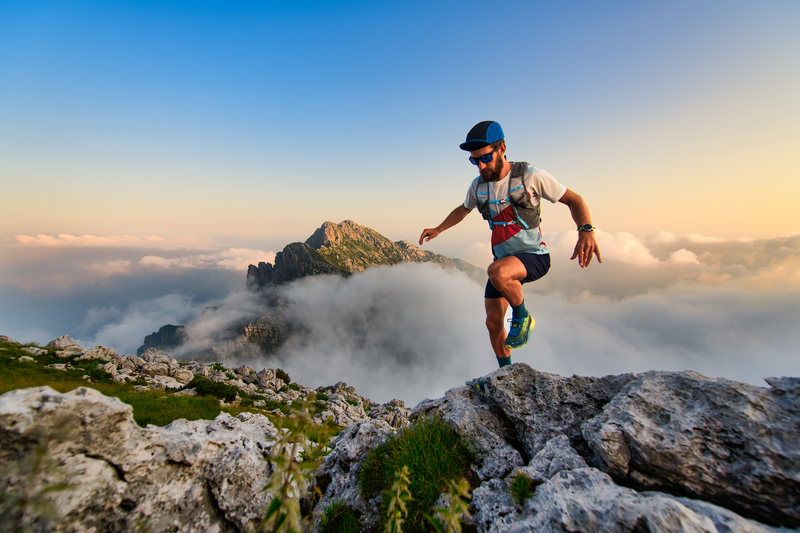
94% of researchers rate our articles as excellent or good
Learn more about the work of our research integrity team to safeguard the quality of each article we publish.
Find out more
ORIGINAL RESEARCH article
Front. Pharmacol. , 09 January 2025
Sec. Pharmacology of Anti-Cancer Drugs
Volume 15 - 2024 | https://doi.org/10.3389/fphar.2024.1512864
This article is part of the Research Topic AI Research in Cancer Pharmacology View all 3 articles
Platelet-derived growth factor alpha (PDGFRA) plays a significant role in various malignant tumors. PDGFRA expression boosts thyroid cancer cell proliferation and metastasis. Radiorefractory thyroid cancer is poorly differentiated, very aggressive, and resistant to radioiodine therapy. Thus, novel anticancer drugs that inhibit its metastasis are urgently required. In this context, we proposed the PDGFRA inhibitors by an optimized structure-based drug design approach. We performed a virtual screening of metabolites derived from anticancer medicinal plants (Swertia chirayita, Myristica fragrans, and Datura metel) and successfully identified seven hits, namely cis-Grossamide K, Daturafoliside O, N-cis-feruloyltyramine, Maceneolignan H, Erythro-2-(4-allyl-2, 6-dimethoxyphenoxy)-1-(3, 4, 5-trimethoxyphenyl) propan-1, 3-diol, Myrifralignan C, and stigmasteryl-3-O-β-glucoside as potential PDGFRA inhibitors. Not only the top 7 hits exhibited higher docking scores in docking simulation but also optimal drug-likeness and non-toxic profiles in pharmacokinetics analysis among 119 compounds. Our top hits are non-mutagenic, can cross the blood-brain barrier, and inhibit p-glycoprotein, while the N-cis-feruloyltyramine has the potential to become a lead compound. The protein-ligand stability of the top 3 hits, namely cis-Grossamide K, Daturafoliside O, and N-cis-feruloyltyramine, and their interactions at the potential binding site of target protein were confirmed through molecular dynamic simulations. We also analyzed pharmacophoric features for stable binding in the PDGFRA active site. These drug candidates were further characterized to predict their biological activity spectra in the human body and medicinal characteristics to know their extensive behavior in laboratory testing. This study necessitates the in-vitro and in-vivo studies to confirm the potential of our hits for the discovery of novel therapeutics against the thyroid cancer.
Multiple variations or mutations in gene expression cause an imbalance in cellular proliferation, subsequently leading to cancerous cell development. Cancer has been identified as the most prevalent cause of mortality in developed as well as developing countries globally over the years, making it a chronic public health concern (Choudhari et al., 2020). A study reports that, by 2050, there will probably be 35 million novel cases of cancer reported yearly and 77% increase from 2022 level (Bray et al., 2024). Cancerous cells hold peculiar characteristics such as continued development signal of self-sufficiency, uncontrolled replication, gene instability, resisting programmed cell death, ongoing angiogenesis, and a variety of mutations (Madhavan et al., 2021).
Platelet-derived growth factor receptors alpha and beta (PDGFRA and PDGFRB) are membrane receptors that are involved in transduction of extra-cellular signals into cells, which is a critical process in a number of diseases, including many types of cancer, such as prostate cancer, ovarian, breast, pancreatic, and liver cancer, immune-mediated pathologies like systemic sclerosis (SSc), and viral infections (Mozzicafreddo et al., 2023). PDGFRs are glycoprotein dimer molecules comprised of an extracellular ligand-binding region separated into five Ig-like domains that connect to an intracellular receptor tyrosine kinase (RTK) domain via a single transmembrane alpha helix. PDGFRA binds to its corresponding ligand, platelet-derived growth factor (PDGF), and subsequently activates downstream signaling pathways to regulate cell proliferation, migration, and angiogenesis. However, these signaling pathways can also cause endothelial cell adhesion, collagen synthesis, and myofibroblast proliferation and chemotaxis. They are increased in pathological situations, which are also associated with viral infections, in patients suffering from SSc or other progressive fibrotic diseases (Alvarez et al., 2006). Overexpression of PDGFRA is strongly linked to radioiodine resistance and distant metastasis in human thyroid cancer, particularly radiorefractory thyroid cancer (RAIR-TC). In all cell and animal models, overexpression and abnormal activation of PDGFRA lowered thyroglobulin protein (TG) and sodium-iodide symporter (NIS) expression levels via altering thyroid transcription factor 1 (TTF1) transcriptional activity and nuclear translocation. Moreover, the findings show that when PDGFRA was suppressed, radioiodine absorption was restored, although migration, invasion potential, and tumor burden were all reduced (Salem et al., 2020).
Because the human PDGFRA has emerged as one of the most significant therapeutic targets for the aforementioned diseases, its modulation or inhibition is an important aspect that directs the pursuit of novel ligands or newly acquired information for developing novel, potent drugs, particularly for the treatment of cancer. Recently, Sorafenib was approved as a PDGFRA inhibitor by the National Medical Product Administration, and Lenvatinib has now entered clinical trials. While Sorafenib is known to cause severe hypertension along with fatigue, diarrhea, hand-foot skin reactions, and treatment-emerging adverse effects (Hansen et al., 2017), Lenvatinib showed rare side effects like encephalopathy syndrome and takotsubo cardiomyopathy added to hypertension, weight loss, and fatigue (Hamidi et al., 2022).
Structure-based drug design (SBDD), which stimulates the development of new drugs with a potential affinity for therapeutic targets, has proven to be an invaluable resource for rapid and cost-effective lead identification. SBDD is a dynamic, iterative, and effective strategy for drug development that involves the structural evaluation of targets. It has the potential to lower the time and expense of creating novel drug-lead compounds with therapeutic applications. In the current study, we applied the bioinformatics approach to demonstrate the correlation between PDGFRA and radioiodine resistance in thyroid cancer and created a molecular docking and molecular dynamic simulation model of PDGFRA inhibitors. In this regard, we selected three herbal medicinal plants by thorough literature survey that are also reported to have anti-cancer activity, namely Swertia chirayita (Roxb.) H. Karst. [Gentianaceae] (Verma et al., 2024), Datura metel L. [Solanaceae] (Islam et al., 2023), and Myristica fragrans Houtt. [Myristicacea] (Prakash and Gupta, 2013). We prepared an in-house library of 804 phytochemicals from these anti-cancer plants and screened them against PDGFRA to predict the best binding affinities. Post-docking analysis consisting of in-depth biological and metabolic behavior of top hits within the human body, followed by pharmacokinetic and pharmacophoric analysis, molecular dynamic simulations, biological activity spectra, and medicinal characteristics to assist in the treatment of radiorefractory thyroid cancer (RAIR-TC) more safely and potentially.
By an extensive literature survey, we identified PDGFRA protein that belongs to the family of RTKs as a potent therapeutic target against cancer. Studies confirmed the role of PDGFRA mRNA and protein expression levels increased dramatically in thyroid cancer (Ong et al., 2018).
PDGFRA X-ray crystallographic structure was retrieved from PDB (ID: 6JOL) with a resolution of 1.9Å because protein structures with resolution between 1.5 and 2.5Å are promising for docking studies. Its structure contains an active site with the co-crystallized ligand imatinib and also has complete side chains.
The downloaded PDB structure of PDGFRA was refined in MOE (Molecular Operating Environment (MOE), 2022.02 Chemical Computing Group ULC, 1010 Sherbooke St. West, Suite #910, Montreal, QC, Canada, H3A 2R7, MOE2022. v11.18.1) for docking purposes. Firstly, all water molecules and extra ligands were removed from the protein structure to optimize the PDGFRA structure and to avoid any interference during docking. These eliminations cause empty spaces in the structure, which were filled by protonation with default parameters. After incorporating hydrogen ions, the protein structure was subjected to an energy minimization process to ensure stability using the Merck molecular force field (MMFF94x) and the conjugate gradient method (Dib et al., 2024).
The already reported binding site of PDGFRA was selected using the MOE site finder tool. This tool created dummy atoms at the active site residues to estimate the binding site within accessible pockets. Consequently, ligands were confined to interact only within these specified binding sites.
We investigated the three herbal medicinal plants known to possess anticancer activity by a broad literature survey. We prepared an in-house set of 804 phytochemicals from S. chirayita (125 metabolites), D. metel (414 metabolites), and M. fragrans (265 metabolites) (Figure 1). We selected native ligand imatinib as standard for our study.
Figure 1. (A) Percentage of metabolites from each selected plant, including Swertia chirayita (16%–125 metabolites), Datura metel (51%–414 metabolites), and Myristica fragrans (33%–265 metabolites); (B) Important active site residues of platelet-derived growth factor receptor alpha (PDGFRA). The demonstrated active site residues are frequently involved in catalytic binding of target receptors.
Imatinib was chosen as a standard drug due to its established efficacy as a tyrosine kinase inhibitor in the treatment of chronic myeloid leukemia (CML) and gastrointestinal stromal tumors (GISTs). Its ability to specifically inhibit PDGFRA makes it a relevant benchmark for evaluating the binding and inhibitory effects of novel compounds in our study (Joensuu et al., 2023).
The structures of standard and all ligands were downloaded from the PubCHEM database (https://pubchem.ncbi.nlm.nih.gov) or drawn in ChemDraw and saved in mdl mol format. In MOE, all structures were converted into mdb database for the docking process, followed by energy minimization (Muhammad et al., 2021).
Induced-fit docking was performed using MOE default parameters as performed previously (Shah et al., 2021; Dib et al., 2024). The results were obtained as S-scores with best-docked conformations of ligands. The S-score measures the optimized orientation, binding mode, and site of ligand and binding affinity between ligand and receptor. Five conformations of the protein-ligand complex were generated, and one with the best binding free energy and binding interactions was selected for subsequential analysis. A set of 804 phytochemicals and standard imatinib was docked against the PDGFRA receptor. The compounds showed an S-score higher than imatinib, which was selected for in-depth study.
To ensure the reliability and accuracy of screening by docking protocol, we used a standard validation process using PyMOL (The PyMOL Molecular Graphics System, Version 3.0 Schrödinger, LLC). The standard imatinib docked complex, prepared in MOE, was superimposed on the original PDB complex (PDB ID: 6JOL) to derive the RMSD (root mean square deviation) value. The acceptable RMSD value is less than 2Å, and superimposition validates the docking protocol as both complexes are aligned on each other at the same binding site (Shah et al., 2024b).
The drug-likeness analysis is the way of determining the capability of a compound or metabolite to be used as a drug. The drug-likeness rules by Lipinski et al. (1997), Viswanadhan et al. (2011), Veber et al. (2002), Egan et al. (2000), Muegge et al. (2001) and bioavailability score were employed to determine the drug-likeness of our compounds. The compounds showed docking scores higher than standard imatinib and were selected for drug-likeness analysis using the SwissADME server (http://www.swissadme.ch/).
The pharmacokinetic analysis of top compounds was executed by ADMETlab 3.0 (https://admetlab3.scbdd.com/), a web-based program that can determine ADMET (absorption, distribution, metabolism, excretion, and toxicity) properties. ADMET outlines the effects of drugs in the human body, which include human intestinal absorption, VDss, BBB permeability, CaCo2 cell line permeability, cytochrome P450 metabolic enzyme inhibition, clearance, the AMES test, and carcinogenicity, among others.
The Pharmacophore Query Editor in the MOE software package was used to develop an intricate pharmacophore model to investigate the molecular interactions of the top two active ligands within the PDGFRA receptor’s active site. This optimized model identified multiple essential pharmacophoric variables required for binding, such as hydrogen bond donors and acceptors, aromatic and Pi ring centers, hydrophobic atoms, and charged anionic and cationic atoms. To ensure precision, all pharmacophoric features were fine-tuned to a radius of 1.0 Å, with a tolerance of 1.2 and a minimum inclusion criterion of 50% (Ahmad et al., 2024).
The complexes were prepared by filling in the missing residues using SwissPDBViewer (Guex and Peitsch, 1997). Subsequently, followed by protonation adjustment using the APBS (Adaptive Poisson-Boltzmann Solver) web service (Jurrus et al., 2018). The visualization of the molecular poses of the ligand, exploring the different form configurations extracted over time, was performed using the PyMOL software (Schrödinger and DeLano, 2020).
The protein-ligand complex was subjected to study using the GROningenMAchine for Chemical Simulations (GROMACS) v2024.2 (Abraham et al., 2015). The atomistic simulations were conducted to analyze the structural changes of the complex formed by platelet-derived growth factor receptor alpha (PDGFRA) and natural inhibitors, utilizing the CHARMM36 all-atom force field based on the protocol already published (de Paula Junior et al., 2022). Subsequently, a “cubic” type box with edges of 2 nm was created for solvating the system with water molecules using TIP3P (Jorgensen et al., 1983). For the interactions studied, the following number of solvent (SOL) molecules were added: 25,374 for the PDGFRA-imatinib complex, 25,381 for the PDGFRA-daturafoliside O complex, 25,389 for the PDGFRA-N-cis-feruloyltyramine complex, and 25,379 for the PDGFRA-cis-Grossamide K complex. Additionally, ions were employed to neutralize the system. Electrostatic interactions were simulated using the fast Particle-Mesh Ewald approach, while van der Waals interactions (vdW) were calculated using the cutoff scheme. Initially, the complex underwent energy minimization using the steepest descent approach to remove bad contacts and clashes (Khan et al., 2024). The first step of equilibration was (constant number of particles, volume, and temperature) NVT ensemble using a V-rescale thermostat at 300 K, and pressure equilibration was attained using Berendsen coupling with a compressibility of 4.5 × 10−5 bar in NPT ensemble. Molecular dynamics (MD) simulations were conducted for a time interval of 100 ns under periodic boundary conditions. The MD results were subjected to various analyses using built-in modules of GROMACS, including the dynamic stability of the complex assessed via RMSD, the root mean square fluctuation (RMSF) of the protein residues, the radius of gyration (Rg) to determine the protein’s compactness, the number of hydrogen bond interactions between protein and ligand atoms, interaction energy (which computes both Coulombic short-range and Lennard-Jones short-range energy interactions), Gibbs free energy landscapes of the first two principal components (PC1 and PC2) evaluated using the gmx sham command, principal component analysis (PCA) performed with the gmx anaeig command using the -proj parameter, and the solvent-accessible surface area (SASA) evaluated in nm2, which is calculated using gmx sasa. These analyses were conducted to understand the protein folding dynamics and stability.
The Way2Drug informational computational platform (https://www.way2drug.com/passonline/predict.php) provides access to drug approval data in the United States and Russia, as well as computational capabilities for predicting the biological activity of drug-like organic compounds. The Prediction of Activity Spectra of Substances (PASS) algorithm examines the training set’s structure-activity relationships (SAR). It contains a considerable number of noncongeneric molecules with diverse biological activity (Poroikov et al., 2000). PASS prediction was performed on our recognized hits.
The SwissADME server, which assesses the efficacy of drug candidates in biological testing, was employed to determine the medicinal qualities of the top ligands. Permitted toxicity level, chemical susceptibility, potent reaction independent of the primary target, and synthetic accessibility were determined.
Cancer, a second-leading cause of mortality, is a genetic disorder characterized by the uncontrollable multiplication of aberrant cells in the body and their dissemination to other body parts (Gomes et al., 2021). Thyroid cancer is one of the most frequent endocrine tumors, with an increasing global incidence, and RAIR-TC has a tragic survival rate due to low radioiodine uptake. PDGFRA is known to minimize the production of TG protein and NIS by interrupting the transcriptional activity and nuclear localization of TTF1, reducing the uptake of radioiodine (Yu et al., 2022). PDGFRA inhibition led to the restoration of radioiodine absorption, while invasion potential and tumor burden can be dropped.
The MOE site-finding tool indicated several active sites, though only one active site previously reported in the literature was selected (Keretsu et al., 2020). The selected active site contains amino acid residues Arg597, Leu599, Lys627, Glu644, Val658, Leu660, Thr674, Cys677, Val815, His816, Arg817, Cys835, Asp836, and Phe856, among others. The important binding residues of this active site (Glu644, Met648, Leu660, Val815, His816, Arg817, Cys835, and Asp836) (Keretsu et al., 2020) are represented in Figure 1.
MOE software was used for docking, and ligands were graded according to their binding affinities (S-score). The highest S-scores are related to larger negative binding energies, indicating stronger binding affinity. S-scores estimate binding energies, which correlate with Gibbs free energy. Non-covalent interactions such as hydrogen bonds (H-bonds), ionic bonds, and hydrophobic interactions are critical for the stability and selectivity of protein-ligand complexes. H-bonds have a considerable effect on drug binding affinity to target receptors.
In this study, we screened 804 phytochemicals from three herbal anti-cancer plants against PDGFRA. Native ligand imatinib showed a docking score of −7.49 kcal/mol (Supplementary Table S1). Our 119 compounds showed docking scores greater than standard and were subjected to drug-likeness analyses. We further compared our results with other renowned standards of PDGFRA protein, i.e., Crenolanib, Lenvatinib, and Sorafenib. These drugs represent significant advances in targeting PDGFRA mutations in various cancers. Crenolanib is a potent inhibitor of imatinib-resistant PDGFRA mutations, such as the D842V mutation, making it effective for malignancies like gastrointestinal stromal tumors with PDGFRA mutations (Heinrich et al., 2012). Lenvatinib is a multi-kinase inhibitor targeting PDGFRA, showing efficacy in improving outcomes in radioiodine-refractory thyroid cancer and hepatocellular carcinoma (Zschäbitz and Grüllich, 2018) and lastly, Sorafenib, though less effective against PDGFRA D842V mutations, can inhibit certain PDGFRA-driven tumors and is used to treat PDGFRA-mutant cancers, particularly in cases of imatinib resistance (Lierman et al., 2006). Significantly, our more than half library showed docking scores greater than Crenolanib, Lenavatinib, and Sofarenib against PDGFRA. The docking scores of these standards and their comparisons with our hits have been described in Figure 2. Out of 119, we identified 7 hits that follow the acceptable druglikeness criteria. Among 804 phytochemicals, 36 were found to be inactive against the PDGFRA receptor (Supplementary Table S1). These inactive metabolites emerged based on their absence from the MOE docking simulation output files. This absence implies that the compounds did not have substantial binding affinity for PDGFRA, as indicated by the S-scores. Focusing on compounds with higher binding affinities increases selection efficiency, prioritizes active compounds for further assessment and optimization, and ensures that resources are directed toward those with the greatest therapeutic effects.
Figure 2. Docking score of various standards against PDGFRA and their comparisons with our hits, namely cis-Grossamide K, Daturafoliside O, and N-cis-feruloyltyramine.
Physicochemical parameters and drug-like assets are integral drug properties that must be addressed during the drug development process (Rasgania et al., 2023). The drug-likeness rules for the top 119 compounds, along with the standard drug imatinib, which have been identified as potent PDGFRA inhibitors with relatively high S-scores, were analyzed (Supplementary Table S2). Their drug-likeness is confirmed by rules, namely Lipinski, Ghose, Veber, EGAN, MUEGGE, and bioavailability score using the SwissADME server. According to the Lipinski rule, a most authentic chemo-informatics filter, the molecular weight of a drug should be less than 500 g/mol, with no more than 5 hydrogen bond donors and 10 acceptors, a logP value of less than 5, and a TPSA value of less than 140 Å2 (Lipinski et al., 1997). Ghose filter sets drug-likeness requirements as measured log P is between −0.4 and 5.6, molecular weight is between 160 and 480, molar refractivity is between 40 and 130, and total atom count is between 20 and 70 (Viswanadhan et al., 2011). Veber’s rule suggests a drug can attain adequate oral bioavailability if it has 10 or fewer rotatable bonds (RTB) and TPSA of 140 Å2 (Veber et al., 2002; Shah et al., 2024a). The Egan filter uses multivariate statistics to develop guidelines (logP ≤5.88, TPSA <131.6 Å2) for compounds that humans well and poorly absorb (Egan et al., 2000). When assessing membrane permeability, just two descriptors (logP and TPSA) were chosen to ensure good bioavailability. It is important to consider that bioavailability filters may remove substances that enter cells via carrier-mediated transport or active absorption. Muegge’s rule modified the property ranges and provided further specifications to distinguish between drug-like and nondrug-like molecules. These are MW (200–600), LogP (-2–5), PSA ≤150, number of rings (NR)≤7, number of carbons (NC) > 4, number of heteroatoms (NH) > 1, RB ≤ 15, HBD ≤5, and HBA ≤10 (Muegge et al., 2001). After detailed analysis of 119 compounds according to the standard drug-likeness rules (Table 1), we identified 7 hits that fall in standard drug-likeness criteria. These compounds are cis-Grossamide K (compound 1), Daturafoliside O (compound 2), N-cis-feruloyltyramine (compound 3), Maceneolignan H (compound 4), Erythro-2-(4-allyl-2,6-dimethoxyphenoxy)-1-(3,4,5-trimethoxyphenyl) propan-1,3-diol (compound 5), Myrifralignan C (compound 6), and stigmasteryl-3-O-β-glucoside (compound 7).
Our all hits follow the Lipinski rule and the Egan rule completely. Compounds 1, 2, and 7 showed some violations of Ghose filters. Compounds 4 and 5 have one violation of the Veber filter. Compound 7 showed only one violation of Muegge filter. The bioavailability score of 0.55 validates that all the compounds have good absorption (Oashi et al., 2011). These remarkable results confirm that our hits share structural similarities with ideal drugs. Our hits satisfactory adherence to druglikeness standards is due to their physicochemical qualities falling within an acceptable range (Table 1). This association is significant because it improves the suitability of hits for potential drug development.
To assure the docking protocol, two complexes were prepared, one as a reference complex downloaded from pdb (ID; 6JOL), and one was our docked complex with identical co-crystallized ligand used as standard in this study. These both complexes were aligned in PyMOL. Firstly, there was a pair-wise alignment of 279 atoms from both of the complexes, but many atoms were rejected. There were 3 refinement cycles and 3 atoms rejected in the first cycle. One atom for each second and third cycle was rejected, which reduced the number of atoms of alignment to 274 for each complex. Then atoms were processed to final alignment (Figure 3A) with an executed RMSD value of 0.75Å. Our RMSD score is in the ideal range, closer to zero, indicating that our docked complex is almost perfectly aligned with the PDB reference complex. It verifies the validity of our docking approach because the docking of our other compounds in the library performed on the same active site as the docking of the standard because they co-crystallized in the same place, as indicated by the PDB ID. Our docking results show that the standard compound attaches to a comparable pocket, which supports the validity of our docking method (Figure 3A).
Figure 3. (A) Superimposition of standard docked complex and PDB reference complex (ID; 6JOL) (B) 2D (C) 3D interaction of imatinib with PDGFRA.
Protein-ligand interaction analysis was performed on the top 7 hits, namely cis-Grossamide K (compound 1), Daturafoliside O (compound 2), N-cis-feruloyltyramine (compound 3), Maceneolignan H (compound 4), Erythro-2-(4-allyl-2,6-dimethoxyphenoxy)-1-(3,4,5-trimethoxyphenyl) propan-1,3-diol (compound 5), Myrifralignan C (compound 6), and stigmasteryl-3-O-β-glucoside (compound 7).
Standard imatinib, a co-crystallized ligand of PDGFRA protein, has a docking score of −7.49 kcal/mol against this receptor. It showed two polar interactions with Leu651 and Leu660 of PDGFRA at the distances of 2.88Å for each having energies −1.9 and −3.0 kcal/mol, respectively. The standard showed one pi-H with Lys812 having binding energy −0.6 kcal/mol at the distance of 4.89 Å (Figures 3B,C). Compound 1, cis-Grossamide K, exhibited the highest S-score of −8.16 against the PDGFRA protein. The target protein showed interactions in MOE with this ligand by pi-H bond with Leu660 having energy −0.8 kcal/mol at the distance of 3.99Å. This compound showed two additional interactions with Arg817 and Cys835 in PyMol (Figure 4). That drug candidate was isolated from D. metel. Cis-grossamide K was reported for effectively inhibiting melanin formation and cell viability in Melan-a cells, with IC50 and LD50 values of 54.24 and 163.60 μM, respectively. The chemical group ascribed to their anti-melanogenic effect is the 3,4-disubstituted phenyl, used as a depigmenting and skin-whitening agent (Leonard et al., 2021).
Figure 4. Molecular interactions of protein PDGFRA (green) with Cis-grossamide inhibitor (purple) (compound 1). (A) 3D image of interacting residues (red) of PDGFRA; (B) 2D interaction profile of Cis-grossamide.
Compound 2, Daturafoliside O, displayed a higher docking score of −7.89 against the target receptor isolated from D. metel L. [Solanaceae]. This ligand showed interactions with the target receptor by forming H-bonds (Supplementary Figure S1). It forms one interaction with His816 at the distance of 3.22 Å, having −0.9 kcal/mol energy. It also showed one H-bond with Asp836 having energy −3.1 kcal/mol at the distance of 2.83 Å. This compound showed additional interaction in Pymol with Lys627 residue. Daturafoliside O is reported for its inhibitory activity in nitric oxide production and also a good source of bioactive substances (Guo et al., 2018).
Compound 3, N-cis-feruloyltyramine, has a docking score of −7.83 kcal/mol. This ligand was isolated from D. metel L. [Solanaceae] and showed interaction with the target protein by two polar bonds (Figure 5). It formed one H-bond with Glu644 at the distance of 2.99 Å with energy −1.0 kcal/mol. It also showed one significant interaction with Asp836 having 2.82 Å distance and −2.88 kcal/mol energy. N-cis-feruloyltyramine showed the highest relevance for treatment of rheumatoid arthritis as screened from pathway target compounds (Zhang et al., 2023).
Figure 5. Molecular interactions of protein PDGFRA (green) with N-cis feruloyltyramine (light blue) (compound 3). (A) 3D image of interacting residues (red) of PDGFRA; (B) 2D interaction profile of N-cis feruloyltyramine.
Compound 4, Maceneolignan H, has a docking score of −7.58 kcal/mol against cancerous proteins. This compound was isolated from M. fragrans Houtt. [Myristicacea] and didn’t show any interacting residues with PDGFRA in MOE. It showed one interaction with Arrg817 in pyMOL (Supplementary Figures S2A, B). Maceneolignans are known to inhibit soluble epoxide hydrolase (sEH) (Oanh et al., 2023).
Compound 5, Erythro-2-(4-allyl-2, 6-dimethoxyphenoxy)-1-(3, 4, 5-trimethoxyphenyl) propan-1, 3-diol isolated from M. fragrans Houtt. [Myristicacea] showed docking score of −7.55 kcal/mol against target receptor. This compound showed one polar interaction with Phe856 at the distance of 2.83 Å with energy −2.5 kcal/mol (Supplementary Figures S2C, D). It showed further interaction with Arg817 in PyMOL. It is effective in antibacterial and antimicrobial treatment.
Compound 6, Myrifralignan C, exhibited a docking score of −7.51 against PDGFRA. This compound showed only one interaction in PyMOL with Arg817 (Supplementary Figures S3A, B). It was isolated from M. fragrans Houtt. [Myristicacea]. A study shows that Myrifralignan C can considerably inhibit the expression of nitric oxide synthase mRNA. This compound also possesses inflammatory activities (Cao et al., 2015).
Compound 7, stigmasteryl-3-O-β-glucoside, has a docking score of −7.49 against the PDGFRA protein. This compound showed two polar interactions with Glu644 and Met648 at the distances 3.00 Å and 3.19 Å, having energy −0.8 and −2.3 kcal/mol (Supplementary Figures S3C, D). It showed additional interaction with Asp836 of the target protein in pyMOL. It was isolated from M. fragrans Houtt. [Myristicacea]. Stigmasteryl-3-O-β-glucoside is reported for its antioxidant and anti-inflammatory activity (Zhanga et al., 2015).
Owing to the chemical diversity, confirmed affinity in the active site of PDGFRA, and the promising results in our drug-likeness analysis of our top 7 hits, this provides compelling evidence for proceeding with these compounds into experimental analysis to investigate their therapeutic potential further.
The objective was to selectively identify the different pharmacophoric characteristics of our hits by using a phenolic scaffold. This approach is intended to maximize their affinity and efficacy in inhibiting the PDGFRA receptor, hence improving their potential as pharmaceutical agents. To construct a pharmacophore, we introduced all the pharmacophoric features of phenolic scaffold in cis-Grossamide K and Daturafoliside O. The feature identified in cis-Grossamide K (Figure 6) is G7 labeled “Aro,” along with “hybrid,” with a radius of 0.50Å, suggesting a region in the compound that can participate in both π−π stacking and hydrophobic interactions. G17 labeled as “Don&Acc” indicates that this region is important for forming specific hydrogen bond acceptors and donors in the active site of PDGFRA. G19 in this compound is labeled as “Acc,” describing its specificity in forming hydrogen bond acceptors. These interactions can define the binding affinity, specificity, and overall biological activity of cis-Grossamide K. The features identified in Daturafoliside O (Figure 6) are G24 and G25 bonds, both labeled with “Don&Acc,” with a radius of 0.50Å for forming H-bond acceptors and donors with active site residues of PDGFRA.
Figure 6. (A) cis-Grossamide K; (B) Daturafoliside O, representing molecular structure with annotated pharmacophoric features, contribute in binding to PDGFRA protein.
The interaction profile of cis-Grossamide K reveals different bonding types, while Daturafoliside O solely shows hydrogen bonding. Despite the presence of the same functional components, such peculiar bonding can be important to these compounds’ therapeutic efficacy. This also explains the disparity in their docking scores, as cis-Grossamide K (−8.16 kcal/mol) has greater docking scores for target proteins than Daturafoliside O (−7.89 kcal/mol) (Supplementary Table S1). The specified pharmacophoric aspects are critical in the drug development process for PDGFRA inhibitors because they represent the important chemical capabilities required for compounds to interact with key residues of PDGFRA. Further investigation into these properties might help develop more potent and selective drugs by ensuring that future pharmaceuticals contain the structural components required for efficient binding.
The co-crystallized structure (6JOL-imatinib) demonstrated stability throughout the entire simulation, with the variation in the pose of imatinib ranging from 0.07 nm to 0.55 nm, and an average of 0.41 nm. This was followed by the stability of the system (6JOL-Daturafoliside O), with a variation of 1.04 nm to 0.06 nm, and an average of 0.63 nm. The complex (6JOL-N-cis-feruloyltyramine) showed the best performance in the variation of the ligand pose in the catalytic pocket of the target, with a range of 0.36 nm to 0.04 nm, and an average of 0.20 nm. However, the same performance was not observed in the system (6JOL-cis-Grossamide K), which produced a variation of 1.40 nm to 0.04 nm and an average of 0.91 nm (Figure 7A).
Figure 7. Molecular dynamics of protein 6JOL with its respective inhibitors. (A) Overlay of RMSD of four inhibitors; (B) Fluctuation analysis of the four systems overlaid in RMSF; (C) Analysis of radius of gyration over 100 ns.
The RMSF graphs reveal that all systems were stable without significant fluctuation, with variation below 0.3 nm as described in the literature (Figure 7B) (Lindahl et al., 2001). The principle of Rg is defined as the ratio between the accessible surface area of a protein and that of a sphere of the same volume. This principle is fundamental for understanding the protein folding mechanism (Galzitskaya et al., 2008). As shown in Figure 7C, all systems were highly stable and compact without evidence of residue disorder.
The stability of the four systems under analysis becomes evident when observing their geometric conformations. In Figure 8A–C, we observe low perturbation of the ligands imatinib, daturafoliside O, and n-cis-feruloyltyramine in the binding site of 6JOL. This analysis supports the hypothesis of high complementarity of the studied complexes, as they occupy the same catalytic pocket as the co-crystallized structure. However, in Figures 8D, despite the protein being compact and showing low variation in protein residues, the ligand cis-Grossamide K forces its way out of the catalytic pocket, causing fluctuation in the RMSD. The crystallographic structure of PDGFRA with imatinib (PDB ID 6JOL) shows a gap of 80 residues between domains 2 and 3 of the protein, this means there is a part of the protein where the resolution was not sufficient to capture these specific residues. These missing residues may be located in regions of high flexibility or areas that are difficult to resolve.
Figure 8. 3D visualization of the molecular poses of the four inhibitors in the 6JOL target at intervals of 00 ns, 20 ns, 40 ns, 60 ns, 80 ns, and 100 ns. (A) Snapshots of 6JOL/imatinib; (B) Snapshots of 6JOL/daturafoliside O; (C) Snapshots of 6JOL/n-cis-feruloyltyramine; (D) Snapshots of 6JOL/cis-Grossamide K.
The quantification of the number of hydrogen bonds revealed the existence of hydrogen interactions between the protein and the ligand throughout the entire simulation for all four evaluated systems (Figure 9). The highest participation in these interactions, with an average of 2.0, was evident for 6JOL/imatinib and 6JOL/n-cis-feruloyltyramine. The presence of these interactions suggests high complementarity of the ligand to the target, with minimal perturbation in the protein’s binding pocket (Rehman et al., 2023).
Figure 9. Analysis of the number of hydrogen bonds: (A) 6JOL/imatinib; (B) 6JOL/daturafoliside O; (C) 6JOL/n-cis-ferulovltvramine; (D) 6JOL/cis-Grossamide K.
The protein-ligand interaction energies were confirmed by calculating the Coulomb (Coul-SR) and Lennard-Jones (LJ-SR) terms. Protein-ligand interaction energies calculated by Coulomb (Coul-SR) and Lennard-Jones (LJ-SR) terms help identify strong inhibitors. Coul-SR reflects electrostatic interactions, where more negative values indicate stronger ionic or dipole binding. LJ-SR accounts for van der Waals forces, with lower values suggesting better fit and fewer steric clashes. Strong candidates typically show highly negative total interaction energy, indicating stable and specific binding to the target site. This balance of electrostatic and van der Waals contributions highlights ligands that effectively inhibit protein activity. During the simulation, the total energy values obtained were as follows: 6JOL/imatinib produced a value of −323,28 kJ/mol, 6JOL/daturafoliside O−154,99 kJ/mol, 6JOL/n-cis-ferulovltvramine −249,39 kJ/mol, and 6JOL/cis-Grossamide K −202,38 kJ/mol. These findings suggest that the compounds daturafoliside O and n-cis-ferulovltvramine are strong candidates for inhibiting PDGFRA (Figures 10A–D).
Figure 10. Calculation of Coul-SR and LJ-SR interaction energies of Protein-LIG. (A) 6JOL/imatinib; (B) 6JOL/daturafoliside O; (C) 6JOL/n-cis-ferulovltvramine; (D) 6JOL/cis-Grossamide K.
Principal Component Analysis (PCA) was performed for the four systems (PDGFRA - imatinib; PDGFRA - daturafoliside O; PDGFRA - N-cis-feruloyltyramine; PDGFRA - cis-Grossamide K) from the trajectories produced in GROMACS. The two-dimensional projections of conformational changes during the simulation were plotted in a 2D graph based on the first five eigenvectors, highlighting differences in their trajectories and conformational changes over 100 ns. In the PDGFRA - imatinib system, two regions were occupied in the PCA plot, suggesting a conformational change with overlap and sharing with the systems PDGFRA - daturafoliside O, PDGFRA - N-cis-feruloyltyramine, and PDGFRA - cis-Grossamide K (Figures 11A–D). In the 2D plot of PDGFRA - N-cis-feruloyltyramine, a more restricted conformational space was observed, suggesting a stabilizing effect of the compound with reduced structural fluctuations. However, in the PCA analysis of the PDGFRA - cis-Grossamide K system, regions outside the plane observed in PDGFRA - imatinib were noted, with a broader range of conformational states, reflecting structural fluctuations. In conclusion, the PCA analyses indicate that the inhibitors prevent the movement of the protein and conformational changes, reducing its conformational variability and internal motions, and have a different coupling mechanism compared to PDGFRA - imatinib.
Figure 11. Principal Component Analysis (PCA) of PDGFRA in four systems: (A) PDGFRA - imatinib; (B) PDGFRA - daturafoliside O; (C) PDGFRA - N-cis-feruloyltyramine; (D) PDGFRA - cis-Grossamide K.
The free energy landscape reveals differences in the conformations adopted by the analyzed systems. In the co-crystalized structure, two regions with two energy minima are observed after binding with imatinib, resulting in a free energy of 12.5 kJ/mol. In the PDGFRA - daturafoliside system, two regions of lower energy are also identified, with a total free energy of 12.5 kJ/mol, located near the regions of the co-crystalized system in terms of position. In the free energy landscape of PDGFRA - N-cis-feruloyltyramine, a single region with thermodynamic stability and a free energy of 13.5 kJ/mol appears. Finally, in the PDGFRA - cis-Grossamide K complex, three energy minima are formed with distinct positions compared to the co-crystalized complex, presenting an interaction energy of 12.7 kJ/mol (Figures 12A–D). Based on these results, it can be concluded that the binding of the inhibitors to the protein blocks its activity by stabilizing specific conformations and altering the formation of energy minima.
Figure 12. The Gibbs free energy landscape, displayed through PC1 and PC2, shows lower energy systems as the areas in deeper blue on the contour map. (A) PDGFRA - imatinib; (B) PDGFRA - daturafoliside O; (C) PDGFRA - N-cis-feruloyltyramine; (D) PDGFRA - cis-Grossamide K.
Next, the analysis of the solvent-accessible surface area (SASA) was performed to understand the dynamics of the hydrophilic and hydrophobic regions and the stability of the folding of the PDGFRA protein. The consistent average SASA values, observed in Figure 13 for the complexes of the compounds imatinib (149.61 nm2), daturafoliside O (148.76 nm2), N-cis-feruloyltyramine (146.58 nm2), and cis-Grossamide K (148.24 nm2), indicated more compact structures with reduced solvent exposure, suggestive of a stabilized protein conformation over 100 ns.
Figure 13. Solvent-accessible surface area (SASA) of the respective complexes: In red, PDGFRA-imatinib; in cyan, PDGFRA-daturafoliside O; in blue, PDGFRA-N-cis-feruloyltyramine; in violet, PDGFRA-cis-Grossamide K.
Understanding ADMET properties is critical for ensuring the overall safety and efficacy of drug candidates, as more than half of drug molecules are unable to be tested in clinical trials due to insufficient ADMET characteristics. 7 most active ligands that follow the standard druglikeness rules (Table 1) were chosen for ADMET analysis. ADMET characteristics of medicinal compounds were predicted using the ADMETlab 3.0 server. The studied parameters include P-gp inhibitor, Caco2 cell line permeability, human intestinal absorption (HIA), volume of distributions (VDss), BBB permeation metabolic enzyme inhibitors, total clearance, AMES toxicity, and carcinogenicity (Table 2).
PDGFRA inhibition can also decrease neurological defects, edema (brain swelling), and Evan blue extravasation within 1–3 days after intracerebral hemorrhage (ICB) (Ma et al., 2011). PDFRA inhibitors should cross the blood-brain barrier to reduce brain impairment. All the hit compounds predicted permeation for the blood-brain barrier (BBB). All seven compounds showed BBB + permeation value in the range of 0–1. P-glycoprotein (P-gp) is abundantly present in cancerous cells and removes chemotherapy drugs from inside the cells. An anti-cancer drug should block P-gp to prevent it from expelling chemotherapy drugs out of the cells. The value of P-gp inhibitors should be in between 0 and 1, and all hit compounds meet this criterion (Table 2). The capacity of oral medicine to pass through the Caco-2 cell line, which originates from human colorectal adenocarcinoma epithelial cells, is used to assess absorption parameters. A high Caco-2 permeability score (−5.15 log cm/s) suggests the drug is absorbed efficiently (Awortwe et al., 2014). Compound 1 cis-Grossamide K showed a CaCO2 permeability value of −5.23, indicating higher absorption in the body. All top hits showed CaCO2 values greater than −4.5 log cm/s.
The drugs show good intestinal absorption in humans if their value is from 0 to 0.3. Notably, our hit compounds fall in this range (Table 2). VDss reveals how the drug is dispersed throughout the body, with more remaining in the plasma. A low VDss suggests strong water solubility or high plasma protein binding. In contrast, a high VDss shows a high concentration in tissues, possibly due to high lipid solubility or tissue binding (Obach et al., 2008). Log L/kg < -0.15 implies low VDss, whereas log L/kg > 0.45 suggests excellent VDss. Compounds 1, 2, 3, 5, and 6 showed optimal VDss values, while compound 4 and 7 showed the highest VDss values greater than 1 (Table 2).
CYP1A2 is mostly present in the liver and plays a role in the metabolism of a variety of drugs, the activation of heterocyclic and aryl amines, which can cause cancer. The most common CYP enzyme present in the small intestine and liver, CYP3A4, is particularly intriguing because it has been proven to catalyze the metabolism of over half of all therapeutic medicines (Yim et al., 2020). Compounds showed values above 0.8 are good inhibitors of these metabolic enzymes (Table 2). Clearance is calculated by dividing the amount of drug in the plasma (measured in mg/min) by its concentration (measured in mg/mL). The total clearance capacity of the body is determined by the sum of drug clearance from the plasma by the liver, kidneys, and other organs. The top 3 compounds, cis-Grossamide K, Daturafoliside O, and N-cis-feruloyltyramine, have the highest clearance value from the body, while all compounds showed a good clearance value greater than 1 mL/min/kg. The AMES test is used to predict the toxicity of chemical compounds. Compounds with an AMES value greater than 0.1 are likely to be non-toxic. Compounds 1, 3, and 4 are found to be non-toxic, among others. All hits showed negative results for the carcinogenic test (Table 2). This analysis helped identify the safe, efficient, and non-toxic compounds to be used as PDGFRA inhibitors.
One of the significant characteristics of a chemical compound is its biological activity, which is caused by their interaction in a biological system. The PASS training set includes a list of the activities and properties that each organic chemical may exhibit under optimum conditions. The depicted activity spectra are represented in PASS as an ordered list of activities with the probabilities “to be active” (Pa) and “to be inactive” (Pi). We filtered the top 3 hits cis-Grossamide K, Daturafoliside O, and N-cis-feruloyltyramine for PASS prediction (Supplementary Figure S4). A high Pa value for any biological activity suggests that the compound is very probable to have that particular trait.
Compound 1, cis-Grossamide K, showed free radical scavenger and antioxidant properties with a p-value of 0.827 and 0.741, respectively, to protect the human body from the harmful effects of free radicals. This compound also possesses HIF1-alpha inhibition activity and appears to block hypoxia-inducible factor 1-alpha (HIF1A) expression, which may result in lowered expression of HIF1A downstream target genes important for tumor growth and survival, as well as a reduction in tumor cell proliferation [46]. Compound 2, Daturafoliside O, showed antineoplastic property to be used as an anti-cancer drug with a probability of 0.853. This compound also showed hepatoprotectant, antieczematic, and hepatic disorder treatment activity, among others (Supplementary Figure S4). Compound 3, N-cis-feruloyltyramine, showed preneoplastic activity to inhibit the formation of malignant tumors (Chodak et al., 1980) with a pa value of 0.812. This compound showed JAK2 expression inhibition activity to treat inflammatory conditions such as ulcerative colitis and rheumatoid arthritis (Miller et al., 2014). It also showed MMP9 expression inhibition activity to suppress the invasion, proliferation, and tumor cell growth to help in the therapy of thyroid cancer (Li et al., 2023). These predictions might help to confirm the promising activities of our hits within the human body.
Following the ADMET study of the top 7 hits, we investigated their many medicinal attributes in biological experiments, including reactivity, potent response, and biosynthetic availability using SwissADME (Supplementary Table S3). PAINS (pan-assay interference compounds) are promiscuous or hitter substances that contain substructures demonstrating strong activity in biological assays regardless of the main target (Prabha and Ezhilarasi, 2021). Our all hits showed activity only against the primary target PDGFRA, as they showed no alerts against the PAIN assay. This indicates that they are likely to exhibit specific biological activity, which is crucial for targeting specific therapeutic pathways. Brenk detects molecules with acceptable toxicity, metabolic stability, and appropriate chemical reactivity (Prabha and Ezhilarasi, 2021). All top compounds showed one alert for the Brenk assay, while compound 6 didn’t show any alert, suggesting they possess suitable chemical reactivity, metabolic stability, and acceptable toxicity levels, essential for safe and effective drug design. Compound 3 completely followed the lead-likeness rule, while the others showed two or three violations. Synthetic accessibility refers to how easy it is to synthesize compounds. However, all of the leading drug candidates have a high synthetic accessibility score (SAS) (Supplementary Table S3), suggesting these compounds can be synthesized relatively easily, facilitating their production and further development. Overall, these results underscore the strong potential of the identified compounds in medicinal chemistry, highlighting their suitability for further exploration as therapeutic agents with favorable medicinal properties.
This research is invaluable for identifying worthwhile hits and is a significant advancement in the development of PDGFRA inhibitor drug design. Through a series of advanced in silico techniques, our study suggests that the seven leading natural products, including cis-Grossamide K, Daturafoliside O, N-cis-feruloyltyramine, Maceneolignan H, Erythro-2-(4-allyl-2, 6-dimethoxyphenoxy)-1-(3, 4, 5-trimethoxyphenyl) propan-1, 3-diol, Myrifralignan C, and stigmasteryl-3-O-β-glucoside, might serve as potential inhibitors for cancer. These compounds showed docking scores higher than standard imatinib, Crenolanib, Lenavatinib, and Sofarenib. The detailed ligand-protein interaction analysis of top hits demonstrated their strong affinity in the binding pocket of PDGFRA protein, including frequently binding residues, i.e., Leu660, Arg817, and Asp836. The MD simulations confirmed the stability of the top 3 hits, namely cis-Grossamide K, Daturafoliside O, and N-cis-feruloyltyramine. The druglikeness and pharmacokinetic analysis of our compounds evaluate their ability to be safe and efficient drugs. Additionally, pharmacokinetic analysis, PASS prediction, and medicinal characteristics calculation contributed to their detailed biological activity. Notably, among our top hits, N-cis-feruloyltyramine has the potential to become a lead compound according to the results of the lead-likeness analysis. The efficiency and least toxic effects of our hits demand the experimental studies to prevent tumor malignancies.
The original contributions presented in the study are included in the article/Supplementary Material, further inquiries can be directed to the corresponding authors.
HK: Formal Analysis, Investigation, Methodology, Writing–original draft. FS: Investigation, Writing–original draft. IA: Investigation, Methodology, Writing–original draft. VJ: Formal Analysis, Methodology, Software, Writing–original draft. UN: Investigation, Methodology, Writing–review and editing. RU: Investigation, Methodology, Funding acquisition, Writing–review and editing. HD: Data curation, Investigation, Methodology, Software, Writing–review and editing. KO: Conceptualization, Formal Analysis, Software, Writing–review and editing. MS: Conceptualization, Project administration, Resources, Supervision, Visualization, Writing–review and editing.
The author(s) declare that financial support was received for the research, authorship, and/or publication of this article. Authors wish to thank Researchers Supporting Project Number (RSP 2025R110) at King Saud University Riyadh Saudi Arabia for financial support.
We are thankful to the Bahauddin Zakariya University, Multan, Pakistan for providing the necessary infrastructure to perform this research.
The authors declare that the research was conducted in the absence of any commercial or financial relationships that could be construed as a potential conflict of interest.
The author(s) declare that no Generative AI was used in the creation of this manuscript.
All claims expressed in this article are solely those of the authors and do not necessarily represent those of their affiliated organizations, or those of the publisher, the editors and the reviewers. Any product that may be evaluated in this article, or claim that may be made by its manufacturer, is not guaranteed or endorsed by the publisher.
The Supplementary Material for this article can be found online at: https://www.frontiersin.org/articles/10.3389/fphar.2024.1512864/full#supplementary-material
Abraham, M. J., Murtola, T., Schulz, R., Páll, S., Smith, J. C., Hess, B., et al. (2015). Gromacs: high performance molecular simulations through multi-level parallelism from laptops to supercomputers. SoftwareX 1-2, 19–25. doi:10.1016/j.softx.2015.06.001
Ahmad, I., Khalid, H., Perveen, A., Shehroz, M., Nishan, U., Rahman, F. U., et al. (2024). Identification of novel quinolone and quinazoline alkaloids as phosphodiesterase 10A inhibitors for Parkinson’s disease through a computational approach. ACS Omega 9, 16262–16278. doi:10.1021/acsomega.3c10351
Alvarez, R. H., Kantarjian, H. M., and Cortes, J. E. (2006). Biology of platelet-derived growth factor and its involvement in disease. Mayo Clin. Proc. 81, 1241–1257. doi:10.4065/81.9.1241
Awortwe, C., Fasinu, P. S., and Rosenkranz, B. (2014). Application of Caco-2 cell line in herb-drug interaction studies: current approaches and challenges. J. Pharm. Pharm. Sci. 17, 1–19. doi:10.18433/j30k63
Bray, F., Laversanne, M., Sung, H., Ferlay, J., Siegel, R. L., Soerjomataram, I., et al. (2024). Global cancer statistics 2022: GLOBOCAN estimates of incidence and mortality worldwide for 36 cancers in 185 countries. CA Cancer J. Clin. 74, 229–263. doi:10.3322/caac.21834
Cao, G.-Y., Xu, W., Yang, X.-W., Gonzalez, F. J., and Li, F. (2015). New neolignans from the seeds of Myristica fragrans that inhibit nitric oxide production. Food Chem. 173, 231–237. doi:10.1016/j.foodchem.2014.09.170
Chodak, G. W., Haudenschild, C., Gittes, R. F., and Folkman, J. (1980). Angiogenic activity as a marker of neoplastic and preneoplastic lesions of the human bladder. Ann. Surg. 192, 762–771. doi:10.1097/00000658-198012000-00012
Choudhari, A. S., Mandave, P. C., Deshpande, M., Ranjekar, P., and Prakash, O. (2020). Phytochemicals in cancer treatment: from preclinical studies to clinical practice. Front. Pharmacol. 10, 1614–1617. doi:10.3389/fphar.2019.01614
de Paula Junior, V. F., van Tilburg, M. F., Morais, P. A., Júnior, F. F. M., Lima, E. G., Oliveira, V. T. dos S., et al. (2022). Quantum Biochemistry and MM-PBSA description of the ZIKV NS2B-NS3 protease: insights into the binding interactions beyond the catalytic triad pocket. Int. J. Mol. Sci. 23, 10088. doi:10.3390/ijms231710088
Dib, H., Abu-Samha, M., Younes, K., and Abdelfattah, M. A. O. (2024). Evaluating the physicochemical properties–activity relationship and discovering new 1,2-dihydropyridine derivatives as promising inhibitors for PIM1-kinase: evidence from principal component analysis, molecular docking, and molecular dynamics studies. Pharmaceuticals 17, 880. doi:10.3390/ph17070880
Egan, W. J., Merz, K. M., and Baldwin, J. J. (2000). Prediction of drug absorption using multivariate statistics. J. Med. Chem. 43, 3867–3877. doi:10.1021/jm000292e
Galzitskaya, O. V., Bogatyreva, N. S., and Ivankov, D. N. (2008). Compactness determines protein folding type. J. Bioinform Comput. Biol. 06, 667–680. doi:10.1142/S0219720008003618
Gomes, D., Silvestre, S., Duarte, A. P., Venuti, A., Soares, C. P., Passarinha, L., et al. (2021). In silico approaches: a way to unveil novel therapeutic drugs for cervical cancer management. Pharmaceuticals 14, 741. doi:10.3390/ph14080741
Guex, N., and Peitsch, M. C. (1997). SWISS-MODEL and the Swiss-Pdb Viewer: an environment for comparative protein modeling. Electrophoresis 18, 2714–2723. doi:10.1002/elps.1150181505
Guo, R., Liu, Y., Xu, Z. P., Xia, Y. G., Yang, B. Y., and Kuang, H. X. (2018). Withanolides from the leaves of Datura metel L. Phytochemistry 155, 136–146. doi:10.1016/j.phytochem.2018.08.005
Hamidi, S., Boucher, A., Lemieux, B., Rondeau, G., Lebœuf, R., Ste-Marie, L. G., et al. (2022). Lenvatinib therapy for advanced thyroid cancer: real-life data on safety, efficacy, and some rare side effects. J. Endocr. Soc. 6, bvac048–7. doi:10.1210/jendso/bvac048
Hansen, C. R., Grimm, D., Bauer, J., Wehland, M., and Magnusson, N. E. (2017). Effects and side effects of using sorafenib and sunitinib in the treatment of metastatic renal cell carcinoma. Int. J. Mol. Sci. 18, 461. doi:10.3390/ijms18020461
Heinrich, M. C., Griffith, D., McKinley, A., Patterson, J., Presnell, A., Ramachandran, A., et al. (2012). Crenolanib inhibits the drug-resistant PDGFRA D842V mutation associated with imatinib-resistant gastrointestinal stromal tumors. Clin. Cancer Res. 18, 4375–4384. doi:10.1158/1078-0432.CCR-12-0625
Islam, T., Ara, I., Islam, T., Sah, P. K., Almeida, R. S. de, Matias, E. F. F., et al. (2023). Ethnobotanical uses and phytochemical, biological, and toxicological profiles of Datura metel L.: a review. Curr. Res. Toxicol. 4, 100106. doi:10.1016/j.crtox.2023.100106
Joensuu, H., Wardelmann, E., Eriksson, M., Reichardt, A., Hall, K. S., Schütte, J., et al. (2023). KIT and PDGFRA mutations and survival of gastrointestinal stromal tumor patients treated with adjuvant imatinib in a randomized trial. Clin. Cancer Res. 29, 3313–3319. doi:10.1158/1078-0432.CCR-22-3980
Jorgensen, W. L., Chandrasekhar, J., Madura, J. D., Impey, R. W., and Klein, M. L. (1983). Comparison of simple potential functions for simulating liquid water. J. Chem. Phys. 79, 926–935. doi:10.1063/1.445869
Jurrus, E., Engel, D., Star, K., Monson, K., Brandi, J., Felberg, L. E., et al. (2018). Improvements to the APBS biomolecular solvation software suite. Protein Sci. 27, 112–128. doi:10.1002/pro.3280
Keretsu, S., Ghosh, S., and Cho, S. J. (2020). Molecular modeling study of c-kit/pdgfrα dual inhibitors for the treatment of gastrointestinal stromal tumors. Int. J. Mol. Sci. 21, 8232–8325. doi:10.3390/ijms21218232
Khan, M. F., Ali, A., Rehman, H. M., Noor Khan, S., Hammad, H. M., Waseem, M., et al. (2024). Exploring optimal drug targets through subtractive proteomics analysis and pangenomic insights for tailored drug design in tuberculosis. Sci. Rep. 14, 10904. doi:10.1038/s41598-024-61752-6
Leonard, W., Zhang, P., Ying, D., and Fang, Z. (2021). Lignanamides: sources, biosynthesis and potential health benefits–a minireview. Crit. Rev. Food Sci. Nutr. 61, 1404–1414. doi:10.1080/10408398.2020.1759025
Li, Z., Wei, J., Chen, B., Wang, Y., Yang, S., Wu, K., et al. (2023). The role of MMP-9 and MMP-9 inhibition in different types of thyroid carcinoma. Molecules 28, 3705. doi:10.3390/molecules28093705
Lierman, E., Folens, C., Stover, E. H., Mentens, N., Van Miegroet, H., Scheers, W., et al. (2006). Sorafenib is a potent inhibitor of FIP1L1-PDGFRalpha and the imatinib-resistant FIP1L1-PDGFRalpha T674I mutant. Blood 108, 1374–1376. doi:10.1182/blood-2006-02-004457
Lindahl, E., Hess, B., and van der Spoel, D. (2001). GROMACS 3.0: a package for molecular simulation and trajectory analysis. J. Mol. Model 7, 306–317. doi:10.1007/s008940100045
Lipinski, C. A., Lombardo, F., Dominy, B. W., and Feeney, P. J. (1997). Experimental and computational approaches to estimate solubility and permeability in drug discovery and development settings. Adv. Drug Deliv. Rev. 23, 3–26. doi:10.1016/s0169-409x(00)00129-0
Ma, Q., Huang, B., Khatibi, N., Rolland, W., Suzuki, H., Zhang, J. H., et al. (2011). PDGFR-α inhibition preserves blood-brain barrier after intracerebral hemorrhage. Ann. Neurol. 70, 920–931. doi:10.1002/ana.22549
Madhavan, A., Sankar, P., Ravindranath, K. J., Soundhararajan, R., and Srinivasan, H. (2021). Screening the efficacy of compounds from ghee to control cancer: an in silico approach. Biointerface Res. Appl. Chem. 11, 14115–14126. doi:10.33263/BRIAC116.1411514126
Miller, C. P., Thorpe, J. D., Kortum, A. N., Coy, C. M., Cheng, W. Y., Yang, T. H. O., et al. (2014). JAK2 expression is associated with tumor-infiltrating lymphocytes and improved breast cancer outcomes: implications for evaluating JAK2 inhibitors. Cancer Immunol. Res. 2, 301–306. doi:10.1158/2326-6066.CIR-13-0189
Mozzicafreddo, M., Benfaremo, D., Paolini, C., Agarbati, S., Svegliati Baroni, S., and Moroncini, G. (2023). Screening and analysis of possible drugs binding to PDGFRα: a molecular modeling study. Int. J. Mol. Sci. 24, 9623. doi:10.3390/ijms24119623
Muegge, I., Heald, S. L., and Brittelli, D. (2001). Simple selection criteria for drug-like chemical matter. J. Med. Chem. 44, 1841–1846. doi:10.1021/jm015507e
Muhammad, I., Rahman, N., Gul-E-nayab, , Nishan, U., and Shah, M. (2021). Antidiabetic activities of alkaloids isolated from medicinal plants. Braz. J. Pharm. Sci. 57, 1–14. doi:10.1590/s2175-97902020000419130
Oanh, V. T., Phong, N. V., Min, B. S., Yang, S. Y., and Kim, J. A. (2023). Insights into the inhibitory activities of neolignans and diarylnonanoid derivatives from nutmeg (Myristica fragrans Houtt.) seeds on soluble epoxide hydrolase using in vitro and in silico approaches. J. Enzyme Inhib. Med. Chem. 38, 2251099. doi:10.1080/14756366.2023.2251099
Oashi, T., Ringer, A. L., Raman, E. P., and MacKerell, A. D. (2011). Automated selection of compounds with physicochemical properties to maximize bioavailability and druglikeness. J. Chem. Inf. Model 51, 148–158. doi:10.1021/ci100359a
Obach, R. S., Lombardo, F., and Waters, N. J. (2008). Trend analysis of a database of intravenous pharmacokinetic parameters in humans for 670 drug compounds. Drug Metabolism Dispos. 36, 1385–1405. doi:10.1124/dmd.108.020479
Ong, H. S., Gokavarapu, S., Tian, Z., Li, J., Xu, Q., Zhang, C. P., et al. (2018). PDGFRA mRNA overexpression is associated with regional metastasis and reduced survival in oral squamous cell carcinoma. J. Oral Pathology and Med. 47, 652–659. doi:10.1111/jop.12713
Poroikov, V. V., Filimonov, D. A., Borodina, Y. V., Lagunin, A. A., and Kos, A. (2000). Robustness of biological activity spectra predicting by computer program PASS for noncongeneric sets of chemical compounds. J. Chem. Inf. Comput. Sci. 40, 1349–1355. doi:10.1021/ci000383k
Prabha, B., and Ezhilarasi, M. R. (2021). Synthesis, spectral characterization, in vitro and in silico studies of Benzodioxin pyrazoline derivatives. Biointerface Res. Appl. Chem. 11, 9126–9138. doi:10.33263/BRIAC112.91269138
Prakash, E., and Gupta, D. (2013). Cytotoxic activity of ethanolic extract of myristica fragrans (Houtt) against seven human cancer cell lines. Univers. J. Food Nutr. Sci. 1, 1–3. doi:10.13189/ujfns.2013.010101
Rasgania, J., Gavadia, R., and Jakhar, K. (2023). Facile synthesis, pharmacological and in silico analysis of succinimide derivatives: an approach towards drug discovery. J. Mol. Struct. 1274, 134424. doi:10.1016/j.molstruc.2022.134424
Rehman, H. M., Sajjad, M., Ali, M. A., Gul, R., Irfan, M., Naveed, M., et al. (2023). Identification of NS2B-NS3 protease inhibitors for therapeutic application in zikv infection: a pharmacophore-based high-throughput virtual screening and MD simulations approaches. Vaccines (Basel) 11, 131. doi:10.3390/vaccines11010131
Salem, M. S. H., Abdel Aziz, Y. M., Elgawish, M. S., Said, M. M., and Abouzid, K. A. M. (2020). Design, synthesis, biological evaluation and molecular modeling study of new thieno[2,3-d]pyrimidines with anti-proliferative activity on pancreatic cancer cell lines. Bioorg Chem. 94, 103472. doi:10.1016/j.bioorg.2019.103472
Shah, M., Bashir, S., Jaan, S., Nawaz, H., Nishan, U., Abbasi, S. W., et al. (2021). Computational analysis of plant-derived terpenes as α -glucosidase inhibitors for the discovery of therapeutic agents against type 2 diabetes mellitus. South Afr. J. Bot. 143, 462–473. doi:10.1016/j.sajb.2021.09.030
Shah, M., Yamin, R., Ahmad, I., Khalid, H., Abbasi, S. W., Nishan, U., et al. (2024a). Identifying plant-derived antiviral alkaloids as dual inhibitors of SARS-CoV-2 main protease and spike glycoprotein through computational screening. Front. Pharmacol. 15, 1369659–1369723. doi:10.3389/fphar.2024.1369659
Shah, M., Yamin, R., Ahmad, I., Wu, G., Jahangir, Z., Shamim, A., et al. (2024b). In-silico evaluation of natural alkaloids against the main protease and spike glycoprotein as potential therapeutic agents for SARS-CoV-2. Plos ONE 19, e0294769. doi:10.1371/journal.pone.0294769
Veber, D. F., Johnson, S. R., Cheng, H.-Y., Smith, B. R., Ward, K. W., and Kopple, K. D. (2002). Molecular properties that influence the oral bioavailability of drug candidates. J. Med. Chem. 45, 2615–2623. doi:10.1021/jm020017n
Verma, D., Pundir, S., Goyal, R., Aljabali, A. A. A., Dua, K., Chellappan, D. K., et al. (2024). Versatile therapeutic potential of Swertia chirayita (roxb.) H. Karst: a review. Chem. Afr. 7, 2941–2961. doi:10.1007/s42250-024-00978-3
Viswanadhan, V. N., Rajesh, H., and Balaji, V. N. (2011). Atom type preferences, structural diversity, and property profiles of known drugs, leads, and nondrugs: a comparative assessment. ACS Comb. Sci. 13, 327–336. doi:10.1021/co2000168
Yim, S. K., Kim, K., Chun, S., Oh, T., Jung, W., Jung, K., et al. (2020). Screening of human CYP1A2 and CYP3A4 inhibitors from seaweed in silico and in vitro. Mar. Drugs 18, 603–615. doi:10.3390/MD18120603
Yu, X., Zhu, X., Zhang, L., Qin, J. J., Feng, C., and Li, Q. (2022). In silico screening and validation of PDGFRA inhibitors enhancing radioiodine sensitivity in thyroid cancer. Front. Pharmacol. 13, 883581–883612. doi:10.3389/fphar.2022.883581
Zhang, Z., Ren, M., Zhu, Y., Huang, Y., and Hu, Y. (2023). Integration of UPLC-Q-TOF-MS/MS, chemometrics and network pharmacology to discovery potential quality markers in Sinomenii Caulis, 1–9.
Zhanga, C. R., Jayashree, E., Kumar, P. S., and Nair, M. G. (2015). Antioxidant and antiinflammatory compounds in nutmeg (myristica fragrans) pericarp as determined by in vitro assays. Nat. Prod. Commun. 10, 1399–1402. doi:10.1177/1934578x1501000822
Keywords: anticancer, natural products, drug design, ethnopharmacology, virtual screening
Citation: Khalid H, Sattar F, Ahmad I, Junior VFdP, Nishan U, Ullah R, Dib H, Omari KW and Shah M (2025) Computer-assisted discovery of natural inhibitors for platelet-derived growth factor alpha as novel therapeutics for thyroid cancer. Front. Pharmacol. 15:1512864. doi: 10.3389/fphar.2024.1512864
Received: 17 October 2024; Accepted: 17 December 2024;
Published: 09 January 2025.
Edited by:
Jong-Min Kim, University of Minnesota Morris, United StatesReviewed by:
Titilayo Omolara Johnson, University of Jos, NigeriaCopyright © 2025 Khalid, Sattar, Ahmad, Junior, Nishan, Ullah, Dib, Omari and Shah. This is an open-access article distributed under the terms of the Creative Commons Attribution License (CC BY). The use, distribution or reproduction in other forums is permitted, provided the original author(s) and the copyright owner(s) are credited and that the original publication in this journal is cited, in accordance with accepted academic practice. No use, distribution or reproduction is permitted which does not comply with these terms.
*Correspondence: Khaled W. Omari, a2hhbGVkLm9tYXJpQGF1bS5lZHUua3c=; Mohibullah Shah, bW9oaWJAYnp1LmVkdS5waw==, bW9oaWJ1c2JAZ21haWwuY29t
†These authors have contributed equally to this work
Disclaimer: All claims expressed in this article are solely those of the authors and do not necessarily represent those of their affiliated organizations, or those of the publisher, the editors and the reviewers. Any product that may be evaluated in this article or claim that may be made by its manufacturer is not guaranteed or endorsed by the publisher.
Research integrity at Frontiers
Learn more about the work of our research integrity team to safeguard the quality of each article we publish.