- Center of Pharmacometrics and Systems Pharmacology, University of Florida, Orlando, FL, United States
Background: In-vivo CRISPR Cas genome editing is a complex therapy involving lipid nanoparticle (LNP), messenger RNA (mRNA), and single guide RNA (sgRNA). This novel modality requires prior modeling to predict dose-exposure-response relationships due to limited information on sgRNA and mRNA biodistribution. This work presents a QSP model to characterize, predict, and translate the Pharmacokinetics/Pharmacodynamics (PK/PD) of CRISPR therapies from preclinical species (mouse, non-human primate (NHP)) to humans using two case studies: transthyretin amyloidosis and LDL-cholesterol reduction.
Methods: PK/PD data were sourced from literature. The QSP model incorporates mechanisms post-IV injection: 1) LNP binding to opsonins in liver vasculature; 2) Phagocytosis into the Mononuclear Phagocytotic System (MPS); 3) LNP internalization via endocytosis and LDL receptor-mediated endocytosis in the liver; 4) Cellular internalization and transgene product release; 5) mRNA and sgRNA disposition via exocytosis and clathrin-mediated endocytosis; 6) Renal elimination of LNP and sgRNA; 7) Exonuclease degradation of sgRNA and mRNA; 8) mRNA translation into Cas9 and RNP complex formation for gene editing. Monte-Carlo simulations were performed for 1000 subjects and showed a reduction in serum TTR.
Results: The rate of internalization in interstitial layer was 0.039 1/h in NHP and 0.007 1/h in humans. The rate of exocytosis was 6.84 1/h in mouse, 2690 1/h in NHP, and 775 1/h in humans. Pharmacodynamics were modeled using an indirect response model, estimating first-order degradation rate (0.493 1/d) and TTR reduction parameters in NHP.
Discussion: The QSP model effectively characterized biodistribution and dose-exposure relationships, aiding the development of these novel therapies. The utility of platform QSP model can be paramount in facilitating the discovery and development of these novel agents.
Introduction
In the past decade, the field of genomic medicine has undergone a transformative change with the advent of clustered regularly interspaced short palindromic repeats (CRISPR) and CRISPR-associated protein (Cas) systems (Jinek et al., 2012; Abdelhady et al., 2023). These systems, originally identified as an adaptive immune mechanism in bacteria, have paved the way for precise, programmable genome editing, revolutionizing the potential for curative therapies in human disease (Jinek et al., 2012). The transition of CRISPR-Cas from a basic research tool to a clinical therapeutic modality has marked a significant milestone in the pursuit of treating genetic disorders at their root cause.
The development of in vivo CRISPR therapies represents a cutting-edge frontier in medicine, offering a direct and efficient method to correct genetic defects directly within the body. Unlike respective ex vivo approaches, which require cell extraction, modification, and reinfusion, in vivo CRISPR therapies involve the systemic delivery of the gene-editing components, targeting the cells in their natural physiological environment. This approach not only broadens the therapeutic potential to a wider range of diseases, including those affecting organs and tissues difficult to access ex vivo, but also promises a more streamlined and potentially less invasive treatment option (Abdelhady et al., 2023; Çerçi et al., 2023).
Both preclinical studies and clinical trials have shown promising results in utilizing CRISPR-Cas systems for in vivo applications (Çerçi et al., 2023). The ability to directly correct or modify genes within the patient’s body circumvents the complexities and limitations associated with cell extraction and reinfusion processes, opening new avenues for treating a plethora of genetic conditions.
Furthermore, the advancements in delivery mechanisms, such as lipid nanoparticles (LNPs), have significantly enhanced the precision in terms of targeting the liver or any other target organ of interest by an antibody-based LNP system, efficiency, and safety of these in vivo gene-editing therapies, making them a viable option for clinical applications (Kumar et al., 2023).
In vivo CRISPR therapies are administered via intravenous infusion. They consist of three components: 1) delivery vehicle, 2) single guide RNA (sgRNA), and 3) messenger RNA (mRNA). For the delivery vehicle, both the LNP and adeno-associated virus (AAV) are used. Changes in the delivery vehicle will impact the pharmacokinetics (PK) of CRISPR therapies (Abdelhady et al., 2023; Kavita et al., 2023; Suzuki and Ishihara, 2021; Akinc et al., 2010). In this paper, we focus on the LNP as the delivery vehicle encapsulating the sgRNA and mRNA product.
Gene editing via sgRNA and mRNA is accomplished by the following sequential steps: 1) post-injection entering into the liver; 2) internalization of the bound LNP via receptor-mediated endocytosis binding to a low-density lipoprotein (LDL) receptor via apolipoprotein E (ApoE) present on the surface of the LNP or unbound LNP via macropinocytosis (Miyazawa et al., 2024; Paunovska et al., 2022; Bisgaier et al., 1989); 3) entering into the cellular layer and releasing the sgRNA and mRNA product via endosomal escape (Hou et al., 2021); 4) mRNA getting translated into Cas protein of interest (Abdelhady et al., 2023; Miyazawa et al., 2024); 5) sgRNA and Cas protein will form a ribonucleoprotein (RNP) complex to perform gene editing by entering the nucleus, where it recognizes the protospacer adjacent motif (PAM) on the complementary DNA strand, the sgRNA binds to the target site, and Cas9 undergoes conformational changes, leading to DNA cleavage (Abdelhady et al., 2023; Li et al., 2019; Rouet and Christ, 2019); 6) the sgRNA and mRNA might also escape from the cell via exocytosis and can get internalized back via clathrin-dependent endocytosis or caveola-mediated endocytosis and macropinocytosis (Wu and Li, 2021; Del Toro Runzer et al., 2023; Desai et al., 2019); and 7) the sgRNA, mRNA, and LNP exhibit lysosomal degradation (Fujiwara et al., 2016).
To our knowledge, there is no prior model published that characterizes and predicts the in vivo PK/pharmacodynamics (PD) of CRISPR-Cas9 therapies across species due to the novelty of these therapeutics, their unique biodistribution, and their complex mechanism-of-action. As a result, there is a need to develop a translational platform quantitative systems pharmacology (QSP) model that can capture these processes across multiple species to inform the development of in vivo CRISPR therapies from preclinical species to the clinic and to predict the first-in-human (FIH) dosing (Shah and Betts, 2012; Betts et al., 2019; Ayyar and Song, 2024; Ayyar et al., 2021; Singh et al., 2021; Mager and Jusko, 2001; Mager and Jusko, 2008). This objective is approached in the current paper in a stepwise fashion, starting with the extraction of available PK-PD studies in mice, non-human primates (NHPs), and humans from the literature. We developed a translational QSP model to characterize the whole body to intracellular PK-PD actions of CRISPR-Cas therapy and physiologically scaled the platform model across species. In order to evaluate the impact of drug-specific parameters on the biodistribution of in vivo CRISPR-Cas therapy across species, a global sensitivity analysis (GSA) was conducted. Monte Carlo simulations were performed to understand and characterize the dose–response relationship for NTLA-2001 in vivo CRISPR-Cas therapy in patients suffering from transthyretin amyloidosis (Kotit, 2023).
Methods
Model development
The data used to build the translational QSP model for in vivo CRISPR-Cas9 gene therapy were obtained from the published literature, as given in Table 1. The PK data for NTLA-2001 in mice were obtained from a published study (Finn et al., 2018). The reported dataset for mice includes mean pharmacokinetic measurements in plasma for mRNA and sgRNA, sampled at 0, 0.2, 0.4, 0.6, 0.8, 1, 10, and 25 h and quantified using the quantitative polymerase chain reaction (qPCR) assay. The mice were dosed with a bolus dose of 2 mg/kg total RNA, in which 33.3% and 66.7% were calculated to be sgRNA and mRNA, and the total LNP dose was calculated to be 36.7 mg/kg based on the published source (Gillmore et al., 2021).
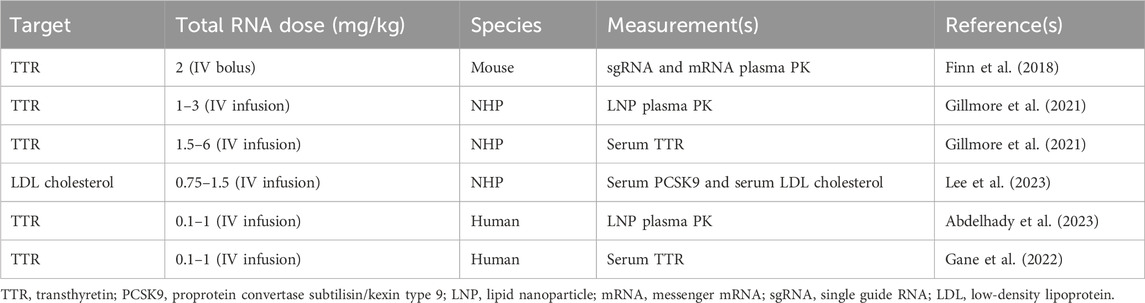
Table 1. Summary of published preclinical and clinical datasets which are used in order to develop the proposed translational QSP model.
The PK for the lipid nanoparticle of NTLA-2001 targeting transthyretin (TTR) amyloidosis in NHPs has been reported following a 2-h infusion of 1, 2, or 3 mg/kg total RNA, in which 33.3% and 66.7% was sgRNA and mRNA, respectively, and the total LNP doses were 18.5, 36.7, and 55.5 mg/kg based on a published report (Gillmore et al., 2021). The plasma PK was sampled at 1.5, 4, and 8 h in cynomolgus monkeys. The biomarkers for this drug include the TTR protein; the concentration of TTR was quantified using enzyme-linked immunosorbent assay (ELISA); the biomarker response for NTLA-2001 was reported for a short-term infusion of 2 h for doses of 1.5, 3, and 6 mg/kg total RNA; and the total LNP doses were 27.75, 68.82, and 137.64 mg/kg (Gillmore et al., 2021). The biomarkers for a second case study for the investigational drug VERVE-101 include PCSK9 measured in blood and LDL cholesterol (Lee et al., 2023). The response was reported for a short-term infusion of 2 h for doses of 0.75 and 1.5 mg/kg of total RNA and 17.2 and 27.75 mg/kg of LNP calculated based on the published literature (Gillmore et al., 2021). The PCSK9 sampled in blood and LDL cholesterol was quantified using a commercial immunoassay kit (Protein Simple Ella Simple Plex Human PCSK9) and Beckman Coulter AU680 analyzer, respectively (Lee et al., 2023).
The mean plasma pharmacokinetics of the LNP for NTLA-2001 was reported in humans following a short-term infusion of 2 h for doses of 0.1, 0.3, 0.7, and 1 mg/kg of total RNA and 1.85, 5.55, 12.9, and 18.5 mg/kg of LNP calculated based on the published literature (Abdelhady et al., 2023), and the pharmacokinetic data for the LNP were sampled from 0 to 50 h (Abdelhady et al., 2023). The TTR biomarker response was digitized from the literature for NTLA-2001, and TTR reduction was sampled at 7, 14, and 28 days (Gane et al., 2022).
Model structure and workflow
A stepwise approach was applied to develop and validate the platform model using data leveraged from in vivo studies in mice and NHPs, and clinical responses in humans (Shah and Betts, 2012; Ayyar and Song, 2024; Ayyar et al., 2021; Li and Shah, 2019). A mechanistic model was developed in order to characterize the unknown drug-specific attributes for the in vivo CRISPR-Cas9 gene therapy (Ayyar and Song, 2024). The schematic diagram given in Supplementary Figure S1 represents the model structure. The model consists of one systemic compartment, which further includes a compartment to represent the mononuclear phagocyte system (MPS) (Kumar et al., 2023; Hoshyar et al., 2016) in which the drug will be internalized following a first-order rate constant of phagocytosis into the MPS (
The translational quantitative systems pharmacology model was developed to characterize the unique biodistribution of the three components associated with the therapy, namely, LNP, mRNA, and sgRNA (Shah and Betts, 2012; Betts et al., 2019; Ayyar et al., 2021). The model structure is shown in Figures 1A–C, and the series of Ordinary Differential Equations (ODEs) are provided in Supplementary Information S1. The model includes a liver compartment divided into vascular, interstitial, and cellular regions, and the kidney compartment is only present in the LNP and sgRNA, but it is absent in mRNA due to its higher molecular weight, which the mRNA will not eliminate via renal clearance (Meibohm and Zhou, 2012; Oladipo et al., 2022; Chen et al., 2022). The gene therapy enters the tissue vascular space via arterial plasma flow and exits via the venous plasma flow. Lymph flow is represented as 0.2% of the plasma flow. Once the LNP enters the vascular layer of the liver, it can get internalized into the MPS represented by
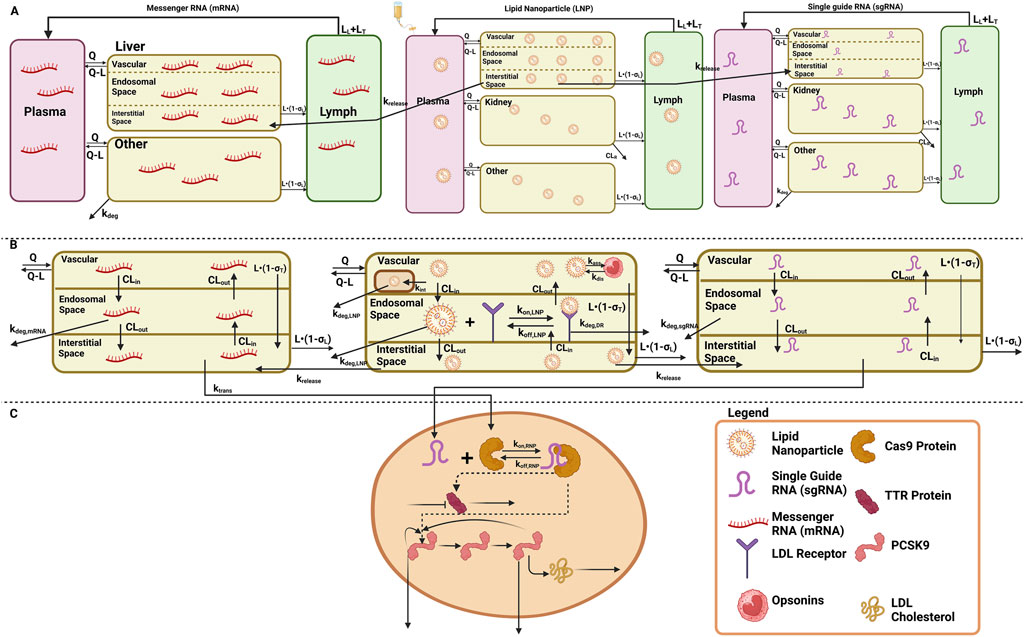
Figure 1. Schematic of the translational QSP model elucidating the disposition of three components, LNP, sgRNA, and mRNA, for in vivo CRISPR-Cas therapy. (A) A system PK model depicting the whole-body disposition for in vivo CRISPR-Cas therapy. The liver compartment within the model is divided into sub-compartments. (B) Organ-level structure of the system PK model for the disposition of all the components of in vivo CRISPR-Cas therapy, that is, LNP, sgRNA, and mRNA. (C) Cell-level system PD for in vivo CRISPR-Cas therapy.
According to the pharmacology and mechanism of action, the RNP complex cleaves the target DNA via indirect mechanisms by changing the DNA, causing a reduction in the protein level (Abdelhady et al., 2023). Therefore, the PD is modeled on a cellular layer via indirect response model-I, dependent on the concentration of the RNP in the liver as the TTR for the first case study (Ayyar et al., 2021; Mager and Jusko, 2008), and for the second case study, a feedback loop model (Friberg et al., 2002; Liu et al., 2022; Xia et al., 2021) was used based on the mechanism of action and pharmacology for PCSK9 as well as LDL cholesterol was measured in the blood samples the correction of biomarkers happened at the site of secretion, reduction in LDL-cholesterol is captured via precursor dependent model (Ayyar et al., 2021).
Model development
Step 1: Development of the mechanistic model across species
A mechanistic model was developed to characterize unknown drug-specific attributes to understand unique PK/PD properties. The developed model, which is shown in Supplementary Figure S1, had a lot of drug-specific attributes, and data from all three species were modeled simultaneously to account for drug-specific attributes, which were then used for building a complex QSP model. Inter-individual variability (IIV) was turned on during this time to account for variability between species. The rate of association
Step 2: Development of the translational QSP model in mice
A translational QSP model comprises a liver, kidney, and remainder (Figures 1A–C) for LNP and sgRNA, while for mRNA, the model comprises a liver and remainder. The dose was administered in the plasma compartment. The rate of endocytosis
Step 3: Development of the translational QSP model in NHPs.
The translational model was scaled to NHPs and was used to characterize the PK/PD properties of CRISPR-Cas9 therapy. The rate of endocytosis of the LNP, sgRNA, and mRNA was estimated
Step 4: Development of the translational QSP model in humans
The translational model was then scaled in humans where the plasma pharmacokinetics of LNPs was obtained after a short-term infusion of 2 h for doses of 0.1, 0.3, 0.7, and 1 mg/kg of total RNA. The rate of endocytosis for the LNP, sgRNA, and mRNA
Model assumptions
Several key assumptions were made during the model building process: 1) single-pore disposition was assumed for sgRNA (Li and Shah, 2019; Baxter et al., 1994), 2) immediate release of the transgene product was assumed after administration (Miyazawa et al., 2024), 3) mRNA was assumed to be eliminated via exonuclease metabolism and tissue catabolism (Meibohm and Zhou, 2012), 4) negligible degradation of the RNP complex was assumed, and 5) assuming editing from the liver affects the reduction in biomarkers in the plasma as the liver is the target organ of interest for gene editing.
Software
Data were extracted from the literature and represented as mean values using WebPlotDigitizer (Rohatgi, 2024). The analysis was performed in Monolix 2023R1 (Simulations Plus, 2023). The residual error model used per output that was implemented in the final model was
Here,
Global sensitivity analysis
A GSA was performed considering the complexity of the systems model (Marino et al., 2008; Alden et al., 2013). Sobol GSA was performed in Simbiology (Inc. TM, 2022) in different species in which drug-specific parameters were simultaneously perturbed with a sampling size of 1,000. The lower and upper bounds were different for each parameter and were based on the physiologically plausible value. SOBOL-based sensitivity indexes were simulated for 30 h. SOBOL indexes describe the importance of the parameter, along with its positive or negative correlation with the model output. The model output for mice was selected as the AUC of plasma PK for sgRNA and mRNA, the model output for NHPs was selected as the AUC of plasma PK for the LNP, and the model output for humans was selected as the AUC of plasma PK for LNPs.
Results
Modeling the tissue-level kinetics for unknown drug-specific attributes
A mechanistic model was built to characterize the tissue-level kinetics fitted to mouse, NHP, and human datasets simultaneously that included the measurements of the pharmacokinetics of the LNP, sgRNA, and mRNA. The model recapitulated the concentration of the delivery vehicle, as well as the mRNA and sgRNA in plasma, as shown in Supplementary Figure S2. The drug-specific attributes such as the rate of release of the transgene product from the complex
QSP modeling in mice: a case study with investigational gene therapy
The QSP model (Figures 1A–C and Supplementary Figure S1) was fitted to the mouse pharmacokinetics data, for sgRNA and mRNA in plasma (Finn et al., 2018). The model characterized the PK profile for a dose of 2 mg/kg of total RNA (Figure 2A). The drug-specific attribute such as the rate of exocytosis of the LNP, sgRNA, and mRNA
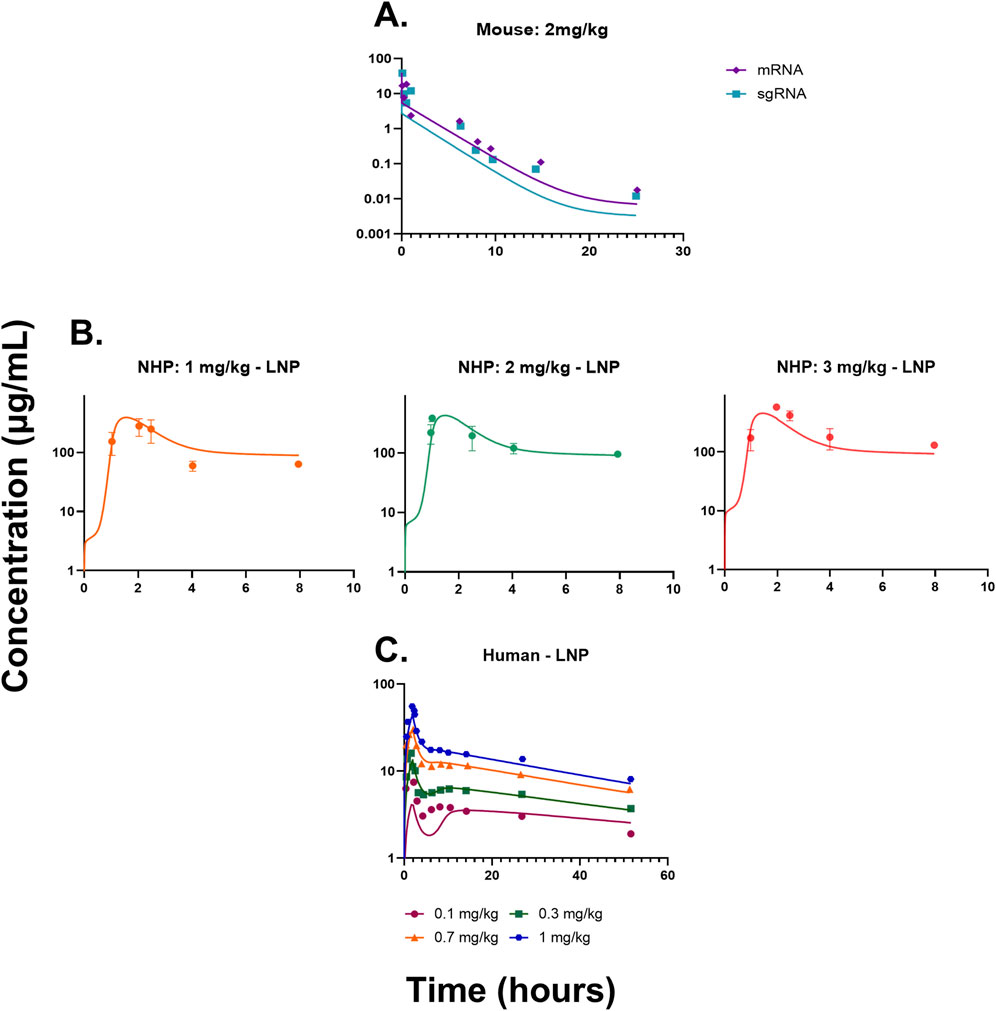
Figure 2. (A) Model fittings for sgRNA and mRNA in plasma for mice from a dose of 2 mg/kg of total RNA dosed via IV bolus. (B) Model fittings for LNPs in plasma for NHPs for doses of 1, 2, and 3 mg/kg of IV infusion for 2 h. (C) Model fittings for LNPs in plasma for humans for doses of 0.1, 0.3, 0.7, and 1 mg/kg of IV infusion for 2 h. The points are the observed data obtained from the literature (Abdelhady et al., 2023; Finn et al., 2018; Gillmore et al., 2021), and the lines are the model fittings.
Translational QSP modeling in NHPs: a case study with NTLA-2001 and VERVE-101
The QSP model was then scaled to NHPs using its respective physiological flows and volumes, which are provided in Supplementary Tables S1, S2. The model recapitulated the dose-dependent changes in PK (Figure 2B) (Gillmore et al., 2021). The drug-specific attributes such as the rate of endocytosis for the LNP, sgRNA, and mRNA
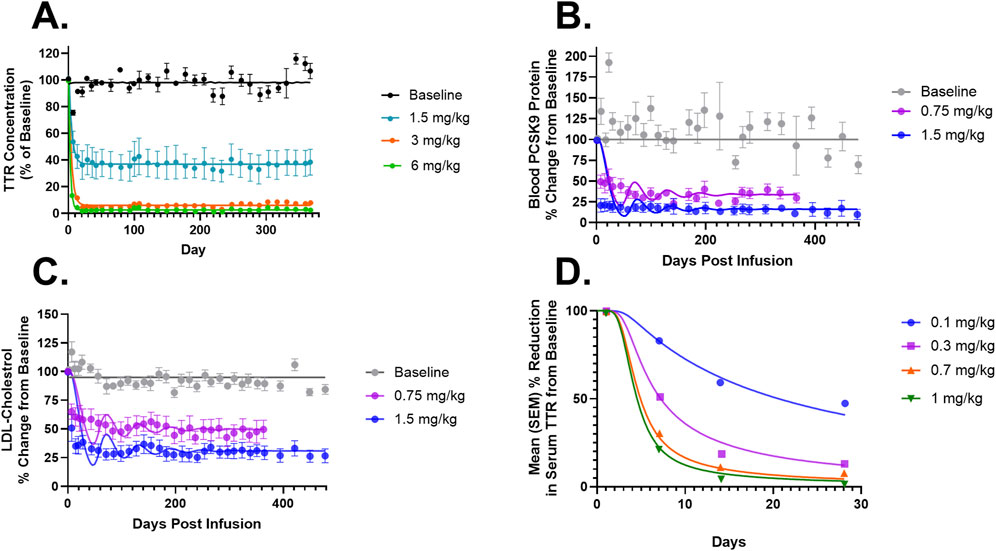
Figure 3. (A) Model fittings for serum TTR proteins in NHPs for doses of 1.5, 3, and 6 mg/kg via IV infusion for 2 h. (B) Model fittings for serum PCSK9 levels in NHPs for doses of 0.75 and 1.5 mg/kg via IV infusion for 2 h. (C) Model fittings for serum LDL cholesterol levels in NHPs for doses of 0.75 and 1.5 mg/kg via IV infusion for 2 h. (D) Model fittings for serum TTR proteins in humans for doses of 0.1, 0.3, 0.7, and 1 mg/kg via IV infusion for 2 h. The points are the observed data obtained from the literature (Gillmore et al., 2021; Lee et al., 2023; Gane et al., 2022).
Translational QSP modeling in humans: a case study with NTLA-2001
The QSP model was scaled to humans using physiological flows and volumes, as shown in Supplementary Tables S1, S2. The resulting model characterized the dose-dependent changes in the PK of LNPs, and it modestly under-predicted the lowest dose (Figure 2C). The drug-specific attributes such as the rate of endocytosis for the LNP, sgRNA, and mRNA
Monte Carlo simulations for the QSP model
Figure 4 describes the Monte Carlo simulations (Singh et al., 2021) for the percentage change from baseline of change in serum TTR protein after the administration of in vivo CRISPR-Cas9 gene therapy. Monte Carlo simulations were performed for 1,000 subjects, assuming 20% variability between the subjects. The simulations revealed that a lower dose of 0.1 mg/kg of total RNA administered exhibited responses in fewer patients, whereas the subsequent dose level of 0.3 mg/kg exhibited a higher response with fewer chances of relapse. The other two higher doses (0.7 mg/kg and 1 mg/kg) of the total RNA administered showed a higher depth of response.
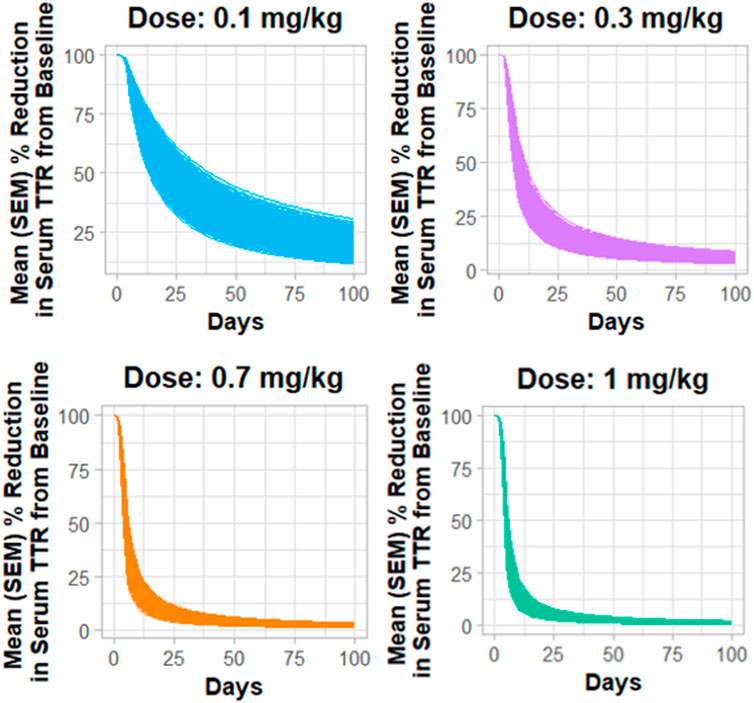
Figure 4. Monte Carlo simulations for serum TTR proteins in humans assuming for 20% variability for the assessment of the dose–response relationship.
Model predictive assessment
In order to assess the predictive performance of the built translational QSP model, Figure 5 shows that the model adequately predicts all the in vivo CRISPR disposition in multiple species, as indicated by the line of identity.
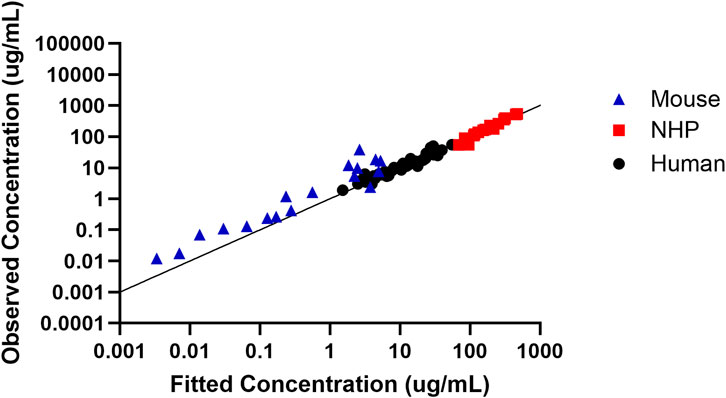
Figure 5. Assessment of the model predictive performance in multiple species. Observed versus fitted concentration of in vivo CRISPR-Cas therapy in multiple species with the line of identity.
Global sensitivity analysis
Figures 6A–C describe a time-variant GSA (SOBOL) on the QSP model developed for NTLA-2001 to characterize the impact on plasma profiles. For mouse sgRNA and mRNA plasma profiles, the rate of endocytosis for LNP, sgRNA, and mRNA, as well as the rate of exocytosis of LNP, sgRNA and mRNA, was found to be sensitive. The rate of degradation of the LNP-LDL complex, as well as the rate of release of the transgene product, was found to be influential on the SOBOL indexes. For NHPs, the rate of dissociation for LNPs to opsonins, as well as the rate of association for LNPs to opsonins, was found to be sensitive on the SOBOL indexes. For humans, the rate of association for LNPs to opsonins and the rate of dissociation for LNPs to opsonins were found to be sensitive in SOBOL indexes.
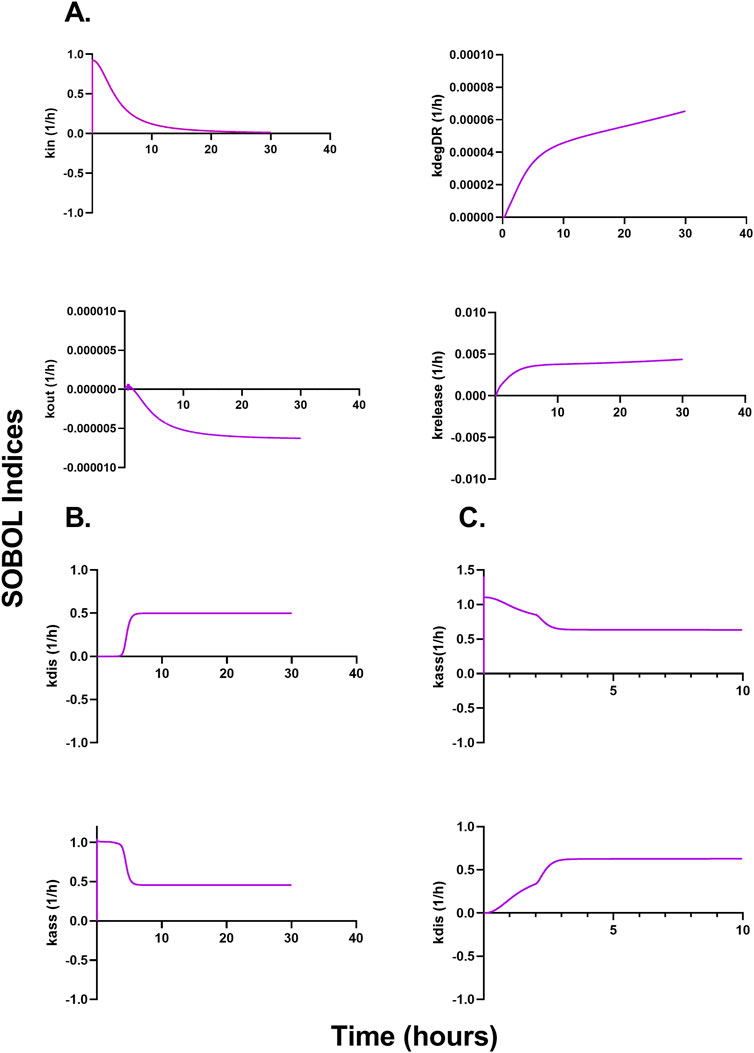
Figure 6. Global sensitivity analysis (GSA) in multiple species accessing the impact of parameters on the model output according to SOBOL indexes. (A) Outcomes of GSA on mice. (B) Outcomes of GSA on NHPs. (C) Outcomes of GSA on humans.
Discussion
Due to their high selectivity for targeted gene editing, CRISPR-Cas-based therapies, as well as other oligonucleotide therapeutics, have gained significant traction across industries and academia over the past decade. CRISPR-Cas therapies offer an ability to edit genes selectively and correct the protein production to cure diseases. Currently, there are five in vivo CRISPR-Cas therapies under development (Abdelhady et al., 2023). The quantitative impact of the putative determinants of CRISPR-Cas activity is only partially understood. It has unique PK-PD characteristics compared to small molecule- and protein-based therapeutics, and it is challenging to establish PK-PD relationships for CRISPR-Cas. For example, there are no established paradigms to leverage preclinical data to predict the safe dose for CRISPR-Cas in humans. In gene therapies, there are previously published studies in oligonucleotides for siRNA, which include a mechanistic platform mPBPK-PD model developed for GaINAc-siRNA, which translates the information from preclinical species to humans (Ayyar and Song, 2024; Ayyar et al., 2021; Zhang et al., 2020). There are previously published models for LNPs, which are present in single species; however, they do not translate the results to clinical settings (Kumar et al., 2023; Mager et al., 2012; Dong et al., 2015; Rajoli et al., 2015; Henrique et al., 2017; Gilkey et al., 2015; MacCalman et al., 2009). An mPBPK/QSP translational model which describes the tissue disposition and protein expression dynamics of LNP-mRNA was found. The study also predicted the efficacious dose via a translational model and included virtual patient population to determine the dosing schedule and efficacious dose (Miyazawa et al., 2024). This model has applications for vaccines and mRNA therapeutics, but this model cannot cover the disposition of CRIPSR-Cas9 and its components, such as sgRNA, as well as the formation of the ribonucleoprotein complex to exert its effect.
In this paper, we developed a novel QSP platform model to characterize the biodistribution and mechanism of action of in vivo CRISPR-Cas9 therapy. The model recapitulated the PK of preclinical species such as mice and NHPs, as well as was translated to humans. The model was built with the data obtained from the literature (Abdelhady et al., 2023; Miyazawa et al., 2024; Finn et al., 2018; Gillmore et al., 2021; Lee et al., 2023; Gane et al., 2022), which include the measurements of sgRNA and mRNA in plasma of mice, and LNPs in plasma of NHPs and humans for characterization of disposition. There are measurements in serum plasma for TTR proteins, as well as for PCSK9 and LDL cholesterol levels, for a different in vivo CRISPR-Cas therapy. The biodistribution model includes receptor-mediated endocytosis of LNPs, the internalization in the mononuclear phagocyte system, bio-corona formation via binding with opsonins in plasma, the release of sgRNA and mRNA, tissue catabolism, and renal clearance. Briefly, once the LNP enters the vascular space, the drug undergoes opsonization by plasma proteins, which leads to bio-corona formation, or the LNP will escape the vascular space by undergoing phagocytosis by MPS cells. These cells are responsible for the distribution and clearance of LNPs. This rate of internalization was fixed to a reported value (Miyazawa et al., 2024). The rate constant for receptor-mediated endocytosis for LNP-, clathrin-, or caveola-mediated endocytosis for sgRNA and mRNA was fixed in mice by allometry scaling from NHPs, whereas it was estimated in NHPs as 0.039 1/h and humans as 0.007 1/h. This value indicated that the value of uptake in the liver cells decreases across species, and further experiments are needed to confirm the change. The rate of exocytosis was estimated to be 6.84 1/h in mice, 2,690 1/h in NHPs, and 775 1/h in humans. The association with opsonins was estimated in NHPs and humans to be 5.06 1/h and 56.8 1/h, respectively, indicating that the LNP undergoes higher opsonization across species, whereas the rate of dissociation with opsonins was estimated to be 8.64 1/h in NHP and 0.186 1/h in humans, respectively, indicating that the LNP has a decreased amount of dissociation with opsonins across species. The total LDL receptor concentration across species decreases from 539 ug/mL estimated in NHPs to 84.5 ug/mL, being a possible reason for the lower uptake of LNP.
To summarize, a translational QSP model was successfully developed to characterize the preclinical-to-clinical translation of in vivo CRISPR-Cas therapies. The model characterized the mechanism of action of two different drugs, NTLA-2001 and VERVE-101, in addition to characterizing the complex biodistribution. Processes such as exocytosis of the LNPs and dissociation of LNPs from opsonins characterized a redistribution phase in the LNP exposure. The model also simulated the variability in response using Monte Carlo simulations. This QSP platform can be applied in the translation of in vivo CRISPR-Cas gene therapies, can be used to gather information regarding the first-in-human dose for these therapies, and provides us with a better understanding of the dose–exposure–response relationship for this modality.
Following the generation of key mechanistic data, future modifications can be done to the model by accounting for changes in physiochemical properties (charge and pI) of the delivery vehicle, as well as accounting for the structural asymmetry of mRNA and sgRNA. A future modification can account for the complex biodistribution of AAV, which is not well explained as a delivery vehicle for CRISPR-Cas therapies. This model can be further expanded based on mRNA and sgRNA PK data in all the organs of the body to characterize the whole-body biodistribution for this modality. Experiments for the characterization of fluid-phase macropinocytosis for mRNA and sgRNA can help leverage the information about the uptake for the transgene product, similar to the experiments used for the characterization of antibodies (Haigler et al., 1979). Furthermore, a drug–trial–disease model can be linked with a toxicokinetic model to characterize the toxicity, as well as help predict the first-in-human dose. The utility of this QSP model will include translating the preclinical PK and PD to humans and help guide the development and translation of in vivo CRISPR-Cas therapies.
Conclusion
In summary, the approach taken in this work demonstrated that several basic, well-established processes that govern pharmacokinetics, such as species physiology, binding, transport, and target-mediated drug disposition, as well as pharmacodynamics such as mechanism of action, biomarkers, and turnover processes, can be assembled to build a translational QSP model for novel CRISPR-Cas therapies. The proposed framework will promote the mechanistic and quantitative reasoning to guide experimental designs during the preclinical and early clinical development of CRISPR-Cas therapies. This systems model will form the basis of characterizing the PK-PD properties of CRISPR-Cas therapeutics.
Data availability statement
The original contributions presented in the study are included in the article/Supplementary Material; further inquiries can be directed to the corresponding authors.
Author contributions
DD: conceptualization, formal analysis, investigation, methodology, validation, visualization, writing–original draft, and writing–review and editing. SS: conceptualization and writing–original draft. RC: conceptualization and writing–original draft.
Funding
The authors declare that no financial support was received for the research, authorship, and/or publication of this article.
Acknowledgments
The authors thank Dr. Vivaswath Ayyar, Dr. Weirong Wang, Dr. Navin Goyal, and Dr. Mahesh Samtani for their valuable comments and feedback, which significantly impacted the research in this article.
Conflict of interest
The authors declare that the research was conducted in the absence of any commercial or financial relationships that could be construed as a potential conflict of interest.
Publisher’s note
All claims expressed in this article are solely those of the authors and do not necessarily represent those of their affiliated organizations, or those of the publisher, the editors, and the reviewers. Any product that may be evaluated in this article, or claim that may be made by its manufacturer, is not guaranteed or endorsed by the publisher.
Supplementary material
The Supplementary Material for this article can be found online at: https://www.frontiersin.org/articles/10.3389/fphar.2024.1454785/full#supplementary-material
References
Abdelhady, A. M., Phillips, J. A., Xu, Y., and Stroh, M. (2023). Clinical pharmacology and translational considerations in the development of CRISPR-based therapies. Clin. Pharmacol. Ther. 114 (3), 591–603. doi:10.1002/cpt.3000
Akinc, A., Querbes, W., De, S., Qin, J., Frank-Kamenetsky, M., Jayaprakash, K. N., et al. (2010). Targeted delivery of RNAi therapeutics with endogenous and exogenous ligand-based mechanisms. Mol. Ther. 18 (7), 1357–1364. doi:10.1038/mt.2010.85
Alden, K., Read, M., Timmis, J., Andrews, P. S., Veiga-Fernandes, H., and Coles, M. (2013). Spartan: a comprehensive tool for understanding uncertainty in simulations of biological systems. PLoS Comput. Biol. 9 (2), e1002916. doi:10.1371/journal.pcbi.1002916
Ayyar, V. S., and Song, D. (2024). Mechanistic pharmacokinetics and pharmacodynamics of GalNAc-siRNA: translational model involving competitive receptor-mediated disposition and RISC-dependent gene silencing applied to givosiran. J. Pharm. Sci. 113 (1), 176–190. doi:10.1016/j.xphs.2023.10.026
Ayyar, V. S., Song, D., Zheng, S., Carpenter, T., and Heald, D. L. (2021). Minimal physiologically based pharmacokinetic-pharmacodynamic (mPBPK-PD) model of N-Acetylgalactosamine-Conjugated small interfering RNA disposition and gene silencing in preclinical species and humans. J. Pharmacol. Exp. Ther. 379, 134–146. doi:10.1124/jpet.121.000805
Baxter, L. T., Zhu, H., Mackensen, D. G., and Jain, R. K. (1994). Physiologically based pharmacokinetic model for specific and nonspecific monoclonal antibodies and fragments in normal tissues and human tumor xenografts in nude mice. Cancer Res. 54 (6), 1517–1528.
Betts, A., Haddish-Berhane, N., Shah, D. K., van der Graaf, P. H., Barletta, F., King, L., et al. (2019). A translational quantitative systems pharmacology model for CD3 bispecific molecules: application to quantify T cell-mediated tumor cell killing by P-cadherin LP DART(®). Aaps J. 21 (4), 66. doi:10.1208/s12248-019-0332-z
Bisgaier, C. L., Siebenkas, M. V., and Williams, K. J. (1989). Effects of apolipoproteins A-IV and A-I on the uptake of phospholipid liposomes by hepatocytes. J. Biol. Chem. 264 (2), 862–866. doi:10.1016/s0021-9258(19)85022-6
Çerçi, B., Uzay, I. A., Kara, M. K., and Dinçer, P. (2023). Clinical trials and promising preclinical applications of CRISPR/Cas gene editing. Life Sci. 312, 121204. doi:10.1016/j.lfs.2022.121204
Chen, W., Ma, Y., Liu, X., and Zhu, D. (2022). Polyester materials for mRNA delivery. Explor Target Antitumor Ther. 3 (2), 117–127. doi:10.37349/etat.2022.00075
Del Toro Runzer, C., Anand, S., Mota, C., Moroni, L., Plank, C., van Griensven, M., et al. (2023). Cellular uptake of modified mRNA occurs via caveolae-mediated endocytosis, yielding high protein expression in slow-dividing cells. Mol. Ther. Nucleic Acids 32, 960–979. doi:10.1016/j.omtn.2023.05.019
den Roover, S., and Aerts, J. L. (2023). Unveiling the intricacies of gene delivery: caveolae-mediated endocytosis induces efficient mRNA delivery in slow-dividing cells. Mol. Ther. Nucleic Acids 33, 545–547. doi:10.1016/j.omtn.2023.07.024
Desai, A. S., Hunter, M. R., and Kapustin, A. N. (2019). Using macropinocytosis for intracellular delivery of therapeutic nucleic acids to tumour cells. Philos. Trans. R. Soc. Lond B Biol. Sci. 374 (1765), 20180156. doi:10.1098/rstb.2018.0156
Dong, D., Wang, X., Wang, H., Zhang, X., Wang, Y., and Wu, B. (2015). Elucidating the in vivo fate of nanocrystals using a physiologically based pharmacokinetic model: a case study with the anticancer agent SNX-2112. Int. J. Nanomedicine 10, 2521–2535. doi:10.2147/IJN.S79734
Finn, J. D., Smith, A. R., Patel, M. C., Shaw, L., Youniss, M. R., van Heteren, J., et al. (2018). A single administration of CRISPR/Cas9 lipid nanoparticles achieves robust and persistent in vivo genome editing. Cell Rep. 22 (9), 2227–2235. doi:10.1016/j.celrep.2018.02.014
Friberg, L. E., Henningsson, A., Maas, H., Nguyen, L., and Karlsson, M. O. (2002). Model of chemotherapy-induced myelosuppression with parameter consistency across drugs. J. Clin. Oncol. 20 (24), 4713–4721. doi:10.1200/JCO.2002.02.140
Fujiwara, Y., Wada, K., and Kabuta, T. (2016). Lysosomal degradation of intracellular nucleic acids—multiple autophagic pathways. J. Biochem. 161 (2), 145–154. doi:10.1093/jb/mvw085
Gane, E. J., Täubel, J., Pilebro, B., Fontana, M., Kao, J., Maitland, M., et al. (2022). In vivo CRISPR/Cas9 editing of the TTR gene with NTLA-2001 in patients with transthyretin amyloidosis-dose selection considerations. J. Hepatology 77, S58–S59. doi:10.1016/s0168-8278(22)00520-7
Gilkey, M., Krishnan, V., Scheetz, L., Jia, X., Rajasekaran, A., and Dhurjati, P. (2015). Physiologically based pharmacokinetic modeling of fluorescently labeled block copolymer nanoparticles for controlled drug delivery in leukemia therapy. CPT pharmacometrics and Syst. Pharmacol. 4 (3), e00013–e00074. doi:10.1002/psp4.13
Gillmore, J. D., Gane, E., Taubel, J., Kao, J., Fontana, M., Maitland, M. L., et al. (2021). CRISPR-Cas9 in vivo gene editing for transthyretin amyloidosis. N. Engl. J. Med. 385 (6), 493–502. doi:10.1056/NEJMoa2107454
Grimm, E., van der Hoeven, F., Sardella, D., Willig, K. I., Engel, U., Veits, N., et al. (2022). A Clathrin light chain A reporter mouse for in vivo imaging of endocytosis. PLoS One 17 (9), e0273660. doi:10.1371/journal.pone.0273660
Haigler, H. T., McKanna, J. A., and Cohen, S. (1979). Rapid stimulation of pinocytosis in human carcinoma cells A-431 by epidermal growth factor. J. Cell Biol. 83 (1), 82–90. doi:10.1083/jcb.83.1.82
Harwood, H. J., and Pellarin, L. D. (1997). Kinetics of low-density lipoprotein receptor activity in Hep-G2 cells: derivation and validation of a Briggs-Haldane-based kinetic model for evaluating receptor-mediated endocytotic processes in which receptors recycle. Biochem. J. 323 (Pt 3), 649–659. doi:10.1042/bj3230649
Henrique, S. A., Lima, Jr E., Vasquez Mansilla, M., Zysler, R. D., Mojica Pisciotti, M. L., Locatelli, C., et al. (2017). A physiologically based pharmacokinetic model to predict the superparamagnetic iron oxide nanoparticles (SPIONs) accumulation in vivo. Eur. J. Nanomedicine 9 (2), 79–90. doi:10.1515/ejnm-2017-0001
Hoshyar, N., Gray, S., Han, H., and Bao, G. (2016). The effect of nanoparticle size on in vivo pharmacokinetics and cellular interaction. Nanomedicine (Lond). 11 (6), 673–692. doi:10.2217/nnm.16.5
Hou, X., Zaks, T., Langer, R., and Dong, Y. (2021). Lipid nanoparticles for mRNA delivery. Nat. Rev. Mater. 6 (12), 1078–1094. doi:10.1038/s41578-021-00358-0
Jinek, M., Chylinski, K., Fonfara, I., Hauer, M., Doudna, J. A., and Charpentier, E. (2012). A programmable dual-RNA-guided DNA endonuclease in adaptive bacterial immunity. Science 337 (6096), 816–821. doi:10.1126/science.1225829
Johnson, L. A., Olsen, R. H., Merkens, L. S., DeBarber, A., Steiner, R. D., Sullivan, P. M., et al. (2014). Apolipoprotein E-low density lipoprotein receptor interaction affects spatial memory retention and brain ApoE levels in an isoform-dependent manner. Neurobiol. Dis. 64, 150–162. doi:10.1016/j.nbd.2013.12.016
Kavita, U., Sun, K., Braun, M., Lembke, W., Mody, H., Kamerud, J., et al. (2023). PK/PD and bioanalytical considerations of AAV-based gene therapies: an IQ consortium industry position paper. Aaps J. 25 (5), 78. doi:10.1208/s12248-023-00842-1
Kotit, S. (2023). Lessons from the first-in-human in vivo CRISPR/Cas9 editing of the TTR gene by NTLA-2001 trial in patients with transthyretin amyloidosis with cardiomyopathy. Glob. Cardiol. Sci. Pract. 2023 (1), e202304. doi:10.21542/gcsp.2023.4
Kumar, M., Kulkarni, P., Liu, S., Chemuturi, N., and Shah, D. K. (2023). Nanoparticle biodistribution coefficients: a quantitative approach for understanding the tissue distribution of nanoparticles. Adv. Drug Deliv. Rev. 194, 114708. doi:10.1016/j.addr.2023.114708
Lee, R. G., Mazzola, A. M., Braun, M. C., Platt, C., Vafai, S. B., Kathiresan, S., et al. (2023). Efficacy and safety of an investigational single-course CRISPR base-editing therapy targeting PCSK9 in nonhuman primate and mouse models. Circulation 147 (3), 242–253. doi:10.1161/CIRCULATIONAHA.122.062132
Li, Y., Bolinger, J., Yu, Y., Glass, Z., Shi, N., Yang, L., et al. (2019). Intracellular delivery and biodistribution study of CRISPR/Cas9 ribonucleoprotein loaded bioreducible lipidoid nanoparticles. Biomater. Sci. 7 (2), 596–606. doi:10.1039/c8bm00637g
Li, Z., and Shah, D. K. (2019). Two-pore physiologically based pharmacokinetic model with de novo derived parameters for predicting plasma PK of different size protein therapeutics. J. Pharmacokinet. Pharmacodyn. 46 (3), 305–318. doi:10.1007/s10928-019-09639-2
Liu, C., Chen, J., Chen, H., Zhang, T., He, D., Luo, Q., et al. (2022). PCSK9 inhibition: from current advances to evolving future. Cells 11 (19), 2972. doi:10.3390/cells11192972
Liu, S., Chowdhury, E. A., Xu, V., Jerez, A., Mahmood, L., Ly, B. Q., et al. (2024). Whole-body disposition and physiologically based pharmacokinetic modeling of adeno-associated viruses and the transgene product. J. Pharm. Sci. 113 (1), 141–157. doi:10.1016/j.xphs.2023.10.005
MacCalman, L., Tran, C. L., and Kuempel, E. (2009). Development of a bio-mathematical model in rats to describe clearance, retention and translocation of inhaled nano particles throughout the body. J. Phys. Conf. Ser. 151, 012028. doi:10.1088/1742-6596/151/1/012028
Mager, D. E., and Jusko, W. J. (2001). General pharmacokinetic model for drugs exhibiting target-mediated drug disposition. J. Pharmacokinet. Pharmacodyn. 28 (6), 507–532. doi:10.1023/a:1014414520282
Mager, D. E., and Jusko, W. J. (2008). Development of translational pharmacokinetic-pharmacodynamic models. Clin. Pharmacol. Ther. 83 (6), 909–912. doi:10.1038/clpt.2008.52
Mager, D. E., Mody, V., Xu, C., Forrest, A., Lesniak, W. G., Nigavekar, S. S., et al. (2012). Physiologically based pharmacokinetic model for composite nanodevices: effect of charge and size on in vivo disposition. Pharm. Res. 29 (9), 2534–2542. doi:10.1007/s11095-012-0784-7
Marino, S., Hogue, I. B., Ray, C. J., and Kirschner, D. E. (2008). A methodology for performing global uncertainty and sensitivity analysis in systems biology. J. Theor. Biol. 254 (1), 178–196. doi:10.1016/j.jtbi.2008.04.011
Meibohm, B., and Zhou, H. (2012). Characterizing the impact of renal impairment on the clinical pharmacology of biologics. J. Clin. Pharmacol. 52 (1 Suppl. l), 54S–62S. doi:10.1177/0091270011413894
Miyazawa, K., Liu, Y., and Bazzazi, H. (2024). Development of a minimal PBPK-QSP modeling platform for LNP-mRNA based therapeutics to study tissue disposition and protein expression dynamics. Front. Nanotechnol. 6. doi:10.3389/fnano.2024.1330406
Monopoli, M. P., Aberg, C., Salvati, A., and Dawson, K. A. (2012). Biomolecular coronas provide the biological identity of nanosized materials. Nat. Nanotechnol. 7 (12), 779–786. doi:10.1038/nnano.2012.207
Oladipo, E. K., Adeniyi, M. O., Ogunlowo, M. T., Irewolede, B. A., Adekanola, V. O., Oluseyi, G. S., et al. (2022). Bioinformatics designing and molecular modelling of a universal mRNA vaccine for SARS-CoV-2 infection. Vaccines (Basel) 10 (12), 2107. doi:10.3390/vaccines10122107
Paunovska, K., Da Silva Sanchez, A. J., Lokugamage, M. P., Loughrey, D., Echeverri, E. S., Cristian, A., et al. (2022). The extent to which lipid nanoparticles require apolipoprotein E and low-density lipoprotein receptor for delivery changes with ionizable lipid structure. Nano Lett. 22 (24), 10025–10033. doi:10.1021/acs.nanolett.2c03741
Rajoli, R. K., Back, D. J., Rannard, S., Freel Meyers, C. L., Flexner, C., Owen, A., et al. (2015). Physiologically based pharmacokinetic modelling to inform development of intramuscular long-acting nanoformulations for HIV. Clin. Pharmacokinet. 54, 639–650. doi:10.1007/s40262-014-0227-1
Rohatgi, A. (2024). WebPlotDigitizer. Available at: https://automeris.io/WebPlotDigitizer.html.
Rouet, R., and Christ, D. (2019). Efficient intracellular delivery of CRISPR-cas ribonucleoproteins through receptor mediated endocytosis. ACS Chem. Biol. 14 (3), 554–561. doi:10.1021/acschembio.9b00116
Sebastiani, F., Yanez Arteta, M., Lerche, M., Porcar, L., Lang, C., Bragg, R. A., et al. (2021). Apolipoprotein E binding drives structural and compositional rearrangement of mRNA-containing lipid nanoparticles. ACS Nano 15 (4), 6709–6722. doi:10.1021/acsnano.0c10064
Shah, D. K., and Betts, A. M. (2012). Towards a platform PBPK model to characterize the plasma and tissue disposition of monoclonal antibodies in preclinical species and human. J. Pharmacokinet. Pharmacodyn. 39 (1), 67–86. doi:10.1007/s10928-011-9232-2
Singh, A. P., Chen, W., Zheng, X., Mody, H., Carpenter, T. J., Zong, A., et al. (2021). Bench-to-bedside translation of chimeric antigen receptor (CAR) T cells using a multiscale systems pharmacokinetic-pharmacodynamic model: a case study with anti-BCMA CAR-T. CPT Pharmacometrics Syst. Pharmacol. 10 (4), 362–376. doi:10.1002/psp4.12598
Sternberg, S. H., Redding, S., Jinek, M., Greene, E. C., and Doudna, J. A. (2014). DNA interrogation by the CRISPR RNA-guided endonuclease Cas9. Nature 507 (7490), 62–67. doi:10.1038/nature13011
Suzuki, Y., and Ishihara, H. (2021). Difference in the lipid nanoparticle technology employed in three approved siRNA (Patisiran) and mRNA (COVID-19 vaccine) drugs. Drug Metab. Pharmacokinet. 41, 100424. doi:10.1016/j.dmpk.2021.100424
Wu, Z., and Li, T. (2021). Nanoparticle-mediated cytoplasmic delivery of messenger RNA vaccines: challenges and future perspectives. Pharm. Res. 38 (3), 473–478. doi:10.1007/s11095-021-03015-x
Xia, X. D., Peng, Z. S., Gu, H. M., Wang, M., Wang, G. Q., and Zhang, D. W. (2021). Regulation of PCSK9 expression and function: mechanisms and therapeutic implications. Front. Cardiovasc. Med. 8, 764038. doi:10.3389/fcvm.2021.764038
Keywords: quantitative systems pharmacology, gene therapy, liver disease, rare disease, translational modeling
Citation: Desai DA, Schmidt S and Cristofoletti R (2024) A quantitative systems pharmacology (QSP) platform for preclinical to clinical translation of in-vivo CRISPR-Cas therapy. Front. Pharmacol. 15:1454785. doi: 10.3389/fphar.2024.1454785
Received: 25 June 2024; Accepted: 30 August 2024;
Published: 20 September 2024.
Edited by:
Takeo Nakanishi, Takasaki University of Health and Welfare, JapanReviewed by:
Duverney Chaverra-Rodriguez, National University of Colombia, ColombiaHanwen Wang, Johns Hopkins University, United States
Copyright © 2024 Desai, Schmidt and Cristofoletti. This is an open-access article distributed under the terms of the Creative Commons Attribution License (CC BY). The use, distribution or reproduction in other forums is permitted, provided the original author(s) and the copyright owner(s) are credited and that the original publication in this journal is cited, in accordance with accepted academic practice. No use, distribution or reproduction is permitted which does not comply with these terms.
*Correspondence: Devam A. Desai, ZGV2YW1kZXNhaUB1ZmwuZWR1; Rodrigo Cristofoletti, cmNyaXN0b2ZvbGV0dGlAdWZsLmVkdQ==