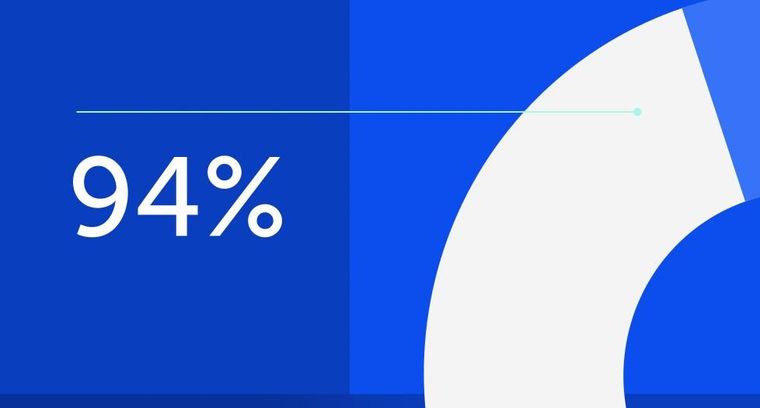
94% of researchers rate our articles as excellent or good
Learn more about the work of our research integrity team to safeguard the quality of each article we publish.
Find out more
ORIGINAL RESEARCH article
Front. Pharmacol.
Sec. Experimental Pharmacology and Drug Discovery
Volume 16 - 2025 | doi: 10.3389/fphar.2025.1574990
This article is part of the Research TopicAdvancing Drug Discovery with AI: Drug-Target Interactions, Mechanisms of Action, and ScreeningView all 5 articles
The final, formatted version of the article will be published soon.
Select one of your emails
You have multiple emails registered with Frontiers:
Notify me on publication
Please enter your email address:
If you already have an account, please login
You don't have a Frontiers account ? You can register here
Introduction: Candidiasis, mainly caused by Candida albicans, poses a serious threat to human health. The escalating drug resistance in C. albicans and the limited antifungal options highlight the critical need for novel therapeutic strategies. Methods: We evaluated 12 machine learning models on a self-constructed dataset with known anti-C. albicans activity. Based on their performance, the optimal model was selected to screen our separate in-house compound library with unknown anti-C. albicans activity for potential antifungal agents. The anti-C. albicans activity of the selected compounds was confirmed through in vitro drug susceptibility assays, hyphal growth assays, and biofilm formation assays. Through transcriptomics, proteomics, iron rescue experiments, CTC staining, JC-1 staining, DAPI staining, molecular docking, and molecular dynamics simulations, we elucidated the mechanism underlying the anti-C. albicans activity of the compound.Result: Among the evaluated machine learning models, the best predictive model was an ensemble learning model constructed from Random Forests and Categorical Boosting using soft voting. It predicts that Dp44mT exhibits potent anti-C. albicans activity. The in vitro tests further verified this finding that Dp44mT can inhibit planktonic growth, hyphal formation, and biofilm formation of C. albicans. Mechanistically, Dp44mT exerts antifungal activity by disrupting cellular iron homeostasis, leading to a collapse of mitochondrial membrane potential and ultimately causing apoptosis. Conclusion: This study presents a practical approach for predicting the antifungal activity of com-pounds using machine learning models and provides new insights into the development of antifungal compounds by disrupting iron homeostasis in C. albicans.
Keywords: ensemble learning, multi-omics, Dp44mT, iron homeostasis, Candida albicans
Received: 11 Feb 2025; Accepted: 11 Apr 2025.
Copyright: © 2025 Chai, Jiang, Lu and Huang. This is an open-access article distributed under the terms of the Creative Commons Attribution License (CC BY). The use, distribution or reproduction in other forums is permitted, provided the original author(s) or licensor are credited and that the original publication in this journal is cited, in accordance with accepted academic practice. No use, distribution or reproduction is permitted which does not comply with these terms.
* Correspondence: Hui Lu, Tongji University, Shanghai, China
Disclaimer: All claims expressed in this article are solely those of the authors and do not necessarily represent those of their affiliated organizations, or those of the publisher, the editors and the reviewers. Any product that may be evaluated in this article or claim that may be made by its manufacturer is not guaranteed or endorsed by the publisher.
Supplementary Material
Research integrity at Frontiers
Learn more about the work of our research integrity team to safeguard the quality of each article we publish.