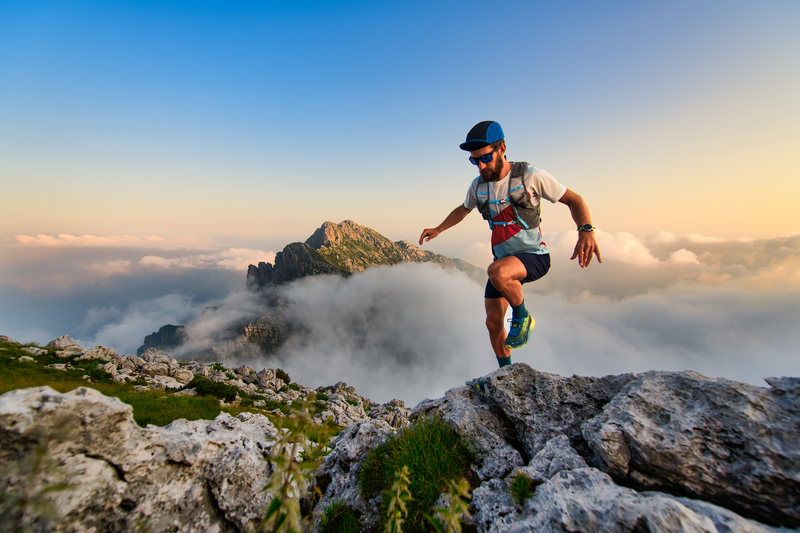
94% of researchers rate our articles as excellent or good
Learn more about the work of our research integrity team to safeguard the quality of each article we publish.
Find out more
ORIGINAL RESEARCH article
Front. Pharmacol.
Sec. Experimental Pharmacology and Drug Discovery
Volume 16 - 2025 | doi: 10.3389/fphar.2025.1553743
This article is part of the Research Topic Advancing Drug Discovery with AI: Drug-Target Interactions, Mechanisms of Action, and Screening View all 4 articles
The final, formatted version of the article will be published soon.
You have multiple emails registered with Frontiers:
Please enter your email address:
If you already have an account, please login
You don't have a Frontiers account ? You can register here
Background: Predicting drug-target interaction (DTI) is a crucial phase in drug discovery. The core of DTI prediction lies in appropriate representations learning of drug and target. Previous studies have confirmed the effectiveness of graph neural networks (GNNs) in drug compound feature encoding. However, these GNN-based methods do not effectively balance the local substructural features with the overall structural properties of the drug molecular graph.In this study, we proposed a novel model named GNNBlockDTI to address the current challenges. We combined multiple layers of GNN as a GNNBlock unit to capture the hidden structural patterns from drug graph within local ranges. Based on the proposed GNNBlock, we introduced a feature enhancement strategy to re-encode the obtained structural features, and utilized gating units for redundant information filtering. To simulate the essence of DTI that only protein fragments in the binding pocket interact with drugs, we provided a local encoding strategy for target protein using variant convolutional networks.Results: Experimental results on three benchmark datasets demonstrated that GNNBlockDTI is highly competitive compared to the state-of-the-art models. Moreover, the case study of drug candidates ranking against different targets affirms the practical effectiveness of GNNBlockDTI.
Keywords: Graph neural network, Drug Discovery, graph representation learning, Molecular substructure, Drug-target Interaction Drug-target Interaction Prediction
Received: 31 Dec 2024; Accepted: 13 Feb 2025.
Copyright: © 2025 Deng, Shi, Ge, Hu, Wang, Qin, Pan, Mao and Yang. This is an open-access article distributed under the terms of the Creative Commons Attribution License (CC BY). The use, distribution or reproduction in other forums is permitted, provided the original author(s) or licensor are credited and that the original publication in this journal is cited, in accordance with accepted academic practice. No use, distribution or reproduction is permitted which does not comply with these terms.
* Correspondence:
Changsheng Shi, Third Affiliated Hospital of Wenzhou Medical University, Wenzhou, Zhejiang Province, China
Ruiquan Ge, Hangzhou Dianzi University, Hangzhou, China
Riqian Hu, University of California, San Diego, La Jolla, 92093, California, United States
Feiwei Qin, Hangzhou Dianzi University, Hangzhou, China
Cheng Pan, Sanda University, Shanghai, 201209, Shanghai Municipality, China
Haixia Mao, Shenzhen Polytechnic, Shenzhen, 518055, Guangdong, China
Qing Yang, Third Affiliated Hospital of Wenzhou Medical University, Wenzhou, Zhejiang Province, China
Disclaimer: All claims expressed in this article are solely those of the authors and do not necessarily represent those of their affiliated organizations, or those of the publisher, the editors and the reviewers. Any product that may be evaluated in this article or claim that may be made by its manufacturer is not guaranteed or endorsed by the publisher.
Research integrity at Frontiers
Learn more about the work of our research integrity team to safeguard the quality of each article we publish.