- 1Department of Pharmacy, The First Affiliated Hospital of Fujian Medical University, Fuzhou, China
- 2Department of Pharmacy, National Regional Medical Center, Binhai Campus of the First Affiliated Hospital, Fujian Medical University, Fuzhou, China
Objective: This study aimed to compare the hypoglycemic effects of four SGLT2 inhibitors (dapagliflozin, canagliflozin, empagliflozin, and ipragliflozin), simulate the 24-h urinary glucose excretion (UGE) of these inhibitors in T2DM patients with renal insufficiency, and investigate optimal dosage regimen for the SGLT2 inhibitor in these patients.
Method: We established a physiologically based pharmacokinetic (PBPK) model of SGLT2 inhibitors using the PK-Sim software, and the renal physiological tissue structure was expanded to include renal tubules using the MoBi software. The PBPK/PD (pharmacodynamics) model of SGLT2 inhibitors was validated following comparison of the observed plasma concentration and pharmacokinetic parameters.
Result: The model simulation results showed that 71.4% of the predicted pharmacokinetic parameters AUC (area under the curve) and Cmax (peak concentration) closely matched the observed values within 0.8–1.3 folds accuracy. Further, 83.9% of the predicted concentration-time curves and 84.65% of the predicted 24-h urinary glucose excretion aligned with the observed data points within 0.5–2 folds accuracy. The MPE, AFE and AAFE values for all concentration-time data points were 0.90, 1.07 and 1.08, indicating that the predictive performance of the PBPK/PD model was robust and reliable. It was predicted that optimal hypoglycemic effects would be achieved in T2DM patients with mild, moderate, and severe renal insufficiency, when treated with ipragliflozin 50 mg qd, dapagliflozin 10 mg qd or canagliflozin 100 mg qd, empagliflozin 10 mg, respectively.
Conclusion: This study provided a scientific basis for optimizing the dosage regimen in T2DM patients with renal insufficiency.
1 Introduction
The proximal convoluted tubule (PCT) in the kidney contains two sodium-glucose co-transporters, SGLT1 and SGLT2. These co-transporters are responsible for the reabsorption of approximately 99% of the glomerular filtered glucose. SGLT2 inhibitors are a new class of hypoglycemic agents that compete with glucose for the SGLT2 transporter to lower blood glucose levels, change threshold to concentrations, and enhance urinary glucose excretion (UGE) in patients with type 2 diabetes mellitus (T2DM) (Maurer et al., 2011). At present, a variety of SGLT2 inhibitors have been approved at home and abroad for managing blood glucose in patients with T2DM. For example, dapagliflozin, canagliflozin, and empagliflozin have been approved for market sale by the European Medicines Agency and the U.S. Food and Drug Administration. Ipragliflozin, luseogliflozin, and tofogliflozin also have been submitted to the Pharmaceuticals and Medical Devices Agency in Japan (Garcia-Ropero et al., 2018).
Diabetes mellitus (DM) is a common clinical disease, with 20%–40% of diabetic patients eventually developing chronic kidney disease (CKD). The kidneys of patients with CKD undergo structural and functional damage, and their glomerular filtration rate (GFR) progressively decreases (Devineni et al., 2015). Impaired renal function affects the pharmacokinetics (PK) and pharmacodynamics (PD) of SGLT2 inhibitors. Therefore, special attention should be given to their hypoglycemic effect and safety in patients with CKD and diabetic nephropathy (Khurana et al., 2015). Studies have shown that the area under the curve (AUC) of SGLT2 inhibitors in patients with T2DM and renal insufficiency increases as renal function declines, compared with patients with T2DM and normal renal function. For example, the AUC of canagliflozin increases by 15%, 29%, and 53% in patients with T2DM and mild, moderate, and severe renal insufficiency, respectively (Khurana et al., 2015). Similarly, ipragliflozin shows an increase in AUC of 40% and 47% in patients with T2DM and moderate and severe renal insufficiency, respectively (Scheen, 2015). The hypoglycemic effect of SGLT2 inhibitors decreases with declining renal function in patients with T2DM. For example, in patients with mild, moderate, and severe renal insufficiency, the UGE of dapagliflozin decreases by 42%, 83%, and 84%, respectively (Kasichayanula et al., 2013), while that of empagliflozin decreases by 37%, 43%, and 81%, respectively (Scheen, 2015).
Besides, there are significant differences in the efficacy of different SGLT2 inhibitors among patients with T2DM and normal renal function. Zaccardi et al. conducted a meta-analysis of 38 randomized controlled trials involving 23,997 participants to evaluate the efficacy and safety of SGLT2 inhibitors in adults with T2DM (Zaccardi et al., 2016). They found that, compared to other SGLT2 inhibitors (canagliflozin 100 mg, dapagliflozin 5 or 10 mg, and empagliflozin 10 or 25 mg), canagliflozin 300 mg significantly reduced HbA1c levels and fasting glucose in patients with T2DM. Similarly, Pinto et al. also concluded that canagliflozin 300 mg showed better efficacy than other SGLT2 inhibitors, empagliflozin 25 mg was more effective than dapagliflozin 10 mg, and empagliflozin (10 mg and 25 mg) showed similar efficacy to canagliflozin 100 mg (Pinto et al., 2022). However, to date, no literature or clinical studies have reported differences in efficacy among different SGLT2 inhibitors in patients with T2DM and renal insufficiency. Moreover, the application of SGLT2 inhibitors in patients with T2DM and renal insufficiency is limited. For example, dapagliflozin and empagliflozin are recommended for patients with eGFR >45 mL/min/1.73 m2, while canagliflozin can be used in patients with eGFR >30 mL/min/1.73 m2 (Grempler et al., 2012; Washburn and Poucher, 2013). Therefore, the dosage regimen of SGLT2 inhibitors in patients with T2DM and different degrees of CKD require further clarification.
In recent years, the PBPK model has developed rapidly in the academic and pharmaceutical fields, becoming integral to the drug development process (Kuepfer et al., 2016). The PBPK model demonstrates robust predictive ability by prospectively simulating concentration-time curves in the specific compartments. It quantitatively characterizes drug exposure at the site of action and investigates the pharmacological effects on specific organs or tissues. Initially established and verified in healthy subjects, the PBPK model then adjusts relevant physiological parameters for application in specific populations. This sequential approach yields PK/PD data for diverse populations. The extrapolation of the PBPK model transforms exploratory trials requiring a large number of subjects into confirmatory trials requiring only a small number of subjects. This approach improves trial efficiency and safety, reduces costs, shortens trial durations, and realizes the development of reasonably optimized dosage regimens under various pathological conditions (Perez-Urizar et al., 2000).
The purposes of this study were twofold. First, we aimed to construct a PBPK model for SGLT2 inhibitors combined with the mathematical model of UGE, and simulate and validate the PK and PD of SGLT2 inhibitors in both healthy subjects and T2DM patients with renal insufficiency. Second, we aimed to compare the hypoglycemic effects of four SGLT2 inhibitors (dapagliflozin 5 mg and 10 mg, canagliflozin 100 mg and 300 mg, empagliflozin 10 mg and 25 mg, and ipragliflozin 50mg and 100 mg), simulate the 24-h UGE of these inhibitors in patients with T2DM and renal insufficiency, and investigate optimal dosage regimen for the SGLT2 inhibitor in these patients.
2 Methods
2.1 The modeling process
First, using PK-Sim® and MoBi® version 11 software programs produced by Open Systems Pharmacology Suite/Leverkusen (Germany), we established the SGLT2-inhibitor PBPK/PD model in healthy subjects. Subsequently, age, weight, BMI, and other parameters were adjusted using the built-in algorithm of the PK-Sim software to obtain the physiological parameters of patients with T2DM, and establish the SGLT2-inhibitor PBPK/PD model of patients with T2DM and normal renal function. Then, by setting the GFR of virtual patients combined with the renal insufficiency tool in the PK-Sim software, the PBPK/PD model was extrapolated to patients with T2DM and renal insufficiency. The observed values validated the SGLT2-inhibitor PBPK/PD model. The clinically measured PK/PD data was obtained by GetData Graph Digitizer version 2.26 (GetData software development company, Montgomery St, Kogarah NSW, Australia) and plotted by OriginLab Origin® 2024 (MicroCal, Northampton, Massachusetts, USA). The modeling process was shown in Figure 1.
2.2 Pharmacokinetic (PK)/pharmacodynamic (PD) data
We searched for scientific papers related to dapagliflozin, canagliflozin, empagliflozin, and ipragliflozin on the PubMed and Google Scholar websites, and information was collected on hypoglycemic therapy with SGLT2 inhibitors alone in patients with T2DM and healthy subjects. Specifically, we extracted: (1) number of subjects, therapeutic regimens, and doses; (2) plasma drug-time curve data points; (3) 24-h urinary glucose excretion; and (4) disease status (healthy or T2DM) and renal function status (Supplementary Table S1).
2.3 Establishment and validation the SGLT2-inhibitor PBPK model
Physicochemical parameters (lipophilicity, solubility, and molecular weight, among others) of the drug and the affinity parameters of SGLTs for glucose were obtained from the literature and DrugBank database and were input into the PK-Sim software to construct the SGLT2-inhibitor PBPK model (Drugbank, 2024; Drugbank, 2025a; Drugbank, 2025b; Drugbank, 2025c) (Table 1).
2.3.1 The PBPK model of healthy subjects
The SGLT2 inhibitors were primarily metabolized into inactive products by UDP-glycosyltransferases (such as UGT1A9, UGT2B4, and UGT2B7), and a few SGLT2 inhibitors—such as dapagliflozin and canagliflozin—were metabolized into inactive products by cytochrome P450 enzymes (like CYP3A4, CYP2C9, CYP2D6, among others). The SGLT2 inhibitors and their inactive metabolites were mainly excreted in the feces and urine, with a small amount of the drug excreted in the urine as a prototype drug.
The modeling parameters from Mori K et al. and Callegari E et al. were incorporated into the PBPK model of dapagliflozin (Callegari et al., 2021; Mori et al., 2016). Further, we verified the reliability of the dapagliflozin PBPK model using the clinical measured data sets of single and multiple oral doses of 10 and 20 mg of dapagliflozin (Kasichayanula et al., 2014). According to the clinical pharmacokinetic studies in healthy subjects, the liver clearance (CL) values for empagliflozin and ipragliflozin were 0.09 and 0.16 L/h/kg, respectively (Veltkamp et al., 2011; Zhang et al., 2023). We combined the parameter identification modules of PK-Sim software with clinical measurement data sets to optimize the gastrointestinal permeability coefficients and cell permeability coefficients of empagliflozin and ipragliflozin.
Previous mass balance studies showed that canagliflozin is mainly metabolized via glucuronidation by UGT1A9 and UGT2B4 to two inactive O-glucuronide metabolites, and has only minor (7% in humans) metabolism by CYP3A4. For the contribution to glucuronidation, the relative contribution rates of UGT1A9 and UGT2B4 metabolism were 83% and 17%, respectively (Scheen, 2014). Utilizing the PK-Sim software and based on clinical data from Devineni D, et al., where healthy subjects were administered a 300 mg intravenous dose of canagliflozin, the total hepatic clearance was calculated to be 1.4 L/μmol/min (Devineni et al., 2015b). Subsequently, by integrating the relative contributions of hepatic enzymes and applying the formula CLUGT1A9 or UGT2B4 = hepatic clearance rate in healthy subjects * relative contribution rate of hepatic enzymes, the clearances for UGT1A9 and UGT2B4 were found to be 1.2 L/μmol/min and 0.14 L/μmol/min, respectively. The extra clearance rate, CLOther Enzyme, which represents the metabolic action of CYP3A4, is calculated using the formula CLOther Enzyme = CLhealthy - CLUGT1A9 - CLUGT2B4, yielding a value of 0.06 L/μmol/min. In addition, canagliflozin was a P-glycoprotein (P-gp) substrate, based on clinical drug interaction study with cyclosporine, the Km and Kcat values of P-gp were optimized to be 0.19 μmol/L and 2.4 min−1, respectively (Devineni et al., 2015c).
2.3.2 The PBPK model of T2DM patient with normal renal function
The age, weight, and BMI of patients with T2DM and normal renal function were set according to the demographic characteristics of the patients in the clinical trial (Supplementary Table S1). Meanwhile, the corresponding physiological parameters of the virtual patients were generated using the built-in algorithm of the software to establish and verify the PBPK model of patients with T2DM.
2.3.3 The PBPK model of patients with T2DM and renal insufficiency
After verifying the predictive performance of the PBPK model of SGLT2 inhibitors in healthy subjects and patients with T2DM and normal renal function, the corresponding GFR value was set according to the observed values of patients with renal insufficiency. The physiological parameters were generated by the built-in database of the model, and the PBPK model was extrapolated to the population of patients with T2DM and renal insufficiency. Subsequently, the PBPK model was validated using clinically measured data.
2.4 Establishment and validation the SGLT2-inhibitor PD model
In patients with T2DM, glucose that remained after glomerular filtration was distributed along the proximal tubules, and the excess glucose that accumulated in the bladder was subsequently excreted in the urine (Zhang et al., 2023). Using MoBi software, the physiological structure of the kidney tissue was extended to include the renal tubules, which were divided into three segments: the proximal renal tubules (S1), the distal renal tubules and collecting tubules (S2), and the bladder lumen (S3). The PD model of SGLT2 inhibition incorporated the physiological parameters related to renal tubules (Supplementary Table S2) and used Supplementary Equations S1–S7 to describe the changes in glucose concentration in the proximal renal tubule and to calculate the urinary glucose excretion over time (Wang et al., 2022). The detailed modeling process for the PD model is described in the “supplementary methods” section of the supplementary materials.
The predicted drug-concentration-time curve was compared to the observed data set, and the fold error between the predicted and observed values of the PK parameters (AUC, Cmax) was obtained. Subsequently, the predictive performance of the PBPK/PD model for SGLT2 inhibitors was comprehensively evaluated. The fold error was calculated was calculated by Equation 1. If the fold error was between 0.5 and 2.0, the predictive performance of the model was considered acceptable (Huang and Rowland, 2012). In addition, Equations 2–4 were used to calculate the mean prediction error (MPE), the average folding error (AFE) and the absolute average folding error (AAFE) of all concentration-time data points to assess the precision and the bias of the model.
where “Predicted” represents the predicted value and “Observed” represents the clinically measured value or the observed value.
2.5 Based on the PBPK/PD model of SGLT2-inhibitor to explore dosage regimen in patients with renal insufficiency
The PBPK/PD model of SGLT2 inhibitors was used to predict 24 h urinary glucose excretion in patients with T2DM and renal insufficiency following administration of the daily dose of each SGLT2 inhibitor (dapagliflozin 5 mg and 10 mg, canagliflozin 100 mg and 300 mg, empagliflozin 10 mg and 25 mg, and ipragliflozin 50 mg and 100 mg). After comparing the four SGLT2 inhibitors, the hypoglycemic effects were used to determine the recommended optimal dosage regimen for patients with T2DM and renal insufficiency. Considering the 30-year-old European T2DM virtual patient population as an example, the simulated sample was set to 100 people, 50% of whom were men, and the average GFR of patients with normal renal function and mild, moderate, and severe renal insufficiency were 105, 75, 45, and 15 mL/min, respectively.
2.6 Population simulation
The influence of individual variations in the population physiological parameters on the simulation results were investigated using the PK-Sim population simulation block. The demographic characteristics of the virtual population were consistent with clinically measured data from the literature, and the individual parameters of the virtual subjects were randomly generated using the distribution range defined by the PK-Sim software. The simulated sample was set to 1,000 people, 50% of whom were male. The demographic characteristics of the simulated population are shown in Supplementary Table S1. The dosage regimen of the virtual subjects was consistent with that reported in the literature (Veltkamp et al., 2011; Zhang et al., 2023). The predictive performance of the model was further validated by evaluating whether the clinically measured values fell within the 90% probability lines of the predicted drug concentration-time curve of the model.
3 Results
3.1 Establishment and validation of the SGLT2-inhibitor PBPK model
3.1.1 The PBPK model of healthy subjects and patients with T2DM and normal renal function
This study successfully established and validated PBPK models for dapagliflozin, canagliflozin, empagliflozin, and ipragliflozin. The results showed that 86.5% of the predicted concentration-time curves between the clinical measured data points were within 0.5–2 folds (Figure 2A), and 76.8% of the predicted pharmacokinetic parameters (AUC, Cmax) of the SGLT2 inhibitors between the observed values were within 0.8–1.3 folds (Figures 2D, E; Table 2). The MPE, AFE and AAFE values for concentration-time data points in healthy subjects and patients with T2DM and normal renal function were 0.73, 1.11 and 1.16, respectively.
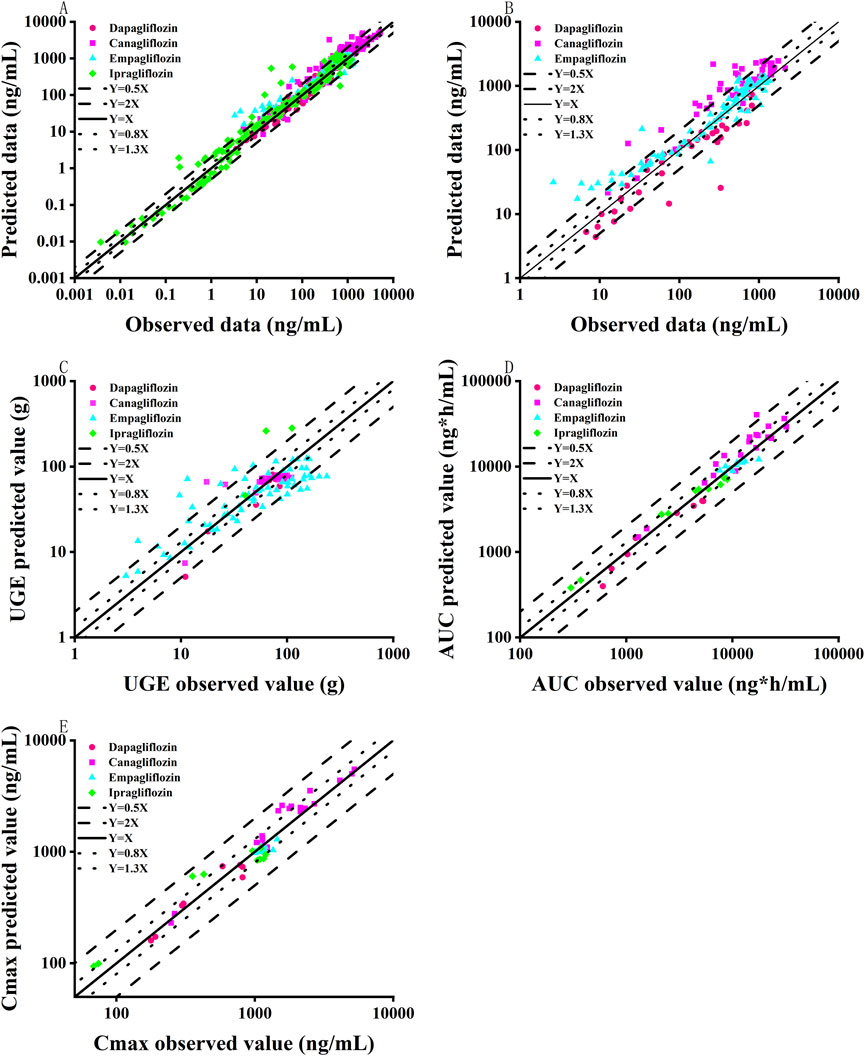
Figure 2. Goodness-of-fit plot of SGLT2 inhibitor PBPK/PD model predictions with measured data points. (A) Comparison of drug concentration-time curve fitting in patients with normal renal function; (B) Comparison of drug concentration-time curve fitting in patients with renal insufficiency; (C) Urinary glucose excretion; (D) Pharmacokinetic parameter AUC; (E) Pharmacokinetic parameter Cmax; Black solid line represents multiple error of 1-fold; Blue dotted line represents 0.8–1.3 folds; Black lines represent 0.5-2 folds.
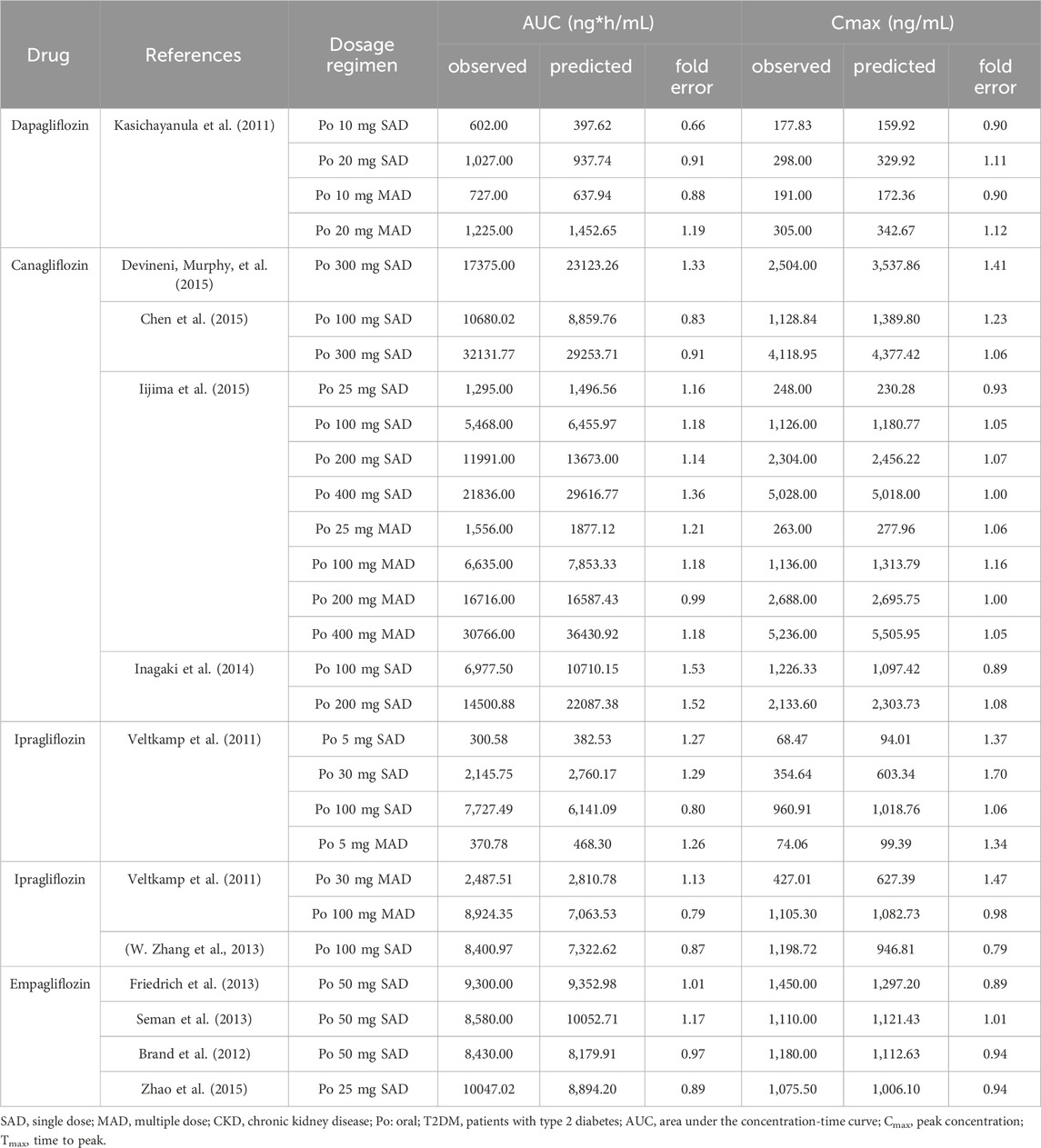
Table 2. Comparison of predicted and observed pharmacokinetic parameters in healthy subjects and T2DM patients with normal renal function.
3.1.2 The PBPK model of patients with T2DM and renal insufficiency
After verifying the predictive performance of the SGLT2 inhibitor PBPK model in healthy subjects and patients with T2DM and normal renal function, the PBPK model was extrapolated to patients with T2DM and renal insufficiency by adjusting the GFR and physiological parameters of virtual T2DM patients according to clinical-patient mean values. As shown in Figure 2B and Table 3, 80.2% of the predicted concentration-time curves between the clinically measured data points were within 0.5–2 folds, and 64.3% of the predicted SGLT2-inhibitor pharmacokinetic parameters (AUC, Cmax, etc.) between the measured values were within 0.8–1.3 folds. In patients with T2DM and renal insufficiency, the MPE, AFE and AAFE values for concentration-time data points were 0.90, 1.07 and 1.08, respectively.
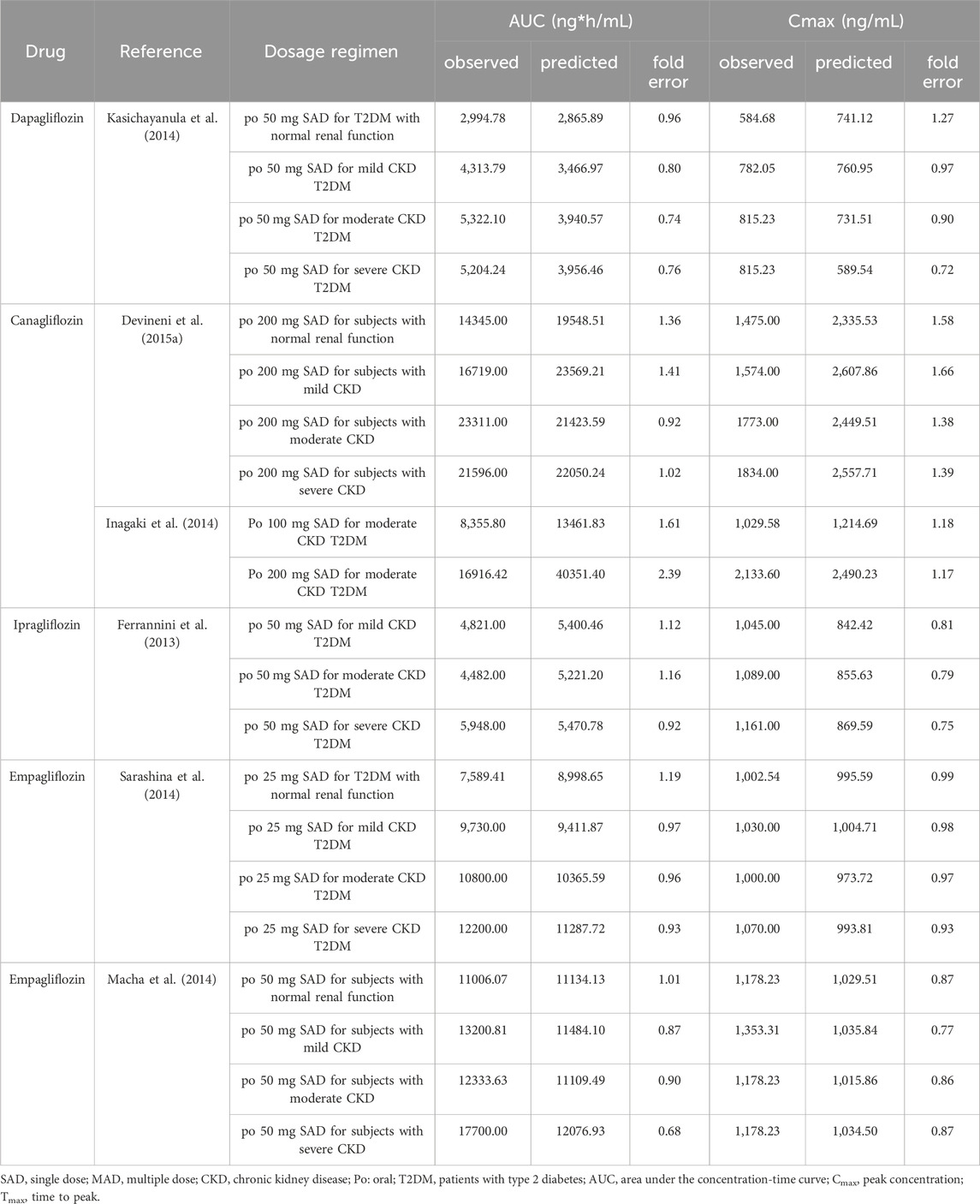
Table 3. Comparison of predicted and observed pharmacokinetic parameters in subjects with normal renal function and T2DM patients with renal insufficiency.
The performance of the model, extraction of measured data, physiological changes in the process of drug administration, and accuracy of the sampling time points may have affected the prediction results. Overall, the predicted concentration-time curves were close to the observed values; specifically, 71.4% of the predicted pharmacokinetic parameters (AUC, Cmax) between the clinically measured values were within 0.8–1.3 folds, and 84.65% of the predicted concentration-time curves between the clinically measured data points were within 0.5–2 folds. The MPE, AFE and AAFE values for all concentration-time data points were 0.90, 1.07 and 1.08. Therefore, the predictive performance of the SGLT2-inhibitor PBPK/PD model was acceptable.
3.2 Establishment and validation of the SGLT2-inhibitor PD model
The SGLT2-inhibitor PD model was established and validated in healthy subjects and normal and renal impaired patients with T2DM. As shown in Figure 2C, 80.2% of the predicted 24-h urinary glucose excretion values were within 0.5–2 folds. The MPE, AFE and AAFE values for 24-h urinary glucose excretion values were −0.11, 0.76 and 1.41, respectively.
3.3 Based on the PBPK/PD model of SGLT2-inhibitor to explore dosage regimen in patients with renal insufficiency
The SGLT2-inhibitor PBPK/PD model was used to predict the concentration-time curve and UGE in patients with T2DM and normal renal function or renal insufficiency. The SGLT2-inhibitor PD model predicted the 24-h UGE in patients with T2DM using the daily dose of SGLT2 inhibitors, as shown in Figure 3 and Table 4. The hypoglycemic effects of the four SGLT2 inhibitors were as follows: (1) ipragliflozin 50 mg qd and 100 mg qd > empagliflozin 25 mg qd > empagliflozin 10 mg qd > canagliflozin 300 mg qd > canagliflozin 100 mg qd > dapagliflozin 10 mg qd > dapagliflozin 5 mg qd in T2DM patients with normal renal function; (2) ipragliflozin 50 mg qd and 100 mg qd > empagliflozin 25 mg qd > empagliflozin 10 mg qd > canagliflozin 300 mg qd > canagliflozin 100 mg qd > dapagliflozin 10 mg qd > dapagliflozin 5 mg qd in T2DM patients with mild renal insufficiency; (3) dapagliflozin 10 mg qd > canagliflozin 100 mg qd > dapagliflozin 5 mg qd > canagliflozin 300 mg qd > empagliflozin 10 mg qd and 25 mg qd > ipragliflozin 50mg and 100 mg qd in patients with T2DM and moderate renal insufficiency; (4) empagliflozin 10 mg qd and 25 mg qd > canagliflozin 100 mg qd > dapagliflozin 10 mg qd > canagliflozin 300 mg qd > dapagliflozin 5 mg qd > ipragliflozin 100 mg qd > ipragliflozin 50 mg qd in patients with T2DM and severe renal insufficiency.
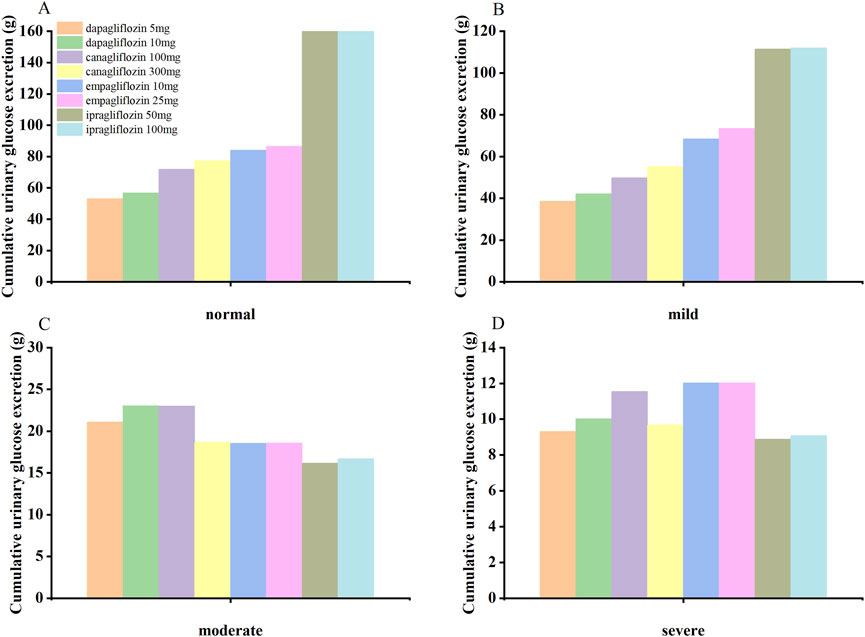
Figure 3. The SGLT2 inhibitor PBPK/PD model predicted the cumulative 24-hour urinary glucose excretion in T2DM patients with normal renal function (A) and mild (B), moderate (C), and severe (D) renal insufficiency.
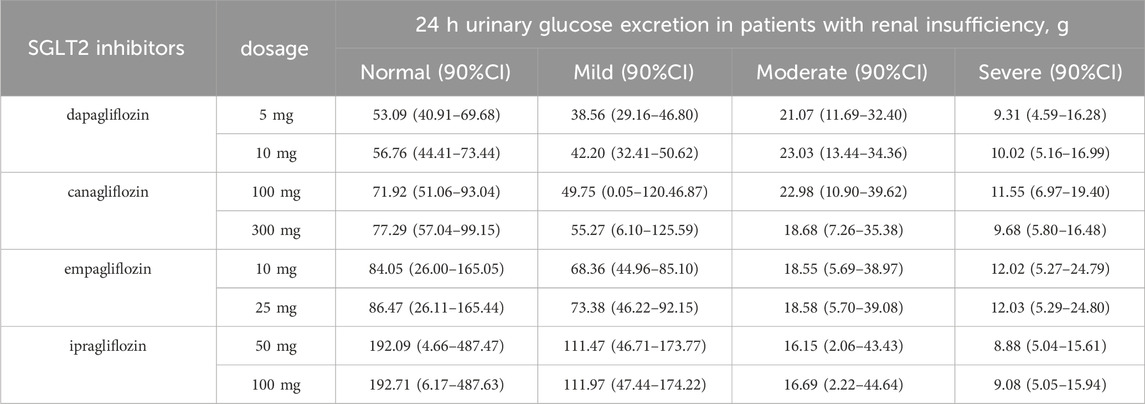
Table 4. Based on the PBPK/PD model of SGLT2 inhibitors predicted the 24-h urinary glucose excretion in type 2 diabetic patients with renal insufficiency.
3.4 Population simulation
According to the demographic characteristics of the clinical patients and the dosage regimen, the PBPK/PD model of SGLT2 inhibitors simulated the concentration-time curve and 24-h UGE in patients with T2DM. As shown in Supplementary Figures S1–S10, 81% of the clinically measured points in the concentration-time curve and 85% of the observed values of UGE were within the 90% confidence interval of the virtual population. The simulation results suggested that the PBPK/PD model of SGLT2 inhibitors had a high predictive performance.
4 Discussions
Studies have shown that SGLT2 inhibitors could benefit patients with CKD and show promise for treating patients with T2DM and renal insufficiency (Fioretto et al., 2018; Mende, 2022). However, the full potential of SGLT2 inhibitors in CKD remains underexplored. The differences in the efficacy of different SGLT2 inhibitors in patients with T2DM and renal insufficiency have not been reported. In this study, we constructed a PBPK model of SGLT2 inhibitors, combined with a mathematical model of UGE, to simulate and predict changes in the PK and PD of SGLT2 inhibitors in healthy subjects and patients with T2DM and renal insufficiency. We recommended dosage regimens for patients with renal insufficiency to achieve the lowest dose and the maximum therapeutic effect from the perspective of quantitative system pharmacology. It was predicted that optimal hypoglycemic effects would be achieved in T2DM patients with mild, moderate, and severe renal insufficiency, when treated with ipragliflozin 50 mg qd, canagliflozin 100 mg qd or dapagliflozin 10 mg qd, empagliflozin 10 mg, respectively. The PBPK/PD model of the SGLT2 inhibitor simulation results further verified the conclusion of clinical studies by Pinto et al. (2022), Zaccardi et al. (2016). This result provides guidance for selecting SGLT2 inhibitors in patients with T2DM and renal insufficiency, however, it still needs to be verified in controlled clinical trials.
Hummel et al. reported the binding and dissociation rate constants (Kd and Koff) values of Dapagliflozin for SGLT1 and SGLT2. Grempler et al. studied the inhibitory efficacy and selectivity of different SGLT2 inhibitors on the transporter SGLTs (Grempler et al., 2012; Hummel et al., 2012). The selectivity affinity of canagliflozin, empagliflozin, and ipragliflozin for SGLT1 was 0.5 times, 6 times, and 2 times that of dapagliflozin, respectively, and canagliflozin and ipragliflozin were more inhibitory than dapagliflozin against SGLT2 with 2 and 4.4 times stronger inhibition strengths, respectively. The SGLT1 Kd values of canagliflozin, empagliflozin, and ipragliflozin were set to 1.25 mol/L, 15 mol/L, and 5 mol/L, respectively. The SGLT2 Kd values of canagliflozin and ipragliflozin were set to 3.14 * 10–3 μmol/L and 6.93 * 10–3 μmol/L, respectively (Table 1). The PD model of empagliflozin overestimated urinary glucose excretion in patients with T2DM 1–3 h after 25 mg empagliflozin administration, which may be due to differences in the in vivo and in vitro binding rate constants of empagliflozin to the SGLT2 transporter (Grempler et al., 2012).
Evidence supporting the use of SGLT2 inhibitors in patients with severe CKD is limited due to increased risks of adverse reactions like diabetic ketoacidosis, hypovolemia, and acute kidney injury in diabetic patients, making it often contraindicated in those with severe renal insufficiency (GFR <30 mL/min/1.73 m2) (Qiu et al., 2021). Nonetheless, a number of published controlled clinical trials have confirmed that CKD patients show good tolerance to SGLT2 inhibitors, such as, CREDENCE (ClinicalTrials.gov: NCT02065791), DAPA-CKD (ClinicalTrials.gov: NCT03036150), EMPA-KIDNEY (ClinicalTrials.gov: NCT03594110), significantly delaying the onset and development of diabetic nephropathy, reducing mortality from end-stage renal disease, and showing sustained benefits with long-term treatment (Heerspink et al., 2020; Perkovic et al., 2019; The et al., 2023). In our study, using a PBPK/PD model of SGLT2 inhibitors, the PK/PD of patients with T2DM and severe renal insufficiency were analyzed and compared for efficacy. We propose that in urgent cases where the use of SGLT2 inhibitors is necessary, empagliflozin 10 mg qd should be considered to achieve an optimal hypoglycemic effect.
An interesting finding from this study is that the decreased reabsorption capacity of SGLT transporters is a significant factor contributing to the reduced efficacy of SGLT2 inhibitors in patients with T2DM and renal insufficiency. Using the PBPK/PD model of SGLT2 inhibitors, our study analyzed the reabsorption capacity of the SGLT transporter system in patients with CKD and found that in patients with T2DM and moderate or severe renal insufficiency, the reabsorption capacity of the SGLT1 transporter decreased by 15% and 50%, respectively, while that of the SGLT2 transporter decreased by 60% and 90%, respectively (see Supplementary Table S3). Nakamura et al. findings also suggest that the impaired reabsorption capacity of the SGLT system limits the efficacy of SGLT2 inhibitors (Nakamura et al., 2004). They conducted a rat study and found significant reductions in the Vmax values of both SGLT1 and SGLT2 in isolated renal apical membrane vesicles from rats subjected to 5/6 nephrectomy compared with the control group. Specifically, SGLT1 and SGLT2 Vmax values were reduced by 56.7% and 47.8%, respectively, while the Km value was not significantly changed.
Moreover, we found that the degree of decrease in the reabsorption capacity of the SGLT2 transporter in patients with renal insufficiency was similar to the degree of decrease in the UGE at 24 h after SGLT2 inhibitors treatment. For example, the 24 h UGE with treatment of dapagliflozin was decreased by 42%, 83%, and 84% in patients with mild, moderate, and severe renal insufficiency, respectively (Kasichayanula et al., 2013), further supporting the notion that reduced efficacy of SGLT2 inhibitors in patients with renal insufficiency correlates with impaired SGLT2 transporter function. In conclusion, our results suggested that the function of SGLT2 transporter may change in T2DM co-occurring with renal insufficiency, thus contributing to the reduced efficacy of SGLT2 inhibitors in this population.
The predictive performance of the PD model for SGLT2 inhibitors established in this study still requires improvement. Firstly, the model relied on manually inputting the average glucose level of patients and could not dynamically simulate changes in glucose and insulin levels following food intake nor accurately predict the decline in average blood glucose after SGLT2 inhibitor treatment. Secondly, it was unable to reliably predict the changes in blood glucose within 24 h. Thirdly, the liver and kidneys are interlinked in drug metabolism and excretion processes. Renal impairment can indirectly influence hepatic metabolic capabilities, affecting factors such as drug protein binding and the expression of hepatic enzymes. Our model of SGLT2 inhibitors for patients with renal impairment has not yet accounted for these factors (Butrovich et al., 2022). Furthermore, the clinically measured data used to validate the PK/PD model in patients with renal insufficiency were limited, especially in the PD model. More clinically measured data are needed to further validate the reliability of the model.
5 Conclusion
We successfully established the PBPK/PD model of SGLT2 inhibitors to simulate the PK and PD in healthy people and T2DM patients with renal insufficiency. The PBPK model simulated 24-h urinary glucose excretion in patients with renal insufficiency to compare the hypoglycemic effects of four SGLT2 inhibitors, the model simulation results recommend ipragliflozin 50 mg qd in patients with normal renal function and patients with mild renal insufficiency, dapagliflozin 10 mg qd or canagliflozin 100 mg qd in patients with moderate renal insufficiency, and empagliflozin 10 mg qd in patients with severe renal insufficiency to achieve the lowest dose and the maximum therapeutic effect. This study provided a scientific basis for optimizing the dosage regimen in T2DM patients with different degrees of CKD.
Data availability statement
The original contributions presented in the study are included in the article/Supplementary Material, further inquiries can be directed to the corresponding author.
Author contributions
GG: Data curation, Formal Analysis, Investigation, Methodology, Software, Validation, Writing–original draft. MK: Data curation, Formal Analysis, Methodology, Software, Validation, Writing–original draft. JX: Data curation, Formal Analysis, Methodology, Validation, Writing–review and editing. WW: Data curation, Formal Analysis, Investigation, Methodology, Writing–review and editing. JC: Data curation, Formal Analysis, Project administration, Software, Writing–review and editing. CK: Data curation, Formal Analysis, Investigation, Methodology, Writing–review and editing. PH: Data curation, Formal Analysis, Investigation, Software, Writing–review and editing. CL: Data curation, Formal Analysis, Funding acquisition, Software, Writing–review and editing.
Funding
The author(s) declare that financial support was received for the research and/or publication of this article. The research was supported by Joint Funds for the Innovation of Science and Technology, Fujian Province (No. 2023Y9038), Natural Science Fundation of Fujian Province (No. 2023J01600), Fujian Provincial Health Technology Project (No. 2023CXA017).
Conflict of interest
The authors declare that the research was conducted in the absence of any commercial or financial relationships that could be construed as a potential conflict of interest.
Generative AI statement
The author(s) declare that no Generative AI was used in the creation of this manuscript.
Publisher’s note
All claims expressed in this article are solely those of the authors and do not necessarily represent those of their affiliated organizations, or those of the publisher, the editors and the reviewers. Any product that may be evaluated in this article, or claim that may be made by its manufacturer, is not guaranteed or endorsed by the publisher.
Supplementary material
The Supplementary Material for this article can be found online at: https://www.frontiersin.org/articles/10.3389/fphar.2025.1520268/full#supplementary-material
References
Alkabbani, W., and Gamble, J. M. (2021). Profile of ipragliflozin, an oral SGLT-2 inhibitor for the treatment of type 2 diabetes: the evidence to date. Drug Des. Devel Ther. 15, 3057–3069. doi:10.2147/DDDT.S281602
Balazki, P., Schaller, S., Eissing, T., and Lehr, T. (2018). A quantitative systems pharmacology kidney model of diabetes associated renal hyperfiltration and the effects of SGLT inhibitors. CPT Pharmacometrics Syst. Pharmacol. 7 (12), 788–797. doi:10.1002/psp4.12359
Brand, T., Macha, S., Mattheus, M., Pinnetti, S., and Woerle, H. J. (2012). Pharmacokinetics of empagliflozin, a sodium glucose cotransporter-2 (SGLT-2) inhibitor, coadministered with sitagliptin in healthy volunteers. Adv. Ther. 29 (10), 889–899. doi:10.1007/s12325-012-0055-3
Butrovich, M. A., Tang, W., Boulton, D. W., Nolin, T. D., and Sharma, P. (2022). Use of physiologically based pharmacokinetic modeling to evaluate the impact of chronic kidney disease on CYP3A4-mediated metabolism of saxagliptin. J. Clin. Pharmacol. 62 (8), 1018–1029. doi:10.1002/jcph.2043
Callegari, E., Lin, J., Tse, S., Goosen, T. C., and Sahasrabudhe, V. (2021). Physiologically-based pharmacokinetic modeling of the drug-drug interaction of the UGT substrate ertugliflozin following Co-administration with the UGT inhibitor mefenamic acid. CPT Pharmacometrics Syst. Pharmacol. 10 (2), 127–136. doi:10.1002/psp4.12581
Chen, X., Hu, P., Vaccaro, N., Polidori, D., Curtin, C. R., Stieltjes, H., et al. (2015). Pharmacokinetics, pharmacodynamics, and safety of single-dose canagliflozin in healthy Chinese subjects. Clin. Ther. 37 (7), 1483–1492.e1. doi:10.1016/j.clinthera.2015.04.015
Devineni, D., Curtin, C. R., Marbury, T. C., Smith, W., Vaccaro, N., Wexler, D., et al. (2015a). Effect of hepatic or renal impairment on the pharmacokinetics of canagliflozin, a sodium glucose co-transporter 2 inhibitor. Clin. Ther. 37 (3), 610–628.e4. doi:10.1016/j.clinthera.2014.12.013
Devineni, D., Murphy, J., Wang, S. S., Stieltjes, H., Rothenberg, P., Scheers, E., et al. (2015b). Absolute oral bioavailability and pharmacokinetics of canagliflozin: a microdose study in healthy participants. Clin. Pharmacol. Drug Dev. 4 (4), 295–304. doi:10.1002/cpdd.162
Devineni, D., Vaccaro, N., Murphy, J., Curtin, C., Mamidi, R. N., Weiner, S., et al. (2015c). Effects of rifampin, cyclosporine A, and probenecid on the pharmacokinetic profile of canagliflozin, a sodium glucose co-transporter 2 inhibitor, in healthy participants. Int. J. Clin. Pharmacol. Ther. 53 (2), 115–128. doi:10.5414/CP202158
Devineni, D., Vaccaro, N., Polidori, D., Stieltjes, H., and Wajs, E. (2015d). Single- and multiple-dose pharmacokinetics and pharmacodynamics of canagliflozin, a selective inhibitor of sodium glucose co-transporter 2, in healthy participants. Int. J. Clin. Pharmacol. Ther. 53 (2), 129–138. doi:10.5414/CP202218
Drugbank (2024). Canagliflozin: uses, interactions, mechanism of action | DrugBank online. Available online at: https://go.drugbank.com/drugs/DB08907.
Drugbank (2025a). Dapagliflozin: uses, interactions, mechanism of action | DrugBank online. Available online at: https://go.drugbank.com/drugs/DB06292.
Drugbank (2025b). Empagliflozin: uses, interactions, mechanism of action | DrugBank online. Available online at: https://go.drugbank.com/drugs/DB09038.
Drugbank (2025c). Ipragliflozin: uses, interactions, mechanism of action | DrugBank online. Available online at: https://go.drugbank.com/drugs/DB11698.
Ferrannini, E., Veltkamp, S. A., Smulders, R. A., and Kadokura, T. (2013). Renal glucose handling: impact of chronic kidney disease and sodium-glucose cotransporter 2 inhibition in patients with type 2 diabetes. Diabetes Care 36 (5), 1260–1265. doi:10.2337/dc12-1503
Fioretto, P., Del Prato, S., Buse, J. B., Goldenberg, R., Giorgino, F., Reyner, D., et al. (2018). Efficacy and safety of dapagliflozin in patients with type 2 diabetes and moderate renal impairment (chronic kidney disease stage 3A): the DERIVE Study. Diabetes Obes. Metab. 20 (11), 2532–2540. doi:10.1111/dom.13413
Friedrich, C., Metzmann, K., Rose, P., Mattheus, M., Pinnetti, S., and Woerle, H. J. (2013). A randomized, open-label, crossover study to evaluate the pharmacokinetics of empagliflozin and linagliptin after coadministration in healthy male volunteers. Clin. Ther. 35 (1), A33–A42. doi:10.1016/j.clinthera.2012.12.002
Garcia-Ropero, A., Badimon, J. J., and Santos-Gallego, C. G. (2018). The pharmacokinetics and pharmacodynamics of SGLT2 inhibitors for type 2 diabetes mellitus: the latest developments. Expert Opin. Drug Metab. Toxicol. 14 (12), 1287–1302. doi:10.1080/17425255.2018.1551877
Grempler, R., Thomas, L., Eckhardt, M., Himmelsbach, F., Sauer, A., Sharp, D. E., et al. (2012). Empagliflozin, a novel selective sodium glucose cotransporter-2 (SGLT-2) inhibitor: characterisation and comparison with other SGLT-2 inhibitors. Diabetes Obes. Metab. 14 (1), 83–90. doi:10.1111/j.1463-1326.2011.01517.x
Heerspink, H. J. L., Stefansson, B. V., Correa-Rotter, R., Chertow, G. M., Greene, T., Hou, F. F., et al. (2020). Dapagliflozin in patients with chronic kidney disease. N. Engl. J. Med. 383 (15), 1436–1446. doi:10.1056/NEJMoa2024816
Huang, S. M., and Rowland, M. (2012). The role of physiologically based pharmacokinetic modeling in regulatory review. Clin. Pharmacol. Ther. 91 (3), 542–549. doi:10.1038/clpt.2011.320
Hummel, C. S., Lu, C., Liu, J., Ghezzi, C., Hirayama, B. A., Loo, D. D., et al. (2012). Structural selectivity of human SGLT inhibitors. Am. J. Physiol. Cell Physiol. 302 (2), C373–C382. doi:10.1152/ajpcell.00328.2011
Iijima, H., Kifuji, T., Maruyama, N., and Inagaki, N. (2015). Pharmacokinetics, pharmacodynamics, and safety of canagliflozin in Japanese patients with type 2 diabetes mellitus. Adv. Ther. 32 (8), 768–782. doi:10.1007/s12325-015-0234-0
Inagaki, N., Kondo, K., Yoshinari, T., Ishii, M., Sakai, M., Kuki, H., et al. (2014). Pharmacokinetic and pharmacodynamic profiles of canagliflozin in Japanese patients with type 2 diabetes mellitus and moderate renal impairment. Clin. Drug Investig. 34 (10), 731–742. doi:10.1007/s40261-014-0226-x
Jo, H., Pilla Reddy, V., Parkinson, J., Boulton, D. W., and Tang, W. (2021). Model-informed pediatric dose selection for dapagliflozin by incorporating developmental changes. CPT Pharmacometrics Syst. Pharmacol. 10 (2), 108–118. doi:10.1002/psp4.12577
Kasichayanula, S., Chang, M., Hasegawa, M., Liu, X., Yamahira, N., LaCreta, F. P., et al. (2011). Pharmacokinetics and pharmacodynamics of dapagliflozin, a novel selective inhibitor of sodium-glucose co-transporter type 2, in Japanese subjects without and with type 2 diabetes mellitus. Diabetes Obes. Metab. 13 (4), 357–365. doi:10.1111/j.1463-1326.2011.01359.x
Kasichayanula, S., Liu, X., Lacreta, F., Griffen, S. C., and Boulton, D. W. (2014). Clinical pharmacokinetics and pharmacodynamics of dapagliflozin, a selective inhibitor of sodium-glucose co-transporter type 2. Clin. Pharmacokinet. 53 (1), 17–27. doi:10.1007/s40262-013-0104-3
Kasichayanula, S., Liu, X., Pe Benito, M., Yao, M., Pfister, M., LaCreta, F. P., et al. (2013). The influence of kidney function on dapagliflozin exposure, metabolism and pharmacodynamics in healthy subjects and in patients with type 2 diabetes mellitus. Br. J. Clin. Pharmacol. 76 (3), 432–444. doi:10.1111/bcp.12056
Khurana, M., Vaidyanathan, J., Marathe, A., Mehrotra, N., Sahajwalla, C. G., Zineh, I., et al. (2015). Canagliflozin use in patients with renal impairment-Utility of quantitative clinical pharmacology analyses in dose optimization. J. Clin. Pharmacol. 55 (6), 647–656. doi:10.1002/jcph.466
Kuepfer, L., Niederalt, C., Wendl, T., Schlender, J. F., Willmann, S., Lippert, J., et al. (2016). Applied concepts in PBPK modeling: how to build a PBPK/PD model. CPT Pharmacometrics Syst. Pharmacol. 5 (10), 516–531. doi:10.1002/psp4.12134
Macha, S., Mattheus, M., Halabi, A., Pinnetti, S., Woerle, H. J., and Broedl, U. C. (2014). Pharmacokinetics, pharmacodynamics and safety of empagliflozin, a sodium glucose cotransporter 2 (SGLT2) inhibitor, in subjects with renal impairment. Diabetes Obes. Metab. 16 (3), 215–222. doi:10.1111/dom.12182
Maurer, T. S., Ghosh, A., Haddish-Berhane, N., Sawant-Basak, A., Boustany-Kari, C. M., She, L., et al. (2011). Pharmacodynamic model of sodium-glucose transporter 2 (SGLT2) inhibition: implications for quantitative translational pharmacology. AAPS J. 13 (4), 576–584. doi:10.1208/s12248-011-9297-2
Mende, C. W. (2022). Chronic kidney disease and SGLT2 inhibitors: a review of the evolving treatment landscape. Adv. Ther. 39 (1), 148–164. doi:10.1007/s12325-021-01994-2
Mori, K., Saito, R., Nakamaru, Y., Shimizu, M., and Yamazaki, H. (2016). Physiologically based pharmacokinetic-pharmacodynamic modeling to predict concentrations and actions of sodium-dependent glucose transporter 2 inhibitor canagliflozin in human intestines and renal tubules. Biopharm. Drug Dispos. 37 (8), 491–506. doi:10.1002/bdd.2040
Nakamura, N., Masuda, S., Takahashi, K., Saito, H., Okuda, M., and Inui, K. (2004). Decreased expression of glucose and peptide transporters in rat remnant kidney. Drug Metab. Pharmacokinet. 19 (1), 41–47. doi:10.2133/dmpk.19.41
Perez-Urizar, J., Granados-Soto, V., Flores-Murrieta, F. J., and Castaneda-Hernandez, G. (2000). Pharmacokinetic-pharmacodynamic modeling: why? Arch. Med. Res. 31 (6), 539–545. doi:10.1016/s0188-4409(00)00242-3
Perkovic, V., Jardine, M. J., Neal, B., Bompoint, S., Heerspink, H. J. L., Charytan, D. M., et al. (2019). Canagliflozin and renal outcomes in type 2 diabetes and nephropathy. N. Engl. J. Med. 380 (24), 2295–2306. doi:10.1056/NEJMoa1811744
Pinto, L. C., Rados, D. V., Remonti, L. R., Viana, M. V., Leitao, C. B., and Gross, J. L. (2022). Dose-ranging effects of SGLT2 inhibitors in patients with type 2 diabetes: a systematic review and meta-analysis. Arch. Endocrinol. Metab. 66 (1), 68–76. doi:10.20945/2359-3997000000440
Qiu, M., Ding, L. L., Zhang, M., and Zhou, H. R. (2021). Safety of four SGLT2 inhibitors in three chronic diseases: a meta-analysis of large randomized trials of SGLT2 inhibitors. Diab Vasc. Dis. Res. 18 (2), 14791641211011016. doi:10.1177/14791641211011016
Sarashina, A., Ueki, K., Sasaki, T., Tanaka, Y., Koiwai, K., Sakamoto, W., et al. (2014). Effect of renal impairment on the pharmacokinetics, pharmacodynamics, and safety of empagliflozin, a sodium glucose cotransporter 2 inhibitor, in Japanese patients with type 2 diabetes mellitus. Clin. Ther. 36 (11), 1606–1615. doi:10.1016/j.clinthera.2014.08.001
Scheen, A. J. (2014). Drug-drug interactions with sodium-glucose cotransporters type 2 (SGLT2) inhibitors, new oral glucose-lowering agents for the management of type 2 diabetes mellitus. Clin. Pharmacokinet. 53 (4), 295–304. doi:10.1007/s40262-013-0128-8
Scheen, A. J. (2015). Pharmacodynamics, efficacy and safety of sodium-glucose co-transporter type 2 (SGLT2) inhibitors for the treatment of type 2 diabetes mellitus. Drugs 75 (1), 33–59. doi:10.1007/s40265-014-0337-y
Seman, L., Macha, S., Nehmiz, G., Simons, G., Ren, B., Pinnetti, S., et al. (2013). Empagliflozin (BI 10773), a potent and selective SGLT2 inhibitor, induces dose-dependent glucosuria in healthy subjects. Clin. Pharmacol. Drug Dev. 2 (2), 152–161. doi:10.1002/cpdd.16
The, E.-K. C. G., Herrington, W. G., Staplin, N., Wanner, C., Green, J. B., Hauske, S. J., et al. (2023). Empagliflozin in patients with chronic kidney disease. N. Engl. J. Med. 388 (2), 117–127. doi:10.1056/NEJMoa2204233
Veltkamp, S. A., Kadokura, T., Krauwinkel, W. J., and Smulders, R. A. (2011). Effect of Ipragliflozin (ASP1941), a novel selective sodium-dependent glucose co-transporter 2 inhibitor, on urinary glucose excretion in healthy subjects. Clin. Drug Investig. 31 (12), 839–851. doi:10.1007/BF03256922
Wang, Z., Wang, G., and Ren, J. (2022). Using a mathematical modeling to simulate pharmacokinetics and urinary glucose excretion of luseogliflozin and explore the role of SGLT1/2 in renal glucose reabsorption. ACS Omega 7 (51), 48427–48437. doi:10.1021/acsomega.2c06483
Washburn, W. N., and Poucher, S. M. (2013). Differentiating sodium-glucose co-transporter-2 inhibitors in development for the treatment of type 2 diabetes mellitus. Expert Opin. Investig. Drugs 22 (4), 463–486. doi:10.1517/13543784.2013.774372
Yakovleva, T., Sokolov, V., Chu, L., Tang, W., Greasley, P. J., Peilot Sjogren, H., et al. (2019). Comparison of the urinary glucose excretion contributions of SGLT2 and SGLT1: a quantitative systems pharmacology analysis in healthy individuals and patients with type 2 diabetes treated with SGLT2 inhibitors. Diabetes Obes. Metab. 21 (12), 2684–2693. doi:10.1111/dom.13858
Zaccardi, F., Webb, D. R., Htike, Z. Z., Youssef, D., Khunti, K., and Davies, M. J. (2016). Efficacy and safety of sodium-glucose co-transporter-2 inhibitors in type 2 diabetes mellitus: systematic review and network meta-analysis. Diabetes Obes. Metab. 18 (8), 783–794. doi:10.1111/dom.12670
Zhang, W., Krauwinkel, W. J., Keirns, J., Townsend, R. W., Lasseter, K. C., Plumb, L., et al. (2013). The effect of moderate hepatic impairment on the pharmacokinetics of ipragliflozin, a novel sodium glucose co-transporter 2 (SGLT2) inhibitor. Clin. Drug Investig. 33 (7), 489–496. doi:10.1007/s40261-013-0089-6
Zhang, Y., Xie, P., Li, Y., Chen, Z., and Shi, A. (2023). Mechanistic evaluation of the inhibitory effect of four SGLT-2 inhibitors on SGLT 1 and SGLT 2 using physiologically based pharmacokinetic (PBPK) modeling approaches. Front. Pharmacol. 14, 1142003. doi:10.3389/fphar.2023.1142003
Zhao, X., Cui, Y., Zhao, S., Lang, B., Broedl, U. C., Salsali, A., et al. (2015). Pharmacokinetic and pharmacodynamic properties and tolerability of single- and multiple-dose once-daily empagliflozin, a sodium glucose cotransporter 2 inhibitor, in Chinese patients with type 2 diabetes mellitus. Clin. Ther. 37 (7), 1493–1502. doi:10.1016/j.clinthera.2015.05.001
Keywords: PBPKmodel, PD model, SGLT2 inhibitors, renal insufficiency, type 2 diabetes mellitus
Citation: Guo G, Ke M, Xu J, Wu W, Chen J, Ke C, Huang P and Lin C (2025) Physiologically based pharmacokinetic model of sodium-glucose cotransporter 2 inhibitors predicted pharmacokinetics and pharmacodynamics to explore dosage regimen for patients with type 2 diabetes mellitus and renal insufficiency. Front. Pharmacol. 16:1520268. doi: 10.3389/fphar.2025.1520268
Received: 31 October 2024; Accepted: 17 March 2025;
Published: 31 March 2025.
Edited by:
Jiangxin Wang, Shenzhen University, ChinaReviewed by:
Hinojal Zazo, University of Salamanca, SpainXinning Yang, United States Food and Drug Administration, United States
Sandhya Subash, Washington State University Health Sciences Spokane, United States
Jessica L. Beers, University of Washington, United States
Copyright © 2025 Guo, Ke, Xu, Wu, Chen, Ke, Huang and Lin. This is an open-access article distributed under the terms of the Creative Commons Attribution License (CC BY). The use, distribution or reproduction in other forums is permitted, provided the original author(s) and the copyright owner(s) are credited and that the original publication in this journal is cited, in accordance with accepted academic practice. No use, distribution or reproduction is permitted which does not comply with these terms.
*Correspondence: Cuihong Lin, bGluY3VpaG9uZzE5NzRAc2luYS5jb20=
†These authors have contributed equally to this work.