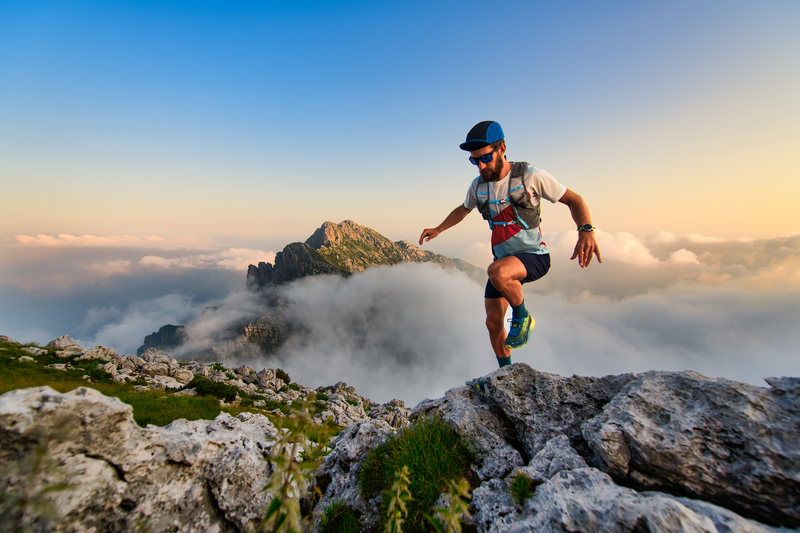
94% of researchers rate our articles as excellent or good
Learn more about the work of our research integrity team to safeguard the quality of each article we publish.
Find out more
ORIGINAL RESEARCH article
Front. Pharmacol.
Sec. Pharmacology of Infectious Diseases
Volume 16 - 2025 | doi: 10.3389/fphar.2025.1511088
This article is part of the Research Topic Multidrug Resistant Bacteria: New Therapeutic Approaches for a Challenging Problem View all articles
The final, formatted version of the article will be published soon.
You have multiple emails registered with Frontiers:
Please enter your email address:
If you already have an account, please login
You don't have a Frontiers account ? You can register here
Aims: To establish a population pharmacokinetic (PopPK) model of polymyxin B (PMB) in critically ill patients based on steady-state trough (Ctrough,ss) and peak (Cpeak,ss) concentrations, optimize the dosing regimen, and evaluate the consistency of 24-hour steady-state area under the concentration-time curve (AUCss,24h) estimation between model-based and the two-point (Ctrough,ss and Cpeak,ss) methods. Methods: PopPK modeling was performed using NONMEM, Monte Carlo simulations were used to optimize PMB dosing regimens. Bland-Altman analysis was used to evaluate the consistency between the two AUCss,24h estimation methods.Results: A total of 95 patients, contributing 214 blood samples, were included and categorized into a modeling group (n=80) and a validation group (n=15). A one-compartment model was developed, with creatinine clearance (CrCL) and platelet
Keywords: population pharmacokinetics, Polymyxin B, Critical Illness, Dosing optimization, concordance
Received: 14 Oct 2024; Accepted: 20 Feb 2025.
Copyright: © 2025 Yang, Yu, Gan, Cheng, Yang, Xiong, Liu and Chen. This is an open-access article distributed under the terms of the Creative Commons Attribution License (CC BY). The use, distribution or reproduction in other forums is permitted, provided the original author(s) or licensor are credited and that the original publication in this journal is cited, in accordance with accepted academic practice. No use, distribution or reproduction is permitted which does not comply with these terms.
* Correspondence:
Fang Liu, Department of Pharmacy,The First Hospital Affiliated to Army Medical University, Chongqing, China
Chuan Yong Chen, Department of Pharmacy,The First Hospital Affiliated to Army Medical University, Chongqing, China
Disclaimer: All claims expressed in this article are solely those of the authors and do not necessarily represent those of their affiliated organizations, or those of the publisher, the editors and the reviewers. Any product that may be evaluated in this article or claim that may be made by its manufacturer is not guaranteed or endorsed by the publisher.
Research integrity at Frontiers
Learn more about the work of our research integrity team to safeguard the quality of each article we publish.