- 1Department of Pharmacy, Children’s Hospital of Fudan University, National Children’s Medical Center, Shanghai, China
- 2Department of Nephrology, Children’s Hospital of Fudan University, National Children’s Medical Center, Shanghai, China
Purpose: Rituximab has proven efficacy in children with idiopathic nephrotic syndrome (INS). However, vast majority of children inevitably experience relapse with B-cell repletion, necessitating repeat course of rituximab, which may increase the risk of adverse effects. The timing of additional dosing and optional dosing regimen of rituximab in pediatric patients with INS have yet to be determined. This study aimed to identify factors that influence disease relapse and B-cell repletion to provide tailored treatment.
Methods: LASSO and random survival forest were performed on 143 children to screen covariates which were then included in Cox regression model to determine the biomarkers of relapse and establish a nomogram. A kinetic-pharmacodynamic (K-PD) model was developed in 59 children to characterize the time course of CD19+ B-cell after rituximab treatment. Monte Carlo simulation was conducted to explore a mini-dose regimen with larger intervals.
Results: Nomogram contained 7 predictors of relapse including neutrophil-to-lymphocyte ratio, duration of B-cell depletion, duration of disease, urine immunoglobulin G to creatinine ratio, urine transferrin, duration of maintenance immunosuppressant and hemoglobin. As a direct PD indicator, each 1-month increase of duration of B-cell depletion decreased risk of relapse by 21.4% (HR = 0.786; 95% CI: 0.635–0.972; p = 0.026). The K-PD model predicted t1/2 (CV%) of rituximab and CD19+ B-cell to be 11.6 days (17%) and 173.3 days (22%), respectively. Immunoglobulin A is an important covariate of ED50. Simulation of a mini-dose regimen with larger intervals (three 150 mg every 2 monthly) indicted longer B-cell depletion time (>7 months) compared to standard regimen.
Conclusion: The nomogram indicated optimal infusion timing before relapse and the K-PD model provided tailored rituximab regimens for children with INS to reduce safety risks and financial burden.
1 Introduction
Idiopathic nephrotic syndrome (INS), characterized by heavy proteinuria, hypoalbuminemia, edema and hyperlipidemia, is the most common glomerular disease in children (Trautmann et al., 2023). Although most cases are steroid sensitive NS and respond well to steroids therapy, up to 50% of children develop frequent relapsing or steroid dependent NS (FRSDNS), requiring addition of steroid-sparing immunosuppressants (Trautmann et al., 2023; Webb et al., 2019). Among the children who do not respond, defined as having steroid-resistant NS, most respond to immunosuppressants, mainly with calcineurin inhibitors, while children in whom no response is observed are described as multi drug resistant. It has been demonstrated that overexpression of P-glycoprotein on lymphocytes result in poor response and steroid resistance (Prasad et al., 2015). The changes in histological appearance to focal segmental glomerulosclerosis are associated with multi drug resistance. Also circulating factors are present in multi-drug-resistant NS (Vivarelli et al., 2023; Ying et al., 2021). Rituximab, a B-cell-targeting anti-CD20 monoclonal antibody, has emerged as an effective treatment for complicated FRSDNS to induce prolonged remission and avoid steroids toxicities, allowing withdrawal of steroids and concomitant immunosuppressants (Chan et al., 2023; Chan et al., 2022). Clinical efficacy of rituximab depends on B-cell depletion, and responders are prone to relapse following B-cell repletion (Colucci et al., 2016). 80% of children eventually relapse 1 year after rituximab (Chan et al., 2020), necessitating either repeat course of rituximab or addition of steroid-sparing immunosuppressants (Chan and Tullus, 2021). Despite its excellent efficacy and favorable safety profile, repeat courses of rituximab may increase the risk of adverse effects, including infusion reactions and hypogammaglobulinemia, especially in young children (Sinha et al., 2023). However, the timing of additional dosing and optional dosing regimen of rituximab in pediatric patients with INS have yet to be determined. The current dosing regimen is based on the treatment of tumor B-cells, which proliferate much more rapidly than normal B-cells in INS. A study in healthy volunteers demonstrated that 97% depletion of circulating B-cell after infusion of 1 mg/m2 rituximab, although transient, made it conceivable that lower dose than the authorized (375 mg/m2) might be sufficient to deplete all B-cell (Schoergenhofer et al., 2018). Recently, a retrospective study in primary membranous nephropathy (PMN) revealed that monthly mini-dose (100 mg) achieved comparable clinical efficacy with reduced risks and financial burden compared with the standard regimen (Wang et al., 2023). The kinetic-pharmacodynamic (K-PD) model is a powerful tool to quantitative dose-response relationship and accurately predicting the clinical efficacy of different regimens in the absence of PK data (Pan et al., 2019). To our knowledge, no K-PD studies reporting the use of rituximab in pediatric patients with INS.
Therefore, we aimed (1) to identify predictor of relapse with special emphasis the relation with CD19+ B-cell to guide re-dosing of rituximab; (2) to investigate covariates associated with efficacy and explore the impact of different dosing regimens on CD19+ B-cell repletion time.
2 Methods
2.1 Patients
We retrospectively collected clinical data of 143 children diagnosed with INS treated with rituximab in Department of Nephrology of Children’ Hospital of Fudan University between January 2014 and December 2022. Inclusion criteria were age <18 years; the first use of rituximab; and at least 6 months of follow-up post-rituximab. Exclusion criteria were congenital or secondary NS [e.g., lupus nephritis, IgA nephropathy, Henoch-Schonlein purpura nephritis (HSPN)], chronic infections such as tuberculosis, HIV, hepatitis B or C and malignancy. CD19+ B-cell counts from 59 children were used to develop a K-PD model of rituximab.
2.2 Treatment regimen
Rituximab (MabThera® and Henlius®, Shanghai, China) course comprised 2 infusions (375 mg/m2, maximum 500 mg) given a week apart after attainment of remission. To prevent infusion reactions, patients received methylprednisolone, acetaminophen and antiallergy medications, approximately 30 min before rituximab treatment. Steroids and concomitant immunosuppressive agents were tapered and discontinued within 3–6 months post-rituximab treatment. Mycophenolate mofetil (MMF) was used to maintain remission after B-cell repletion and its protocol and monitoring were individualized. The initial dose of MMF was 20–30 mg/kg/d and subsequently adjusted to an AUC0–12h levels of 30–60 mg·h/L.
2.3 Data collection
Clinical and laboratory variables were routinely assessed and reviewed from the medical records to identify biomarkers associated with relapse (baseline at relapse) and B-cell repletion (baseline at rituximab treatment), including demographics, renal histology, steroid responsiveness, age at onset, age at rituximab treatment, duration of disease, prior and maintenance immunosuppressants, hematological and biochemical parameters, immunological profile and urine protein panel.
2.4 Outcome
The first outcome was the predictors of relapse with special emphasis the relation with B-cell. Patients who were lost to follow-up or did not relapse were censored at the last follow-up. Remission was defined as urine protein-to-creatinine ratio (UPCR) <0.2 mg/mg or urine dipstick nil or trace for 3 consecutive days. Relapse was defined as UPCR >2.0 mg/mg or urine dipstick ≥3 + for 3 consecutive days (Trautmann et al., 2023).
The second outcome was covariates associated with B-cell repletion and the time course of CD19+ B-cell under different rituximab dosing regimen. The duration of B-cell depletion was defined as the time from the rituximab treatment to the first repletion of CD19+ B-cell in the peripheral blood. B-cell depletion and repletion were defined as CD19+ B-cell <1% and >1% (10/μL) of the total lymphocyte population, respectively (Chan et al., 2022).
2.5 Model construction and evaluation
2.5.1 Cox regression model to predict relapse
Addressing multicollinearity and the overfitting problem in high-dimensional data, least absolute shrinkage and selection operator (LASSO) and random survival forests (RSF) were adopted to select significant variables. Lasso regression was a penalized linear regression models in which the coefficients of variables that did not significantly contribute to model performance were shrunk to zero by imposing a shrinkage parameter lambda (λ). The optimal λ was selected the value that yielded the minimum deviance plus one standard deviation in a ten-fold cross-validation process. Features with non-zero coefficients in the LASSO regression with the optimal λ value were retained for subsequent modeling (Lee et al., 2022; Adamichou et al., 2021). RSF algorithms, a non-linear method, was an ensemble of binary decision trees. For each decision trees 1,000 bootstrap sample are randomly drawn from original data, which includes 63% of the observations and remains the left 37% as the out-of-bag data that is used to calculate the prediction error rate. The top 10 optimal variables were selected based on minimum depth and variable importance (VIMP) ranking (Dietrich et al., 2016). The overlapping variables of the two algorithms were entered into Cox regression model to screen core predictive variables and estimate relative risks. Receiver operator characteristic (ROC) curves were performed to evaluate the diagnostic accuracy of predictors of relapse. Kaplan-Meier curves were used to describe relapse-free survival. Restricted cubic spline (RCS) was used to visualize the association of identified variables with relapse risk on a continuous scale. Model performance was assessed using ROC curve, calibration curve, and decision curve analysis (DCA). To facilitate the clinical service, we converted the complex mathematical model into a nomogram.
2.5.2 K-PD model of rituximab to optimize dosing regimen
The time course of CD19+ B-cell was described with a turn-over model where the balance between synthesis and degradation rate was disrupted by rituximab, increasing the latter process (Supplementary Figure S1). Structural model was expressed as follows:
where A1 was initial dose of rituximab, Ke was a first-order elimination rate constant of rituximab, Kin and Kout were the zero and first-order rate constants of synthesis and degradation, respectively. Emax was the maximum effect of rituximab, ED50 was dose of rituximab to achieve 50% of the Emax, E was CD19+ B-cell. The baseline E = Kin/Kout. γ was the Hill coefficient, influencing the shape of curve.
Residual variability was the difference between observed and predicted values. Proportional, additive and mixed additive-proportional residual models were tested. The nonlinear mixed effects modeling approach was used to obtain the population typical values of PK parameters and to identify and quantify the covariates that affect the PK parameters. Inter-individual variability was modelled exponentially as follows:
where θi was individual parameter, θpop was typical value of parameter, ηi was inter-individual variability, that was the deviation of ηi from θpop, which was normally distributed with mean zero and variance of ω2, and β quantified the influence of covariate.
Covariates were tested for their effects on K-PD parameters using a stepwise method with forward inclusion (reduction in objective function value (ΔOFV) by 6.63; p < 0.01) and backward exclusion (ΔOFV = 10.8; p < 0.001) procedure. The model was evaluated by goodness-of-fit plots including the observed versus population and individual predicted values, conditional weighted residuals versus predicted values and time after dose. The visual predictive check (VPC) (1,000 simulations) and bootstrap (500 resamples) were used to evaluate the predictive performance of final model. To quantify the influence of covariates on K-PD parameters, the final model was used to simulate the time course of CD19+ B-cell at covariate of maximum, median and minimum of population in the original dataset. Monte Carlo simulations (1,000 replicates) were conducted for standard regimen and mini-dose regimen with larger intervals to explore new dosing regimen.
2.6 Statistical analysis
Descriptive statistics were summarized using mean ± SD or median [interquartile ranges (IQR)] for continuous variables and frequencies with percentages [n (%)] for categorical variables. Differences in clinical characteristic between patients with and without relapse were compared with t-test or Mann-Whitney U test for continuous variables and χ2 or Fisher’ exact tests for categorical data. Statistical significance was defined as a two-tailed p < 0.05. Analysis was performed using IBM SPSS Statistics (version 27.0) and R software (version 4.2.1). The K-PD model was constructed using the software Phoenix NLME (version 8.4). OriginPro 2021 software (version 9.8.0.200) was used for figures.
3 Results
3.1 Patients
This study included 143 children (109 males). During the follow-up period of 16.7 (10.5–25.2) months, 83 (58.0%) patients relapsed, with median time to relapse of 14.0 (8.6–21.2) months. The median age at onset and at time of rituximab treatment was 4.0 (2.3–7.5) and 7.9 (4.9–10.6) years, respectively. The median duration of disease was 2.2 (1.1–3.8) years. The median duration of maintenance immunosuppressants (DmIS) was 10.0 (3.0–16.0) months. The mean duration of B-cell depletion was 5.5 ± 1.2 months, with large individual variability. Baseline demographical and clinical characteristics were described in Supplementary Table S1.
3.2 Cox regression model to predict relapse
3.2.1 Identification of predictors of relapse
The LASSO regression had excellent performance but minimum number of variables when lambda.1se was 0.133 (Supplementary Figure S2). A total of 12 variables were screened out from 46 candidate variables, including neutrophil-to-lymphocyte ratio (NLR), duration of B-cell depletion, urine immunoglobulin G to creatinine ratio (U-IgG/Cr), urine transferrin (U-TF), duration of disease, DmIS, hemoglobin, albumin, alanine aminotransferase, triglyceride, CD4+ T cell count and prior immunosuppressants. Of RSF, prediction error rate was minimum when the number of trees was 70. The top 10 important variables were selected according to VIMP (Supplementary Figure S3A) and minimal depth (Supplementary Figure S3B), in the order of DmIS, duration of disease, U-IgG/Cr, hemoglobin, NLR, duration of B-cell depletion, CD16+CD56+, CD19+ %, U-TF and 24-h proteinuria. Seven overlapping predictive variables were input into multivariate Cox regression model, all of which remained significant (Table 1). The correlation coefficients among all variables were <0.5 (Supplementary Figure S4). Duration of disease, NLR, U-IgG/Cr and U-TF were positively correlated with relapse, while DmIS, duration of B-cell repletion and hemoglobin were negatively correlated with relapse. In cox regression model, NLR had the greatest effect on relapse, followed by duration of B-cell depletion. Each 1-unit increase of NLR was associated with a 26.5% increased risk of relapse (HR = 1.265; 95% CI: 1.080–1.481; p = 0.003), while each 1-month increase of duration of B-cell depletion was associated with a 21.4% decreased risk of relapse (HR = 0.786; 95% CI: 0.635–0.972; p = 0.026). In addition, ROC curves were performed to compare the diagnostic performance of identified variables on relapse (Supplementary Table S2). Among them, U-IgG/Cr showed the best performance in diagnosing relapse (AUC = 0.693; 95% CI: 0.605–0.781; p < 0.001), followed by U-TF (AUC = 0.667; 95% CI: 0.578–0.775; p = 0.001). The ROC analysis showed that U-IgG/Cr > 70.5 mg/g had a good prevention for relapse with 66.3% sensitivity and 71.7% specificity, while U-TF threshold of 376.0 mg/L had a low sensitivity of 48.2% but a high specificity of 86.7% for relapse. In brief, the ROC (Figure 1) and Kaplan-Meier curves (Supplementary Figure S5) illustrated that the final model could stratify relapse patients well according to identified variable.
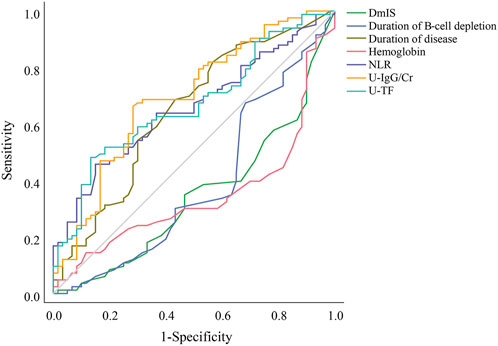
Figure 1. Receiver operating characteristic curves for the diagnostic accuracy of predictors of relapse. DmIS, duration of maintenance immunosuppression; NLR, neutrophil to lymphocyte ratio; U-IgG/Cr, urine immunoglobulin G to creatinine ratio; U-TF, urine transferrin.
3.2.2 Visualization of relationship between variables and relapse
RCS was used to visualize the association of identified variables with relapse risk on a continuous scale (Figure 2). The RCS showed a positive linear association between duration of B-cell depletion and relapse risk with a cutoff of 5.6 months, but a negative linear association between U-TF and relapse risk with a cutoff of 261.2 mg/L. The relapse risk decreased with longer DmIS and higher hemoglobin levels but increased with longer duration of disease and higher U-IgG/Cr level, then reached a plateau. The association between NLR and relapse risk was J shaped, and NLR level >1.52 was associated with an increased relapse risk. The cutoff values of identified variables in RCS model were consistent with those in ROC analysis (Supplementary Table S2), except for U-TF.
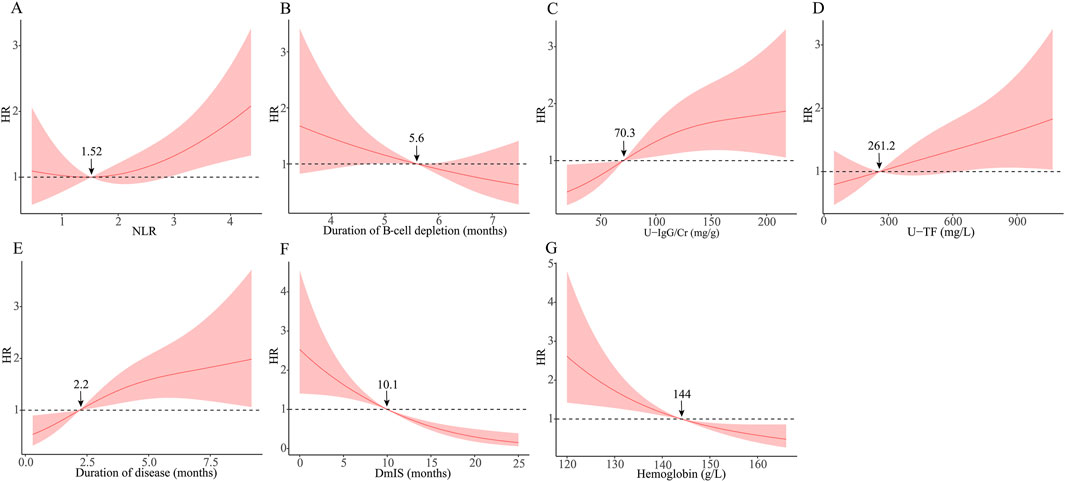
Figure 2. Association between identified variables and relapse risk using restricted cubic spline. The solid line is adjusted HR, the shaded area showing 95% confidence intervals. Reference lines is set at a hazard ratio (HR) of 1.0. DmIS, duration of maintenance immunosuppression; NLR, neutrophil to lymphocyte ratio; U-IgG/Cr, urine immunoglobulin G to creatinine ratio; U-TF, urine transferrin.
3.2.3 Model evaluation and validation
For model discrimination, the AUC for predicting relapse risk at 1-, 2- and 3-year were 0.952 (95% CI: 0.918–0.986), 0.906 (95% CI: 0.851–0.961) and 0.931 (95% CI: 0.861–1.00), respectively (Supplementary Figures S6A–C). For model accuracy, the calibration curves for predicting relapse risk at 1-, 2- and 3-year showed a good consistency between the predicted and observed values (Supplementary Figures S6D–F). The clinical net benefit at 1-, 2- and 3-year were above the two extreme curves in DCA curve, suggesting that the model had better clinical predictive efficacy (Supplementary Figures S6G–I). In all, ROC, calibration and DCA curves indicated that the Cox model had good predictive performance.
3.2.4 Nomogram for predicting individual relapse events
For example, a patient with a duration of disease of 0.9 months, hemoglobin of 156 g/L, NLR of 2.72, U-IgG/Cr of 15.5 mg/g, U-TF of 86.2 mg/L, DmIS of 10 months and duration of B-cell depletion of 6.5 months, had a total score of 513, for a predicted 1-, 2- and 3-year relapse risk of 4.4%, 21.5%, and 41.3%, respectively (Figure 3).
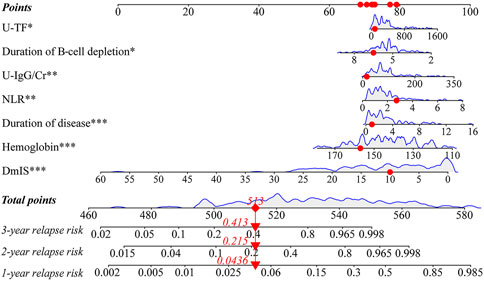
Figure 3. Nomogram for predicting 1-, 2-, and 3-year relapse risk. Red dots represented that a patient with a duration of disease of 0.9 months, hemoglobin of 156 g/L, NLR of 2.72, U-IgG/Cr of 15.5 mg/g, U-TF of 86.2 mg/L, DmIS of 10 months and duration of B-cell depletion of 6.5 months, had a total score of 513, for a predicted 1-, 2- and 3-year relapse risk of 4.4%, 21.5%, and 41.3%, respectively.
3.3 K-PD model of rituximab to optimize dosing regimen
3.3.1 Exploration of predictors of B-cell repletion
A total of 526 measurements of CD19+ B-cell counts from 59 children were available for K-PD model. Baseline characteristics were summarized in Supplementary Table S3. The baseline IgA level and CD19+ B-cell counts were 1.15 ± 0.52 g/L and 727.18 (423.60–964.76)/μL, respectively. The CD19+ B-cell counts decreased to <10/μL in all patients following rituximab and gradually recovered within 5.1 ± 1.2 months. The parameter estimates of K-PD model were summarized in Table 2. The elimination rate constants of rituximab (Ke) and CD19+ B-cell (Kout) suggested t1/2 (CV%) of 14.1 (9.9%) days and 99 (11.4%) days, respectively. A mixed additive-proportional residual model best described residual variability. The PD parameter ED50 was 1.31 mg. Baseline IgA levels were identified as a significant covariate of ED50, explaining 27.4% inter-individual variability.
3.3.2 Model evaluation and validation
The observed values versus individual (Figure 4A) and population predicted values (Figure 4B) were uniformly distributed around the reference line, indicating a good fit of the model, except for a discordance of observed and population predicted values at high values. The conditional weighted residuals versus population predicted values (Figure 4C) and time after dose (Figure 4D) were homogenously distributed within ± 2 unit, confirming that the model had a good stability and accuracy, and without systematic deviation over time, respectively. The population estimations of parameters in the final model were close to median obtained from the bootstrap method and within 95% CI, which demonstrated that the model was stability (Table 2). For VPC, the observed quantiles were consistent with predicted quantiles for the 5th, 50th and 95th percentiles and within the 95% CI, which suggested that the model prediction was accurate (Figure 4E). The time course of CD19+ B-cell repletion was simulated at covariate IgA levels at maximum, median and minimum of 0.11, 1.15 and 2.26 g/dL, showing a great effect of IgA on CD19+ B-cell repletion (Figure 4F).
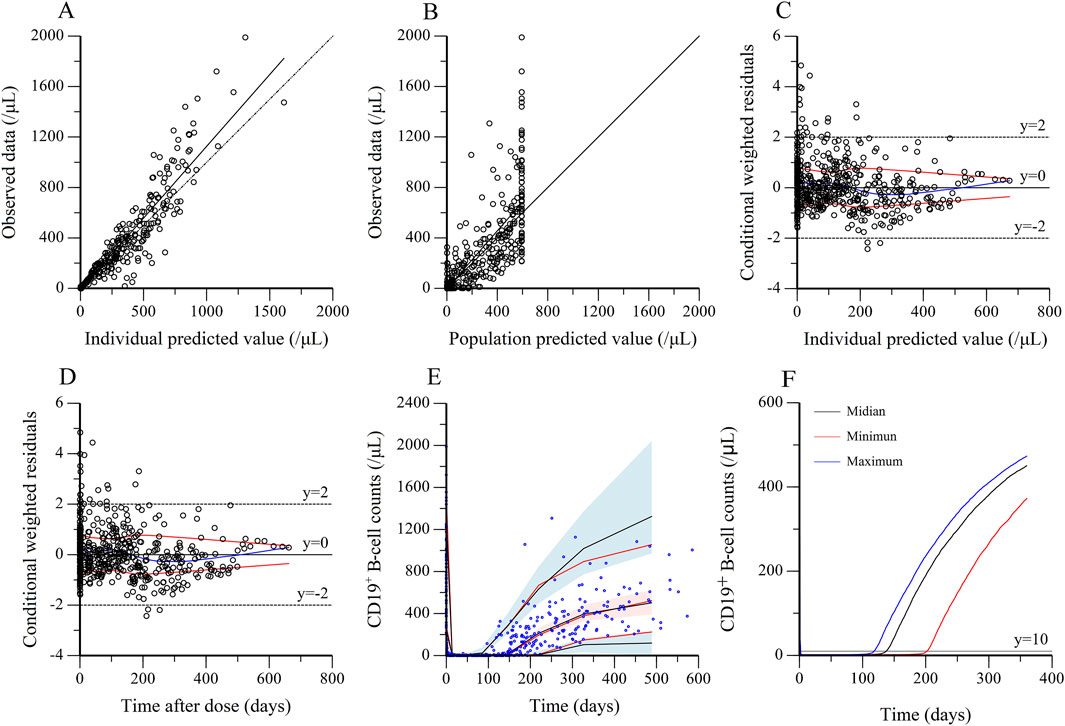
Figure 4. Evaluation and validation of the final kinetic-pharmacodynamic model. (A) Observed data versus individual predicted value. (B) Observed data versus population predicted value. (C) Conditional weighted residuals versus individual predicted. (D) Conditional weighted residuals versus time after dose. (E) Visual predictive check for prediction accuracy of the final model, in which circles were raw data, the solid red lines represent the 5th, 50th, and 95th percentiles of the observed data. The solid black lines represent the 5th, 50th, and 95th percentiles of the simulated data. The shaded areas represent the 90% confidence interval around the 5th, 50th and 95th percentiles of the simulated data. (F) The effect of IgA on B-cell repletion. The simulation is performed by varying IgA level for two weekly 375 mg/m2 rituximab treatment. The maximum, median and minimum of IgA were 0.11, 1.15 and 2.26 g/dL, respectively.
3.3.3 Dosing simulation
Based on covariate model, we carried out simulations for both standard dose and monthly mini-dose to predict CD19+ B-cell counts (Figure 5A; Table 3). For standard regimen, the suppression of rituximab on CD19+ B-cell weakened with the decrease of dosing frequency, but not significant. However, the repletion of CD19+ B-cell occurred more rapidly with single infusion of 375 mg/m2 compared to 4 weekly 375 mg/m2. For monthly mini-dose with larger interval, six monthly 100 mg and three 200 mg every 2 monthly can maintained B-cell depletion for more than 8 months, much longer than 2 weekly 375 mg/m2. When dose was reduced to three 150 mg every 2 monthly and three 100 mg every 2 monthly, the suppression of B-cell lasted for more than 7 months in 95% and 90% of patients, respectively (Figure 5B). A dose of three 150 mg every two monthly was recommended for children with INS, with lower cumulative dose and safety risks.
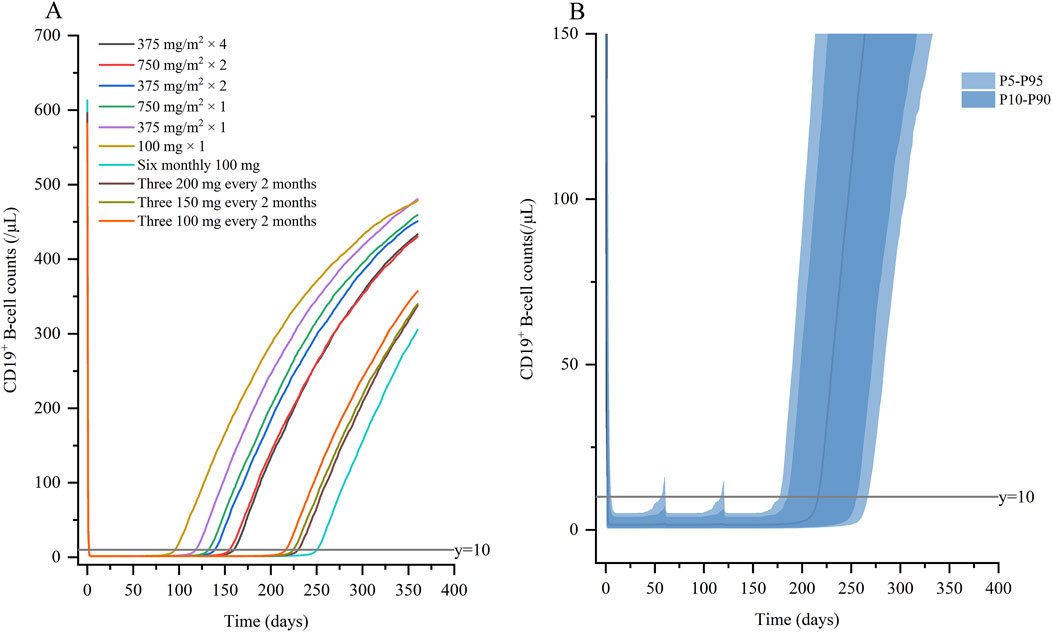
Figure 5. Simulated profiles of suppression of CD19+ B-cell (A) under variable dosing regimens of rituximab; (B) for three 100 mg every 2 months. Percentile bands of simulated data are represented in different shades of blue.
4 Discussion
A nomogram was established for predicting relapse to guide timing of additional dosing, which not only confirmed the relationship between B-cell repletion and relapse, but also identified NLR, U-IgG/Cr and U-TF as predictors in pediatric INS for the first time. Second, a K-PD model was first built in pediatric INS to predict B-cell repletion and explore new dosing regimens.
NLR was positively associated with risk of relapse (HR = 1.265; 95% CI: 1.080–1.481; p = 0.003) with a cutoff value of 2.13. Our finding was similarly to two previous studies in patients with IgA nephropathy, one showing NLR <2.43 as an effective predictor of corticosteroid response (HR = 1.252; 95% CI: 1.097–1.407; p < 0.001) (Yang et al., 2019), the other showing NLR >2.67 as an independent risk factor for diseases progression (HR = 1.74; 95% CI: 0.98–3.05; p = 0.043) (Wang et al., 2021). Furthermore, higher NLR (>2.41) was an independent indicator for poor renal prognosis in IgA vasculitis with nephritis (HR = 2.689; 95% CI: 1.044–6.927; p = 0.040) (Li et al., 2022). NLR reflected the balance between inflammatory and immune response. Increased NLR indicated an ongoing nonspecific inflammatory pathway and relatively inadequate immunity status, resulting in decline in ability to resist disease (Dong et al., 2023). Inflammation was the most important trigger of progressive tubulointerstitial fibrosis and renal scarring (Wang et al., 2021).
Another two biomarkers were U-IgG/Cr and U-TF. Massive excretion of high molecular weight protein (IgG) reflected severe disruption of glomerular selectivity permeability, while increased U-TF level was due to decreased tubular reabsorption, both of which were associated with tubulointerstitial inflammation and damage (Bazzi et al., 2001; Branten et al., 2005; Tang et al., 2002). Previous studies in patients with PMN reported that patients with U-IgG/Cr <110 mg/g had higher remission and lower progression to chronic renal failure (CKD) (Bazzi et al., 2001), and that U-IgG >250 mg/24 h was an independent predictor for development of renal insufficiency (Branten et al., 2005). Besides, IgG fractional excretion was a good predictor of CKD progression and responsiveness to immunosuppressants in IgA nephropathy (Bazzi, 2014). Also, U-TF demonstrated an excellent ability to predict active lupus nephritis and response to rituximab (Davies et al., 2021). And a study in children with HSP showed that U-IgG (OR = 1.48; 95% CI: 1.23–1.85; p < 0.010) and U-TF (OR = 1.50; 95% CI: 1.17–2.22; p = 0.013) were significantly associated with progression of renal involvement (Liu et al., 2019). Of note, PSI, the ratio between the urine clearance of IgG and that of TF, proven to be a good predictor of rituximab response in adult INS (Allinovi et al., 2022).
In clinical practice, depletion and repletion of CD19+ B-cell was a pharmacologic marker to follow rituximab response and relapse. An earlier study enrolled 46 children with multi-drug-dependent NS (Ravani et al., 2013), suggested that successful response to rituximab was associated with longer time to CD20 B-cell repletion, which was 185 (95% CI: 104–267) days in children who achieved prednisone and calcineurin-inhibitor-free remission for 6 months versus those who did not [109 (45–172) days; p = 0.01]. Another study of 37 children with complicated SDNS, early B-cell repletion (<5 months) was an independent risk factor for early relapse (<12 months) after rituximab treatment (Fujinaga and Hirano, 2014). Recently, a study involving 61 children with SDNS showed one-year relapse-free survival was significantly associated with time to B-cell depletion [HR of relapse 0.78 (0.63–0.97) per month of B-cell depletion] (Hogan et al., 2019). In addition, a retrospective cohort study of children with FRSDNS concluded that longer relapse-free periods were associated with longer B-cell depletion period (>6.0 months, HR = 0.36; 95% CI: 0.22–0.61; p < 0.001) (Choi et al., 2024). These findings were consistent with our result, whereby longer B-cell depletion period (>5.7 months; HR = 0.786; 95% CI: 0.635–0.972, p = 0.026) had a good prevention for relapse. The efficacy of B-cell-depleting agents (e.g., rituximab) at maintaining long-term remission supported an immune mediated etiology and emphasized a central role for B-cells in INS pathogenesis. Recently, the identification of pathogenic antibodies directed against podocytes, such as anti-nephrin antibodies reinforced the idea of the involvement of B-cells, and in particular antibody-secreting B-cells, in the development of INS. Finally, relapse of INS often coincided with an immunity-triggering event, including respiratory tract infections, gastroenteritis, and allergic reactions, reminding us of the importance of humoral immunity in the development of this disease (Al-Aubodah et al., 2024; Dorval et al., 2024).
Next, we developed a K-PD model including IgA to predict B-cell repletion and to estimate individual dose. We showed that the K-PD model was able to uncover underlying PK information in the absence of rituximab concentrations, especially for pediatric patients with difficulty in blood collection. The t1/2 of rituximab (14.1 days) was comparable to that reported in previous study, such as PMN (14.7 days) (Liang et al., 2024), rheumatoid arthritis (17.3 days) (Lioger et al., 2017) and autoimmune diseases (19.3 days) (Pan et al., 2019). Compared to current K-PD models of rituximab in autoimmune diseases (Pan et al., 2019; Riva et al., 2023), the t1/2 of CD19+ B-cell (99 days) was higher than that reported by the former (35 days), but lower than the latter (173 days), and ED50 was similar (1.31 versus 0.81 versus 0.692 mg), while Emax were higher (390 versus 35.2 versus 155 mg). Baseline IgA levels as covariate of ED50, were further included to improve the model. Patients with higher IgA levels required higher rituximab dose, meaning that those had a shorter time to B-cell repletion at the same dose. Consistent with the results from a retrospective cohort study of 224 patients with autoimmune diseases, higher levels of IgA (HR = 1.21, 95% CI: 1.01–1.45, p = 0.040) were positively associated with B-cell repletion (Nie et al., 2023). In addition, two studied in patients with rheumatoid arthritis demonstrated that a negative correlation between baseline IgA levels and time to B-cell repletion (p = 0.007), and patients with elevated baseline IgA levels had significantly higher B-cell counts 5 months after rituximab compared to those with normal IgA levels (p = 0.04) (De La Torre et al., 2012a; De La Torre et al., 2012b). The association of serum IgA with B-cell kinetics is not so clear, but suggests that microbial dysbiosis or infection-triggered mucosal immunity may play a role. The mucosal immune system was known to primarily consist of lymphoid tissues that were distributed in the skin, oral cavity, respiratory tract, urinary tract, and the gastrointestinal tract. IgA as the first line of defense against bacterial and viral infection, which was predominant at mucosa immunity response (He et al., 2020). Children with INS encountered multiple relapses, at least 50% of which were triggered by infection, the most common of which are respiratory, gastrointestinal and urinary tract infections (Uwaezuoke, 2015; Christian et al., 2022). Dysbiosis of oral or gut microbiota might occur in children with relapsing INS (Kawalec and Kiliś-Pstrusińska, 2022; Ye et al., 2024). It has been demonstrated in patients with IgA nephropathy, microbial dysbiosis or infections prime mucosal B-cell activation, leading to overproduction of IgA (Floege and Feehally, 2016). It has previously been reported that early recovery in serum IgA levels after rituximab was due to rapid replenishment of peripherally circulating IgA+ plasmablasts/plasma cells which were derived from mucosal B-cells in response to microbial stimulation, leading to subsequent flares and continuous production of autoantibodies. In autoimmune patients, circulating autoreactive plasmablasts can contribute to systemic autoantibody production (Mei et al., 2010; Kridin and Ahmed, 2020).
Although the off-label use of RTX was widely adopted for INS treatment, the optimal dose was still not known. Based on covariate model, simulation showed that B-cell depletion could last for more than 8 months with six monthly 100 mg doses and three 200 mg every 2 monthly. The cumulative dose of simulation was equal to that in patients with BSA of 0.82 m2 (median value of our population) based on two weekly 375 mg/m2. We further explored reduced dose and recommend that a minimum dose of three 150 mg every 2 monthly for children with INS. The cumulative dose of three 150 mg every two monthly was reduced by 165 mg compared with two weekly 375 mg/m2. According to the price of approximately 2294 RMB per 100 mg rituximab in China, 165 mg rituximab could result in costs of approximately 3785 RMB, which exceeded the average monthly income of Chinese residents (3268 RMB). Three 100 mg every 2 monthly only maintained B-cell depletion for more than 7 months in 90% of children. Children above 90% percentile experienced a transient B-cell repletion before the next dose, which might lead to relapse. This was comparable with previously published papers on rituximab for PMN. One study reported that a single dose of rituximab 100 mg could achieve B-cell depletion in 87.5% of individuals, and this depletion could be maintained for at least 1 month. Monthly rituximab 100 mg appeared as a potential effective regimen for treating PMN (Wang et al., 2023). The other study built a population PK-PD model for rituximab and recommended the novel regimen (6 monthly 100 mg) with the comparable ability and superior duration time of B-cell depletion (>7 months) compared with standard dose (four weekly 375 mg/m2), while simulation of a dose of 100 mg every 2 months was insufficient for long time B-cell depletion, supporting our results (Liang et al., 2024). Standard regimen could not fully exploit the potential of rituximab. The maintenance of a minimum level of drug for a prolonged time, seemed to be more important rather than the rapid achievement of a very high dose for a shorter time, meanwhile the lowering rituximab dose would yield reduced medical costs and safety risk.
The main limitation of this study was its retrospective design. However, the inclusion of a large number of pediatric patients with long-term follow-up, were the major strength of this study. Another limitation was the study design did not include histology because it had not been identified as a significant predictor (Chan et al., 2020). Future studies should aim to incorporate histological findings, as they might offer additional predictive value.
5 Conclusion
We demonstrated that duration of B-cell depletion was an important predictor of relapse in children with INS and established an accurate nomogram. Then, we built a reliable K-PD model to predict post-rituximab B-cell repletion in the absence of PK concentrations. The nomogram indicated optimal infusion timing before relapse and the K-PD model provided tailored treatment for children with INS to reduce safety risks and financial burden. In future studies, the accuracy of the nomogram and K-PD model need to be verified in clinical practice.
Data availability statement
The original contributions presented in the study are included in the article/Supplementary Material, further inquiries can be directed to the corresponding authors.
Ethics statement
The studies involving humans were approved by the Institutional Review Boards of Children’ Hospital of Fudan University [2022 (346)]. The studies were conducted in accordance with the local legislation and institutional requirements. The ethics committee/institutional review board waived the requirement of written informed consent for participation from the participants or the participants’ legal guardians/next of kin because Informed consent was waived due to all data was fully anonymized.
Author contributions
ZiL: Conceptualization, Formal Analysis, Funding acquisition, Methodology, Validation, Visualization, Writing–original draft, Writing–review and editing. QS: Data curation, Writing–review and editing. HX: Supervision, Writing–review and editing. ZhL: Supervision, Writing–review and editing.
Funding
The author(s) declare that financial support was received for the research, authorship, and/or publication of this article. This work was supported by the Youth Program of Children’s Hospital of Fudan University (No. EKQM202437).
Conflict of interest
The authors declare that the research was conducted in the absence of any commercial or financial relationships that could be construed as a potential conflict of interest.
Generative AI statement
The author(s) declare that no Generative AI was used in the creation of this manuscript.
Publisher’s note
All claims expressed in this article are solely those of the authors and do not necessarily represent those of their affiliated organizations, or those of the publisher, the editors and the reviewers. Any product that may be evaluated in this article, or claim that may be made by its manufacturer, is not guaranteed or endorsed by the publisher.
Supplementary material
The Supplementary Material for this article can be found online at: https://www.frontiersin.org/articles/10.3389/fphar.2024.1526936/full#supplementary-material
References
Adamichou, C., Genitsaridi, I., Nikolopoulos, D., Nikoloudaki, M., Repa, A., Bortoluzzi, A., et al. (2021). Lupus or not? SLE Risk Probability Index (SLERPI): a simple, clinician-friendly machine learning-based model to assist the diagnosis of systemic lupus erythematosus. Ann. Rheum. Dis. 80 (6), 758–766. doi:10.1136/annrheumdis-2020-219069
Al-Aubodah, T. A., Piccirillo, C. A., Trachtman, H., and Takano, T. (2024). The autoimmune architecture of childhood idiopathic nephrotic syndrome. Kidney Int. S0085-2538 (24), 00804–4. doi:10.1016/j.kint.2024.10.027
Allinovi, M., Trivioli, G., Lugli, G., Villanti, M., Gianassi, I., Antognoli, G., et al. (2022). Proteinuria selectivity index predicts response to rituximab in adults with minimal change disease and focal segmental glomerulosclerosis. Nephrol. Dial. Transpl. 37 (4), 789–791. doi:10.1093/ndt/gfab323
Bazzi, C. (2014). Toward 100% prediction of progression by fractional excretion of IgG in combination with nephron loss in IgA nephropathy. Kidney Int. 86 (5), 1062. doi:10.1038/ki.2014.247
Bazzi, C., Petrini, C., Rizza, V., Arrigo, G., Beltrame, A., Pisano, L., et al. (2001). Urinary excretion of IgG and alpha(1)-microglobulin predicts clinical course better than extent of proteinuria in membranous nephropathy. Am. J. Kidney. Dis. 38 (2), 240–248. doi:10.1053/ajkd.2001.26080
Branten, A. J., du Buf-Vereijken, P. W., Klasen, I. S., Bosch, F. H., Feith, G. W., Hollander, D. A., et al. (2005). Urinary excretion of beta2-microglobulin and IgG predict prognosis in idiopathic membranous nephropathy: a validation study. J. Am. Soc. Nephrol. 16 (1), 169–174. doi:10.1681/ASN.2004040287
Chan, E. Y., and Tullus, K. (2021). Rituximab in children with steroid sensitive nephrotic syndrome: in quest of the optimal regimen. Pediatr. Nephrol. 36 (6), 1397–1405. doi:10.1007/s00467-020-04609-0
Chan, E. Y., Webb, H., Yu, E., Ghiggeri, G. M., Kemper, M. J., Ma, A. L., et al. (2020). Both the rituximab dose and maintenance immunosuppression in steroid-dependent/frequently-relapsing nephrotic syndrome have important effects on outcomes. Kidney Int. 97 (2), 393–401. doi:10.1016/j.kint.2019.09.033
Chan, E. Y., Yap, D. Y., Colucci, M., Ma, A. L., Parekh, R. S., and Tullus, K. (2023). Use of rituximab in childhood idiopathic nephrotic syndrome. Clin. J. Am. Soc. Nephrol. 18 (4), 533–548. doi:10.2215/CJN.08570722
Chan, E. Y., Yu, E. L. M., Angeletti, A., Arslan, Z., Basu, B., Boyer, O., et al. (2022). Long-term efficacy and safety of repeated rituximab to maintain remission in idiopathic childhood nephrotic syndrome: an international study. J. Am. Soc. Nephrol. 33 (6), 1193–1207. doi:10.1681/ASN.2021111472
Choi, N., Min, J., Kim, J. H., Kang, H. G., and Ahn, Y. H. (2024). Efficacy and safety of long-term repeated use of rituximab in pediatric patients with nephrotic syndrome. Pediatr. Nephrol. 39 (3), 771–780. doi:10.1007/s00467-023-06124-4
Christian, M. T., Webb, N. J. A., Mehta, S., Woolley, R. L., Afentou, N., Frew, E., et al. (2022). Evaluation of daily low-dose prednisolone during upper respiratory tract infection to prevent relapse in children with relapsing steroid-sensitive nephrotic syndrome: the PREDNOS 2 randomized clinical trial. J.A.M.A. Pediatr. 176 (3), 236–243. doi:10.1001/jamapediatrics.2021.5189
Colucci, M., Carsetti, R., Cascioli, S., Casiraghi, F., Perna, A., Ravà, L., et al. (2016). B cell reconstitution after rituximab treatment in idiopathic nephrotic syndrome. J. Am. Soc. Nephrol. 27 (6), 1811–1822. doi:10.1681/ASN.2015050523
Davies, J. C., Carlsson, E., Midgley, A., Smith, E. M. D., Bruce, I. N., Beresford, M. W., et al. (2021). A panel of urinary proteins predicts active lupus nephritis and response to rituximab treatment. Rheumatol. Oxf. 60 (8), 3747–3759. doi:10.1093/rheumatology/keaa851
De La Torre, I., Leandro, M. J., Edwards, J. C., and Cambridge, G. (2012a). Baseline serum immunoglobulin levels in patients with rheumatoid arthritis: relationships with clinical parameters and with B-cell dynamics following rituximab. Clin. Exp. Rheumatol. 30 (4), 554–560.
De La Torre, I., Leandro, M. J., Valor, L., Becerra, E., Edwards, J. C., and Cambridge, G. (2012b). Total serum immunoglobulin levels in patients with RA after multiple B-cell depletion cycles based on rituximab: relationship with B-cell kinetics. Rheumatol. Oxf. 51 (5), 833–840. doi:10.1093/rheumatology/ker417
Dietrich, S., Floegel, A., Troll, M., Kühn, T., Rathmann, W., Peters, A., et al. (2016). Random Survival Forest in practice: a method for modelling complex metabolomics data in time to event analysis. Int. J. Epidemiol. 45 (5), 1406–1420. doi:10.1093/ije/dyw145
Dong, G., Gan, M., Xu, S., Xie, Y., Zhou, M., and Wu, L. (2023). The neutrophil-lymphocyte ratio as a risk factor for all-cause and cardiovascular mortality among individuals with diabetes: evidence from the NHANES 2003–2016. Cardiovasc. Diabetol. 22 (1), 267. doi:10.1186/s12933-023-01998-y
Dorval, G., Colucci, M., and Boyer, O. (2024). B-cell repertoire and functions in idiopathic nephrotic syndrome: what to learn from single-cell RNA sequencing? Kidney Int. 106 (4), 556–559. doi:10.1016/j.kint.2024.05.032
Floege, J., and Feehally, J. (2016). The mucosa-kidney axis in IgA nephropathy. Nat. Rev. Nephrol. 12 (3), 147–156. doi:10.1038/nrneph.2015.208
Fujinaga, S., and Hirano, D. (2014). Risk factors for early relapse during maintenance therapy after a single infusion of rituximab in children with steroid-dependent nephrotic syndrome. Pediatr. Nephrol. 29 (3), 491–492. doi:10.1007/s00467-013-2674-6
He, J. W., Zhou, X. J., Lv, J. C., and Zhang, H. (2020). Perspectives on how mucosal immune responses, infections and gut microbiome shape IgA nephropathy and future therapies. Theranostics 10 (25), 11462–11478. doi:10.7150/thno.49778
Hogan, J., Dossier, C., Kwon, T., Macher, M. A., Maisin, A., Couderc, A., et al. (2019). Effect of different rituximab regimens on B cell depletion and time to relapse in children with steroid-dependent nephrotic syndrome. Pediatr. Nephrol. 34 (2), 253–259. doi:10.1007/s00467-018-4052-x
Kawalec, A., and Kiliś-Pstrusińska, K. (2022). Gut microbiota alterations and primary glomerulonephritis in children: a review. Int. J. Mol. Sci. 24 (1), 574. doi:10.3390/ijms24010574
Kridin, K., and Ahmed, A. R. (2020). Post-rituximab immunoglobulin M (IgM) hypogammaglobulinemia. Autoimmun. Rev. 19 (3), 102466. doi:10.1016/j.autrev.2020.102466
Lee, A. M., Hu, J., Xu, Y., Abraham, A. G., Xiao, R., Coresh, J., et al. (2022). Using machine learning to identify metabolomic signatures of pediatric chronic kidney disease etiology. J. Am. Soc. Nephrol. 33 (2), 375–386. doi:10.1681/ASN.2021040538
Li, Q., Shi, S., Liu, L., Lv, J., Zhu, L., and Zhang, H. (2022). Neutrophil-to-lymphocyte ratio as an independent inflammatory indicator for poor renal prognosis in adult IgA vasculitis with nephritis. Int. Immunopharmacol. 111, 109178. doi:10.1016/j.intimp.2022.109178
Liang, H., Deng, Z., Niu, S., Kong, W., Liu, Y., Wang, S., et al. (2024). Dosing optimization of rituximab for primary membranous nephropathy by population pharmacokinetic and pharmacodynamic study. Front. Pharmacol. 15, 1197651. doi:10.3389/fphar.2024.1197651
Lioger, B., Edupuganti, S. R., Mulleman, D., Passot, C., Desvignes, C., Bejan-Angoulvant, T., et al. (2017). Antigenic burden and serum IgG concentrations influence rituximab pharmacokinetics in rheumatoid arthritis patients. Br. J. Clin. Pharmacol. 83 (8), 1773–1781. doi:10.1111/bcp.13270
Liu, H., Cui, W., Liu, H., and Zhang, C. (2019). Predicative value of urinary protein biomarkers on delayed renal involvement in children with Henoch-Schönlein purpura. Sci. China Life Sci. 62 (12), 1594–1596. doi:10.1007/s11427-018-9544-0
Mei, H. E., Frölich, D., Giesecke, C., Loddenkemper, C., Reiter, K., Schmidt, S., et al. (2010). Steady-state generation of mucosal IgA+ plasmablasts is not abrogated by B-cell depletion therapy with rituximab. Blood 116 (24), 5181–5190. doi:10.1182/blood-2010-01-266536
Nie, Y., Li, J., Wu, D., Yang, Y., Zhang, L., Bai, W., et al. (2023). B cell repopulation trajectory after rituximab treatment in autoimmune diseases: a longitudinal observational study. Clin. Exp. Med. 23 (8), 4787–4795. doi:10.1007/s10238-023-01186-y
Pan, S., Yu, H., Surti, A., Cheng, I., Marks, S. D., Brogan, P. A., et al. (2019). Pharmacodynamics of rituximab on B lymphocytes in paediatric patients with autoimmune diseases. Br. J. Clin. Pharmacol. 85 (8), 1790–1797. doi:10.1111/bcp.13970
Prasad, N., Jaiswal, A. K., Agarwal, V., Yadav, B., Sharma, R. K., Rai, M., et al. (2015). Differential alteration in peripheral T-regulatory and T-effector cells with change in P-glycoprotein expression in Childhood Nephrotic Syndrome: a longitudinal study. Cytokine 72 (2), 190–196. doi:10.1016/j.cyto.2014.12.028
Ravani, P., Ponticelli, A., Siciliano, C., Fornoni, A., Magnasco, A., Sica, F., et al. (2013). Rituximab is a safe and effective long-term treatment for children with steroid and calcineurin inhibitor-dependent idiopathic nephrotic syndrome. Kidney Int. 84 (5), 1025–1033. doi:10.1038/ki.2013.211
Riva, N., Brstilo, L., Sancho-Araiz, A., Molina, M., Savransky, A., Roffé, G., et al. (2023). Population pharmacodynamic modelling of the CD19+ suppression effects of rituximab in paediatric patients with neurological and autoimmune diseases. Pharmaceutics 15 (11), 2534. doi:10.3390/pharmaceutics15112534
Schoergenhofer, C., Schwameis, M., Firbas, C., Bartko, J., Derhaschnig, U., Mader, R. M., et al. (2018). Single, very low rituximab doses in healthy volunteers - a pilot and a randomized trial: implications for dosing and biosimilarity testing. Sci. Rep. 8 (1), 124. doi:10.1038/s41598-017-17934-6
Sinha, A., Mathew, G., Arushi, A., Govindarajan, S., Ghanapriya, K., Grewal, N., et al. (2023). Sequential rituximab therapy sustains remission of nephrotic syndrome but carries high risk of adverse effects. Nephrol. Dial. Transpl. 38 (4), 939–949. doi:10.1093/ndt/gfac228
Tang, S., Leung, J. C., Tsang, A. W., Lan, H. Y., Chan, T. M., and Lai, K. N. (2002). Transferrin up-regulates chemokine synthesis by human proximal tubular epithelial cells: implication on mechanism of tubuloglomerular communication in glomerulopathic proteinura. Kidney. Int. 61 (5), 1655–1665. doi:10.1046/j.1523-1755.2002.00301.x
Trautmann, A., Boyer, O., Hodson, E., Bagga, A., Gipson, D. S., Samuel, S., et al. (2023). IPNA clinical practice recommendations for the diagnosis and management of children with steroid-sensitive nephrotic syndrome. Pediatr. Nephrol. 38 (3), 877–919. doi:10.1007/s00467-022-05739-3
Uwaezuoke, S. N. (2015). Steroid-sensitive nephrotic syndrome in children: triggers of relapse and evolving hypotheses on pathogenesis. Ital. J. Pediatr. 41, 19. doi:10.1186/s13052-015-0123-9
Vivarelli, M., Gibson, K., Sinha, A., and Boyer, O. (2023). Childhood nephrotic syndrome. Lancet 402 (10404), 809–824. doi:10.1016/S0140-6736(23)01051-6
Wang, S., Deng, Z., Wang, Y., Bao, W., Zhou, S., Cui, Z., et al. (2023). Monthly mini-dose rituximab for primary anti-PLA2R-positive membranous nephropathy: a personalized approach. B.M.C. Nephrol. 24 (1), 146. doi:10.1186/s12882-023-03206-1
Wang, S., Dong, L., Pei, G., Jiang, Z., Qin, A., Tan, J., et al. (2021). High neutrophil-to-lymphocyte ratio is an independent risk factor for end stage renal diseases in IgA nephropathy. Front. Immunol. 12, 700224. doi:10.3389/fimmu.2021.700224
Webb, N. J. A., Woolley, R. L., Lambe, T., Frew, E., Brettell, E. A., Barsoum, E. N., et al. (2019). Long term tapering versus standard prednisolone treatment for first episode of childhood nephrotic syndrome: phase III randomised controlled trial and economic evaluation. B. M. J. 365, l1800. doi:10.1136/bmj.l1800
Yang, H., Zhang, W., Li, Y., and Li, R. (2019). Neutrophil-to-lymphocyte ratio: an effective predictor of corticosteroid response in IgA nephropathy. Int. Immunopharmacol. 74, 105678. doi:10.1016/j.intimp.2019.105678
Ye, Q., Liu, H., Meng, H., Wang, D., Zhang, J., Zhu, S., et al. (2024). Comprehensive mapping of saliva by multiomics in children with idiopathic nephrotic syndrome. Nephrol. Carlt. 29 (9), 565–578. doi:10.1111/nep.14308
Keywords: rituximab, idiopathic nephrotic syndrome, kinetic-pharmacodynamic model, relapse, B-cell
Citation: Li Z, Shen Q, Xu H and Li Z (2025) Kinetic-pharmacodynamic model to predict post-rituximab B-cell repletion as a predictor of relapse in pediatric idiopathic nephrotic syndrome. Front. Pharmacol. 15:1526936. doi: 10.3389/fphar.2024.1526936
Received: 12 November 2024; Accepted: 17 December 2024;
Published: 07 January 2025.
Edited by:
Yang Zhou, Brown University, United StatesReviewed by:
Catherine M. T. Sherwin, University of Western Australia, AustraliaSaurabh Chaturvedi, Delhi Pharmaceutical Sciences and Research University, India
Copyright © 2025 Li, Shen, Xu and Li. This is an open-access article distributed under the terms of the Creative Commons Attribution License (CC BY). The use, distribution or reproduction in other forums is permitted, provided the original author(s) and the copyright owner(s) are credited and that the original publication in this journal is cited, in accordance with accepted academic practice. No use, distribution or reproduction is permitted which does not comply with these terms.
*Correspondence: Zhiping Li, NzgxMjczNDU3QHFxLmNvbQ==; Hong Xu, aHh1QHNobXUuZWR1LmNu
†These authors have contributed equally to this work