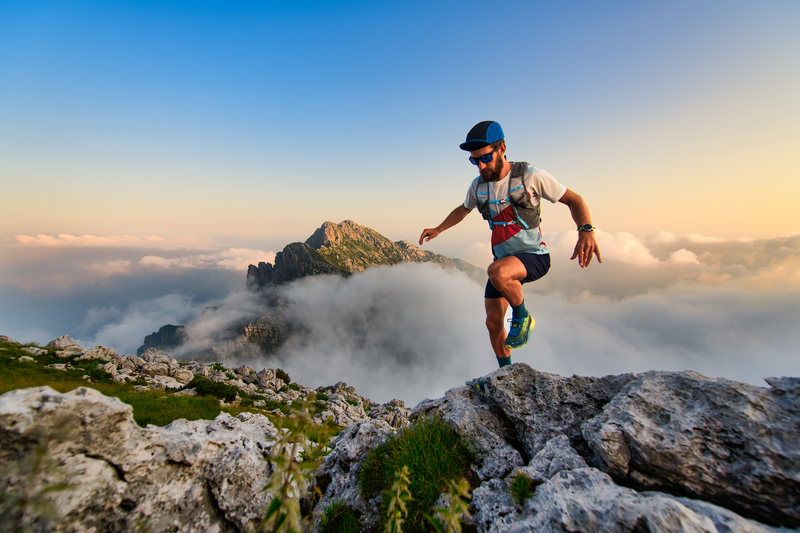
94% of researchers rate our articles as excellent or good
Learn more about the work of our research integrity team to safeguard the quality of each article we publish.
Find out more
ORIGINAL RESEARCH article
Front. Pharmacol.
Sec. Experimental Pharmacology and Drug Discovery
Volume 16 - 2025 | doi: 10.3389/fphar.2025.1569765
This article is part of the Research Topic Intelligent Computing for Integrating Multi-Omics Data in Disease Diagnosis and Drug Development View all 3 articles
The final, formatted version of the article will be published soon.
You have multiple emails registered with Frontiers:
Please enter your email address:
If you already have an account, please login
You don't have a Frontiers account ? You can register here
Pancreatic ductal adenocarcinoma (PDAC) remains one of the deadliest cancers due to its late diagnosis, resistance to therapy, and a dismal five-year survival rate of only 12%. Overexpression of PKMYT1-a key regulator of the cell cyclecorrelates with poor patient outcomes, making it a promising therapeutic target.In this study, we identify CMNPD31124, a novel marine-derived indole alkaloid, as a potent PKMYT1 inhibitor. Molecular docking revealed that CMNPD31124 has superior binding affinity compared to the reference compound Cpd 4, forming robust interactions with critical residues such as Molecular dynamics simulations further demonstrated its stable binding conformation and dynamic adaptability, with Chai-1 modeling supporting a covalent binding mechanism at the PKMYT1 active site. Importantly, in vitro assays showed that CMNPD31124 exhibits an IC₅₀ of 18.6 μM in MiaPaCa-2 cells and 31.7 μM in BXPC3 cells, while concentrations up to 80 μM did not significantly affect normal pancreatic cells. Despite these promising results, toxicity predictions indicate potential hepatotoxicity and neurotoxicity, highlighting the need for further structural optimization.This work lays a solid foundation for the rational design of PKMYT1 inhibitors by integrating computational methods with insights from marine natural products.
Keywords: PDAC, Marine Natural Products, covalent inhibitor, Molecular docking and dynamics, Biological evaluations, Toxicity assessment
Received: 01 Feb 2025; Accepted: 10 Mar 2025.
Copyright: © 2025 Huang, Wang, Chen and Xu. This is an open-access article distributed under the terms of the Creative Commons Attribution License (CC BY). The use, distribution or reproduction in other forums is permitted, provided the original author(s) or licensor are credited and that the original publication in this journal is cited, in accordance with accepted academic practice. No use, distribution or reproduction is permitted which does not comply with these terms.
* Correspondence:
Chaojie Huang, Department of Colorectal Surgery, Sir Run Run Shaw Hospital, Zhejiang University School of Medicine, Hangzhou, China
Yunyun Xu, Hangzhou Medical College, Hangzhou, 310053, Zhejiang Province, China
Disclaimer: All claims expressed in this article are solely those of the authors and do not necessarily represent those of their affiliated organizations, or those of the publisher, the editors and the reviewers. Any product that may be evaluated in this article or claim that may be made by its manufacturer is not guaranteed or endorsed by the publisher.
Research integrity at Frontiers
Learn more about the work of our research integrity team to safeguard the quality of each article we publish.