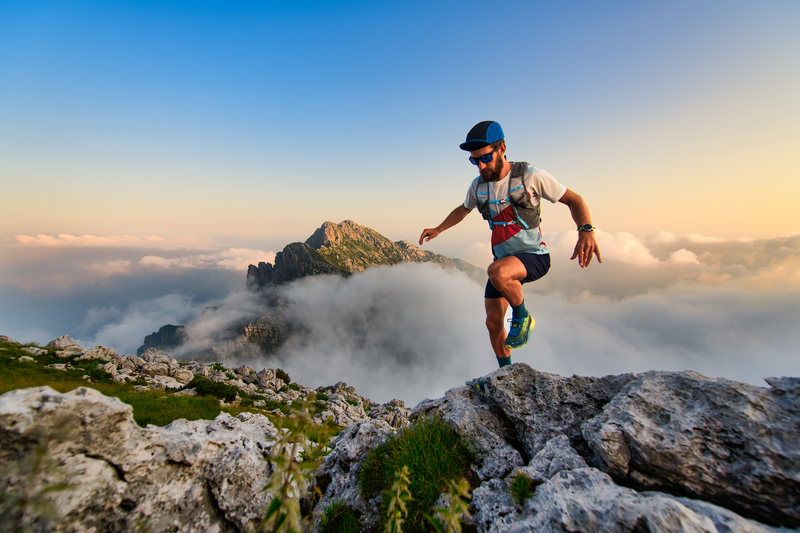
94% of researchers rate our articles as excellent or good
Learn more about the work of our research integrity team to safeguard the quality of each article we publish.
Find out more
ORIGINAL RESEARCH article
Front. Pharmacol.
Sec. Drugs Outcomes Research and Policies
Volume 16 - 2025 | doi: 10.3389/fphar.2025.1534552
This article is part of the Research Topic Clinical Pharmacist Service Promotes the Improvement of Medical Quality Volume II View all 47 articles
The final, formatted version of the article will be published soon.
You have multiple emails registered with Frontiers:
Please enter your email address:
If you already have an account, please login
You don't have a Frontiers account ? You can register here
Background Optimizing prescription practices for surgical patients is crucial due to the complexity and sensitivity of their medication regimens. To enhance medication safety and improve patient outcomes by introducing a machine learning (ML)-based warning model integrated into a pharmacist-led Surgical Medicines Prescription Optimization and Prediction (SMPOP) service. Method A retrospective cohort design with a prospective implementation phase was used in a tertiary hospital. The study was divided into three phases: (1) Data analysis and ML model development (April 1, 2019 to March 31, 2022), (2) Establishment of a pharmacist-led management model (April 1, 2022 to March 31, 2023), and (3) Outcome evaluation (April 1, 2023 to March 31, 2024). Key variables, including gender, age, number of comorbidities, type of surgery, surgery complexity, days from hospitalization to surgery, type of prescription, type of medication, route of administration, and prescriber’s seniority were collected. The data set was divided into training set and test set in the form of 8:2. The effectiveness of the SMPOP service was evaluated based on prescription appropriateness, adverse drug reactions (ADRs), length of hospital stay, total hospitalization costs, and medication expenses. Results In Phase 1, 6,983 prescriptions were identified as potential prescription errors (PPEs) for ML model development, with 43.9% of them accepted by prescribers. The Random Forest (RF) model performed the best (AUC = 0.893) and retained high accuracy with 12 features (AUC = 0.886). External validation showed an AUC of 0.786. In Phase 2, SMPOP services were implemented, which effectively promoted effective communication between pharmacists and physicians and ensured the successful implementation of intervention measures. The SMPOP service was fully implemented. In Phase 3, the acceptance rate of pharmacist recommendations rose to 71.3%, while the length of stay, total hospitalization costs, and medication costs significantly decreased (p < 0.05), indicating overall improvement compared to Phase 1. Conclusion SMPOP service enhances prescription appropriateness, reduces ADRs, shortens stays, and lowers costs, underscoring the need for continuous innovation in healthcare.
Keywords: Medication Errors, machine learning, Pharmacists, Patient Safety, Surgical Procedures
Received: 26 Nov 2024; Accepted: 25 Feb 2025.
Copyright: © 2025 Li, Yue, Zhang, Zheng and Shang. This is an open-access article distributed under the terms of the Creative Commons Attribution License (CC BY). The use, distribution or reproduction in other forums is permitted, provided the original author(s) or licensor are credited and that the original publication in this journal is cited, in accordance with accepted academic practice. No use, distribution or reproduction is permitted which does not comply with these terms.
* Correspondence:
Nan Shang, First Hospital of Shanxi Medical University, Taiyuan, China
Disclaimer: All claims expressed in this article are solely those of the authors and do not necessarily represent those of their affiliated organizations, or those of the publisher, the editors and the reviewers. Any product that may be evaluated in this article or claim that may be made by its manufacturer is not guaranteed or endorsed by the publisher.
Research integrity at Frontiers
Learn more about the work of our research integrity team to safeguard the quality of each article we publish.