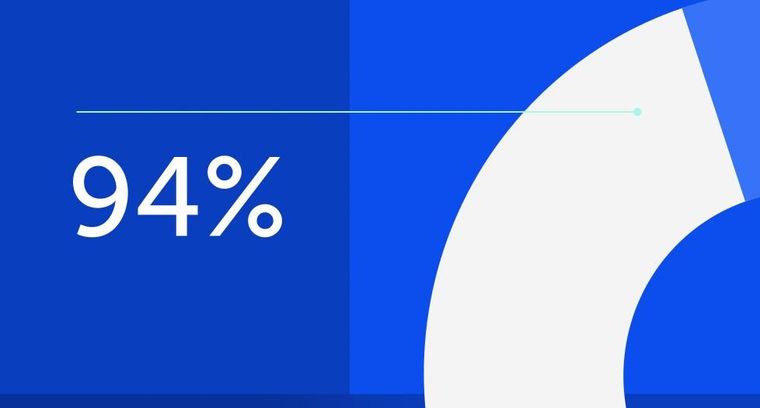
94% of researchers rate our articles as excellent or good
Learn more about the work of our research integrity team to safeguard the quality of each article we publish.
Find out more
ORIGINAL RESEARCH article
Front. Pharmacol.
Sec. Drug Metabolism and Transport
Volume 16 - 2025 | doi: 10.3389/fphar.2025.1578117
This article is part of the Research TopicIntegrated PK/PD and Drug Metabolism Approaches in Drug Development and EvaluationView all 3 articles
The final, formatted version of the article will be published soon.
Select one of your emails
You have multiple emails registered with Frontiers:
Notify me on publication
Please enter your email address:
If you already have an account, please login
You don't have a Frontiers account ? You can register here
The objective of this study is to develop an artificial intelligence-physiologically based pharmacokinetic (AI-PBPK) model to predict the pharmacokinetic (PK) and pharmacodynamic (PD) properties of aldosterone synthase inhibitors (ASIs), enabling selection of the right candidate with high potency and good selectivity at the drug discovery stage. On a web-based platform, an AI-PBPK model, integrating machine learning and a classical PBPK model for the PK simulation of ASIs, was developed. Baxdrostat, with the most clinical data available, was selected as the model compound. Following calibration and validation using published data, the model was applied to estimate the PK parameters of Baxdrostat, Dexfadrostat, Lorundrostat, BI689648, and the 11β-hydroxylase inhibitor LCI699. The PD of all five compounds was predicted based on plasma free drug concentrations. The results demonstrated that the PK/PD properties of an ASI could be inferred from its structural formula within a certain error range, providing a reference for early ASI lead compounds screening and optimization. Further validation and refinement of this model will enhance its predictive accuracy and expand its application in drug discovery.
Keywords: AI-PBPK, PK/PD simulation, CYP11B2, Aldosterone synthase inhibitors, machine learning
Received: 17 Feb 2025; Accepted: 11 Apr 2025.
Copyright: © 2025 Zhang, Wu, Long, Jin and Liu. This is an open-access article distributed under the terms of the Creative Commons Attribution License (CC BY). The use, distribution or reproduction in other forums is permitted, provided the original author(s) or licensor are credited and that the original publication in this journal is cited, in accordance with accepted academic practice. No use, distribution or reproduction is permitted which does not comply with these terms.
* Correspondence: Bo Liu, School of Chemistry and Chemical Engineering, Shanghai University of Engineering Sciences, Shanghai, 201620, China
Disclaimer: All claims expressed in this article are solely those of the authors and do not necessarily represent those of their affiliated organizations, or those of the publisher, the editors and the reviewers. Any product that may be evaluated in this article or claim that may be made by its manufacturer is not guaranteed or endorsed by the publisher.
Supplementary Material
Research integrity at Frontiers
Learn more about the work of our research integrity team to safeguard the quality of each article we publish.