- 1Laboratory of Pharmacogenomics and Individualized Therapy, Division of Pharmacology and Biosciences, Department of Pharmacy, School of Health Sciences, University of Patras, Patras, Greece
- 2Department of Informatics, Ionian University, Corfu, Greece
- 3Molecular Diagnostics Laboratory, INRASTES, National Centre for Scientific Research “Demokritos”, Athens, Greece
- 4Department of Nephrology and Kidney Transplantation, University Hospital of Patras, Patras, Greece
- 5ANALYSI Biomedical Laboratories S.A., Thessaloniki, Greece
- 6Department of Genetics and Genomics, College of Medicine and Health Sciences, United Arab Emirates University, Al-Ain, United Arab Emirates
- 7ASPIRE Abu Dhabi Precision Medicine Research Institute, Al-Ain, United Arab Emirates
- 8Zayed Center for Health Sciences, United Arab Emirates University, Al-Ain, United Arab Emirates
- 9Clinical Bioinformatics Unit, Department of Pathology, Faculty of Medicine and Health Sciences, Erasmus University Medical Center, Rotterdam, Netherlands
Background: Tacrolimus, an approved first-line calcineurin inhibitor, is widely prescribed in organ transplantation to prevent allograft rejection. Its narrow therapeutic index requires precise management to achieve optimal dosing and to minimize adverse drug events (ADEs) while ensuring its therapeutic efficacy. Among several factors, genetic differences contribute significantly to the inter-individual and inter-ethnic variability in pharmacokinetics (PK) of tacrolimus in kidney transplant recipients. As a result, investigating the role of genetic variation in Greek transplant recipients becomes crucial to optimizing therapeutic strategies and enhancing the efficacy of immunosuppressive treatment.
Hypothesis: Genetic variants which are known to influence the activity of enzymes or drug-transporters critical to tacrolimus pharmacokinetics, may significantly affect the required kidney post-transplant tacrolimus daily dose.
Aim: To assess the correlation of ABCB1 genetic variants (rs1128503, rs2229109) and CYP3A4 (rs2242480, rs4986910) with tacrolimus dose-adjusted trough concentration (C0/D), in Greek kidney transplant recipients.
Methods: Ninety-four unrelated Greek kidney transplant recipients were included in this study from the Department of Nephrology and Kidney Transplantation of the University General Hospital of Patras. Patients’ dose-adjusted trough levels were measured at five distinct time points after transplantation and analyzed in relation to the possible influence of CYP3A4 and correlated with the abovementioned ABCB1 genetic variants using standard genotyping analysis and Sanger sequencing.
Results: The genetic variants rs1128503, rs2229109, rs2242480, rs4986910 did not show any significant association with the daily dosing requirements of tacrolimus for at least 1 year, in Greek patients who have undergone kidney transplant.
Conclusion: It remains uncertain whether these genetic variants influence the assessment of the appropriate tacrolimus dosing 1 year after transplantation in Greek kidney transplant recipients.
Introduction
Chronic kidney disease (CKD) represents a major global public health challenge, encompassing a diverse array of heterogeneous kidney disorders (Piras et al., 2022). It is a progressive chronic disease, characterized by abnormalities in kidney function and/or structure, for at least 3 months (Lameire et al., 2021). In the absence of effective intervention, CKD progresses to end-stage kidney disease (ESKD), necessitating life-sustaining treatments such as dialysis or kidney transplantation (Degraeve et al., 2020). Kidney transplantation is considered the preferred option, as it significantly extends patient survival and enhances quality of life (Ebid et al., 2022). Post-transplant management requires lifelong immunosuppressive therapy (IS) to avoid hyperacute and acute graft rejection, as well as, to sustain long-term graft function. The standard maintenance IS regimen typically consists of tacrolimus (TAC), a calcineurin inhibitor (CNI) that prevents T-cell activation and reduces the risk of organ rejection. This is combined with an anti-metabolite such as mycophenolate mofetil (MMF), and glucocorticoids (Hart et al., 2020). In most transplant centers, the standard initial dose of TAC is 0.15-0.2 mg/kg of body weight, administered in two separate doses.
Tacrolimus is characterized by a narrow therapeutic index and considerable intra- and inter-individual pharmacokinetic variability, emphasizing the critical need for therapeutic drug monitoring (TDM). Maintaining patients’ tacrolimus blood levels within the therapeutic range, is essential to minimize the adverse effects while optimizing therapeutic efficacy (Woillard et al., 2011). High intra-patient variability in tacrolimus exposure is recognized as a predictive marker for poor clinical outcomes (Mendoza Rojas et al., 2019). Tacrolimus pharmacokinetic variability is influenced by several factors, including its poor and highly variable oral bioavailability, food and drug-drug interactions, epigenetic modifications in metabolizing enzymes, genetic variants, demographic factors, gastrointestinal conditions, low serum protein and hematocrit levels, time post-transplantation, treatment non-adherence, and microbiota composition (Degraeve et al., 2020). These factors contribute to the complexity and interindividual variability in tacrolimus pharmacokinetics.
The pharmacokinetic profile of tacrolimus is affected by single nucleotide polymorphisms (SNPs) in genes encoding drug-metabolizing enzymes, drug transporters, drug receptors, targets. Tacrolimus is metabolized by gastrointestinal and hepatic CYP3A isoenzymes, mainly CYP3A5 and CYP3A4. The CYP3A5*3 allele is among the most extensively studied, with evidence supporting the use of CYP3A5 genotyping as a preemptive strategy to guide tacrolimus dosing. CYP3A5 expressers who are carriers of at least one wild-type allele *1 generally require a starting dose 1.5 to 2 times higher than non-expressers (homozygous for the *3 allele) to achieve therapeutic blood concentrations (Birdwell et al., 2015). The CYP3A4*22 allele has also been shown to affect tacrolimus pharmacokinetics, though to a lesser extent. Carriers of this allele have decreased CYP3A4 enzyme activity, potentially resulting in elevated plasma levels of tacrolimus. Furthermore, tacrolimus is a substrate for P-glycoprotein, a multidrug efflux transporter encoded by the ABCB1 gene. In kidney transplant recipients, the combination of recipient CYP3A5 genotype and donor ABCB1 genotype has been associated with an increased risk of tacrolimus-induced nephrotoxicity (Moore et al., 2012).
Although studies suggest that genetic variations in CYP3A4 and ABCB1 may influence tacrolimus plasma concentrations, the evidence remains inconsistent. For instance, certain variants in the CYP3A4 gene, have been associated with altered enzyme activity and plasma drug levels, while other studies fail to confirm these associations (Liu et al., 2017a; Oetting et al., 2018a). Similarly, variations in the ABCB1 gene, have been linked to differences in drug absorption and distribution, but these findings have not been consistently observed across studies (Staatz et al., 2010).
Currently, limited information is available regarding Greek kidney transplant recipients. Therefore, in this study, we further evaluate the potential association between the genetic variants located in the ABCB1 gene, namely, rs1128503 (g.87550285A>G), rs2229109 (g.87550493C>T) and CYP3A4 gene, namely, rs2242480 (g.99763843C>T), rs4986910 (g.99760901A>G), based on GRCh38 reference genome, and the daily tacrolimus dose requirements in this population, during the first 12 months following kidney transplantation.
Materials and methods
Study population
A retrospective study was conducted in 94 unrelated Greek adult kidney recipients treated at the Department of Nephrology and Kidney Transplantation of the University General Hospital of Patras. Each participant had a comprehensive medical history, received a renal transplant from either living or deceased donors, and was maintained on tacrolimus-based immunosuppressive therapy for at least 1-year post-transplant. The initial pre- and post-operative dose was administered per os based on body weight, ranging from 0.15 to 0.2 mg/kg/day for Prograf®. Patients were monitored weekly during the first month post-transplant, biweekly until 3 months, and every 2–3 months thereafter. Therapeutic drug monitoring (TDM) was performed with the ARCHITECT Tacrolimus Assay, which is based on chemiluminescent microparticle immunoassay (CMIA) technology, to adjust patients’ doses within the therapeutic range, at five specific time points (first time, after 1 month, 3 months, 6 months, and finally, 1-year post-transplant). Blood samples were collected for genotyping analysis during routine follow-up visits. Medical records were reviewed to collect patient characteristics, TAC doses (D) and trough blood levels (C0). Dose-adjusted concentrations (C0/D) were also estimated across these time points.
All participants provided written informed and signed consent, and the study received approval from the Research Ethics Committee of the University of Patras, Rion, Greece (17/03/2017). All the experiments involving human subjects were conducted in compliance with the principles outlined in the Declaration of Helsinki.
Genotyping analysis
Genomic DNA from recipients was extracted from peripheral blood leukocytes using a standard phenol/chloroform protocol, followed by the assessment of DNA purity and concentration. PCR amplification conditions are available upon request.
The ABCB1 variants rs1128503 and rs2229109, were genotyped by PCR-RFLP using the EcoO109I and AcuI restriction endonucleases (NEW ENGLAND BioLabs® Inc.) respectively. For CYP3A4 variants rs4986910 and rs2242480, a PCR-based conventional Sanger sequencing method was utilized with the Big Dye Terminator v3.1 Cycle Sequencing Kit (Applied Biosystems, CA, USA). Capillary electrophoresis was performed on the ABI Prism 3130xl DNA Analyzer (Applied Biosystems).
Computational and statistical analysis
The allelic and genotype frequencies were estimated using the gene counting method. Hardy–Weinberg equilibrium was determined using the chi-square goodness of fit test (1 degree of freedom). Continuous variables are presented as means with standard deviations, and categorical data are reported as frequencies and percentages.
Computational analysis was conducted using data science and machine learning libraries in Python, with data preprocessing managed through the pandas library (McKinney, 2011). For three of the four genetic variants—rs2229109, rs1128503, and rs2242480—the preprocessing focused on selecting columns associated with the C0/D ratio at specific time points and the corresponding genotypes for each variant. Samples with unknown or highly unique genotypes, which could introduce class imbalance, were excluded to maintain data integrity. As a result, three distinct datasets were generated, each representing one of the variants. These datasets contain six columns: one identifying the genotype or sample tag, and five tracking the C0/D ratio at key time intervals (first time, 1 month, 3 months, 6 months, and 1 year). The dataset corresponding to rs2242480 comprises of 93 patient samples, while rs1128503 and rs2229109 variants were analyzed in 94 patient samples. The target variable for rs2242480 and rs2229109 is binary, reflecting the presence of two distinct genotypes. In contrast, the target variable for rs1128503 is multi-class, as it represents three unique genotypes. Classic machine learning and statistical analysis methods are applied to each dataset to demonstrate that no significant relationship exists between the input features (C0/D) and the genotype of the respective samples.
To evaluate the association between samples and their corresponding genotypes, dimensionality reduction was applied to each of the three datasets using Principal Component Analysis (PCA). Data visualization was conducted utilizing the matplotlib and seaborn libraries (Tosi, 2009; Waskom, 2021). PCA was employed to reduce the dimensionality of the data, and two-dimensional visualizations were generated for each dataset to facilitate a thorough analysis (Greenacre et al., 2022).
To further assess the association and predictive power of the five retained features in each dataset with respect to the target variable, the PyCaret Python package was utilized (Ahmad and Fahmi, 2024). For the datasets corresponding to rs2242480 and rs2229109, binary classifiers were employed, while for rs1128503, multi-class classifiers were applied. This approach facilitated the evaluation of multiple machine learning models, systematically comparing their performance to determine their ability to predict outcomes based on the selected features. To further investigate the relationship between samples and their corresponding genotypes, Pearson correlation heatmaps were generated for each dataset. This analysis was performed for all three datasets to explore potential correlations between the input features (C0/D) and the target variable, the genotype. By visualizing the correlation matrices as heatmaps, any patterns or associations present within the data could be examined in greater detail. This approach enabled a systematic assessment of the strength and nature of the correlations, providing further evidence to support the hypothesis that no significant relationship exists between the features and the genotypes in each dataset.
Results
Patient demographic characteristics
The characteristics of the 94 Greek kidney transplant recipients included in this study are summarized in Table 1. Of the patients, 61 (64.9%) were male and 33 (35.1%) were female, with a mean age of 41.87 ± 14.01 years at the time of their first kidney transplant. The kidney transplant was received from both living related 19 (20.2%) and deceased donors 75 (79.8%). Diagnoses of kidney-related conditions leading to ESKD were established based on clinical history, physical examination, and endoscopic and histological evaluations. All patients subsequently underwent kidney transplant and received life-long post-transplant tacrolimus therapy.
Allelic and genotype frequencies in kidney transplant recipients
The genotype and allele frequencies of the four genetic variants among these 94 Greek patients are presented in Table 2. All genotype frequencies adhered to Hardy-Weinberg equilibrium (p-value>0.05) except for the rs1128503 variant (χ2 > 3.841). Allele frequencies for most variants, with the exception of rs2229109, were consistent with those previously reported for Caucasian populations in public databases such as Ensembl. For the rs1128503 variant, the genotype distribution was as follows: 17 (18.09%) patients had the G/G genotype, 60 (63.83%) were heterozygous (A/G), and 17 (18.09%) had the A/A genotype. The total allele frequencies were 50% for the G allele and 50% for the A allele. Regarding the rs2229109 variant, 74 (78.72%) had the wild-type C/C genotype, while 20 (21.28%) were heterozygous (C/T). The total allele frequencies were 89.36% for the C allele and 10.64% for the T allele. For the rs4986910 variant, all patients had the wild-type A/A genotype, resulting in an allele frequency of 100% for the A allele. Lastly, for the rs2242480 variant, 75 (79.79%) had the C/C genotype, 18 (19.15%) were heterozygous (C/T), and 1 (1.06%) had the T/T genotype. The total allele frequencies were 89.36% for the C allele and 10.64% for the T allele.
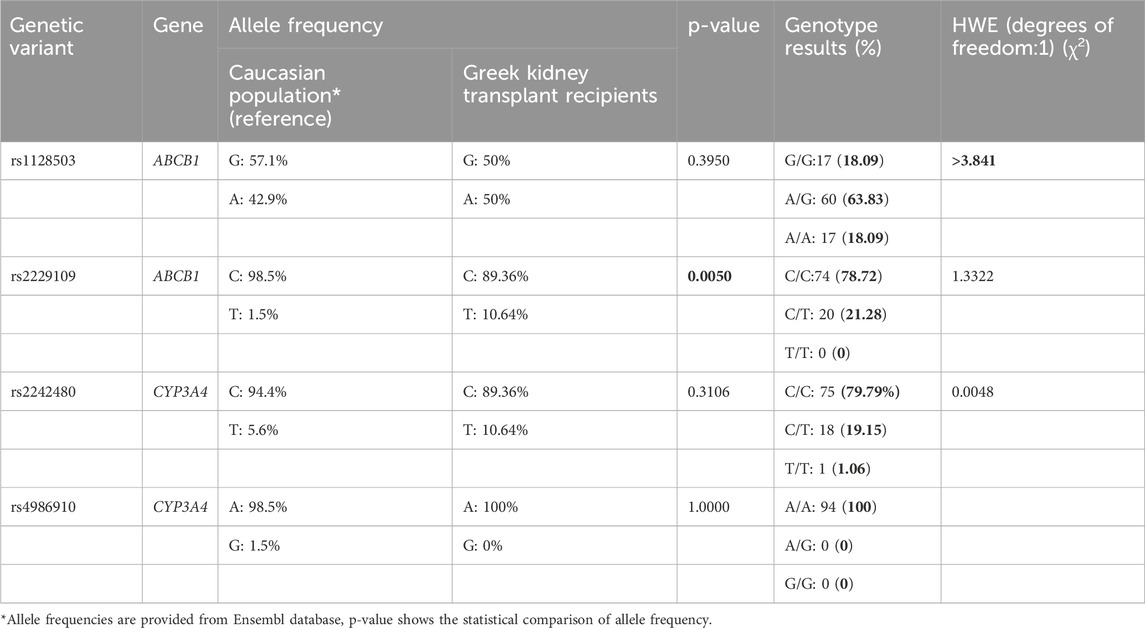
Table 2. Genotype allele frequency, and Hardy-Weinberg equilibrium (HWE) assessment of the four genetic variants.
Association of the genetic variants with the Co/D ratio of tacrolimus in renal transplant recipients
At the first time, and months 1, 3, 6 and 12 after kidney transplantation, the average tacrolimus doses (mg/day) were 6.39 ± 3.26, 6.14 ± 3.58, 5.51 ± 3.23, 4.82 ± 2.95, and 4.26 ± 2.68, respectively. The tacrolimus trough concentrations (C0) and dose-adjusted trough concentration ratios (C0/D) are shown in Table 3.
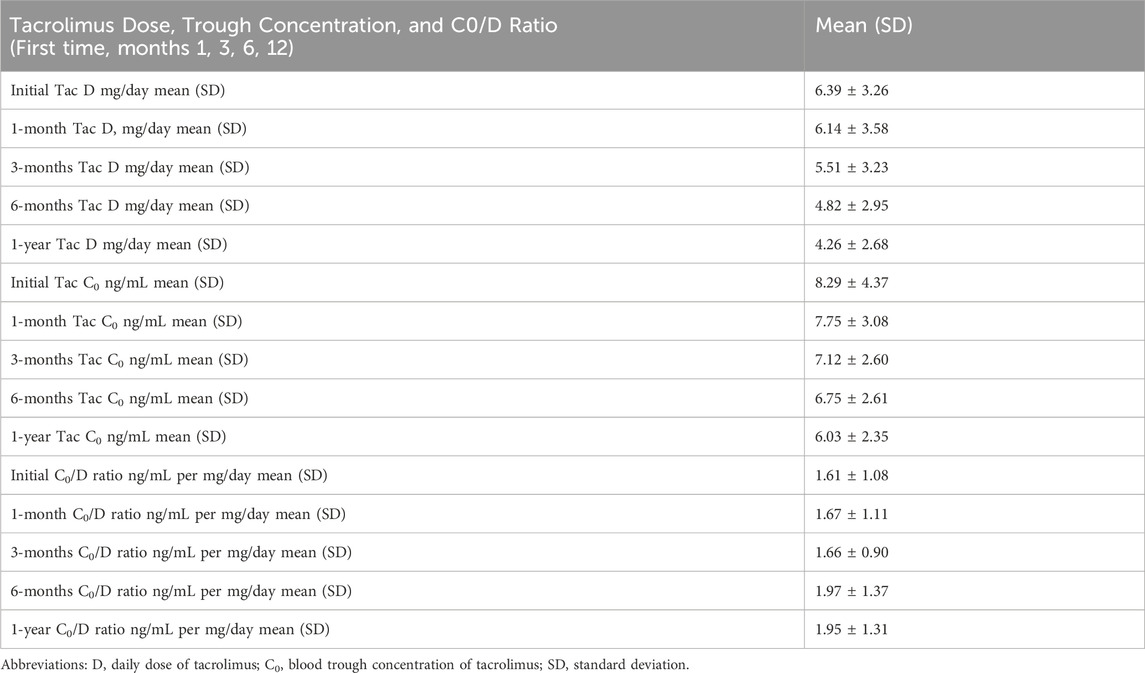
Table 3. Tacrolimus dose (mg/day), trough concentration (ng/mL) and Co/D ratio (ng/ml per mg/day) in Greek kidney transplant patients at the first time, months 1, 3, 6 and 12 after transplantation.
The results of the dimensionality reduction algorithms are presented in Figure 1 for the three genetic variants (A) rs1128503, (B) rs2229109, and (C) rs2242480. In all plots, each dot represents a sample, with colors corresponding to the genotype of each sample. Across all cases, there is no clear pattern of separation between samples, which serves as a clear indication that the C0/D ratio does not exhibit a significant association with genotype.
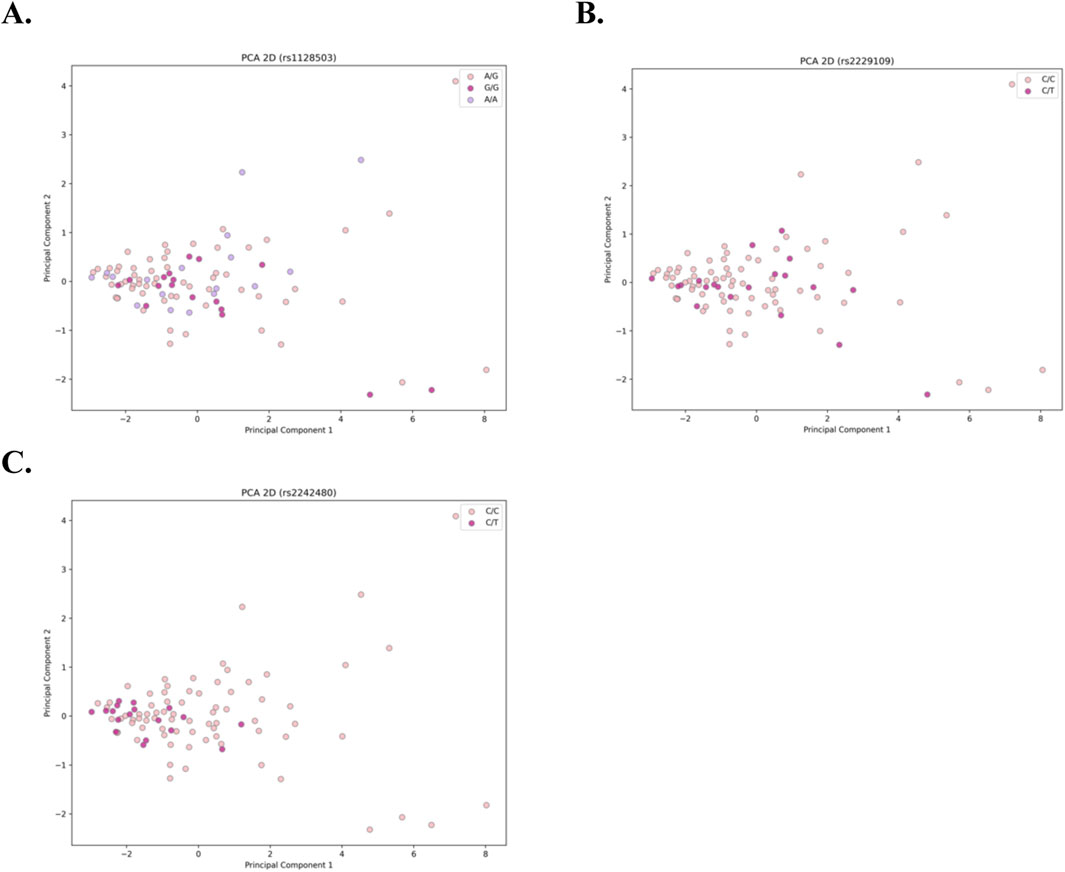
Figure 1. Two-dimensional PCA plots for the 3 genetic variants: (A) rs1128503, (B) rs2229109, and (C) rs2242480. Each dot represents an individual sample, with colors denoting the corresponding genotype. The absence of distinct clustering or separation among samples across all cases indicates no significant association between the C0/D ratio and genotype.
Figure 2 presents the results of the PyCaret model evaluations, showing the confusion matrices for the best-performing model of the three genetic variants (A) rs1128503, (B) rs2229109, and (C) rs2242480. Across all three datasets, the confusion matrices suggest that the models underperform, failing to achieve optimal results for binary and/or multiclass classification. In both binary classification instances, the classifiers are biased towards class 0 whereas in the multiclass instance the model is biased towards class 1. These patterns further indicate that the features derived from the C0/D ratio do not significantly contribute to predicting the genotype in any of the cases.
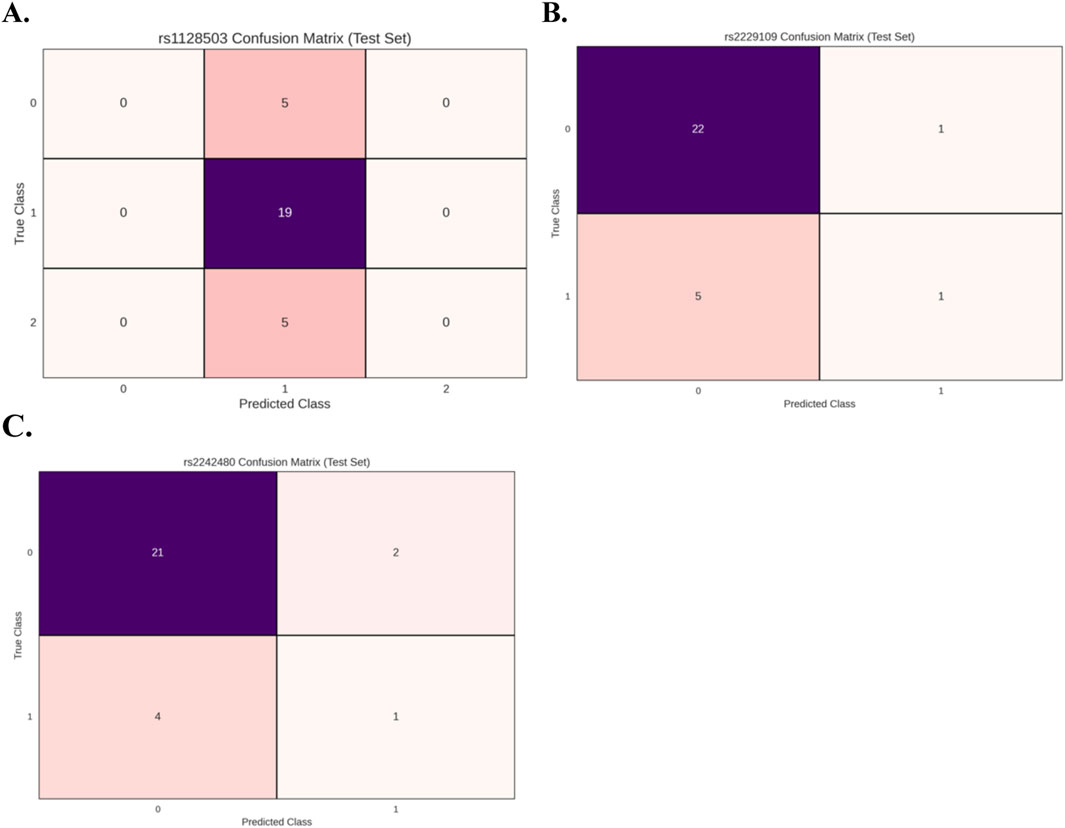
Figure 2. Confusion matrices for the best-performing models evaluated using PyCaret for the 3 genetic variants: (A) rs1128503 (multi-class classification), (B) rs2229109 (binary classification), and (C) rs2242480 (binary classification). The results indicate suboptimal model performance across all datasets. In binary classification cases, the models exhibit a bias toward class 0, while in the multi-class case, the model demonstrates a bias toward class 1. These findings highlight the lack of predictive power of features derived from the C0/D ratio in determining genotype.
Figure 3 presents the per-sample correlation heatmaps for the three datasets (A) rs1128503, (B) rs2229109, and (C) rs2242480 under computational analysis. No distinct correlation pattern is observed between samples in relation to their genotypes. Some samples exhibit strong positive pairwise correlations, while others show weak or no correlation, regardless of whether they share the same genotype. This lack of a consistent correlation pattern further suggests that genotype does not have a significant association with the C0/D ratios across the different time points.
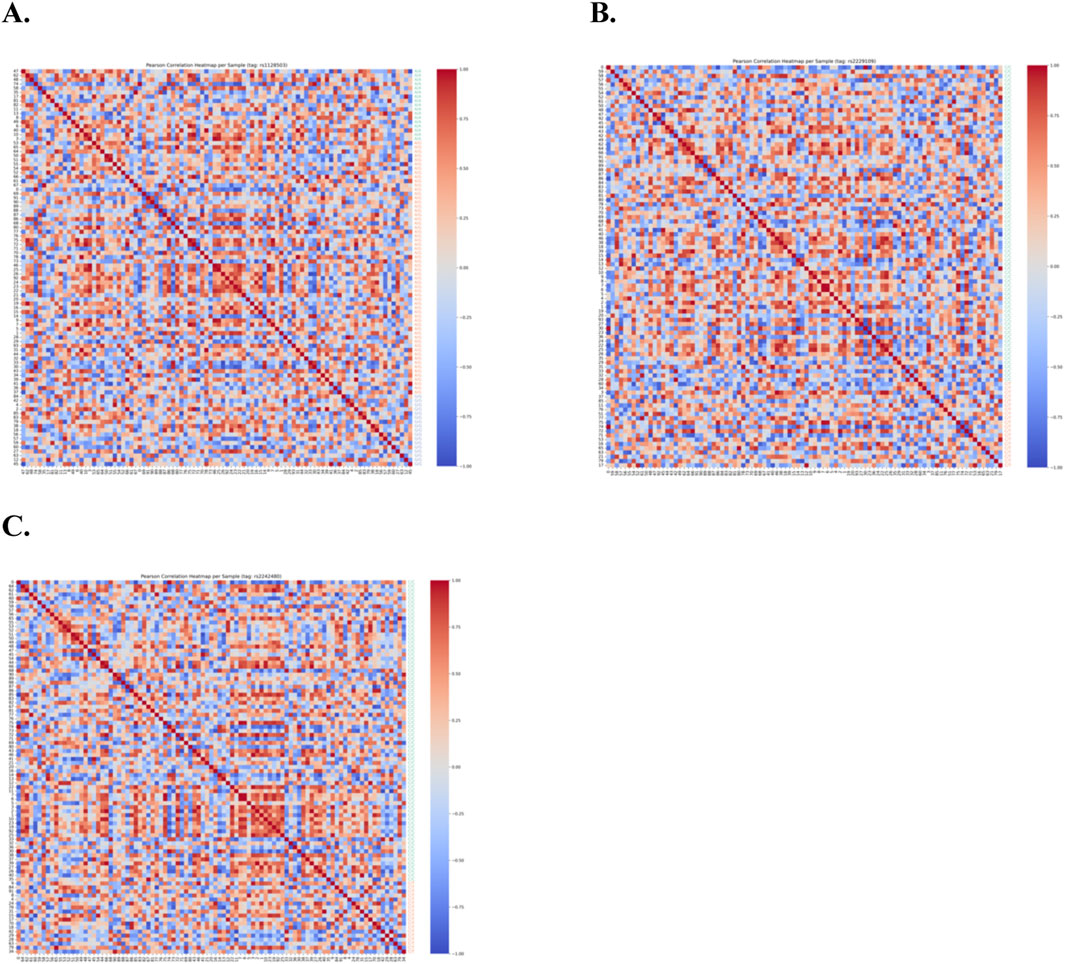
Figure 3. Per-sample Pearson correlation heatmaps for the three datasets: (A) rs1128503, (B) rs2229109, and (C) rs2242480. The heatmaps reveal no distinct correlation patterns between samples and their genotypes. While some samples display strong positive pairwise correlations, others exhibit weak or negligible correlations, regardless of genotype. This inconsistency further supports the conclusion that the C0/D ratios are not significantly associated with genotype across the datasets.
Discussion
The clinical use of tacrolimus is challenging due to its narrow therapeutic range and interindividual pharmacokinetic variability. While therapeutic drug monitoring is commonly utilized to optimize dosing, the risk of underimmunosuppression and acute rejection remain significant concerns. Notably, tacrolimus trough levels can exhibit considerable variability, even during routine monitoring in the stable post-transplant phase (Mohamed et al., 2019). The role of P-glycoprotein and CYP3A enzymes in the pharmacokinetics of tacrolimus has long been acknowledged, as they are key determinants of its metabolism and transport (Hesselink D. et al., 2003). Interestingly though, the current retrospective study failed to demonstrate significant association between genetic variants in the ABCB1 and CYP3A4 genes and the pharmacokinetic profile of tacrolimus in Greek renal transplant recipients, a finding that is consistent with previous cohort studies in other populations (Table 4). Also, the number of cases collected within the scope of the present study (n = 94) is sufficient to suggest that these specific variants are not really clinically relevant in the Greek population.
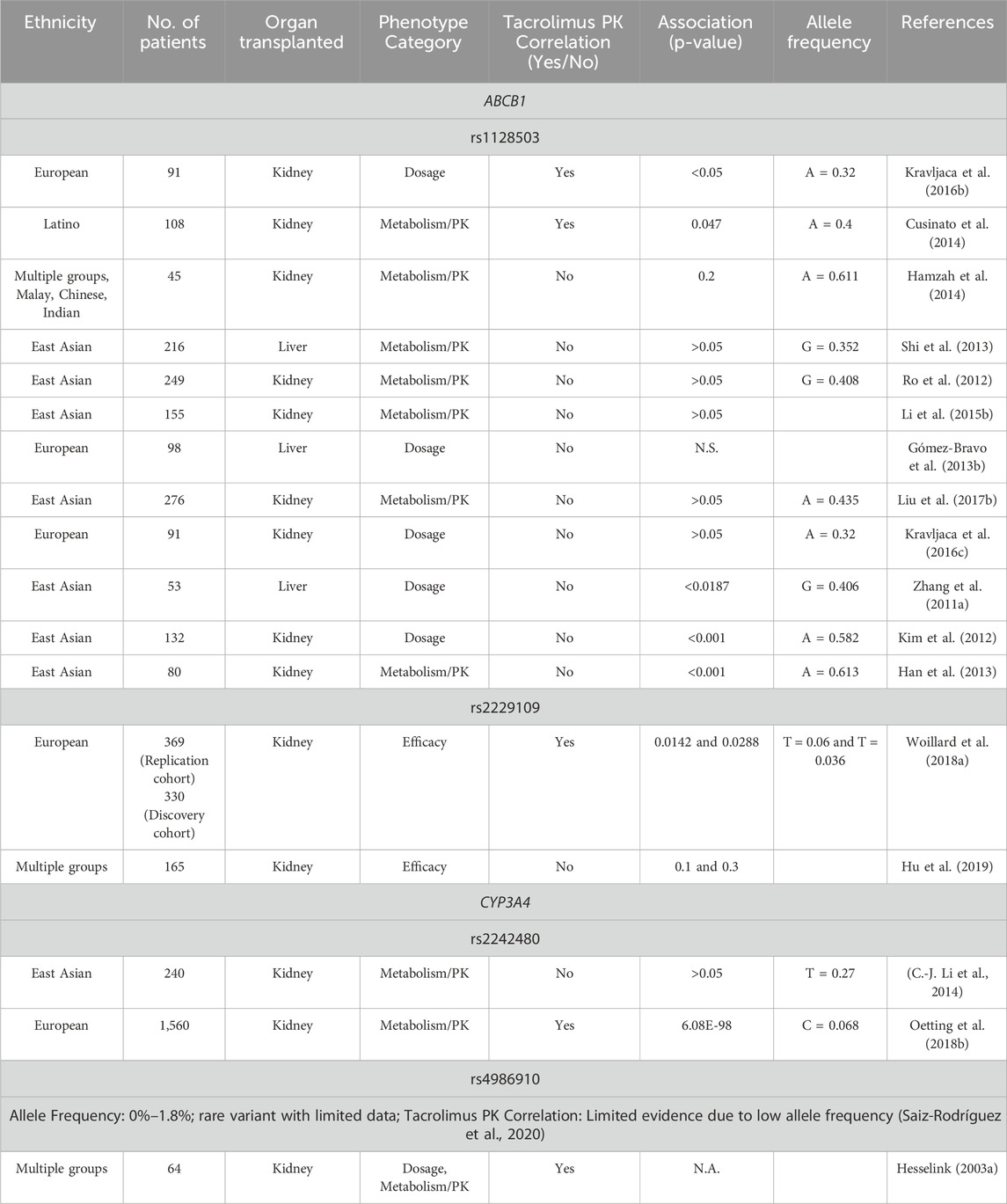
Table 4. Summary of studies investigating the association between ABCB1 and CYP3A4 variants and tacrolimus pharmacokinetics in kidney transplant recipients.
Tacrolimus undergoes counter-transport by P-glycoprotein, and the influence of ABCB1 genetic variants on P-gp expression, function, and its plasma concentration remains controversial. The influence of ABCB1 genetic variants in determining tacrolimus dosing has been questioned in previous studies, with contradictory findings. While studies have reported associations, others have found no significant link between ABCB1 polymorphisms and tacrolimus dose requirements (Haufroid et al., 2006; Mourad et al., 2005). Consistent with these findings, our study observed no statistically significant association between ABCB1 genotypes and any tacrolimus pharmacokinetic variables. One possible explanation is that the effects of ABCB1 polymorphisms may be masked by the influence of more pronounced CYP3A enzyme polymorphisms (Zhang et al., 2011a).
The variability in the relationship between ABCB1 variants and tacrolimus response, may also be influenced by differences in immunosuppression protocols across studies, ethnic-related genetic diversity, or limitations due to small sample sizes (Gómez-Bravo et al., 2013a). For instance, the variant A allele frequency for rs1128503 ranges from 10% to 63% across populations, with a frequency of 43% in Caucasians and 14% in Africans (Ensembl). In contrast, our cohort shows an 18.09% frequency of the A/A homozygous genotype for rs1128503, compared to 1.09% reported by Kravljaca et al. (2016a). This deviation suggests potential selective pressures specific to our cohort of Greek transplant patients, as indicated by Hardy–Weinberg disequilibrium for rs1128503.
The impact of rs1128503 variant on tacrolimus concentrations remains uncertain. While some studies have reported a relatively low association between this SNP and elevated tacrolimus blood concentrations (Cheung et al., 2006; Fredericks et al., 2006a), others have found no significant effect (Jun et al., 2009; Li et al., 2015a). In our study, we observed a negative association between rs1128503 variant and tacrolimus C0/D ratios across five distinct time points. Interestingly, findings from Zhang et al. (2011b) indicated that individuals with the A/A genotype, had higher tacrolimus blood levels compared to those with the G/G genotype.
The complex role of ABCB1 variants in tacrolimus pharmacokinetics is further explored through haplotype studies of the rs1045642, rs2032582, and rs1128503 variants. In a cohort of 81 kidney transplant recipients, the C-G-C haplotype was found to be associated with higher tacrolimus dose requirements compared to the T-T-T haplotype (Anglicheau et al., 2003). These SNPs are in varying degrees of linkage disequilibrium (LD), raising the question whether tacrolimus pharmacokinetics is influenced by a single variant or the combined haplotype (Grinyó et al., 2008; Wang et al., 2005). rs2032582 and rs1045642 have shown strong LD, with reported r2 or D′ values greater than 0.8 (Bochud et al., 2008; Grinyó et al., 2008). While rs2032582 is a nonsynonymous SNP, rs1045642 and rs1128503 are synonymous, suggesting that rs2032582 may have a more direct functional impact on ABCB1 activity. Despite these associations, only rs1045642 has been identified as influencing ABCB1 gene expression (Hoffmeyer et al., 2000). A study with 206 renal transplant recipients found no significant association with ABCB1 haplotypes, especially after adjusting for CYP3A5 status (Fredericks et al., 2006b). Thus, it remains unclear whether these effects are driven by a single SNP or the combined haplotype, as other genetic and clinical factors may also contribute to interindividual variability in tacrolimus response.
The rs2229109 variant was detected in 10.64% of our cohort, a frequency that is markedly higher than the 1.5% reported in broader Caucasian populations. This could be attributed to the small sample size or the ethnically homogenous nature of the Greek cohort. The ABCB1 protein encoded by the wild type rs2229109 allele, is more efficient at transporting tacrolimus compared to the variant allele, suggesting that the p.Ser400Asn amino acid substitution may impair ABCB1’s ability to efflux tacrolimus. This alteration may partially account for interindividual differences in drug response (Dessilly et al., 2014). Additionally, other transporters from the ABC or SLC families, such as MRP2, MRP4, and MATE, which are expressed in the kidney, could minimally contribute to tacrolimus transport (Woillard et al., 2018a). Research on the impact of rs2229109 genetic variant on tacrolimus response is limited. Hu and coworkers (2018) reported that the C/T genotype of rs2229109 did not increase the risk of transplant rejection compared to the C/C genotype, further highlighting the need for more comprehensive studies.
In our study, the rs4986910 variant was not detected among Greek transplant recipients, despite its 1.5% frequency in Caucasians. It can alter the enzyme structure, potentially affecting function, though further investigation is needed to confirm this. According to a study by Hesselink D. A. et al. (2003), organ transplant recipients who carry the variant allele, may require lower tacrolimus doses compared to homozygotes for the wild type allele. However, variant’s low prevalence, limits available clinical evidence on its effect on tacrolimus dosing.
Our findings align with previous evidence indicating no significant association between the C0/D ratio of tacrolimus and rs2242480 in Greek renal transplant patients. Specifically, Li and coworkers (2014) reported that the C/C wild-type genotype was not associated with a likelihood of achieving target concentrations of tacrolimus when compared to the C/T + T/T genotypes in kidney transplant recipients. Similarly, Liu and coworkers (2019) found no significant difference in tacrolimus concentrations between children with nephrotic range proteinuria carrying the T allele and those with the C/C genotype. These findings further support our observation that the rs2242480 variant, does not appear to play a key role in determining tacrolimus plasma levels. Larger prospective studies are necessary to further explore its impact on tacrolimus pharmacokinetics in transplant recipients. This conclusion is further substantiated through the application of well-established statistical and machine learning methodologies, which clearly demonstrated that the C0/D ratio lacks predictive power in relation to the target variable, the genotype of each patient.
The primary limitations of this study include its single-center retrospective study design and a relatively small cohort size. Additionally, the potential combined effects of CYP3A4, CYP3A5, and ABCB1 genetic variants on tacrolimus pharmacokinetics were not investigated. Also, the determination of Greek ancestry was based on self-reported ethnicity, which could have been strengthened by genetic ancestry verification. Moreover, the potential influence of co-medications, particularly those that inhibit or induce CYP3A enzymes, on tacrolimus metabolism was not specifically analyzed, which may have contributed to variability in drug response. Given the population-specific genetic variability, genetic stratification is crucial for more accurate results. Future prospective, multi-center studies with a larger cohort of transplant recipients are necessary to develop a reliable tool for monitoring immune responses.
This study utilized a candidate-gene approach, a hypothesis-driven methodology commonly used to investigate associations between specific single nucleotide variants and complex outcomes. While this approach is valuable, it limits the potential for discovering other relevant pharmacogenes, or rare variants that may be associated with clinical outcomes. To address these limitations, future research would benefit from techniques such as whole genome sequencing, which provide a more agnostic and comprehensive genetic insight. However, these approaches require large patient cohorts to detect statistically significant associations, given the low frequency and penetrance of such genetic variations (Woillard et al., 2018b).
Conclusion
The present study did not find a significant association between ABCB1 rs1128503, rs2229109 and CYP3A4 rs2242480, rs4986910 variants and tacrolimus exposure in Greek renal transplant patients. Based on the findings, no significant correlation between these genetic variants and tacrolimus dosing requirements was revealed. These results underscore the complexity of pharmacogenomic factors on tacrolimus metabolism and highlight the necessity for further research to uncover additional factors contributing to the variability in drug response among renal transplant recipients. Such insights are crucial for advancing personalized medicine, enabling tailored therapeutic strategies, and ultimately improving clinical outcomes in kidney transplantation.
Data availability statement
The raw data supporting the conclusions of this article will be made available by the authors, without undue reservation.
Ethics statement
The studies involving humans were approved by University of Patras Ethics Board. The studies were conducted in accordance with the local legislation and institutional requirements. The participants provided their written informed consent to participate in this study.
Author contributions
AT: Conceptualization, Formal Analysis, Validation, Writing–original draft, Writing–review and editing. KL: Data curation, Formal Analysis, Methodology, Writing–review and editing. EM: Formal Analysis, Methodology, Writing–original draft, Writing–review and editing. MP: Formal Analysis, Writing–review and editing. SS: Data curation, Formal Analysis, Writing–review and editing. KK: Formal Analysis, Writing–review and editing. AJ: Formal Analysis, Writing–review and editing. AG: Formal Analysis, Writing–review and editing. SG: Formal Analysis, Writing–review and editing. BRA: Funding acquisition, Resources, Supervision, Writing–review and editing. AV: Data curation, Formal Analysis, Methodology, Supervision, Writing–review and editing. GPP: Conceptualization, Funding acquisition, Project administration, Resources, Supervision, Writing–original draft, Writing–review and editing.
Funding
The author(s) declare that financial support was received for the research and/or publication of this article. This work was partly funded by a European Commission grant (HORIZON-HLTH-2021-CARE-05–01; Grant Agreement 101057639) to GPP.
Conflict of interest
Authors KK, AG, and SG were employed by ANALYSI Biomedical Laboratories S.A.
The remaining authors declare that the research was conducted in the absence of any commercial or financial relationships that could be construed as a potential conflict of interest.
The author(s) declared that they were an editorial board member of Frontiers, at the time of submission. This had no impact on the peer review process and the final decision.
Generative AI statement
The author(s) declare that no Generative AI was used in the creation of this manuscript.
Publisher’s note
All claims expressed in this article are solely those of the authors and do not necessarily represent those of their affiliated organizations, or those of the publisher, the editors and the reviewers. Any product that may be evaluated in this article, or claim that may be made by its manufacturer, is not guaranteed or endorsed by the publisher.
References
Ahmad, F. N., and Fahmi, H. (2024). Feature importance and binary classification using PyCare. J. Data Sci. Technol. Artif. Intell.
Anglicheau, D., Verstuyft, C. A. A., Laurent-Puig, P., Becquemont, L., Schlageter, M.-H. A. A. G. A., Cassinat, B., et al. (2003). Association of the multidrug resistance-1 gene single-nucleotide polymorphisms with the tacrolimus dose requirements in renal transplant recipients. J. Am. Soc. Nephrol. 14 (7), 1889–1896. doi:10.1097/01.ASN.0000073901.94759.36
Birdwell, K., Decker, B., Barbarino, J., Peterson, J., Stein, C., Sadee, W., et al. (2015). Clinical pharmacogenetics implementation consortium (CPIC) guidelines for CYP3A5 genotype and tacrolimus dosing. Clin. Pharmacol. and Ther. 98 (1), 19–24. doi:10.1002/cpt.113
Bochud, M., Eap, C. B., Maillard, M., Johnson, T., Vollenweider, P., Bovet, P., et al. (2008). Association of ABCB1 genetic variants with renal function in Africans and in Caucasians. BMC Med. Genomics 1, 21. doi:10.1186/1755-8794-1-21
Cheung, C. Y., Op den Buijsch, R. A., Ming Wong, K., Wong Chan, H., Foon Chau, K., Sang Li, C., et al. (2006). Influence of different allelic variants of the CYP3A and ABCB1 genes on the tacrolimus pharmacokinetic profile of Chinese renal transplant recipients. Pharmacogenomics 7 (4), 563–574. doi:10.2217/14622416.7.4.563
Cusinato, D. A. C., Lacchini, R., Romao, E. A., Moysés-Neto, M., and Coelho, E. B. (2014). Relationship of CYP3A5 genotype and ABCB1 diplotype to tacrolimus disposition in Brazilian kidney transplant patients. Br. J. Clin. Pharmacol. 78(2), 364–372. doi:10.1111/bcp.12345
Degraeve, A. L., Moudio, S., Haufroid, V., Chaib Eddour, D., Mourad, M., Bindels, L. B., et al. (2020). Predictors of tacrolimus pharmacokinetic variability: current evidences and future perspectives. Expert Opin. Drug Metabolism and Toxicol. 16 (9), 769–782. doi:10.1080/17425255.2020.1803277
Dessilly, G., Elens, L., Panin, N., Capron, A., Decottignies, A., Demoulin, J.-B., et al. (2014). ABCB1 1199G>A genetic polymorphism (Rs2229109) influences the intracellular accumulation of tacrolimus in HEK293 and K562 recombinant cell lines. PLoS ONE 9 (3), e91555. doi:10.1371/journal.pone.0091555
Ebid, A. I. M., Ismail, D. A., Lotfy, N. M., Mahmoud, M. A., and Elsharkawy, M. (2022). Influence of CYP3A4 *22 and CYP3A5 *3 combined genotypes on tacrolimus dose requirements in Egyptian renal transplant patients. J. Clin. Pharm. Ther. 47 (12), 2255–2263. doi:10.1111/jcpt.13804
Fredericks, S., Moreton, M., Reboux, S., Carter, N. D., Goldberg, L., Holt, D. W., et al. (2006a). Multidrug resistance gene-1 (MDR-1) haplotypes have a minor influence on tacrolimus dose requirements. Transplantation 82 (5), 705–708. doi:10.1097/01.tp.0000234942.78716.c0
Fredericks, S., Moreton, M., Reboux, S., Carter, N. D., Goldberg, L., Holt, D. W., et al. (2006b). Multidrug resistance gene-1 (MDR-1) haplotypes have a minor influence on tacrolimus dose requirements. Transplantation 82 (5), 705–708. doi:10.1097/01.tp.0000234942.78716.c0
Gómez-Bravo, M. A., Salcedo, M., Fondevila, C., Suarez, F., Castellote, J., Rufian, S., et al. (2013a). Impact of donor and recipient CYP3A5 and ABCB1 genetic polymorphisms on tacrolimus dosage requirements and rejection in Caucasian Spanish liver transplant patients. J. Clin. Pharmacol. 53 (11), 1146–1154. doi:10.1002/jcph.154
Gómez-Bravo, M. A., Salcedo, M., Fondevila, C., Suarez, F., Castellote, J., Rufian, S., et al. (2013b). Impact of donor and recipient CYP3A5 and ABCB1 genetic polymorphisms on tacrolimus dosage requirements and rejection in Caucasian Spanish liver transplant patients. J. Clin. Pharmacol. 53 (11), 1146–1154. doi:10.1002/jcph.154
Greenacre, M., Groenen, P. J. F., Hastie, T., D’Enza, A. I., Markos, A., and Tuzhilina, E. (2022). Principal component analysis. Nat. Rev. Methods Prim. 2 (1), 100. doi:10.1038/s43586-022-00184-w
Grinyó, J., Vanrenterghem, Y., Nashan, B., Vincenti, F., Ekberg, H., Lindpaintner, K., et al. (2008). Association of four DNA polymorphisms with acute rejection after kidney transplantation. Transpl. Int. 21 (9), 879–891. doi:10.1111/j.1432-2277.2008.00679.x
Hamzah, S., Teh, L. K., Siew, J. S. K., Ahmad, G., Wong, H. S., Zakaria, Z. A., et al. (2014). Pharmacogenotyping of CYP3A5 in predicting dose-adjusted trough levels of tacrolimus among Malaysian kidney-transplant patients. Can. J. Physiology Pharmacol. 92 (1), 50–57. doi:10.1139/cjpp-2013-0128
Han, N., Yun, H., Hong, J., Kim, I.-W., Ji, E., Hong, S. H., et al. (2013). Prediction of the tacrolimus population pharmacokinetic parameters according to CYP3A5 genotype and clinical factors using NONMEM in adult kidney transplant recipients. Eur. J. Clin. Pharmacol. 69 (1), 53–63. doi:10.1007/s00228-012-1296-4
Hart, A., Smith, J. M., Skeans, M. A., Gustafson, S. K., Wilk, A. R., Castro, S., et al. (2020). OPTN/SRTR 2018 annual data report: kidney. Am. J. Transplant. 20, 20–130. doi:10.1111/ajt.15672
Haufroid, V., Wallemacq, P., VanKerckhove, V., Elens, L., De Meyer, M., Eddour, D. C., et al. (2006). CYP3A5 and ABCB1 polymorphisms and tacrolimus pharmacokinetics in renal transplant candidates: guidelines from an experimental study. Am. J. Transplant. 6 (11), 2706–2713. doi:10.1111/j.1600-6143.2006.01518.x
Hesselink, D., van Schaik, R. H. N., van der Heiden, I. P., van der Werf, M., Gregoor, P. J. H. S., Lindemans, J., et al. (2003a). Genetic polymorphisms of the CYP3A4, CYP3A5, and MDR-1 genes and pharmacokinetics of the calcineurin inhibitors cyclosporine and tacrolimus. Clin. Pharmacol. and Ther. 74 (3), 245–254. doi:10.1016/S0009-9236(03)00168-1
Hesselink, D. A., Van Schaik, R. H. N., Van Der Heiden, I. P., Van Der Werf, M., Smak Gregoor, P. J. H., Lindemans, J., et al. (2003b). Genetic polymorphisms of the CYP3A4, CYP3A5, and MDR-1 genes and pharmacokinetics of the calcineurin inhibitors cyclosporine and tacrolimus. Clin. Pharmacol. Ther. 74 (3), 245–254. doi:10.1016/S0009-9236(03)00168-1
Hoffmeyer, S., Burk, O., von Richter, O., Arnold, H. P., Brockmöller, J., Johne, A., et al. (2000). Functional polymorphisms of the human multidrug-resistance gene: multiple sequence variations and correlation of one allele with P-glycoprotein expression and activity in vivo. Proc. Natl. Acad. Sci. 97 (7), 3473–3478. doi:10.1073/pnas.050585397
Hu, R., Barratt, D. T., Coller, J. K., Sallustio, B. C., and Somogyi, A. A. (2019). Effect of tacrolimus dispositional genetics on acute rejection in the first 2 weeks and estimated glomerular filtration rate in the first 3 months following kidney transplantation. Pharmacogenetics Genomics 29 (1), 9–17. doi:10.1097/FPC.0000000000000360
Jun, K. R., Lee, W., Jang, M. S., Chun, S., Song, G.-W., Park, K. T., et al. (2009). Tacrolimus concentrations in relation to CYP3A and ABCB1 polymorphisms among solid organ transplant recipients in korea. Transplantation 87 (8), 1225–1231. doi:10.1097/TP.0b013e31819f117e
Kim, I.-W., Moon, Y. J., Ji, E., Kim, K. I., Han, N., Kim, S. J., et al. (2012). Clinical and genetic factors affecting tacrolimus trough levels and drug-related outcomes in Korean kidney transplant recipients. Eur. J. Clin. Pharmacol. 68 (5), 657–669. doi:10.1007/s00228-011-1182-5
Kravljaca, M., Perovic, V., Pravica, V., Brkovic, V., Milinkovic, M., Lausevic, M., et al. (2016a). The importance of MDR1 gene polymorphisms for tacrolimus dosage. Eur. J. Pharm. Sci. 83, 109–113. doi:10.1016/j.ejps.2015.12.020
Kravljaca, M., Perovic, V., Pravica, V., Brkovic, V., Milinkovic, M., Lausevic, M., et al. (2016b). The importance of MDR1 gene polymorphisms for tacrolimus dosage. Eur. J. Pharm. Sci. 83, 109–113. doi:10.1016/j.ejps.2015.12.020
Kravljaca, M., Perovic, V., Pravica, V., Brkovic, V., Milinkovic, M., Lausevic, M., et al. (2016c). The importance of MDR1 gene polymorphisms for tacrolimus dosage. Eur. J. Pharm. Sci. 83, 109–113. doi:10.1016/j.ejps.2015.12.020
Lameire, N. H., Levin, A., Kellum, J. A., Cheung, M., Jadoul, M., Winkelmayer, W. C., et al. (2021). Harmonizing acute and chronic kidney disease definition and classification: report of a kidney disease: improving global outcomes (KDIGO) consensus conference. Kidney Int. 100 (3), 516–526. doi:10.1016/j.kint.2021.06.028
Li, C.-J., Li, L., Lin, L., Jiang, H.-X., Zhong, Z.-Y., Li, W.-M., et al. (2014). Impact of the CYP3A5, CYP3A4, COMT, IL-10 and POR genetic polymorphisms on tacrolimus metabolism in Chinese renal transplant recipients. PLoS ONE 9 (1), e86206. doi:10.1371/journal.pone.0086206
Li, Y., Yan, L., Shi, Y., Bai, Y., Tang, J., and Wang, L. (2015a). CYP3A5 and ABCB1 genotype influence tacrolimus and sirolimus pharmacokinetics in renal transplant recipients. SpringerPlus 4 (1), 637. doi:10.1186/s40064-015-1425-5
Li, Y., Yan, L., Shi, Y., Bai, Y., Tang, J., and Wang, L. (2015b). CYP3A5 and ABCB1 genotype influence tacrolimus and sirolimus pharmacokinetics in renal transplant recipients. SpringerPlus 4 (1), 637. doi:10.1186/s40064-015-1425-5
Liu, F., Ou, Y.-M., Yu, A.-R., Xiong, L., and Xin, H.-W. (2017a). Long-term influence of CYP3A5, CYP3A4, ABCB1, and NR1I2 polymorphisms on tacrolimus concentration in Chinese renal transplant recipients. Genet. Test. Mol. Biomarkers 21 (11), 663–673. doi:10.1089/gtmb.2017.0088
Liu, F., Ou, Y.-M., Yu, A.-R., Xiong, L., and Xin, H.-W. (2017b). Long-term influence of CYP3A5, CYP3A4, ABCB1, and NR1I2 polymorphisms on tacrolimus concentration in Chinese renal transplant recipients. Genet. Test. Mol. Biomarkers 21 (11), 663–673. doi:10.1089/gtmb.2017.0088
McKinney, W. (2011). Pandas: a foundational Python library for data analysis and statistics. Available online at: https://api.semanticscholar.org/CorpusID:61539023.
Mendoza Rojas, A., Hesselink, D. A., van Besouw, N. M., Baan, C. C., and van Gelder, T. (2019). Impact of low tacrolimus exposure and high tacrolimus intra-patient variability on the development of de novo anti-HLA donor-specific antibodies in kidney transplant recipients. Expert Rev. Clin. Immunol. 15 (12), 1323–1331. doi:10.1080/1744666X.2020.1693263
Mohamed, M. E., Schladt, D. P., Guan, W., Wu, B., van Setten, J., Keating, B. J., et al. (2019). Tacrolimus troughs and genetic determinants of metabolism in kidney transplant recipients: a comparison of four ancestry groups. Am. J. Transplant. 19 (10), 2795–2804. doi:10.1111/ajt.15385
Moore, J., McKnight, A. J., Döhler, B., Simmonds, M. J., Courtney, A. E., Brand, O. J., et al. (2012). Donor ABCB1 variant associates with increased risk for kidney allograft failure. J. Am. Soc. Nephrol. 23 (11), 1891–1899. doi:10.1681/ASN.2012030260
Mourad, M., Mourad, G., Wallemacq, P., Garrigue, V., Van Bellingen, C., Van Kerckhove, V., et al. (2005). Sirolimus and tacrolimus trough concentrations and dose requirements after kidney transplantation in relation to CYP3A5 and MDR1 polymorphisms and steroids. Transplantation 80 (7), 977–984. doi:10.1097/01.TP.0000174131.47469.D2
Oetting, W. S., Wu, B., Schladt, D. P., Guan, W., Remmel, R. P., Dorr, C., et al. (2018a). Attempted validation of 44 reported SNPs associated with tacrolimus troughs in a cohort of kidney allograft recipients. Pharmacogenomics 19 (3), 175–184. doi:10.2217/pgs-2017-0187
Oetting, W. S., Wu, B., Schladt, D. P., Guan, W., Remmel, R. P., Dorr, C., et al. (2018b). Attempted validation of 44 reported SNPs associated with tacrolimus troughs in a cohort of kidney allograft recipients. Pharmacogenomics 19 (3), 175–184. doi:10.2217/pgs-2017-0187
Piras, D., Lepori, N., Cabiddu, G., and Pani, A. (2022). How genetics can improve clinical practice in chronic kidney disease: from bench to bedside. J. Personalized Med. 12 (2), 193. doi:10.3390/jpm12020193
Ro, H., Min, S.-I., Yang, J., Moon, K. C., Kim, Y. S., Kim, S. J., et al. (2012). Impact of tacrolimus intraindividual variability and CYP3A5 genetic polymorphism on acute rejection in kidney transplantation. Ther. Drug Monit. 34 (6), 680–685. doi:10.1097/FTD.0b013e3182731809
Saiz-Rodríguez, M., Almenara, S., Navares-Gómez, M., Ochoa, D., Román, M., Zubiaur, P., et al. (2020). Effect of the most relevant CYP3A4 and CYP3A5 polymorphisms on the pharmacokinetic parameters of 10 CYP3A substrates. Biomedicines 8 (4), 94. doi:10.3390/biomedicines8040094
Shi, Y., Li, Y., Tang, J., Zhang, J., Zou, Y., Cai, B., et al. (2013). Influence of CYP3A4, CYP3A5 and MDR-1 polymorphisms on tacrolimus pharmacokinetics and early renal dysfunction in liver transplant recipients. Gene 512 (2), 226–231. doi:10.1016/j.gene.2012.10.048
Staatz, C. E., Goodman, L. K., and Tett, S. E. (2010). Effect of CYP3A and ABCB1 single nucleotide polymorphisms on the pharmacokinetics and pharmacodynamics of calcineurin inhibitors: Part II. Clin. Pharmacokinet. 49 (4), 207–221. doi:10.2165/11317550-000000000-00000
Tosi, S. (2009). Matplotlib for Python developers. Birmingham, United Kingdom: Packt Publishing Ltd.
Wang, D., Johnson, A. D., Papp, A. C., Kroetz, D. L., and Sadée, W. (2005). Multidrug resistance polypeptide 1 (MDR1, ABCB1) variant 3435C>T affects mRNA stability. Pharmacogenetics Genomics 15 (10), 693–704. doi:10.1097/01.fpc.0000178311.02878.83
Waskom, M. (2021). seaborn: statistical data visualization. J. Open Source Softw. 6 (60), 3021. doi:10.21105/joss.03021
Woillard, J., de Winter, B. C. M., Kamar, N., Marquet, P., Rostaing, L., and Rousseau, A. (2011). Population pharmacokinetic model and Bayesian estimator for two tacrolimus formulations – twice daily Prograf ® and once daily Advagraf ®. Br. J. Clin. Pharmacol. 71(3), 391–402. doi:10.1111/j.1365-2125.2010.03837.x
Woillard, J.-B., Gatault, P., Picard, N., Arnion, H., Anglicheau, D., and Marquet, P. (2018a). A donor and recipient candidate gene association study of allograft loss in renal transplant recipients receiving a tacrolimus-based regimen. Am. J. Transplant. 18 (12), 2905–2913. doi:10.1111/ajt.14894
Woillard, J.-B., Gatault, P., Picard, N., Arnion, H., Anglicheau, D., and Marquet, P. (2018b). A donor and recipient candidate gene association study of allograft loss in renal transplant recipients receiving a tacrolimus-based regimen. Am. J. Transplant. 18 (12), 2905–2913. doi:10.1111/ajt.14894
Zhang, X., Wang, Z., Fan, J., Liu, G., and Peng, Z. (2011a). Impact of interleukin-10 gene polymorphisms on tacrolimus dosing requirements in Chinese liver transplant patients during the early posttransplantation period. Eur. J. Clin. Pharmacol. 67 (8), 803–813. doi:10.1007/s00228-011-0993-8
Keywords: kidney transplantation, tacrolimus, FK506, C/D ratio, Greek population
Citation: Tsironi A, Lazaros K, Mendrinou E, Papasotiriou M, Siamoglou S, Kydonopoulou K, John A, Gerou A, Gerou S, Ali BR, Vrahatis AG and Patrinos GP (2025) Impact of CYP3A4 and ABCB1 genetic variants on tacrolimus dosing in Greek kidney transplant recipients. Front. Pharmacol. 16:1538432. doi: 10.3389/fphar.2025.1538432
Received: 02 December 2024; Accepted: 27 February 2025;
Published: 19 March 2025.
Edited by:
Luis Abel Quiñones, University of Chile, ChileReviewed by:
Karel Allegaert, KU Leuven, BelgiumErika Cecchin, Aviano Oncology Reference Center (IRCCS), Italy
Copyright © 2025 Tsironi, Lazaros, Mendrinou, Papasotiriou, Siamoglou, Kydonopoulou, John, Gerou, Gerou, Ali, Vrahatis and Patrinos. This is an open-access article distributed under the terms of the Creative Commons Attribution License (CC BY). The use, distribution or reproduction in other forums is permitted, provided the original author(s) and the copyright owner(s) are credited and that the original publication in this journal is cited, in accordance with accepted academic practice. No use, distribution or reproduction is permitted which does not comply with these terms.
*Correspondence: George P. Patrinos, Z3BhdHJpbm9zQHVwYXRyYXMuZ3I=