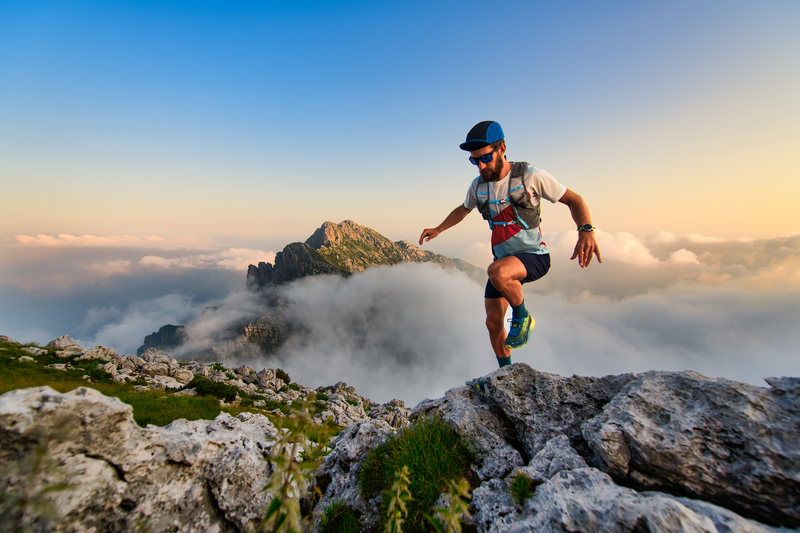
94% of researchers rate our articles as excellent or good
Learn more about the work of our research integrity team to safeguard the quality of each article we publish.
Find out more
EDITORIAL article
Front. Pharmacol. , 25 February 2025
Sec. Predictive Toxicology
Volume 16 - 2025 | https://doi.org/10.3389/fphar.2025.1556352
This article is part of the Research Topic Methods in Predictive Toxicology 2023 View all 5 articles
Editorial on the Research Topic
Methods in predictive toxicology 2023
Predictive toxicology is a pivotal field that bridges the gap between experimental findings and risk assessment, enabling the anticipation and mitigation of adverse drug reactions, and selection of safer drug candidates early in drug discovery and development (Amorim et al., 2024; Tonoyan and Siraki, 2024). As the complexity of chemical substances and pharmaceuticals continues to grow, so does the need for innovative methods to predict their potential toxicities (Pognan et al., 2023). Moreover, modulating novel proteins with limited knowledge of safety profiles demands a robust integration of computational and experimental approaches to gain mechanistic insights into potential adverse outcomes.
This Research Topic, part of the Methods in Pharmacology series, highlights significant advancements in computational modeling, mechanistic insights, and experimental methodologies that enhance predictive toxicology. The articles in this Research Topic demonstrate how these tools, combined with omics technologies and pharmacovigilance data, refine toxicity predictions, reduce reliance on animal testing, and improve our understanding of toxicological mechanisms. Together, these approaches aim to ensure safer therapeutic interventions for patients.
Advancements in omics technologies and computational methods offer deeper mechanistic insights into toxicological processes. Two studies in this Research Topic leverage these approaches to unravel the underlying mechanisms of drug-induced toxicity.
The study “Potential mechanisms underlying podophyllotoxin-induced cardiotoxicity in male rats: toxicological evidence chain (TEC) concept” Ma et al. employs an integrative framework combining transcriptomics and targeted metabolomics to explore the cardiotoxic effects of podophyllotoxin (PPT). By constructing a toxicological evidence chain (TEC), the researchers identify key pathways involved in PPT-induced cardiac injury, including oxidative stress, apoptosis, inflammatory responses, and disruptions in energy metabolism. This comprehensive approach not only elucidates the molecular mechanisms of PPT cardiotoxicity but also provides a template for investigating other compounds with complex toxicological profiles.
In “Quantum chemical calculations of nitrosamine activation and deactivation pathways for carcinogenicity risk assessment,” Göller et al. the authors use quantum chemical calculation to assess the carcinogenic potential of N-nitrosamines, a class of compounds of significant concern due to their presence as impurities in pharmaceuticals. By calculating and comparing activation energy profiles of carcinogenic and non-carcinogenic nitrosamines, they uncover distinct differences in their reactivity and stability. This computational approach predicts mutagenic and carcinogenic risks associated with N-nitrosamines, demonstrating the power of in silico methods in regulatory decision-making.
Pharmacovigilance plays a crucial role in detecting and analyzing adverse drug reactions post-marketing. Two studies in this Research Topic exemplify how large-scale data analysis can provide invaluable insights into drug safety.
In “Osteonecrosis of the jaw in patients with clear cell renal cell carcinoma treated with targeted agents: a case series and large-scale pharmacovigilance analysis,” Wang et al. the authors investigate the association between tyrosine kinase inhibitors (TKIs), immune checkpoint inhibitors (ICIs), and osteonecrosis of the jaw (ONJ). Utilizing the FDA Adverse Event Reporting System (FAERS), they perform disproportionality analyses to detect signals indicating a higher risk of ONJ with specific agents like sunitinib and lenvatinib. This study underscores the importance of continuous monitoring and data mining in identifying rare but serious adverse events, contributing to safer therapeutic strategies for patients with renal cell carcinoma.
Similarly, the article “Post-marketing safety evaluation of lurbinectedin: a pharmacovigilance analysis based on the FAERS database” Li et al. focuses on lurbinectedin, a novel agent approved for metastatic small-cell lung cancer. The researchers employ multiple algorithms to assess adverse event reports and identify known toxicities such as hematological effects and gastrointestinal symptoms. Notably, they also detect a new safety signal—tumor lysis syndrome—highlighting the dynamic nature of drug safety profiles post-approval. This work demonstrates how pharmacovigilance data can reveal emerging risks and guide clinicians in monitoring and managing side effects.
The studies featured in this Research Topic highlight the evolving landscape of predictive toxicology, where interdisciplinary approaches are essential. The integration of pharmacovigilance data with mechanistic studies provides more precise toxicity predictions and contributes to the development of safer drugs. Looking ahead, future research should expand on these methods, by incorporating artificial intelligence and machine learning to analyze complex datasets and identify subtle patterns. Efforts to standardize protocols and promote data sharing will accelerate progress and foster interdisciplinary collaboration. Additionally, the adoption and advancement in high-throughput omics technologies and computational modeling promise to reduce animal testing by providing alternative approaches to assess toxicities. By understanding the molecular basis of toxic effects, researchers can design targeted interventions and develop predictive models that are both accurate and tailored to specific mechanisms, drug classes, or target contexts.
This Research Topic exemplifies how innovative methods are transforming predictive toxicology. By leveraging pharmacovigilance large-scale data analysis with mechanistic insights, these studies contribute significantly to uncovering toxicological insights and drug safety. We thank all the authors for their valuable contributions and the reviewers for their critical feedback. We hope that this Research Topic will inspire further research and dialogue, ultimately advancing the field of predictive toxicology and enhancing its impact on public health.
SJ: Conceptualization, Writing–original draft, Writing–review and editing. SM: Writing–review and editing. VG: Writing–review and editing. MR: Writing–review and editing. AM: Writing–review and editing.
The author(s) declare that financial support was received for the research, authorship, and/or publication of this article. SJ acknowledge support, in part by the Intramural/Extramural Research Program of the NCATS, NIH.
Author VG was employed by UCB. Author AM was employed by Boehringer Ingelheim Pharma GmbH & Co. KG.
The remaining authors declare that the research was conducted in the absence of any commercial or financial relationships that could be construed as a potential conflict of interest.
The authors declare that Gen AI was used in the creation of this manuscript. Generative AI (ChatGPT) was utilized for tasks including content refinement and formatting adjustments to ensure clarity and coherence. All content generated or modified by AI was carefully reviewed and edited by the authors to maintain scientific accuracy and originality.
All claims expressed in this article are solely those of the authors and do not necessarily represent those of their affiliated organizations, or those of the publisher, the editors and the reviewers. Any product that may be evaluated in this article, or claim that may be made by its manufacturer, is not guaranteed or endorsed by the publisher.
Amorim, A. M. B., Piochi, L. F., Gaspar, A. T., Preto, A. J., Rosário-Ferreira, N., and Moreira, I. S. (2024). Advancing drug safety in drug development: bridging computational predictions for enhanced toxicity prediction. Chem. Res. Toxicol. 37, 827–849. doi:10.1021/acs.chemrestox.3c00352
Pognan, F., Beilmann, M., Boonen, H. C. M., Czich, A., Dear, G., Hewitt, P., et al. (2023). The evolving role of investigative toxicology in the pharmaceutical industry. Nat. Rev. Drug Discov. 22, 317–335. doi:10.1038/s41573-022-00633-x
Keywords: predictive toxicology with AI, pharmacovigilance, machine learning, data-driven decision making, drug development, drug safety, computational modeling
Citation: Jain S, Manganelli S, Gryshkova V, Rodrigues MA and Magarkar A (2025) Editorial: Methods in predictive toxicology 2023. Front. Pharmacol. 16:1556352. doi: 10.3389/fphar.2025.1556352
Received: 06 January 2025; Accepted: 10 January 2025;
Published: 25 February 2025.
Edited and Reviewed by:
Ursula Gundert-Remy, Charité University Medicine Berlin, GermanyCopyright © 2025 Jain, Manganelli, Gryshkova, Rodrigues and Magarkar. This is an open-access article distributed under the terms of the Creative Commons Attribution License (CC BY). The use, distribution or reproduction in other forums is permitted, provided the original author(s) and the copyright owner(s) are credited and that the original publication in this journal is cited, in accordance with accepted academic practice. No use, distribution or reproduction is permitted which does not comply with these terms.
*Correspondence: Sankalp Jain, c2Fua2FscC5qYWluQG5paC5nb3Y=
Disclaimer: All claims expressed in this article are solely those of the authors and do not necessarily represent those of their affiliated organizations, or those of the publisher, the editors and the reviewers. Any product that may be evaluated in this article or claim that may be made by its manufacturer is not guaranteed or endorsed by the publisher.
Research integrity at Frontiers
Learn more about the work of our research integrity team to safeguard the quality of each article we publish.