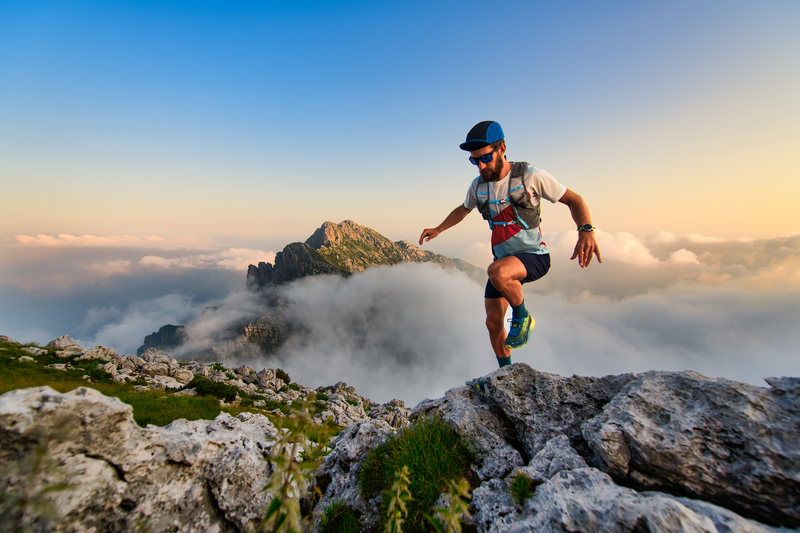
95% of researchers rate our articles as excellent or good
Learn more about the work of our research integrity team to safeguard the quality of each article we publish.
Find out more
ORIGINAL RESEARCH article
Front. Pharmacol.
Sec. Pharmacogenetics and Pharmacogenomics
Volume 16 - 2025 | doi: 10.3389/fphar.2025.1524623
This article is part of the Research Topic Genetic and Pharmacological Frontiers in Cancer Treatment View all articles
The final, formatted version of the article will be published soon.
You have multiple emails registered with Frontiers:
Please enter your email address:
If you already have an account, please login
You don't have a Frontiers account ? You can register here
Introduction: Thyroid cancer, a prevalent endocrine malignancy, has an age-standardized incidence rate of 9.1 per 100,000 people and a mortality rate of 0.44 per 100,000 as of 2024. Despite significant advances in precision oncology driven by large-scale international consortia, gaps persist in understanding the genomic landscape of thyroid cancer and its impact on therapeutic efficacy across diverse populations. Methods: To address this gap, we performed comprehensive data mining and in silico analyses to identify pathogenic variants in thyroid cancer driver genes, calculate allele frequencies, and assess deleteriousness scores across global populations, including African, Amish, Ashkenazi Jewish, East and South Asian, Finnish and non-Finnish European, Latino, and Middle Eastern groups. Additionally, pharmacogenomic profiling, in silico drug prescription, and clinical trial data were analyzed to prioritize targeted therapeutic strategies. Results: Our analysis examined 56,622 variants in 40 thyroid cancer-driver genes across 76,156 human genomes, identifying 5,001 known and predicted oncogenic variants. Enrichment analysis revealed critical pathways such as MAPK, PI3K-AKT-mTOR, and p53 signaling, underscoring their roles in thyroid cancer pathogenesis. High-throughput validation strategies confirmed actionable genomic alterations in RET, BRAF, NRAS, KRAS, and EPHA7. Ligandability assessments identified these proteins as promising therapeutic targets. Furthermore, our findings highlight the clinical potential of targeted drug inhibitors, including vandetanib, dabrafenib, and selumetinib, for improving treatment outcomes. Discussion: This study underscores the significance of integrating genomic insights with pharmacogenomic strategies to address disparities in thyroid cancer treatment. The identification of population-specific oncogenic variants and actionable therapeutic targets provides a foundation for advancing precision oncology. Future efforts should focus on including underrepresented populations, developing population-specific prevention strategies, and fostering global collaboration to ensure equitable access to pharmacogenomic testing and innovative therapies. These initiatives have the potential to transform thyroid cancer care and align with the broader goals of personalized medicine.
Keywords: Thyroid cancer, Pathogenic variants, allele frequencies, Drug prescription, global populations, Pharmacogenomic testing, precision oncology
Received: 07 Nov 2024; Accepted: 01 Apr 2025.
Copyright: © 2025 Espinoza, Echeverría-Garcés, Rivera-Orellana, Bueno- Miño, Castellanos-Molina, Benítez-Núñez and López-Cortés. This is an open-access article distributed under the terms of the Creative Commons Attribution License (CC BY). The use, distribution or reproduction in other forums is permitted, provided the original author(s) or licensor are credited and that the original publication in this journal is cited, in accordance with accepted academic practice. No use, distribution or reproduction is permitted which does not comply with these terms.
* Correspondence:
Andrés López-Cortés, Universidad de las Américas, Quito, Pichincha, Ecuador
Disclaimer: All claims expressed in this article are solely those of the authors and do not necessarily represent those of their affiliated organizations, or those of the publisher, the editors and the reviewers. Any product that may be evaluated in this article or claim that may be made by its manufacturer is not guaranteed or endorsed by the publisher.
Research integrity at Frontiers
Learn more about the work of our research integrity team to safeguard the quality of each article we publish.