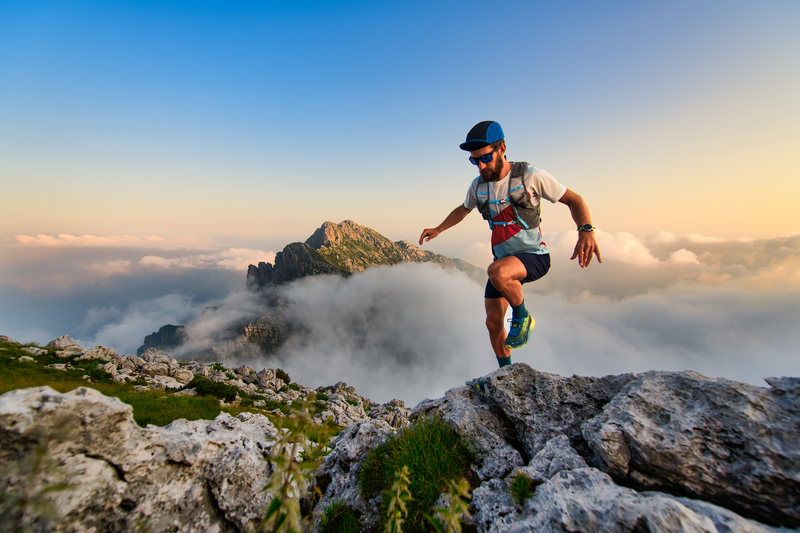
95% of researchers rate our articles as excellent or good
Learn more about the work of our research integrity team to safeguard the quality of each article we publish.
Find out more
ORIGINAL RESEARCH article
Front. Pharmacol.
Sec. Neuropharmacology
Volume 16 - 2025 | doi: 10.3389/fphar.2025.1539032
The final, formatted version of the article will be published soon.
You have multiple emails registered with Frontiers:
Please enter your email address:
If you already have an account, please login
You don't have a Frontiers account ? You can register here
Parkinson's disease (PD), a prevalent and progressive neurodegenerative disorder, currently lacks effective and satisfactory pharmacological treatments.Computational drug repurposing represents a promising and efficient strategy for drug discovery, aiming to identify new therapeutic indications for existing pharmaceuticals.In this study, we employed a drug-target network approach to identify potential therapeutic candidates for Parkinson's disease by computationally repurposing FDAapproved drugs from databases such as DrugBank. This approach yielded a total of 176 drug candidates. A comprehensive review of existing literature was conducted to identify drug candidates, resulting in the selection of 28 compounds not previously reported as pharmacoprotective against Parkinson's disease, yet possessing potential anti-Parkinsonian properties. Subsequent evaluation using Cell Counting Kit-8 (CCK8) assays conclusively demonstrated that Omaveloxolone and Cyproheptadine exerted significant neuroprotective effects in the SH-SY5Y cell model of Parkinson's disease induced by 1-Methyl-4-phenylpyridinium (MPP+). Through the development of a mouse model of Parkinson's disease induced by 1-methyl-4-phenyl-1,2,3,6tetrahydropyridine (MPTP), our study demonstrated that Cyproheptadine effectively inhibited interleukin-6 (IL-6) expression and prevented the downregulation of Tyrosine Hydroxylase (TH) via the MAPK/NFκB signaling pathway. Concurrently, Omaveloxolone alleviates the downregulation of TH, which may be mediated by the activation of the Kelch-like ECH-associated protein 1 (KEAP1)-NF-E2-related factor 2 (Nrf2)/antioxidant response element (ARE) signaling pathway. Both pharmacological agents contributed to the preservation of dopaminergic neurons and amelioration of neurological deficits within the Parkinson's disease model. In conclusion, this study elucidates potential drug candidates for the treatment of Parkinson's disease through the application of computational repurposing, thereby underscoring its efficacy as a drug discovery strategy.
Keywords: Computational repurposing, Parkinson's disease, Omaveloxolone, Cyproheptadine, MAPK/NF κ B signaling pathway, Keap1-Nrf2/ARE signaling pathway
Received: 03 Dec 2024; Accepted: 28 Mar 2025.
Copyright: © 2025 Guo, Wang, Fan, Tao, Ren, Li, Liu, Zhou and Chen. This is an open-access article distributed under the terms of the Creative Commons Attribution License (CC BY). The use, distribution or reproduction in other forums is permitted, provided the original author(s) or licensor are credited and that the original publication in this journal is cited, in accordance with accepted academic practice. No use, distribution or reproduction is permitted which does not comply with these terms.
* Correspondence:
YingZhu Chen, Medical College, Yangzhou University, Yangzhou, 225001, Jiangsu Province, China
Disclaimer: All claims expressed in this article are solely those of the authors and do not necessarily represent those of their affiliated organizations, or those of the publisher, the editors and the reviewers. Any product that may be evaluated in this article or claim that may be made by its manufacturer is not guaranteed or endorsed by the publisher.
Research integrity at Frontiers
Learn more about the work of our research integrity team to safeguard the quality of each article we publish.