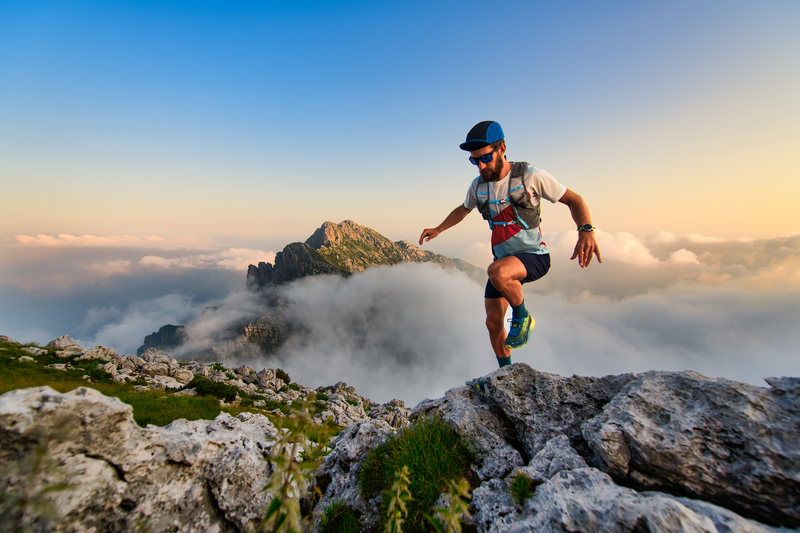
95% of researchers rate our articles as excellent or good
Learn more about the work of our research integrity team to safeguard the quality of each article we publish.
Find out more
ORIGINAL RESEARCH article
Front. Pharmacol. , 17 March 2025
Sec. Pharmacogenetics and Pharmacogenomics
Volume 16 - 2025 | https://doi.org/10.3389/fphar.2025.1553536
Background: Pharmacogenomics offers a possibility of anticipating drug response based on individuals’ genetic profiles and represents a step toward implementation of personalized treatment through routine genetic testing. Development of highthroughput sequencing technologies aided identification and interpretation of variants in many pharmacogenes simultaneously. Nonetheless, the integration of pharmacogenomics into clinical practice is arduous, partly due to insufficient knowledge of ethnic pharmacogenetic data. The aim of our study was to assemble the most comprehensive pharmacogenomics landscape of the Serbian population so far.
Methods: We used genomic data of 881 individuals from Serbia obtained by clinical and whole exome sequencing. Raw sequencing files were processed using an in-house pipeline for alignment and variant calling. For annotation of pharmacogenetics star alleles and determination of phenotypes, we used the PharmCAT and Stargazer tools. Star allele and phenotype frequencies were calculated and compared to worldwide and European populations. Population differentiation was presented through calculation of Wright’s fixation index.
Results: Our results showed that population differentiation was the highest between the Serbian and the worldwide population. In the Serbian population, the most relevant pharmacogenes in terms of star allele frequencies and actionable phenotypes were CYP2B6, NAT2, SLCO1B1, UGT1A1 and VKORC1, that had significantly different distribution compared to other European populations.
Conclusion: In conclusion, significant differences in frequencies of pharmacogenetic phenotypes that influence response to several drug categories including statins and antidepressants indicate that inclusion of data relevant for drug response to genetic reports would be beneficial in the Serbian population. Implementation of pharmacogenetic testing could be achieved through analysis of clinical and whole exome sequencing data.
With a goal of maximizing drug efficacy and safety according to the genetic profile of each patient, pharmacogenomics (PGx) represents an aspect of precision medicine that has greatly benefited from technological advancements (Ng et al., 2017). Expansion and cost-effectiveness of next-generation sequencing (NGS) facilitated shifting from a one-size-fits-all approach towards precision medicine, which focuses on patients’ unique genetic signatures (Morash et al., 2018). Clinical implementation of pharmacogenomics is already being performed, mostly for single genes or panels of variants with a well-described effect on drug response (Tafazoli et al., 2021; Russell et al., 2021). Clinical implementation relies on drug prescribing guidelines provided by pharmacogenomics associations, most notably the Clinical Pharmacogenetics Implementation Consortium (CPIC) and the Dutch Pharmacogenetics Working Group (DPWG). CPIC and DPWG provide a scoring system and level of evidence associated with each gene-drug pair. A similar system for ranking pharmacogenomic evidence is provided by the Pharmacogenomics Knowledge Base (PharmGKB), which is the most comprehensive resource for pharmacogenomics.
Although a considerable amount of data concerning gene effect on drug response is already available, implementation of PGx knowledge into clinical practice is still scarce (Kabbani et al., 2023). Next-generation sequencing is widely used for molecular diagnostics and other clinical analyses, leaving a plethora of genomic data available for identification and interpretation of genetic variants and haplotypes, or star alleles, that influence drug response (Ji et al., 2018). Constant increase of sequencing power and decrease in cost of sequencing offer possibilities for fast and cost-effective, as well as preemptive and comprehensive pharmacogenomics profiling.
Furthermore, interethnic genome landscape differences are important for the implementation of PGx into clinical settings (Karamperis et al., 2024; Zhou and Lauschke, 2022). The genomic landscape of the Serbian population was previously described, showing unique features in this population (Drljaca et al., 2021; Paschou et al., 2014). The data remaining after diagnostic sequencing has a great potential for characterizing the pharmacogenomic profile of a specific population (Nunez-Torres et al., 2023; Hočevar, Maver, and Peterlin, 2019; Zhang and Lauschke, 2019). Meticulous depiction of subpopulations brings the opportunity for better risk stratification, leading to the development of population-specific genotyping strategies (Karamperis et al., 2024; Zhou and Lauschke, 2022; Wright et al., 2018).
Technological advancement elicited a trend of population-wide precision medicine, with many Western Balkan countries reporting specific pharmacogenomic profiles (Hočevar, Maver, and Peterlin, 2019; Proietti et al., 2023; Jmel et al., 2024; Matišić et al., 2023; Perović et al., 2024; Velizarova et al., 2022). In Serbia, a considerable number of studies addressing response to different drugs were performed, including treatment of pediatric acute lymphoblastic leukemia (Dokmanovic et al., 2006; Kotur et al., 2020), inflammatory bowel disease (Stankovic et al., 2020; Jojic et al., 2003), rheumatoid arthritis (Jančić et al., 2013; Jančić et al., 2015) etc. However, a comprehensive understanding of the pharmacogenomic landscape in the Serbian population remains lacking. Re-use of the large amount of NGS data generated through genetic testing of patients with suspected rare diseases presents an opportunity to assess population-level genomic and pharmacogenomic characteristics in Serbia (Gemovic and Veljkovic, 2023; Andjelkovic et al., 2024; Stankovic et al., 2020).
In this study, we aimed to investigate the pharmacogenomic landscape of the Serbian population by analyzing over 50 pharmacogenes using large-scale NGS data and bioinformatics tools tailored for PGx. The focus was to determine the frequency of star alleles and PGx phenotypes and to compare our findings with the worldwide and European population, aiming to identify any unique PGx characteristics of the Serbian population. Another goal of our study was to assess the feasibility of using CES and WES for the detection of PGx variants relevant for drug response and therapy optimization.
The genetic data of 881 individuals from the Serbian population were used in this study. DNA was isolated from blood samples, which were subsequently sequenced either with clinical or whole exome panels. Samples were sequenced over a 2-year period, from 2023 to 2024. For the presented study, data of all patients were de-identified, with subject sex being the only retained information relevant for G6PD genotyping. No phenotype data was available. The study and use of genomic data was approved by the Ethics Committee of the Institute of Molecular Genetics and Genetic Engineering, University of Belgrade (O-EO-046/2023).
The majority of samples (768 samples) underwent clinical exome sequencing (TruSight One Sequencing Panel, Illumina, CA, United States) and the rest of the subjects (113 samples) were sequenced using a whole exome panel (Illumina DNA Prep with Exome 2.0 Plus Enrichment, Illumina, CA, United States). The clinical exome sequencing panel covers selected exons of 4,813 genes, containing variants which have clinical relevance. Whole exome sequencing provides significantly more information, since it covers all exons and even several important intronic regions.
Raw files obtained with NGS were processed using an in-house pipeline that follows the GATK Best Practices guidelines (Poplin et al., 2017). Mapping was performed on the hg38 reference genome assembly provided by GATK, using the bwa-mem algorithm. Alignment files obtained this way were further processed using GATK tools for marking duplicates and recalibrating base quality scores to create analysis-ready BAM files. These were then used to perform variant calling with HaplotypeCaller along with appropriate intervals to create gVCF files, which were combined into two groups: one analyzed with a clinical exome and the other with a whole exome sequencing panel. Joint genotyping and filtering was then performed on these two groups to obtain multi-sample VCF files for downstream analysis. The joint genotyping was done using GenotypeGVCFs with the added flag--force-output-intervals to make sure that known pharmacogenomics variants do not get missed if they have a reference value in the entire group. For this purpose we used genomic positions of all relevant pharmacogenetic variants, provided by the PharmCAT tool (Sangkuhl et al., 2020). Created multi-sample VCF files were ready to use in the PharmCAT downstream analysis that involved pre-processing, allele matching and phenotype assignment. The PharmCAT json2tsv python script and in-house written R script were used to extract and organize data in the multi-sample tabular file format for subsequent statistical analysis.
Additionally, single sample BAM and VCF files were used for analysis with the Stargazer v2.0 tool (Lee et al., 2019). Stargazer is also used for calling star alleles in PGx genes, but unlike PharmCAT, it can detect complex structural variants by referring to read depth for calculation of paralog-specific copy numbers. Read depth is determined by comparing depths in a gene of interest, flagged by--target-gene, and a preselected control gene used as a normalization factor for copy number analysis (flagged by--control-gene). To this end, Stargazer uses BAM files for creation of a specific GATK-DepthOfCoverage format (GDF) file in form of a table, which stores read depth information. By combining annotated VCF with a created GDF, Stargazer extracts variants in selected pharmacogenes.
As a final result, both PharmCAT and Stargazer provide a combination of 2 haplotypes or star alleles (diplotype) for each sample and for each pharmacogene. Also, for each diplotype, a specific phenotype is assigned, related to each pharmacogene.
Genotyping of PGx genes was performed using two different tools: PharmCAT (Sangkuhl et al., 2020) and Stargazer (Lee et al., 2019). PharmCAT is an annotation tool that uses genetic information from VCF files on 18 genes associated with CPIC and DPWG guidelines (ABCG2, CACNA1S, CFTR, CYP2B6, CYP2C19, CYP2C9, CYP3A4, CYP3A5, CYP4F2, DPYD, G6PD, IFNL3, NUDT15, RYR1, SLCO1B1, TPMT, UGT1A1, VKORC1), converts genome variants into PGx star alleles and interprets phenotype given the diplotype information. The output of this software is a report with genotype-based prescribing recommendations.
Stargazer can determine haplotypes in genes covered by PharmCAT as well as 39 other PGx genes (2C cluster, ABCB1, CYP17A1, CYP19A1, CYP1A1, CYP1A2, CYP1B1, CYP26A1, CYP2A13, CYP2A6, CYP2C8, CYP2D6, CYP2E1, CYP2F1, CYP2J2, CYP2R1, CYP2S1, CYP2W1, CYP3A43, CYP3A7, CYP4A11, CYP4A22, CYP4B1, GSTM1, GSTP1, NAT1, NAT2, POR, PTGIS, SLC15A2, SLC22A2, SLCO1B3, SLCO2B1, SULT1A1, TBXAS1, UGT1A4, UGT2B7, UGT2B15, XPC).
Since CES panel does not cover all exons, for genes lacking full coverage only whole exome data were retained, namely, for genes 2C cluster, CYP2S1, GSTM1, NUDT15 and SLC15A2. Moreover, some well-described PGx variants are found in intronic regions, as is the case for the UGT1A1 TATA box short tandem repeats-related alleles. In such cases, frequencies were calculated separately for CES or WES data. Finally, for genes in which all variants are read with both CES and WES, data was gathered and summed frequencies were calculated (Supplementary Data Sheets 2–9). Table 1 shows the summary of genes represented with clinical, whole exome or both sequencing panels.
Table 1. Lists of genes for which variant frequencies were calculated using either data from clinical exome, whole exome or both sequencing panels.
All statistical analysis were performed in R software v.4.3.0.
Quality control of genotyping data was done using HardyWeinberg package (Graffelman, 2015). For each star allele Hardy-Wienberg equilibrium (HWE) was tested by comparing the number of carriers of the considered allele versus all others.
The population pharmacogenomics study compared star allele frequencies in the Serbian population with those in the worldwide, European and Croatian populations. Information on star allele frequencies in the worldwide and European populations defined by single variants was retrieved from the CPIC and the gnomAD (Chen et al., 2024) databases. However, frequencies of star alleles representing haplotypes defined by multiple variants could not be directly obtained from the gnomAD or CPIC databases. Instead, these haplotype frequencies were determined using the LDpair Tool (Machiela and Chanock, 2015), which provides frequencies for variants in linkage disequilibrium based on data from the 1000 Genomes Project. For the Croatian population, frequencies of variants in PGx genes were extracted from previously published population study (Matišić et al., 2023) and compared with frequencies in the Serbian population. All frequency comparisons in this study were performed using the Chi-squared test, provided that the expected frequency in each cell of the 2 × 2 table was at least 5. Otherwise, Fisher’s Exact Test was used. Wright’s fixation index (Fst) was used as a measure of population similarity. Calculation of Fst was performed with bigsnpr (Privé et al., 2018) package. Both Stargazer and PharmCAT provide predicted phenotypes for star alleles in actionable pharmacogenes. Predicted phenotypes that were detected in the Serbian population were compared with European subpopulations (1,000 Genome Project Data - Central European, Finnish, Great Britain, Iberian population in Spain and Tuscans from Italy) (Sherman, Claw, and Lee, 2024) using Fisher’s Exact or Chi-squared test. Bonferroni correction was used to adjust the statistical significance levels for multiple tests in order to reduce the number of false positive results. The correction was applied by dividing 0.05 by the number of pharmacogenes analyzed in population comparisons tests.
Clinical exome samples achieved a mean target coverage of 90×, while whole exome samples achieved a mean target coverage of 73×, with 92% and 86% of all positions achieving depth over 30×, respectively (Supplementary Data Sheet 10).
HWE was used as a measure of genotyping quality. Based on the number of tested pharmacogenes, the level of significance was determined by dividing 0.05 with the number of pharmacogenes with at least 2 different alleles. Adjusted p values were <0.001 (0.05/47), <0.0009 (0.05/54) and <0.002 (0.05/30) for CES, WES or the combination of both panels respectively. Pharmacogenes that harbored variants deviating from HWE were withdrawn from subsequent analysis (Supplementary Table S1; Supplementary Data Sheet 1). If one variant was out of HWE when clinical exome panel was used, but was in HWE if whole exome sequencing was performed, results from whole exome sequencing were retained. Only reference alleles were found in CACNA1S, CYP17A1 and CYP2R1, therefore these pharmacogenes were excluded from further comparisons as well (Supplementary Table S1).
Pharmacogenetic annotation was performed using PharmCAT (Sangkuhl et al., 2020) and Stargazer (Lee et al., 2019) tools. Differences in haplotype calling were noted between PharmCAT and Stargazer (Table 2). These differences were further inspected using BAM files of subjects. Population pharmacogenomic analysis was performed with results from the annotation tool which gave correct results based on variants found in the BAM file (Table 2). Furthermore, when pharmacogenes harbored important intronic variants that could only be detected with whole exome sequencing, only data from WES were retained for subsequent analysis (Table 1). After removing incorrectly annotated pharmacogenes, Stargazer annotated 170 haplotypes across 47 pharmacogenes, while PharmCAT found 62 star alleles in 13 pharmacogenes (Supplementary Table S2).
Table 2. Pharmacogenes annotated with PharmCAT or Stargazer based on tool performance after inspecting BAM files. Discordance in haplotype (star allele) calling between PharmCAT and Stargazer is represented in parenthesis (CES | WES).
According to Stargazer, the highest number of alternative haplotypes were found in CYP2D6 (N = 12), whereas the information about CYP2D6 haplotypes was not available in PharmCAT. PharmCAT reported RYR1 as the most variable pharmacogene, with 18 alternative haplotypes. Out of 52 analyzed pharmacogenes annotated by either Stargazer or PharmCAT, the highest variability (percent of non-reference haplotypes, or alternative haplotypes) was found for 2 genes–CYP3A5 (93%) and CYP2C19 (92%). The least variable pharmacogenes were CYP26A1 (0.1%), CFTR (0.5%), G6PD (1.0%), PTGIS (1.3%), NUDT15 (1.3%), TBXAS1 (3.1%) and TPMT (3.3%) (Supplementary Data Sheet 6). Taking into account PGx actionability and frequency in the Serbian population, the most relevant PGx variants are CYP2C9*2, G6PD Gond, NAT2*7, SLCO1B1*5, SLCO1B1*14, SLCO1B1*15, UGT1A1*6, UGT1A1*28, UGT1A1*36 and VKORC1 rs9923231 (Table 4).
After inspecting the results of PGx annotation with PharmCAT and Stargazer, sequencing panels used herein were compared to determine their usefulness in PGx testing (Table 3). For 7 out of 20 pharmacogenes relevant for drug response and therapy optimization based on CPIC guidelines, that were annotated by PharmCAT or Stargazer, the clinical exome sequencing panel gives sufficient information. However, CES cannot capture most of the variants important for drug response in a number of pharmacogenes (13 out of 20). In such cases, whole exome sequencing is needed (Table 3).
Table 3. Pharmacogenes annotated by PharmCAT or Stargazer that have CPIC published guidelines and can be analysed using clinical exome or whole exome sequencing panels.
Overall, 209 star alleles were detected in the Serbian population and their frequencies were further compared to worldwide and European populations. Allele frequencies in the general and European population were extracted from the gnomAD database and from CPIC, while frequencies of PGx haplotypes in the Croatian population were extracted from a previously published study (Matišić et al., 2023). Bonferroni correction for multiple comparison was applied by dividing 0.05 with 52 (number of analyzed genes) and differences between populations were considered significant when p value was <0.0009. Differences in haplotype frequencies were found for 35 out of 52 pharmacogenes.
Compared to the worldwide population, frequencies of 60 star alleles in 31 pharmacogenes were significantly different in the Serbian population, with SLCO1B1 and NAT1 harboring the highest number of haplotypes with significantly differing frequencies (higher frequency in the Serbian population: SLCO1B1*5 p < 2.2*10−16, SLCO1B1*14 p = 5.2*10−6, SLCO1B1*19 p = 1.6*10−5, NAT1*15 p = 1.1*10−6, NAT1*19 p = 1.8*10−5, NAT1*22 p = 1.3*10−5; higher frequency in worldwide population: SLCO1B1*15 p = 3.4*10−7, SLCO1B1*37 p < 2.2*10−16, NAT1*17 p = 7.6*10−4).
In comparison to the European population, 48 star alleles in 29 pharmacogenes had statistically significant differences (Supplementary Data Sheets 2–5). Similar to the worldwide population comparison, NAT1 had the highest number of star alleles with differing frequencies (higher frequency in the Serbian population: NAT1*15 p = 4.2*10−6, NAT1*19 p = 8.4*10−6, NAT1*22 p = 5.9*10−5; higher frequency in European population: NAT1*17 p = 6.31*10−5).
Haplotype frequencies were also compared to the Croatian population for 14 pharmacogenes. Due to differences in genotyping panels between the Serbian and the Croatian population, comparisons included only variants covered by both studies. We found differences in star allele frequencies in 4 pharmacogenes between the Serbian and the Croatian group (CYP2D6, CYP4F2, SLCO1B1 and UGT1A1). The most distinctive difference was observed for SLCO1B1 star alleles showing lower frequencies in the Serbian population (SLCO1B1*5 p < 2.2*10−16, SLCO1B1*20 p = 0.006, SLCO1B1*37 p < 2.2*10−16).
Star alleles which are considered Level 1 and Level 2 by PharmGKB or level A and B on CPIC and had differing frequencies in Serbian versus other populations are listed in Table 4. Star alleles in level 1/2 and A/B pharmacogenes that had frequencies >1% in the Serbian population and >1% in European and worldwide populations are shown in Figure 1. A complete list of variants with differing frequencies and p values can be found in Supplementary Data Sheets 2, 3, 4, 5.
Table 4. Star alleles with significantly different frequencies in Serbian compared to worldwide or European populations. Pharmacogenes with the clinical annotation level of evidence 1 and 2 (PharmGKB) or level A and B (CPIC) were included.
Figure 1. A and B level PGx variants that were detected in the SRB—Serbian population, EUR–European population and WRL–worldwide population.
Population similarity between Serbian, worldwide and European populations was further expressed through calculating the Wright’s fixation index (Fst). Values of Fst can range between 0 and 1, with higher values indicating a higher differentiation among populations. Overall, population differentiation was not particularly high for any of the analyzed pharmacogenes (Figure 2), with the highest Fst occurring between the Serbian and the worldwide population for CYP2A6 (Fst = 0.31). As expected, the Serbian population was more similar to the European than to the worldwide population (mean Fst between worldwide and Serbian populations = 0.052, mean Fst between European and Serbian populations = 0.047). On average, the lowest Fst was found between the Serbian and the Croatian population (p = 0.007).
Figure 2. A heatmap representing Wright’s fixation index (Fst). Higher Fst values indicate higher degree of population stratification. NA–no data was available for that pharmacogene in the Croatian population. SRB-ALL–Fst between Serbian and worldwide populations. SRB-EUR–Fst between Serbian and European populations. SRB-CRO–Fst between Serbian and Croatian populations. Star allele frequencies in worldwide and European populations were gathered from CPIC or gnomAD databases, while for Croatia, frequencies were extracted from previously published data (Matišić et al., 2023).
Phenotype categories associated with drug categories according to CPIC (level A and B) or PharmGKB (level 1 and 2) were available for 16 out of 52 analyzed pharmacogenes (Table 5). All detected diplotypes alongside predicted phenotypes are listed in Supplementary Data Sheets 7, 8. In accordance with European frequencies, CYP3A5 poor metabolizers represented 85.8% of the Serbian population, while the rest of tested subjects were intermediate metabolizers (Table 5). Even though carriers of *3/*3 diplotype are characterized as poor metabolizers, they require the standard recommended starting dose, while *1/*1 and *1/*3 (normal and intermediate metabolizer) would require a higher recommended starting dose. The highest prevalence of decreased enzymatic function was found in NAT2, with 94.6% of the Serbian population harboring damaging star alleles. The highest percentage of ultrarapid metabolizers was predicted for the CYP1A2 pharmacogene (84.1%).
Comparison of predicted phenotype frequencies between the Serbian and European subpopulations from the 1000 Genomes Project (Central European–CEU, Finnish–FIN, Great Britain–GBR, Tuscans from Italy–TSI and Iberian Spanish–IBS) (Sherman, Claw, and Lee, 2024) was performed for 12 pharmacogenes (ABCG2, CYP2B6, CYP2C19, CYP2C9, CYP2D6, CYP3A5, DPYD, IFNL3, NUDT15, SLCO1B1, TPMT and UGT1A1) and for 10 pharmacogenes in the Croatian population (CYP2B6, CYP2C19, CYP2C9, CYP2D6, CYP3A5, DPYD, NUDT15, SLCO1B1, TPMT and UGT1A1) (Supplementary Data Sheet 9). Bonferroni correction for multiple comparison was applied by dividing 0.05 with 12 or 10 for comparisons with European subpopulations or the Croatian population (number of analyzed pharmacogenes) and differences between populations were considered significant when p value was <0.004 or <0.005 respectively. Significant differences between the Serbian and European subpopulations were noted in phenotype distribution for ABCG2, CYP2B6 and SLCO1B1 pharmacogenes (Figure 3). The Serbian population had a higher prevalence of decreased function status for the ABCG2 pharmacogene compared to Central European and Tuscans from Italy (CEU p = 9.1*10−7, TSI p = 3.2*10−7). European subpopulations had higher frequencies of intermediate metabolizers in CYP2B6 compared to the Serbian population (CEU p = 9.9*10−11, FIN p = 1.6*10−6, GBR p = 1.9*10−7, IBS p = 6.5*10−6, TSI p = 8.1*10−10). Increased enzymatic activity of SLCO1B1 was higher in all European subpopulations except in Finnish compared to the Serbian population (CEU p = 4.6*10−13, GBR p = 4.0*10−10, IBS p = 6.2*10−14, TSI p = 1.4*10−11). Significant differences between the Serbian and the Croatian population were noted for poor metabolizers in CYP2D6, which were more frequent in the Croatian population (p = 0.003) and for poor and rapid metabolizers in SLCO1B1, with the Serbian population having higher frequencies of both poor and rapid metabolizers (poor metabolizers p = 0.0008, rapid metabolizers p = 8.9*10−5). Other pharmacogenes that were analyzed in this part of the study had phenotype frequencies in accordance with European subpopulations and the Croatian population.
Figure 3. Pharmacogenetic phenotypes in SRB–Serbian, CRO–Croatian, CEU–Central European, FIN–Finnish, GBR–Great Britain, IBS–Iberian (from Spain) and TSI–Tuscan (from Italy) populations. Significance was calculated using Chi squared and Fisher’s Exact tests. After Bonferroni correction for multiple testing, p < 0.004 (SRB-EUR) and p < 0.005 (SRB-CRO) were considered significant.
Fast development of NGS technologies revolutionized genomic research and clinical diagnostics. Efforts for implementing NGS into therapy optimization are noted globally, with many working groups gathering to upgrade pharmacogenomics recommendations for drug usage (CPIC, DPWG). This study presents the first comprehensive analysis of star allele frequencies in more than 50 pharmacogenes in the Serbian population from a large group of subjects previously analyzed using NGS, enhancing the knowledge related to potentially important and population specific PGx variants that could be considered as markers for preemptive and predictive PGx testing in the Serbian population.
One of the objectives of this study was to determine whether clinical exome sequencing (CES) and whole exome sequencing (WES) data could be effectively utilized for pharmacogenomics profiling. For 29 analyzed pharmacogenes, variants were successfully annotated regardless of which panel was used for sequencing, indicating applicability of both CES and WES technologies in pharmacogenomic testing. Out of those 29 pharmacogenes, 7 are included in the CPIC guidelines for drug dosing (i.e., ABCG2, SLCO1B1, CYP2C8, CYP2C9, G6PD, RYR1 and TPMT). This could be important since CES is a more cost-effective alternative to WES for comprehensive genomic testing and could be considered a reliable approach for extensive pharmacogenetic analysis. However, when it comes to overall PGx profiling, WES proved to be more informative, additionally covering all relevant pharmacogenes and PGx variants (CYP2C19*17, CYP3A5*3, NUDT15, UGT1A1 TATA box, VKORC1). Nevertheless, for detecting complex structural rearrangements, whole genome sequencing (WGS) is the method of choice. This is particularly important for genotyping of CYP2D6 – in our study, we identified only single nucleotide variants and small indels, even though it would be expected to find at least a few copy number variants in our sample of several hundred individuals. Therefore, we conclude that WGS should be required for precise profiling of CYP2D6. Nonetheless, we showed that employing CES and WES to clinical pharmacogenomics is feasible for many pharmacogenes, especially for those included in published guidelines for drug dose individualization according to patient’s genotype.
Besides testing usability of the applied NGS platforms, we also tested performance of the two most commonly employed tools for annotating pharmacogenomic variants, PharmCAT and Stagazer. Calling of star alleles with both PharmCAT and Stargazer was performed for 18 pharmacogenes. We noticed discordant results in almost half of the analyzed pharmacogenes between these two tools. Discrepancies were mostly related to the possibly outdated or incomplete data of relevant pharmacogenetic variants in Stargazer (particularly variants in RYR1 and variant 711 + 3A>G in CFTR) or PharmCAT (variants in DPYD). Furthermore, inconsistencies between tools were found in the annotation of pharmacogenes that harbored complex haplotypes, where haplotype phasing is dubious without case-parent triads. Since we used unphased genomic data of unrelated individuals, retained diplotypes were the ones that were most probable based on frequencies in the European population. Overall, we determined that using more than one annotation tool for PGx studies is indeed adequate, with additional manual inspection and rigorous quality control to resolve discrepancies between the outputs of different tools.
We further analyzed distribution of pharmacogenetic variants in the Serbian population and compared it to other populations. The most important pharmacogenes detected in the Serbian population, that harbored actionable variants with notable frequency and are included in the CPIC guidelines were CYP2C9, NAT2, SLCO1B1, UGT1A1 and VKORC1. These pharmacogenes could be considered for inclusion into genetic reports, since they affect response to many drugs (anticoagulants, antituberculosis agents, statins, immunosuppresive and anticancer drugs). Significant differences in star allele frequencies were identified in 31, 29, and 4 pharmacogenes when compared to worldwide, European and Croatian populations, respectively. Most of these differences were observed in pharmacogenes with low levels of PGx evidence. However, 12 pharmacogenes showing differences between the Serbian population and worldwide or European populations are classified as Level A or B according to the CPIC guidelines. These include CFTR, CYP2C9, CYP2C19, CYP2D6, CYP4F2, G6PD, NAT2, RYR1, SLCO1B1, TPMT, UGT1A1 and VKORC1. Compared to the worldwide population, the Serbian population exhibits significantly higher frequencies of specific A or B level star alleles (CYP2D6*35, RYR1 c.4178A>G, RYR1 c.10042C>T) as well as lower frequencies of others (CYP2C19*1, G6PD A, NAT2*12, NAT2*13, RYR1 c.10747G>C, SLCO1B1*37). Similar trends were observed when compared with the European population, except that the SLCO1B1*37 variant was additionally found as more frequent, while variants CYP2C19*1, NAT2*12, NAT2*13, RYR1 c.10747G>C were found as less frequent in the Serbian population. These alleles determine the normal or uncertain function of the encoded proteins. Star alleles that influence PGx phenotype and have a higher frequency in the Serbian population compared to other Europeans are NAT2*7, SLCO1B1*5, UGT1A1*36 and VKORC1 -1639A, while UGT1A1*28 had a lower frequency in the Serbian population.
One of the variants that had significantly higher frequency in the Serbian population compared to both worldwide and European population was 711 + 3A>G in CFTR gene. Since genomic data used in our study was from individuals subjected to exome sequencing for diagnostic purposes, we cannot exclude a possibility that in our group some individuals had a diagnosis of cystic fibrosis–hence the higher frequency of a pathogenic variant in our sample.
Moreover, our study showed a significantly higher frequency of VKORC1 -1693A in the Serbian population compared to worldwide and European populations, which is in alignment with previously published data (Kovac et al., 2010). Furthermore, previous results of higher incidence of CYP2C9*3 in the Serbian population (Mizzi et al., 2016), differs from our findings. Said star allele had a frequency in alignment with the European population. As for thiopurine drugs, association studies of drug response and PGx variants were previously conducted on pediatric patients with acute lymphoblastic leukemia and adult patients with inflammatory bowel disease in Serbia. In these studies, frequencies of PGx variants in TPMT (Dokmanovic et al., 2006; Jojic et al., 2003), TYMS, SLC19A11, DHFR, ITPA, ABCC4 and ABCB1 (Milosevic et al., 2018) were reported. Adding to variants TPMT*2, TPMT*3A and TPMT*3C that were previously reported in the Serbian population, this study detected three more star alleles in TPMT – *9, *20 and *43. Frequency of SLCO1B1*5 was previously reported in the Serbian population as well (Kotur et al., 2020). Notably, previous studies report SLCO1B1*5, associated with response to methotrexate, as more frequent than in our results. We detected SLCO1B1*5 as a singular variant and as a part of a haplotype SLCO1B1*15. If we were to combine the frequencies of the variant and the haplotype it constitutes, SLCO1B1*5 would have a similar frequency to the one previously reported. Previous studies had limited samples and used PCR-based genotyping followed with Sanger sequencing or genotyping arrays (Kovac et al., 2010; Mizzi et al., 2016; Kotur et al., 2020; Dokmanovic et al., 2006; Milosevic et al., 2018). Even though most of these studies were conducted on patients with different diagnoses, variants in pharmacogenes are not considered disease causing, hence these results could reflect the genuine PGx profile of the Serbian population. Comparison of our results with previous findings outlines the need for PGx testing prior to the introduction of treatment to different diseases.
In pharmacogenes CACNA1S, CYP17A1 and CYP2R1 only reference alleles were found in the Serbian population. This finding was as expected, since alternative alleles present in position files for star allele calling with Stargazer and PharmCAT are considered rare in the rest of the world as well.
Furthermore, an analysis of population differentiation through Wright’s fixation index calculation showed that the Serbian population exhibited the greatest dissimilarity from the global population, particularly for CYP2A6 (Fst = 0.31). As anticipated, the Serbian and the Croatian population showed the highest level of similarity.
Due to availability of data and geographical proximity in the Western Balkan region, the Croatian population was included in this study for fine-tuning comparison. Population data was also published for the Slovenian population (Hočevar, Maver, and Peterlin, 2019). Owing to different statistical approaches used in our and Slovenian group, further comparisons were not anaysed between the two populations. Frequencies of most of the analyzed star alleles were concordant between two populations. This finding is further corroborated with Wright’s fixation index calculation that showed high genetic similarity between Serbian and Croatian population. The highest difference was found for SLCO1B1*5, SLCO1B1*37 and CYP4F2*3 which were more frequent in the Croatian population. We cannot exclude that this discrepancy might be due to different methodological approaches in the two compared studies–we used clinical and whole exome sequencing, a methodology that covers wider regions of the genome. The group from Croatia used a targeted panel that covers only the most important star alleles, thus other alternative alleles might have been missed in their sample. Moreover, higher frequency in the Croatian population was similar as previously reported for the Serbian population for UGT1A1*28 star allele (Vukovic et al., 2018). The discrepancy that was presented herein is probably due to a different methodological approach–in our study we detected UGT1A1*28 in two forms, as an individual variant and as part of a haplotype (UGT1A1*80+*28). By merging frequencies of UGT1A1*28 and UGT1A1*80+*28, the frequency of UGT1A1*28 would be similar to previously reported data in the Serbian and in the Croatian population.
A significant part of our study represents analysis of PGx phenotypes related to several drug categories in the Serbian population. Many of these phenotypes determine protein function, but there is still a lack of evidence as to how changes in protein structure affect drug metabolism. Therefore, further comparisons between Serbian and other European subpopulations were conducted only for pharmacogenes included in guidelines published by relevant consortia, such as CPIC. We found that a significant portion of the Serbian population (94.5%) carries variants in NAT2 (*5, *6 and *7) that induce decreased enzymatic activity and could contribute to drug induced liver injury in patients treated with isoniazid (Ng et al., 2014). Significant discrepancy was also found in star alleles influencing response to statin therapy, namely, in phenotypes determined by ABCG2 and SLCO1B1. We found that ABCG2 intermediate statin metabolizers were more frequent in the Serbian population than in Central European and Tuscans. In contrast, SLCO1B1 rapid metabolizers were less frequent in the Serbian population. Hepatic uptake of statins is regulated via a transporter encoded by SLCO1B1, while absorption and disposition are modified by a ABCG2-encoded efflux transporter (Rhonda et al., 2022). Even though SLCO1B1 rapid metabolizers were less frequent in the Serbian population compared to European populations, this does not imply that testing for SLCO1B1 irregular responders would be superfluous. Intermediate and poor responders were as expected compared with other Europeans, however, they represented over 20% of our sample. Furthermore, in the Serbian population, CYP2B6 intermediate metabolizers, characterized by reduced enzymatic activity, were more frequent compared to all other analysed subpopulations. Several drugs from the antidepressant category, such as anxiolytics, are thought to be influenced by CYP2B6 phenotype (Radosavljevic et al., 2023). Such findings in our population indicate that the Serbian population may benefit from the introduction of genotyping of patients for variants in NAT2, ABCG2, SLCO1B1 and CYP2B6 prior to administration of antituberculosis agents, statins and antidepressants due to differences that were presented herein.
The main limitation of our study was the number of samples sequenced with clinical exome compared to the whole exome panel. For most of the analyzed pharmacogenes, WES proved to be more efficient, due to the inclusion of many important PGx variants in splicing regions and exons that are not covered by the CES panel. Even though WES provided us with a large number of variants, for pharmacogenomics studies, whole genome sequencing would be even more informative. For example, we detected many star alleles in CYP2D6, but we were not able to detect any copy number variants. Moreover, tools for annotation of star alleles that we used in our study do not annotate a highly polymorphic HLA gene, which plays an important role in response to antiepileptic and antiretroviral therapeutics. Another limitation of our study was that data was de-identified and any tracking of diagnosis, congenital anomaly or therapy response was unfeasible. Furthermore, several databases were used for allele frequency comparisons and Wright’s fixation index calculation. Ideally, all data would be compared to one database with matched sample sizes, however, no such database exists to the best of our knowledge. Frequencies of PGx variants can be found in PharmGKB, but originate from several databases: CPIC, All of US, UK Biobank, gnomAD, 1000 Genomes Project. Frequencies throughout databases should not differ significantly, however, we cannot exclude that the use of several sources affected results presented in our study. An important limitation in population genomic studies is the presence of biases in worldwide datasets, which can significantly influence comparisons using Wright’s fixation index (Fst). The 1000 Genomes project and gnomAD data are skewed towards European ancestry with many global populations still underrepresented. Also, sample size disparities introduce higher variance in allele frequency estimates; if one population has large sample size while other have small, Fst values could be misleading. Furthermore, many datasets lump genetically distinct subpopulations into broad categories and if these subpopulations are not equally represented, intra-population heterogeneity can reduce observed Fst values, masking true differentiation. Therefore, these limitations should be carefully considered when interpreting population differences, particularly when making comparisons against broad, worldwide datasets.
In conclusion, this study represents an in-depth analysis of the pharmacogenomics landscape of the Serbian population and our sample constitutes the largest group of individuals ever analyzed in a PGx study in Serbia. We used data from several databases (CPIC, 1,000 Genome Project, gnomAD, published studies) to compare our findings to other populations. Moreover, we summarized the PGx phenotype distinctions of our population considering different therapeutics that are used in Serbia as well. Our findings reveal interethnic heterogeneity in key pharmacogenes, such as NAT2, SLCO1B1, UGT1A1 and VKORC1 and offer valuable insights into the applicability of various sequencing technologies and variant annotation tools in PGx analysis. We showed that results often depend on used methodology and annotation tools. Therefore, careful selection of pharmacogenes, star alleles, sequencing panels and annotation tools is advised for PGx implementation. Furthermore, the conducted study is the representation of PGx phenotypes specific to the Serbian population, supporting the integration of relevant pharmacogenomic information into genetic reports tailored for this population.
The original contributions presented in the study are included in the article/Supplementary Material, further inquiries can be directed to the corresponding author.
The studies involving humans were approved by the Ethics Committee of the Institute of Molecular Genetics and Genetic Engineering University of Belgrade. The studies were conducted in accordance with the local legislation and institutional requirements. Written informed consent for participation was not required from the participants or the participants’ legal guardians/next of kin in accordance with the national legislation and institutional requirements.
MJ: Conceptualization, Data curation, Formal Analysis, Investigation, Methodology, Visualization, Writing–original draft. DP: Conceptualization, Data curation, Formal Analysis, Methodology, Writing–original draft. BS: Conceptualization, Data curation, Formal Analysis, Methodology, Writing–original draft. NK: Conceptualization, Data curation, Formal Analysis, Methodology, Writing–original draft. BR: Formal Analysis, Investigation, Resources, Writing–original draft. SP: Conceptualization, Funding acquisition, Resources, Supervision, Writing–review and editing. BZ: Conceptualization, Funding acquisition, Project administration, Supervision, Writing–review and editing.
The author(s) declare that financial support was received for the research, authorship, and/or publication of this article. This study was funded by the Horizon Europe PharmGenHUB Project (HORIZON-WIDERA-2021-ACCESS-02, European Commission, Grant Agreement No. 101059870).
The authors declare that the research was conducted in the absence of any commercial or financial relationships that could be construed as a potential conflict of interest.
The author(s) declare that no Generative AI was used in the creation of this manuscript.
All claims expressed in this article are solely those of the authors and do not necessarily represent those of their affiliated organizations, or those of the publisher, the editors and the reviewers. Any product that may be evaluated in this article, or claim that may be made by its manufacturer, is not guaranteed or endorsed by the publisher.
The Supplementary Material for this article can be found online at: https://www.frontiersin.org/articles/10.3389/fphar.2025.1553536/full#supplementary-material
Andjelkovic, M., Klaassen, K., Skakic, A., Marjanovic, I., Kravljanac, R., Djordjevic, M., et al. (2024). Characterization of 13 novel genetic variants in genes associated with epilepsy: implications for targeted therapeutic strategies. Mol. Diagnosis and Ther. 28 (5), 645–663. doi:10.1007/s40291-024-00720-2
Chen, S., Francioli, L. C., Goodrich, J. K., Collins, R. L., Kanai, M., Wang, Q., et al. (2024). A genomic mutational constraint map using variation in 76,156 human genomes. Nature 625 (7993), 92–100. doi:10.1038/s41586-023-06045-0
Dokmanovic, L., Urosevic, J., Janic, D., Jovanovic, N., Petrucev, B., Tosic, N., et al. (2006). Analysis of thiopurine S-methyltransferase polymorphism in the population of Serbia and Montenegro and mercaptopurine therapy tolerance in childhood acute lymphoblastic leukemia. Ther. Drug Monit. 28 (6), 800–806. doi:10.1097/01.ftd.0000249947.17676.92
Drljaca, T., Zukic, B., Kovacevic, V., Gemovic, B., Klaassen-Ljubicic, K., Perovic, V., et al. (2021). The first insight into the genetic structure of the population of modern Serbia. Sci. Rep. 11 (1), 13995. doi:10.1038/s41598-021-93129-4
Gemovic, B., and Veljkovic, N. (2023). The genomic ecosystem of Serbia situation analysis. Centre Fourth Industrial Revolut. Serbia. Available at: https://c4ir.rs/wp-content/uploads/2023/06/ENG_Genomic-Ecosystem-of-Serbia_FINAL-1.pdf.
Graffelman, J. (2015). Exploring diallelic genetic markers: the HardyWeinberg package. J. Stat. Softw. 64 (3). doi:10.18637/jss.v064.i03
Hočevar, K., Maver, A., and Peterlin, B. (2019). Actionable pharmacogenetic variation in the slovenian genomic database. Front. Pharmacol. 10 (March), 240. doi:10.3389/fphar.2019.00240
Jančić, I., Arsenović-Ranin, N., Šefik-Bukilica, M., Živojinović, S., Damjanov, N., Spasovski, V., et al. (2013). 174G/C interleukin-6 gene promoter polymorphism predicts therapeutic response to etanercept in rheumatoid arthritis. Rheumatol. Int. 33 (6), 1481–1486. doi:10.1007/s00296-012-2586-y
Jančić, I., Šefik-Bukilica, M., Živojinović, S., Damjanov, N., Spasovski, V., Kotur, N., et al. (2015). Influence of promoter polymorphisms of the tnf-α (-308g/A) and IL-6 (-174g/C) genes on therapeutic response to etanercept in rheumatoid arthritis. J. Med. Biochem. 34 (4), 414–421. doi:10.2478/jomb-2014-0060
Ji, Y., Yue, S., McMillin, G. A., and Lyon, E. (2018). Clinical pharmacogenomics testing in the era of next generation sequencing: challenges and opportunities for precision medicine. Expert Rev. Mol. Diagnostics 18 (5), 411–421. doi:10.1080/14737159.2018.1461561
Jmel, H., Sarno, S., Giuliani, C., Boukhalfa, W., Abdelhak, S., Luiselli, D., et al. (2024). Genetic diversity of variants involved in drug response among Tunisian and Italian populations toward personalized medicine. Sci. Rep. 14 (1), 5842. doi:10.1038/s41598-024-55239-7
Jojic, N., Urosevic, J., Bojic, B., and Pavlovic, S. (2003). Determination of thiopurine methyltransferase genotype in the patients with inflammatory bowel disease before and during azathioprine therapy. Archives Gastroenterohepatol. 22 (1–2), 5–9.
Kabbani, D., Akika, R., Ahmed, W., Daly, A. K., Cascorbi, I., and Zgheib, N. K. (2023). Pharmacogenomics in practice: a review and implementation guide. Front. Pharmacol. 14 (May), 1189976. doi:10.3389/fphar.2023.1189976
Karamperis, K., Katz, S., Melograna, F., Ganau, F. P., Van Steen, K., Patrinos, G. P., et al. (2024). Genetic ancestry in population pharmacogenomics unravels distinct geographical patterns related to drug toxicity. iScience 27 (10), 110916. doi:10.1016/j.isci.2024.110916
Kotur, N., Lazic, J., Ristivojevic, B., Stankovic, B., Gasic, V., Dokmanovic, L., et al. (2020). Pharmacogenomic markers of methotrexate response in the consolidation phase of pediatric acute lymphoblastic leukemia treatment. Genes 11 (4), 468. doi:10.3390/genes11040468
Kovac, M. K., Maslac, A. R., Rakicevic, L. B., and Radojkovic, D. P. (2010). The c.-1639g>A polymorphism of the VKORC1 gene in Serbian population: retrospective study of the variability in response to oral anticoagulant therapy. Blood Coagulation and Fibrinolysis 21 (6), 558–563. doi:10.1097/MBC.0b013e32833c2988
Lee, S.-been, Wheeler, M. M., Thummel, K. E., and Nickerson, D. A. (2019). Calling star alleles with stargazer in 28 pharmacogenes with whole genome sequences. Clin. Pharmacol. and Ther. 106 (6), 1328–1337. doi:10.1002/cpt.1552
Machiela, M. J., and Chanock, S. J. (2015). LDlink: a web-based application for exploring population-specific haplotype structure and linking correlated alleles of possible functional variants. Bioinformatics 31 (21), 3555–3557. doi:10.1093/bioinformatics/btv402
Matišić, V., Brlek, P., Bulić, L., Molnar, V., Dasović, M., and Primorac, D. (2023). Population pharmacogenomics in Croatia: evaluating the PGx allele frequency and the impact of treatment efficiency. Int. J. Mol. Sci. 24 (17), 13498. doi:10.3390/ijms241713498
Milosevic, G., Kotur, N., Krstovski, N., Lazic, J., Zukic, B., Stankovic, B., et al. (2018). Variants in TPMT, ITPA, ABCC4 and ABCB1 genes as predictors of 6-mercaptopurine induced toxicity in children with acute lymphoblastic leukemia. J. Med. Biochem. 37 (3), 320–327. doi:10.1515/jomb-2017-0060
Mizzi, C., Dalabira, E., Kumuthini, J., Dzimiri, N., Balogh, I., Başak, N., et al. (2016). A European spectrum of pharmacogenomic biomarkers: implications for clinical pharmacogenomics. PLOS ONE 11 (9), e0162866. doi:10.1371/journal.pone.0162866
Morash, M., Mitchell, H., Beltran, H., Elemento, O., and Pathak, J. (2018). The role of next-generation sequencing in precision medicine: a review of outcomes in oncology. J. Personalized Med. 8 (3), 30. doi:10.3390/jpm8030030
Ng, C.-S., Hasnat, A., Maruf, A. A., Ahmed, M. U., Pirmohamed, M., Day, C. P., et al. (2014). N-acetyltransferase 2 (NAT2) genotype as a risk factor for development of drug-induced liver injury relating to antituberculosis drug treatment in a mixed-ethnicity patient group. Eur. J. Clin. Pharmacol. 70 (9), 1079–1086. doi:10.1007/s00228-014-1703-0
Ng, D., Hong, C. S., Singh, L. N., Johnston, J. J., Mullikin, J. C., and Biesecker, L. G. (2017). Assessing the capability of massively parallel sequencing for opportunistic pharmacogenetic screening. Genet. Med. 19 (3), 357–361. doi:10.1038/gim.2016.105
Nunez-Torres, R., Pita, G., Peña-Chilet, M., López-López, D., Zamora, J., Roldán, G., et al. (2023). A comprehensive analysis of 21 actionable pharmacogenes in the Spanish population: from genetic characterisation to clinical impact. Pharmaceutics 15 (4), 1286. doi:10.3390/pharmaceutics15041286
Paschou, P., Drineas, P., Yannaki, E., Razou, A., Kanaki, K., Tsetsos, F., et al. (2014). Maritime route of colonization of Europe. Proc. Natl. Acad. Sci. 111 (25), 9211–9216. doi:10.1073/pnas.1320811111
Perović, S., Vujović, S., Perović, S., and Šćepanović, A. (2024). The role of CYP2C19*2 variant and other factors in clopidogrel resistance in Montenegrin ACS patients. Genet. and Appl. 8 (2). doi:10.31383/ga.vol8iss2ga03
Poplin, R., Ruano-Rubio, V., DePristo, M. A., Fennell, T. J., Carneiro, M. O., Van der Auwera, G. A., et al. (2017). “Scaling accurate genetic variant discovery to tens of thousands of samples.” doi:10.1101/201178
Privé, F., Aschard, H., Ziyatdinov, A., and Blum, M. G. B. (2018). Efficient analysis of large-scale genome-wide data with two R packages: bigstatsr and bigsnpr. Bioinformatics 34 (16), 2781–2787. doi:10.1093/bioinformatics/bty185
Proietti, R., Maranho Neto, G. A., Kunzova, S., Re, O. L., Ahola-Olli, A., Heliste, J., et al. (2023). “Pharmacogenomic profile of a central European urban random population-Czech population. PLOS ONE 18 (4), e0284386. doi:10.1371/journal.pone.0284386
Radosavljevic, M., Strac, D. S., Jancic, J., and Samardzic, J. (2023). The role of pharmacogenetics in personalizing the antidepressant and anxiolytic therapy. Genes 14 (5), 1095. doi:10.3390/genes14051095
Rhonda, M., Niemi, M., Ramsey, L. B., Luzum, J. A., Katriina Tarkiainen, E., Straka, R. J., et al. (2022). The clinical pharmacogenetics implementation Consortium guideline for SLCO1B1, ABCG2, and CYP2C9 genotypes and statin-associated musculoskeletal symptoms. Clin. Pharmacol. and Ther. 111 (5), 1007–1021. doi:10.1002/cpt.2557
Russell, L. E., Zhou, Y., Almousa, A. A., Sodhi, J. K., Nwabufo, C. K., and Lauschke, V. M. (2021). Pharmacogenomics in the era of next generation sequencing – from byte to bedside. Drug Metab. Rev. 53 (2), 253–278. doi:10.1080/03602532.2021.1909613
Sangkuhl, K., Carrillo, M. W., Whaley, R. M., Woon, M., Lavertu, A., Altman, R. B., et al. (2020). Pharmacogenomics clinical annotation tool (pharm CAT). Clin. Pharmacol. and Ther. 107 (1), 203–210. doi:10.1002/cpt.1568
Sherman, C. A., Claw, K. G., and Lee, S.-been (2024). Pharmacogenetic analysis of structural variation in the 1000 genomes project using whole genome sequences. Sci. Rep. 14 (1), 22774. doi:10.1038/s41598-024-73748-3
Stankovic, B., Dragasevic, S., Klaassen, K., Kotur, N., Drazilov, S. S., Zukic, B., et al. (2020). Exploring inflammatory and apoptotic signatures in distinct crohn’s disease phenotypes: way towards molecular stratification of patients and targeted therapy. Pathology - Res. Pract. 216 (6), 152945. doi:10.1016/j.prp.2020.152945
Tafazoli, A., Guchelaar, H.-J., Miltyk, W., Kretowski, A. J., and Swen, J. J. (2021). Applying next-generation sequencing platforms for pharmacogenomic testing in clinical practice. Front. Pharmacol. 12 (August), 693453. doi:10.3389/fphar.2021.693453
Velizarova, M., Abedinov, P., Svinarov, D., Nikolov, V., and Hristova, J. (2022). Frequency distribution of CYP2C9 and VKORC1 mutations among Bulgarian patients and their importance for anticoagulant therapy. Clin. Lab. 68 (12/2022). doi:10.7754/Clin.Lab.2022.220213
Vukovic, M., Radlovic, N., Lekovic, Z., Vucicevic, K., Maric, N., Kotur, N., et al. (2018). UGT1A1 (TA)n promoter genotype: diagnostic and population pharmacogenetic marker in Serbia. Balkan J. Med. Genet. 21 (1), 59–68. doi:10.2478/bjmg-2018-0012
Wright, G. E. B., Carleton, B., Hayden, M. R., and Ross, C. J. D. (2018). The global spectrum of protein-coding pharmacogenomic diversity. Pharmacogenomics J. 18 (1), 187–195. doi:10.1038/tpj.2016.77
Zhang, B., and Lauschke, V. M. (2019). Genetic variability and population diversity of the human SLCO (OATP) transporter family. Pharmacol. Res. 139 (January), 550–559. doi:10.1016/j.phrs.2018.10.017
Keywords: pharmacogenomics, bioinformatics, population genetics, personalized medicine, high-throughput sequencing
Citation: Jelovac M, Pavlovic D, Stankovic B, Kotur N, Ristivojevic B, Pavlovic S and Zukic B (2025) Comprehensive pharmacogenomics profiling of the Serbian population. Front. Pharmacol. 16:1553536. doi: 10.3389/fphar.2025.1553536
Received: 30 December 2024; Accepted: 18 February 2025;
Published: 17 March 2025.
Edited by:
Eva Döring-Brandl, Charité University Medicine Berlin, GermanyReviewed by:
Theodora Katsila, National Hellenic Research Foundation, GreeceCopyright © 2025 Jelovac, Pavlovic, Stankovic, Kotur, Ristivojevic, Pavlovic and Zukic. This is an open-access article distributed under the terms of the Creative Commons Attribution License (CC BY). The use, distribution or reproduction in other forums is permitted, provided the original author(s) and the copyright owner(s) are credited and that the original publication in this journal is cited, in accordance with accepted academic practice. No use, distribution or reproduction is permitted which does not comply with these terms.
*Correspondence: Branka Zukic, YnJhbmthLnp1a2ljQGltZ2dlLmJnLmFjLnJz
Disclaimer: All claims expressed in this article are solely those of the authors and do not necessarily represent those of their affiliated organizations, or those of the publisher, the editors and the reviewers. Any product that may be evaluated in this article or claim that may be made by its manufacturer is not guaranteed or endorsed by the publisher.
Research integrity at Frontiers
Learn more about the work of our research integrity team to safeguard the quality of each article we publish.