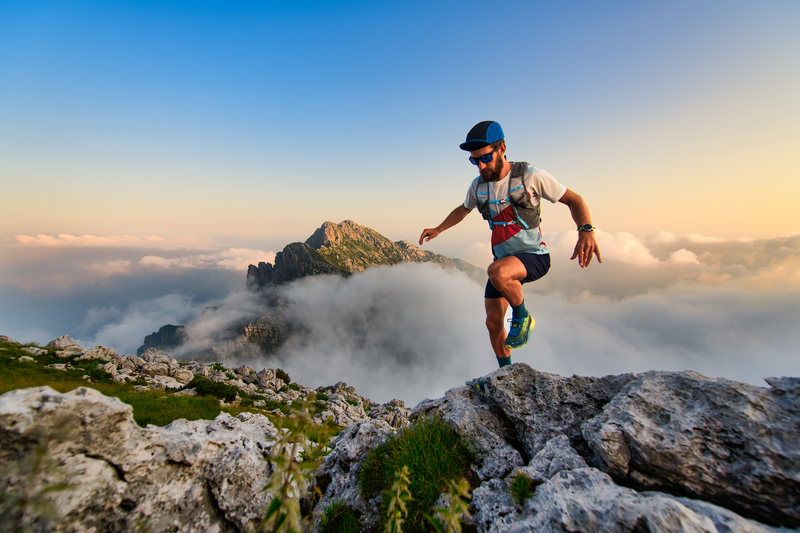
94% of researchers rate our articles as excellent or good
Learn more about the work of our research integrity team to safeguard the quality of each article we publish.
Find out more
REVIEW article
Front. Pharmacol.
Sec. Ethnopharmacology
Volume 16 - 2025 | doi: 10.3389/fphar.2025.1526187
This article is part of the Research Topic Artificial Intelligence in Traditional Medicine Research and Application View all 8 articles
The final, formatted version of the article will be published soon.
You have multiple emails registered with Frontiers:
Please enter your email address:
If you already have an account, please login
You don't have a Frontiers account ? You can register here
Traditional Chinese medicine is a complex medical system characterized by multiple metabolites, targets, and pathways, known for its low drug resistance and significant efficacy. However, challenges persist within Traditional Chinese medicine, including difficulties in assessing the quality of Botanical drugs, reliance on experiential knowledge for disease diagnosis and treatment, and a lack of clarity regarding the pharmacological mechanisms of Traditional Chinese medicine. The advancement of digital intelligence technology is driving a shift towards precision medicine within the Traditional Chinese medicine model. This transition propels Traditional Chinese medicine into an era of precision, intelligence, and digitalization. This paper introduces standard digital intelligence technologies and explores the application of digital intelligence technologies in quality control and evaluation of Traditional Chinese medicine, studies the research status of digital intelligence technologies in assisting diagnosis, treatment and prevention of diseases, and further promotes the application and development of digital intelligence technologies in the field of Traditional Chinese medicine.
Keywords: artificial intelligence, ANN: Artificial neural network, BP: Backpropagation, CNN: Convolutional Neural Network, GC: gas chromatography, GCN: Graphical Convolutional Neural Network, KNN: K-Nearest Neighbour, LC-MS: Liquid Chromatography-Mass Spectrometry
Received: 11 Nov 2024; Accepted: 20 Mar 2025.
Copyright: © 2025 Zhu, liu and gao. This is an open-access article distributed under the terms of the Creative Commons Attribution License (CC BY). The use, distribution or reproduction in other forums is permitted, provided the original author(s) or licensor are credited and that the original publication in this journal is cited, in accordance with accepted academic practice. No use, distribution or reproduction is permitted which does not comply with these terms.
* Correspondence:
xiaonan liu, Shandong University of Traditional Chinese Medicine, Jinan, China
Disclaimer: All claims expressed in this article are solely those of the authors and do not necessarily represent those of their affiliated organizations, or those of the publisher, the editors and the reviewers. Any product that may be evaluated in this article or claim that may be made by its manufacturer is not guaranteed or endorsed by the publisher.
Research integrity at Frontiers
Learn more about the work of our research integrity team to safeguard the quality of each article we publish.