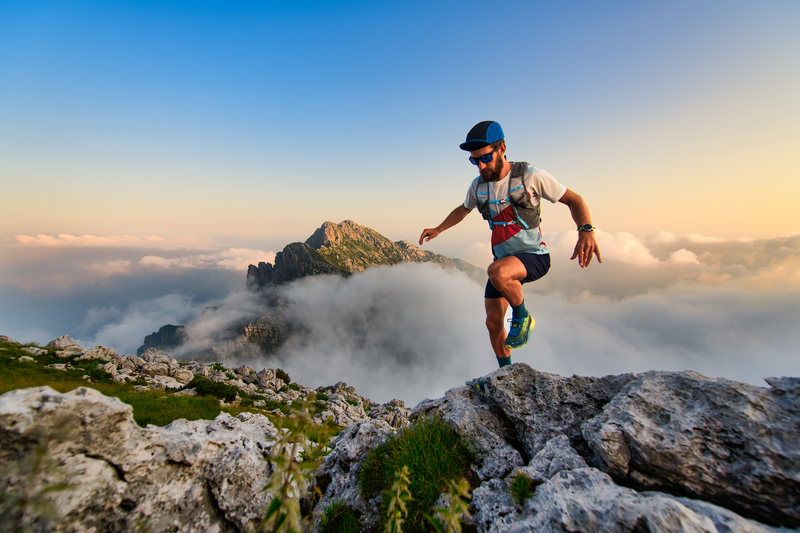
94% of researchers rate our articles as excellent or good
Learn more about the work of our research integrity team to safeguard the quality of each article we publish.
Find out more
ORIGINAL RESEARCH article
Front. Pharmacol.
Sec. Gastrointestinal and Hepatic Pharmacology
Volume 16 - 2025 | doi: 10.3389/fphar.2025.1558709
This article is part of the Research Topic New Targets and Strategies for the Prevention and Treatment of Organ Fibrosis, Volume III View all 11 articles
The final, formatted version of the article will be published soon.
You have multiple emails registered with Frontiers:
Please enter your email address:
If you already have an account, please login
You don't have a Frontiers account ? You can register here
Metabolic syndrome (MetS) is a cluster of several disorders where many challenges hinder effective treatment. The downregulation of SIRT1 or inhibition of its activity is implicated in its pathophysiology. We hypothesized that the combined SIRT1 direct activator E1231 and the SIRT1 stabilizer nicotinamide mononucleotide (NMN) could offer a novel approach to mitigate the pathophysiological features of MetS. Our results revealed that E1231 alone or combined with NMN increased SIRT1 level and activity. This SIRT1 activation was accompanied by upregulation in the IRS-1 and activation of AKT. In parallel, the Nrf2 level and activity were increased while the NFκB activity and subsequent inflammatory cytokines were decreased. Additionally, SIRT1 activation was associated with improved insulin resistance, blood pressure, lipid profile, fasting blood glucose, glucose tolerance, and kidney and liver functions. Moreover, improved liver histology, decreased hepatic fibrosis markers, and increased survival rates were observed. These protective functions were counteracted when EX527, a SIRT1 inhibitor, was dually administered with E1231. Furthermore, correlation analysis revealed that SIRT1 was negatively correlated with NFκB, insulin resistance, and oxidative stress, while positive correlations were observed between SIRT1, p-AKT, and Nrf2 activity. Random Forest regression algorithm and partial dependence plots highlighted the significant roles of SIRT1, IRS-1, p-AKT, and NFκB in predicting MetS severity. These analyses underscore the strong interconnections between these signals. This reinforces the central role of SIRT1 in coordinating a multifaceted protective response against MetS. To conclude, SIRT1 alleviates MetS by modulating AKT/Nrf2/NFκB signaling and their interactions. Further research is necessary to validate these findings.
Keywords: E1231, EX527, SIRT1, metabolic syndrome, AKT/Nrf2/NFκB, Insulin Resistance
Received: 10 Jan 2025; Accepted: 26 Feb 2025.
Copyright: © 2025 Elmorsy, Elashry, Alkhamiss, Alsaykhan, Hamad, Abd Elrahim, Alsoghair, Alharbi, Gabr, Ellethy, Khodeir, Hassan, Elsisi, Farrag, Suliman AlSoqih, Sameh and Saber. This is an open-access article distributed under the terms of the Creative Commons Attribution License (CC BY). The use, distribution or reproduction in other forums is permitted, provided the original author(s) or licensor are credited and that the original publication in this journal is cited, in accordance with accepted academic practice. No use, distribution or reproduction is permitted which does not comply with these terms.
* Correspondence:
Elsayed Abdelhadi Elmorsy, Qassim University, Buraidah, 52571, Al-Qassim, Saudi Arabia
Mustafa Ahmed Abd Elrahim, Shaqra University, Shaqraa, Riyadh, Saudi Arabia
Alshaimaa A Farrag, University of Bisha, BISHA, Saudi Arabia
Sameh Saber, Delta University for Science and Technology, Al Mansurah, Egypt
Disclaimer: All claims expressed in this article are solely those of the authors and do not necessarily represent those of their affiliated organizations, or those of the publisher, the editors and the reviewers. Any product that may be evaluated in this article or claim that may be made by its manufacturer is not guaranteed or endorsed by the publisher.
Research integrity at Frontiers
Learn more about the work of our research integrity team to safeguard the quality of each article we publish.