- 1Department of Oncology, The Affiliated Hospital of Yangzhou University, Yangzhou University, Yangzhou, Jiangsu, China
- 2Department of Hepatobiliary and Pancreatic Surgery, Jilin University Second Hospital, Changchun, Jilin, China
- 3Department of Oncology, Northern Jiangsu People’s Hospital Affiliated to Yangzhou University, Yangzhou University, Yangzhou, Jiangsu, China
- 4Department of Oncology, Baoying Traditional Chinese Medicine Hospital, Yangzhou University, Yangzhou, Jiangsu, China
- 5Department of Thoracic Surgery, The Affiliated Hospital of Yangzhou University, Yangzhou University, Yangzhou, Jiangsu, China
Objective: Metformin as a common antidiabetic drug, has recently found to exert its anti-cancer and immunomodulatory effect in numerous preclinical studies. This study aims to clarify the prognostic impact of metformin use in solid cancer patients receiving immune checkpoint inhibitors (ICIs).
Methods: A retrospective cohort enrolling 516 solid cancer patients who received ICI-based therapy between 2018 and 2023 at three hospitals was analyzed. The primary endpoints included overall survival (OS) and progression-free survival (PFS). In addition, a bioinformatics analysis based on TCGA and GSE cohort was performed to investigate the prognostic significance of metformin target genes (MTGs) and their correlation with immune infiltration in non-small cell lung cancer (NSCLC) patients.
Results: In the entire cohort, a total of 76 patients received metformin before and/or during ICI therapy. The global analysis demonstrated that metformin use was unrelated with the OS (p = 0.064) and PFS (p = 0.059) of ICI-treated cancer patients, which was confirmed in the subgroups of esophagus, hepatobiliary or pancreatic cancer (all p > 0.05). However, metformin use was significantly correlated with better OS (p = 0.012) and PFS (p = 0.005) in ICI-treated lung cancer patients. Metformin use was also identified as an independent favorable prognostic factor for these patients. The bioinformatics analysis identified five favorable prognostic MTGs (RPS6KA5, RORA, SH3BP5, NUPR1, and CD40LG) for NSCLC patients, all of which was downregulated in lung cancer tissues as compared with normal tissues. The expressions of five MTGs not only could effectively stratify the OS of NSCLC patients, but also was correlated with infiltration of immune cells such as CD4+ and CD8+ T cells.
Conclusion: Metformin use was significantly correlated with better OS and PFS in ICI-treated lung cancer patients. MTGs has the potential to serve as novel clinical biomarkers or druggable targets for cancer immunotherapy. Considering study limitations, the actual impact of metformin use on ICI therapy needs to be clarified by more clinical trials.
1 Introduction
Cancer is one of major public health burdens worldwide, with approximately 20 million new cases and 9.7 million relevant deaths estimated in 2022 (Bray et al., 2024). Despite improved precancerous screening and diagnostic techniques, a considerable proportion of cancer patients are initially diagnosed at advanced stage and recommended to receive comprehensive therapies including surgery, chemoradiotherapy, targeted therapy and immunotherapy. The past decade has witnessed the great success of immunotherapy in advanced tumors and its representative drugs are known as immune checkpoint inhibitors (ICIs) including anti-programmed cell death-1 (PD-1), programmed cell death ligand-1 (PD-L1) and Cytotoxic T lymphocyte-associated antigen-4 (CTLA-4) antibodies (Capella et al., 2024; Jama et al., 2024). Although mounting clinical trials have proved their durable anti-cancer efficacy and acceptable toxicity, limited patients are actually found to benefit from ICI therapies. Numerous inherent factors have been closely linked to the efficacy of ICI drugs such as PD-L1 expression, microsatellite status, tumor mutation burden (TMB) and microbiome (Emens et al., 2024; Holder et al., 2024). Previously, our team has identified individual nutritional and performance status as significant factors affecting ICI efficacy (Yan et al., 2023; Wang et al., 2024). In addition, our team have found the actual efficacy of ICI drugs may be also affected by some concomitant medications including antibiotics, proton pump inhibitors, corticosteroids, β-blockers and opioids (Yang et al., 2020; Wang et al., 2021; Liu et al., 2022; Yan et al., 2022; Guo et al., 2024). A recent review has summarized the conflicting results about the impact of concomitant medications on ICI drugs in non-small cell lung cancer (NSCLC), emphasizing the necessity of more investigations on this aspect (Chen et al., 2023). A further understanding about the role of concomitant medications in cancer immunotherapy will undoubtedly contribute to more precise patient management, and finally lead to overall survival benefit.
Metformin, as a first-line medication for type 2 diabetes mellitus (T2DM), has recently exhibited its anti-cancer potential in numerous biological and clinical studies. A national cohort study has found melanoma patients with T2DM who received metformin had reduced risk of cancer-specific mortality (Urbonas et al., 2020). A comprehensive meta-analysis including 166 studies has proved metformin use is significantly correlated with a decreased risk for gastrointestinal, urologic and hematologic cancers (O’connor et al., 2024). Metformin use is also correlated with better clinical outcome in cancer patients and can act as an effective adjuvant therapy combined with traditional chemoradiotherapy (Júnior et al., 2021; Bahardoust et al., 2024). In terms of anti-cancer mechanisms, metformin can directly inhibit the malignant characteristics of cancer cells through activating AMPK signaling, or indirectly prevent tumorigenesis through controlling circulating glucose and insulin levels (Linkeviciute-Ulinskiene et al., 2020). A recent review has closely linked metformin use with increased CD8+ T cells and natural killer (NK) cells, suggesting its potential boosting effect on cancer immunotherapy (Panaampon et al., 2023). However, the actual impact of metformin use on ICI efficacy remains controversial in clinical studies. For instance, Afzal et al. have found ICIs combined with metformin could effectively improve the tumor response, overall survival (OS) and progression-free survival (PFS) of NSCLC patients (Afzal et al., 2019). In contrast, another retrospective study has demonstrated no significant correlation between metformin use and clinical outcome in NSCLC patients receiving nivolumab (Svaton et al., 2020). Moreover, a multicenter retrospective study even has found metformin use was correlated with increased risk of disease progression and death in ICI-treated solid cancer patients (Cortellini et al., 2023). Therefore, more investigations are urgently needed to clarify the actual role of metformin in cancer immunotherapy.
In this study, a multicenter cohort of 516 solid cancer patients receiving ICI-based therapies was used to evaluate the impact of metformin use on patient prognosis. In addition, a comprehensive bioinformatic analysis was performed to investigate the potential correlation between the metformin target genes (MTGs) and immune cells. The study will provide novel insights into the anti-cancer role of metformin, contributing to precise management of concomitant medications during ICI therapy.
2 Materials and methods
2.1 Study design and patient information
Between January 2018 and December 2023, a total of 680 patients were initially selected from three medical centers: The Affiliated Hospital of Yangzhou University (n = 492), Northern Jiangsu People’s Hospital Affiliated to Yangzhou University (n = 120) and Baoying Traditional Chinese Medicine Hospital (n = 68). The inclusion criteria were as follows: 1) age over 18 years old; 2) patients were pathologically diagnosed as solid cancers including lung and digestive cancers; 3) patients received ICI therapy with or without other anti-cancer therapies including chemotherapy, radiotherapy and targeted therapy. The exclusion criteria were as follows: 1) multiple primary tumors; 2) incomplete medical and/or follow-up records; 3) insufficient ICI therapy (less than two cycles); 4) unavailable informed consents for using patient information. As a result, a total of 516 patients were included in the study, among which 76 patients received metformin therapy before and/or during ICI therapy. The flowchart of patient recruitment was shown in Figure 1A. This study was approved by the local ethics committee (No. 2022-YKL11-class 05) and informed consents were acquired from patients or their legal guardians for using their medical and follow-up records in scientific researches.
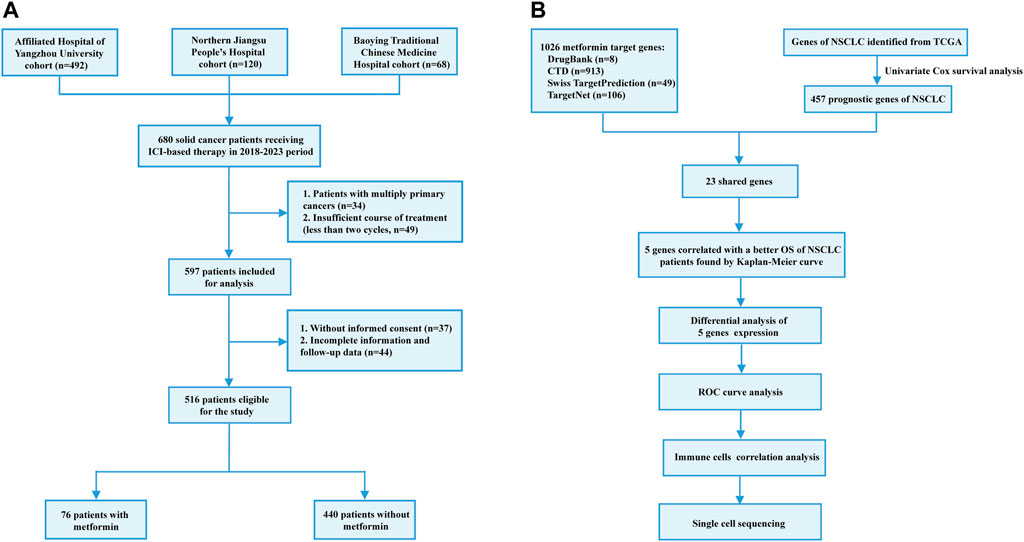
Figure 1. Flowchart of patient recruitment in the retrospective study (A) and identification of metformin target genes (B).
2.2 Treatment strategy
All the included patients received ICI therapy every two or three weeks. The types of ICI drugs were as follows: sintilimab (n = 184), camrelizumab (n = 134), tirelizumab (n = 108), toripalimab (n = 22), pembrolizumab (n = 20), serplulimab (n = 17), nivolumab (n = 11), durvalumab (n = 7), envafolimab (n = 6), atezolizumab (n = 4), penpulimab (n = 2) and adebrelimab (n = 1). 447 and 33 patients received chemotherapy and radiotherapy respectively. 106 patients received targeted therapy and the used drugs were as follows: anlotinib (n = 26), apatinib (n = 23), lenvatinib (n = 20), bevacizumab (n = 16), trastuzumab (n = 8), regorafenib (n = 5), sulfatinib (n = 3), sorafenib (n = 2), pyrotinib (n = 1), furoquinib (n = 1), gefitinib (n = 1) and nimotuzumab (n = 1).
2.3 Follow-up and study endpoints
For oncological evaluation, all the included patients received tumor marker detection and radiological examination every two or three cycles. The anti-cancer therapy response was evaluated based on the Response Evaluation Criteria in Solid Tumors (RECIST) 1.1. The therapy decision was performed based on oncological and safety evaluation. The study endpoints contained OS and PFS. OS was defined as the time interval between the first ICI therapy and death from any cause or the last follow-up. PFS was defined as the time interval between the first ICI therapy and disease progression.
2.4 Identification of prognostic metformin target genes in online databases
The metformin target genes (MTGs) were obtained from the DrugBank (Knox et al., 2024) (https://go.drugbank.com), Comparative Toxicogenomics Database (Davis et al., 2023) (https://ctdbase.org/), Swiss Target Prediction (Daina et al., 2019) (http://www.swisstargetprediction.ch/) and TargetNet (Yao et al., 2016) (http://targetnet.scbdd.com/calcnet/index/). In addition, the transcriptomic data of Lung Adenocarcinoma (LUAD) and Lung Squamous Cell Carcinoma (LUSC) from The Cancer Genome Atlas (TCGA, https://portal.gdc.cancer.gov/v1/) were downloaded as the NSCLC dataset. Firstly, favorable prognostic genes were identified from the NSCLC dataset using the univariate Cox regression method. The eligibility criteria were used as follows: 1) p-value less than 0.05; 2) hazard ratio (HR) value less than 1. Secondly, the shared genes of both the favorable NSCLC prognostic genes and MTGs were selected. Finally, the Kaplan-Meier model was utilized to validate the prognostic value of the prognostic MTGs in the NSCLC dataset. The flowchart of identifying prognostic MTGs was shown in Figure 1B.
2.5 Immune infiltration analysis
The correlation between MTGs and proportions of immune cells was analyzed using single sample gene set enrichment analysis (ssGSEA) in “GSVA” and “GSEABase” packages. In addition, the expressions of MTGs in immune cells of NSCLC patients were analyzed using single-cell sequencing data that were available in TISCH database (http://tisch.comp-genomics.org/home/).
2.6 Statistical analysis
The statistical analysis was performed using SPSS 25.0 or R 4.3.0 software. The correlations between metformin use and clinical features were analyzed using chi-squared test. The survival curves were plotted using Kaplan-Meier model and intergroup difference was evaluated using the log-rank test. Independent prognostic factors were identified using the univariate and multivariate analysis based on Cox proportional hazards regression model. The performance of MTGs in predicting clinical outcome was analyzed using receiver operator characteristic (ROC) curves. A p-value less than 0.05 indicates statistical significance.
3 Results
3.1 General description of patient characteristics in the multicenter cohort
Based on the inclusion and exclusion criteria, a total of 516 patients were finally selected for our retrospective analysis and their clinical features were shown in Table 1. In brief, 123 (23.8%) and 393 (76.2%) patients were female and male respectively, with the overall median age of 68 years old. The most common cancer type was lung cancer (n = 199), followed by esophageal cancer (n = 164), gastrointestinal cancer (n = 85), hepatobiliary and pancreatic cancer (n = 68). 125 (24.2%) patients had previously received tumor resection and 209 (40.5%) patients had smoking history. Only 36 (7.0%) patients received ICI monotherapy, while the others received combined therapies. Before the last follow-up, 294 patients were dead from tumor progression while 55 patients were dead from other reasons such as infection, cerebrovascular diseases and therapy-related adverse events. 76 patients received metformin therapy with drug dose ranging from 500 mg to 2000 mg per day. The correlation analysis demonstrated metformin use were significantly correlated with body mass index (BMI) (p = 0.028) and treatment strategy (p = 0.005). No significant correlation was observed between metformin use and other clinical features including gender (p = 0.973), age (p = 0.955), cancer type (p = 0.231), surgery history (p = 0.453), tumor staging (p = 0.333), ECOG score (p = 0.568) and smoking history (p = 0.286).
3.2 Prognostic significance of metformin use in the multicenter cohort
As shown in Supplemenary Figure S1A, no statistically significant difference was observed in the OS between the metformin group and non-metformin group (p = 0.064). Similarly, the PFS of the metformin group was found to be no better than that of non-metformin group (p = 0.059, Supplemenary Figure S1B). In addition, the univariate analysis failed to identify metformin use as a significant prognostic factor affecting the OS or PFS of the patients (OS: p = 0.073; PFS: p = 0.072; Table 2).
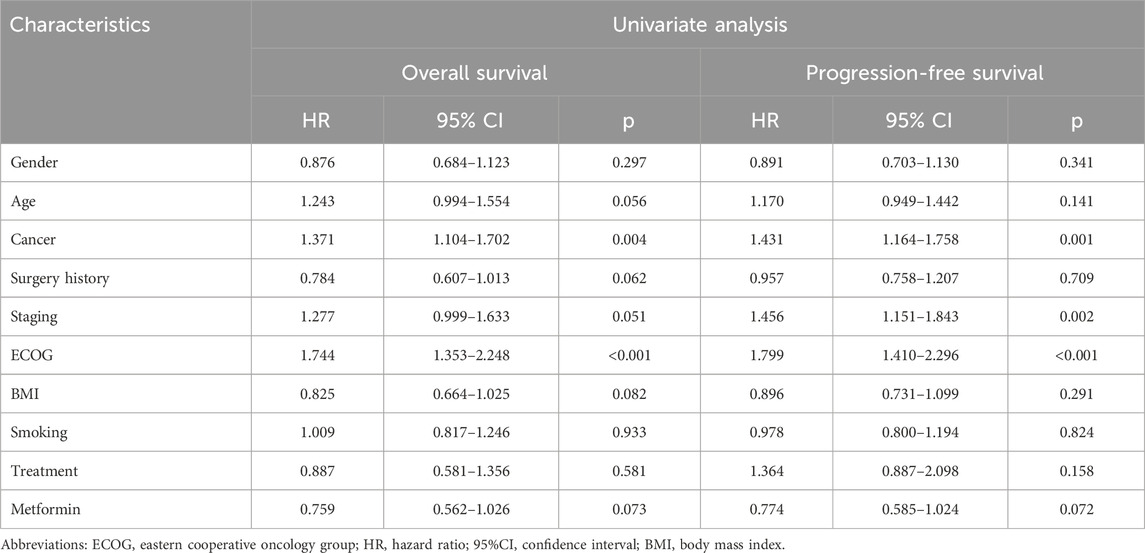
Table 2. Univariate analysis for overall survival and progression-free survival of the entire cohort.
3.3 Prognostic significance of metformin use in the selected cancer types
For further clarifying the prognostic significance of metformin use in ICI-treated patients, the subgroup analysis was performed based on cancer types. In patients with lung cancer (n = 199), 34 patients received metformin therapy. As shown in Figures 2A,B, metformin use was found to be significantly correlated with better OS and PFS in ICI-treated lung cancer patients (OS: p = 0.012; PFS: p = 0.005). This correlation was also statistically significant in the subgroup analysis based on small cell lung cancer (SCLC, OS: p = 0.010, Supplementary Figure S2A; PFS: p = 0.017; Supplementary Figure S2B) and NSCLC (OS: p = 0.033, Supplementary Figure S2C; PFS: p = 0.009; Supplementary Figure S2D). In the univariate analysis, metformin use (p = 0.008), together with tumor staging (p = 0.005), ECOG (p = 0.001) and smoking history (p = 0.005) were significant factors affecting the PFS of lung cancer patients (Table 3). In the multivariate analysis, metformin use (p = 0.013), together with tumor staging (p = 0.017) and smoking history (p = 0.028) were further identified as independent predictive factors for the PFS of lung cancer patients. In terms of OS, metformin use (p = 0.016), ECOG (p = 0.016) and smoking history (p = 0.023) were significant prognostic factors in the univariate analysis (Table 4). However, only metformin use was found to be an independent predictive factor for OS (p = 0.026).
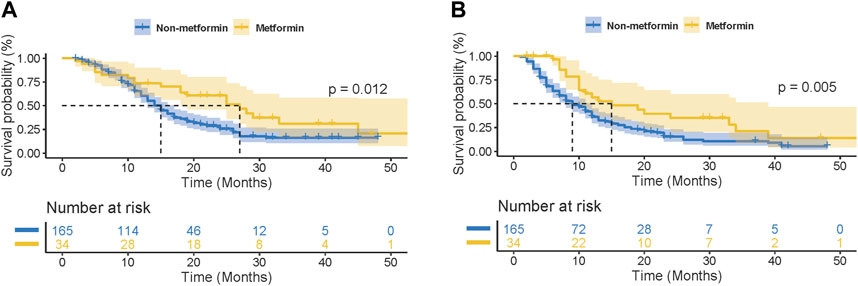
Figure 2. Kaplan-Meier curves for the association of metformin use with overall survival (OS) (A) and progression-free survival (PFS) (B) in lung cancer patients receiving immune checkpoint inhibitors.
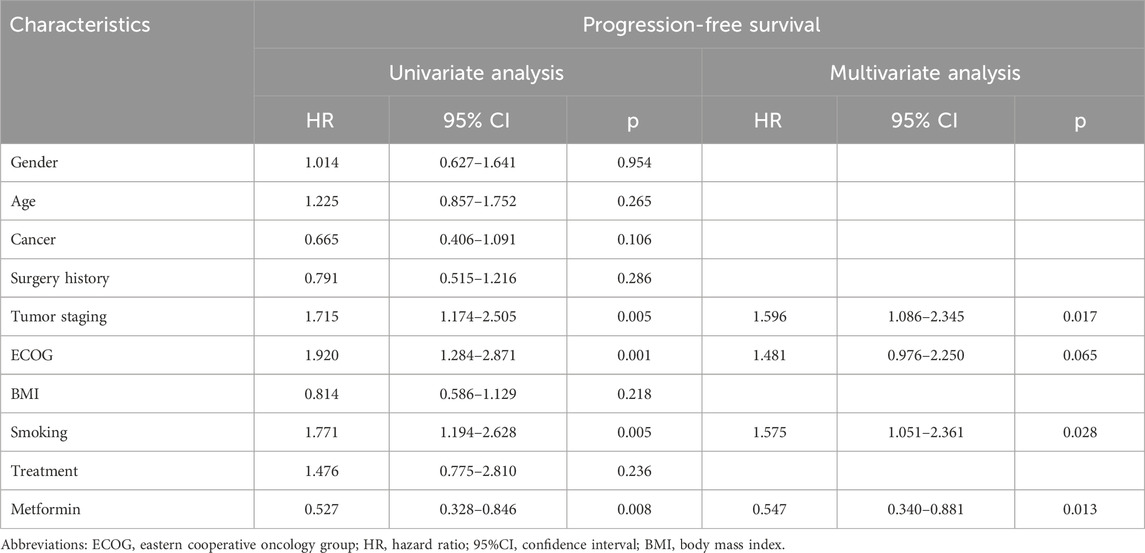
Table 3. Univariate and multivariate analysis for the progression-free survival of the lung cancer cohort.
In patients with esophagus cancer (n = 164), 22 patients received metformin therapy. As shown in Supplementary Figure S3A and B, no significant correlation was observed between metformin use and OS (p = 0.672) or PFS (p = 0.898). In patients with hepatobiliary or pancreatic cancer (n = 68), 11 patients received metformin therapy. The correlation between metformin use and clinical outcome still failed to be statistically significant (OS: p = 0.439, Supplementary Figure S3C; PFS: p = 0.754; Supplementary Figure S3D). We failed to perform the analysis in the gastrointestinal subgroup due to the limited sample size of the metformin group (n = 9).
3.4 Identification of favorable prognostic MTGs in NSCLC patients
Since our subgroup analysis revealed metformin use was associated with better clinical outcome in ICI-treated lung cancer patients, we next aimed to investigate the underlying molecular mechanisms based on network pharmacology. As shown in Figure 3A, a total of 1,026 MTGs were initially identified from four online databases and the details were provided in Supplementary Table S1. Meanwhile, using a univariate Cox regression model, 457 favorable prognostic genes related with NSCLC were identified from the TCGA cohort and the details were provided in Supplementary Table S2. Then, the following 23 shared genes between MTGs and favorable prognostic genes were selected: SLC47A1, CYP17A1, RPS6KA5, TP53INP1, ABCC4, BCL6, CCR2, CD40LG, CD74, CISH, GDF15, GMPR, IL33, MCTP2, NUPR1, PLEKHB1, PLPPR1, PXMP4, SH3BP5, TLR2, TLR5, CA5B, and RORA. The Kaplan-Meier model was used to validate the prognostic significance of these genes in the NSCLC cohort. As a result, five MTGs (RPS6KA5, RORA, SH3BP5, NUPR1 and CD40LG) were found to be significantly correlated with a better OS of NSCLC patients (Figure 3B). The further analysis demonstrated the expressions of these five MTGs were all significantly downregulated in tumor tissues as compared with those in normal tissues in NSCLC patients (Figure 3C). Finally, the ROC analysis was used to evaluate their performance in predicting the OS of NSCLC patients (Figure 3D). The result demonstrated SH3BP5 had the best predictive performance with AUC of 0.935, followed by NUPR1 (AUC = 0.890), RORA (AUC = 0.809), CD40LG (AUC = 0.793) and RPS6KA5 (AUC = 0.656).
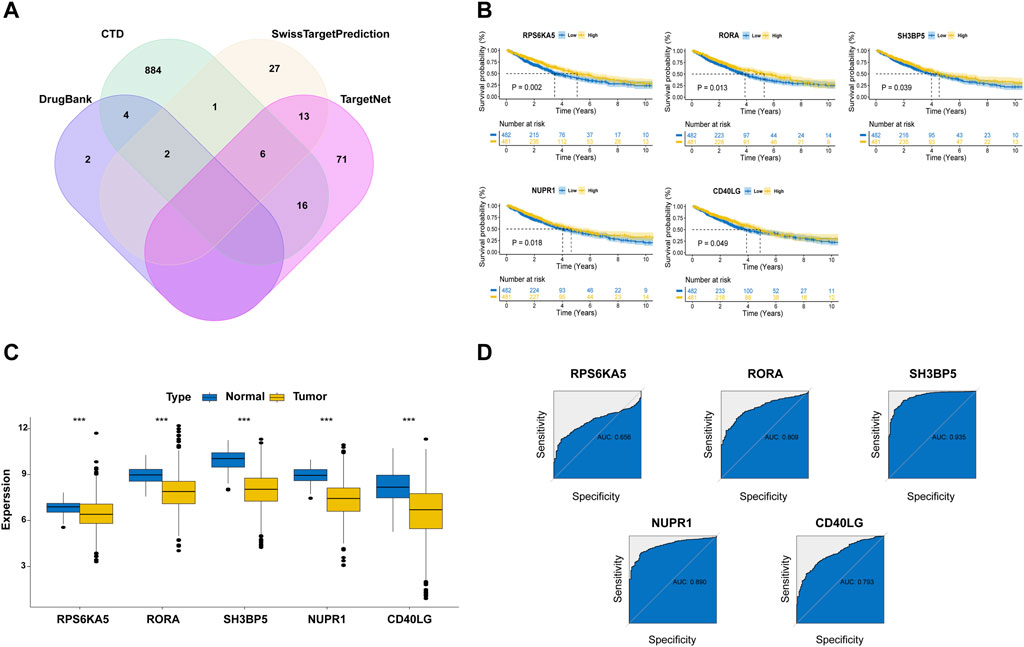
Figure 3. Identification of prognostic metformin target genes (MTGs) in NSCLC patients. (A) Veen plot for the MTGs in the online databases. (B) The survival curves stratified by expressions of five MTGs in NSCLC patients from TCGA cohort. (C) Expressions of five MTGs in the tumor and normal tissues of NSCLC patients from TCGA cohort. (D) Receiver operating characteristic curves for determining the predictive performance of five MTGs in predicting the OS of NSCLC patients from TCGA cohort.
3.5 Correlation of favorable prognostic MTGs with immune infiltration in NSCLC patients
As shown in Figure 4A, the ssGSEA analysis indicated the expressions of four favorable prognostic MTGs (RORA, SH3BP5, NUPR1 and CD40LG) were positively correlated with proportion of most infiltrated immune cells. For example, CD40LG expression was positively correlated with the proportion of activated dendritic cells, B cells, CD8+ T cells, check-point, macrophage, etc. For further investigating the cellular distribution of these MTGs, a NSCLC single-cell dataset was utilized (GSE146100). The distributions of cell types and matched annotations were demonstrated in Figures 4B,C respectively. The relative quantitative analysis for detecting gene expressions in immune cells was then performed and the result was shown in Figure 4D. For example, the expression of RORA was significantly increased in CD4+ T cells, CD8+ T cells and natural killer cells. The expression of NUPR1 was significantly increased in monocytes or macrophages, while that of SH3BP5 was abundant in B cells, natural killer cells and Treg cells. Finally, the cellular localization analysis confirmed the correlation between MTGs expression and immune cells (Figure 4E).
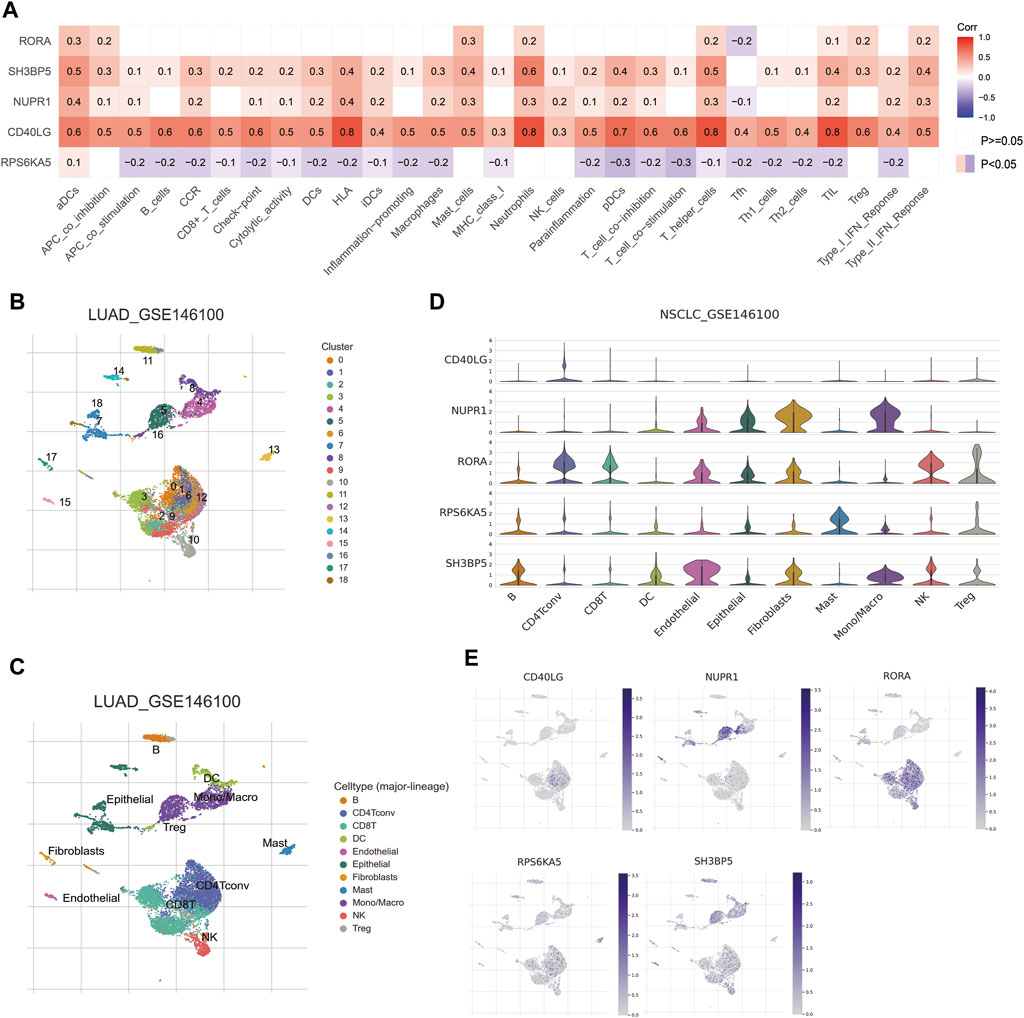
Figure 4. Correlations of prognostic metformin target genes (MTGs) with immune infiltration in NSCLC patients. (A) Correlations of five prognostic MTGs with immune infiltration in NSCLC patients from TCGA cohort. (B and C) Cell distribution (B) and matched annotation (C) of NSCLC patients from GSE146100 cohort. (D) Expression profiles of five prognostic MTGs in various immune cells. (E) Localization of five prognostic MTGs in immune cells.
4 Discussion
Despite encouraging results from biological experiments, the actual efficacy of metformin in combination with anti-cancer therapies is still controversial. In locally advanced NSCLC patients, additional use of metformin resulted in worse clinical outcome and increased toxic events (Tsakiridis et al., 2021). A retrospective analysis found metformin use failed to provide long-term survival benefit in colorectal cancer patients receiving neoadjuvant therapy followed by surgical resection (Sonal et al., 2024). A Phase I/II trial (NCT02949700) is ongoing to identify metformin as a chemo-radiosensitizer for head and neck cancer patients (Kemnade et al., 2023). The preliminary result has demonstrated an improving trend of patient survival in the metformin group, although it failed to reach statistical significance. To our knowledge, a recent meta-analysis including 22 studies has summarized the retrospective studies regarding the role of metformin use in combination with ICI therapy (Shen et al., 2024). The result suggests metformin use is significantly correlated with worse OS (p = 0.004) instead of PFS (p = 0.345) in ICI-treated cancer patients. However, various inherent factors such as patient selection and therapy strategies may result in the heterogeneous results of the meta-analysis. Therefore, more clinical investigations with sufficient sample sizes are urgently needed. In this study, using a multicenter cohort, we found metformin use was significantly correlated with better outcome in ICI-treated lung cancer patients instead of other cancer patients, which may be partly attributed to the role of its target genes in activating immune cells. This finding provides novel evidences for the utilization of metformin as a promising adjuvant drug in cancer immunotherapy.
For the entire cohort, no significant correlation was observed between metformin use and clinical outcome. This finding is consistent with several published retrospective studies (Buti et al., 2021; Gaucher et al., 2021). A recent large-scale multicenter study (n = 1,395) has even found metformin use was associated with increased risk of disease progression and death in ICI-treated patients with advanced solid cancers (Cortellini et al., 2023). The researchers speculated that metformin use may impair the anti-cancer immune system through affecting gut microbiome or immune related cytokines. On the other hand, another multicenter study (n = 878) has demonstrated that concomitant use of metformin was associated with better clinical outcome in ICI-treated cancer patients, while this beneficial effect was not observed in patients who only received metformin before ICI therapy (Chiang et al., 2023). In clinical practice, number prognostic factors vary greatly among different cancers, such as pathological types, therapeutic strategies and immune microenvironment. Therefore, our global analysis may be insufficient to accurately evaluate the correlation between metformin use and ICI efficacy, suggesting the necessity of subgroup analysis.
In our subgroup of lung cancer, the survival analysis demonstrated that metformin use was significantly correlated with better OS and PFS in ICI treated patients. The univariate and multivariate analysis identified metformin use was an independent favorable prognostic factor. These findings collectively supported the beneficial role of metformin in combination with ICI drugs, which was consistent with several published studies. For instance, Afzal et al. found metformin use was correlated with better disease control and response rate in NSCLC patients receiving ICIs as second or third-line therapy (Afzal et al., 2019). Similarly, Yang et al. found the use of metformin with or without dipeptidyl peptidase four inhibitors was correlated with higher objective response rate and longer PFS in metastatic NSCLC patients who received ICI monotherapy (Yang et al., 2023). A published case report demonstrated metformin has the potential to overcome acquired resistance to nivolumab in small cell lung cancer patients (Kim et al., 2021). Some recent mechanism investigations can be used for explaining the beneficial role of metformin use in ICI-treated patients with lung cancer. In lung cancer bearing mice, metformin increased CD8+ T cell infiltration and IFN-γ expression through modulating gut microbiota, contributing to enhanced anti-cancer immunity (Zhao et al., 2024). Metformin was found to promote the formation of memory CD8+ T cells and inhibit their apoptosis, enabling increased tumor-infiltrating CD8+ T cells in lung cancer patients (Zhang et al., 2020). Metformin could even directly decreased the expressions of both PD-1 and PD-L1, creating a favorable microenvironment to prevent tumor immune evasion (Park et al., 2024). It should be noted that two studies failed to demonstrate its beneficial role in ICI-treated lung cancer patients, which may be partly attributed to the potential impact of confounding factors (such as corticosteroids, antibiotics, proton pump inhibitors, etc.) in the multivariate analysis (Svaton et al., 2020; Cortellini et al., 2021).
In the subgroup analysis for esophagus cancer patients, no significant correlation was observed between metformin use and clinical outcome. This result was inconsistent with a mechanism investigation that found metformin improved the immunosuppressive tumor microenvironment in an esophageal spontaneous carcinogenesis rat model (Takei et al., 2022). Although previous studies have confirmed the preventive role of metformin use in esophageal carcinogenesis, relevant clinical evidences for its correlation with ICI drugs are lacking and further efforts are needed (Najafi et al., 2023). With regard to patients with hepatobiliary or pancreatic cancer, the similar result was observed. A recent retrospective study has even found metformin use was associated with worse objective response, median OS and PFS in ICI-treated patients with advanced hepatocellular carcinoma (Kang et al., 2023). This finding was contradictory with a recent comprehensive review that highlighted its role in improving immune microenvironment and regulating expressions of immune genes in hepatocellular carcinoma (Abd El-Fattah and Zakaria, 2022). In pancreatic cancer patients who received gemcitabine-based neoadjuvant chemoradiotherapy, metformin use could reduce pro-tumoral M2 macrophages and increase immune-activating dendritic cells, further supporting its beneficial role in immunotherapy (van Eijck et al., 2024). Considering the great differences between preclinical experiments and clinical studies, more well-designed clinical trials are urgently needed for further validation.
Since we found metformin use was correlated with better clinical outcome in ICI-treated lung cancer patients, we next made efforts to investigate the underlying mechanisms based on the bioinformatics method. As result, we identified five target genes of metformin (RPS6KA5, RORA, SH3BP5, NUPR1, and CD40LG), which were significantly correlated with favorable prognosis and immune infiltration in lung cancer patients. To our knowledge, some recently published studies have correlated these genes with lung cancer. RPS6KA5, as a substrate of MAPK activated protein kinase family, was found to induce humoral immune response and its autoantibody could be used to diagnosis lung cancer (Pei et al., 2020). High RORA expression was proved as an independent favorable factor for OS and correlated with numerous immune checkpoint-related genes such as CD274 and PDCD1LG2 in NSCLC patients (Xian et al., 2022). SH3BP5 was identified as a downstream target of METTL3 that inhibited lung cancer invasion through regulating SH3BP5 mRNA stability in a YTHDF1-dependent manner (Zhang et al., 2024). Metformin upregulated NUPR1 expression in NSCLC cells, while knockdown of NUPR1 induced cell sensitivity to metformin or ionizing radiation (Kim et al., 2022). CD40LG not only could promote the apoptosis of lung cancer cells, but also may be involved in regulating T cell function (Xu et al., 2010; Guo et al., 2023). Although direct clinical evidences are lacking, these MTGs have the potential to be developed as novel clinical biomarkers for ICI-treated lung cancer patients.
Our retrospective study has some inherent limitations. Firstly, the proportion of patients who received metformin was relatively small (76/516, 14.7%), which hampers further subgroup analysis. Therefore, multicenter validations based on larger sample size are essential. Secondly, due to the retrospective nature, numerous heterogeneous factors such as patient selection, cancer type, ICI drug and metformin doses significantly affect the results. For overcoming this limitation, more randomized controlled trials with rigorous design are highly encouraged. Thirdly, we failed to assess the impact of the cumulative effect of metformin doses, duration of DM and other antidiabetic drugs, all of which should be emphasized in our following work. Finally, the bioinformatics method was used to identify prognostic MTGs and clarify their correlations with immune cells, which needs further verification based on clinical samples and biological experiments.
In conclusion, metformin use was significantly correlated with better OS and PFS in ICI-treated lung cancer patients. In addition, five MTGs were identified as prognostic biomarkers for lung cancer patients, which was correlated with infiltration of immune cells. The actual role of metformin and its target genes in cancer immunotherapy still need to be clarified by more work in future.
Data availability statement
The original contributions presented in the study are included in the article/Supplementary Material, further inquiries can be directed to the corresponding authors.
Ethics statement
The studies involving humans were approved by the ethics committee of the Affiliated Hospital of Yangzhou University. The studies were conducted in accordance with the local legislation and institutional requirements. The participants provided their written informed consent to participate in this study.
Author contributions
JW: Data curation, Writing–original draft. JL: Data curation, Formal Analysis, Writing–review and editing. HG: Project administration, Writing–review and editing. WW: Data curation, Resources, Software, Writing–review and editing. JY: Supervision, Validation, Writing–review and editing. JM: Visualization, Writing–review and editing. WF: Data curation, Formal Analysis, Writing–review and editing. HQ: Data curation, Writing–review and editing. YW: Project administration, Writing–review and editing. XY: Funding acquisition, Writing–review and editing. HoG: Validation, Writing–review and editing.
Funding
The author(s) declare that financial support was received for the research, authorship, and/or publication of this article. This research was financially supported by the China National Natural Science Foundation (No. 81902422), Jiangsu Natural Science Foundation (No. BK20231245), Program of Jiangsu Commission of Health (No. M2020024), Program of Yangzhou Commission of Health (No. 2023-2-01), Clinical Translational Foundation of Yangzhou University (No. AHYZUZHXM 202104) and Postgraduate Practice Innovation Program of Jiangsu Province (No. SJCX23_2027).
Conflict of interest
The authors declare that the research was conducted in the absence of any commercial or financial relationships that could be construed as a potential conflict of interest.
Publisher’s note
All claims expressed in this article are solely those of the authors and do not necessarily represent those of their affiliated organizations, or those of the publisher, the editors and the reviewers. Any product that may be evaluated in this article, or claim that may be made by its manufacturer, is not guaranteed or endorsed by the publisher.
Supplementary material
The Supplementary Material for this article can be found online at: https://www.frontiersin.org/articles/10.3389/fphar.2024.1419498/full#supplementary-material
SUPPLEMENTARY FIGURE S1 | Kaplan-Meier curves for the association of metformin use with OS (A) and PFS (B) in solid cancer patients receiving immune checkpoint inhibitors.
SUPPLEMENTARY FIGURE S2 | Prognostic significance of metformin use in the subgroup analysis based on small and non-small cell lung cancer. (A, B) Kaplan-Meier curves for the association of metformin use with OS (A) and PFS (B) in small cell lung cancer patients receiving immune checkpoint inhibitors (ICIs). (C, D) Kaplan-Meier curves for the association of metformin use with OS (C) and PFS (D) in non-small cell lung cancer patients receiving ICIs.
SUPPLEMENTARY FIGURE S3 | Prognostic significance of metformin use in the subgroup analysis based on cancer types. (A, B) Kaplan-Meier curves for the association of metformin use with OS (A) and PFS (B) in esophagus cancer patients receiving ICIs. (C, D) Kaplan-Meier curves for the association of metformin use with OS (C) and PFS (D) in hepatobiliary and pancreatic cancer patients receiving ICIs.
References
Abd El-Fattah, E. E., and Zakaria, A. Y. (2022). Metformin modulate immune fitness in hepatocellular carcinoma: molecular and cellular approach. Int. Immunopharmacol. 109, 108889. doi:10.1016/j.intimp.2022.108889
Afzal, M. Z., Dragnev, K., Sarwar, T., and Shirai, K. (2019). Clinical outcomes in non-small-cell lung cancer patients receiving concurrent metformin and immune checkpoint inhibitors. Lung cancer Manag. 8 (2), LMT11. doi:10.2217/lmt-2018-0016
Bahardoust, M., Mousavi, S., Moezi, Z. D., Yarali, M., Tayebi, A., Olamaeian, F., et al. (2024). Effect of metformin use on survival and recurrence rate of gastric cancer after gastrectomy in diabetic patients: a systematic review and meta-analysis of observational studies. J. Gastrointest. Cancer 55 (1), 65–76. doi:10.1007/s12029-023-00955-y
Bray, F., Laversanne, M., Sung, H., Ferlay, J., Siegel, R. L., Soerjomataram, I., et al. (2024). Global cancer statistics 2022: GLOBOCAN estimates of incidence and mortality worldwide for 36 cancers in 185 countries. CA Cancer J. Clin. 74 (3), 229–263. doi:10.3322/caac.21834
Buti, S., Bersanelli, M., Perrone, F., Tiseo, M., Tucci, M., Adamo, V., et al. (2021). Effect of concomitant medications with immune-modulatory properties on the outcomes of patients with advanced cancer treated with immune checkpoint inhibitors: development and validation of a novel prognostic index. Eur. J. Cancer 142, 18–28. doi:10.1016/j.ejca.2020.09.033
Capella, M. P., Pang, S. A., Magalhaes, M. A., and Esfahani, K. (2024). A review of immunotherapy in non-small-cell lung cancer. Curr. Oncol. 31 (6), 3495–3512. doi:10.3390/curroncol31060258
Chen, J., Chen, S., Luo, H., Long, S., Yang, X., He, W., et al. (2023). The negative effect of concomitant medications on immunotherapy in non-small cell lung cancer: an umbrella review. Int. Immunopharmacol. 124 (Pt B), 110919. doi:10.1016/j.intimp.2023.110919
Chiang, C. H., Chen, Y. J., Chiang, C. H., Chen, C. Y., Chang, Y. C., Wang, S. S., et al. (2023). Effect of metformin on outcomes of patients treated with immune checkpoint inhibitors: a retrospective cohort study. Cancer Immunol. Immunother. 72 (6), 1951–1956. doi:10.1007/s00262-022-03363-6
Cortellini, A., D'Alessio, A., Cleary, S., Buti, S., Bersanelli, M., Bordi, P., et al. (2023). Type 2 diabetes mellitus and efficacy outcomes from immune checkpoint blockade in patients with cancer. Clin. Cancer Res. 29 (14), 2714–2724. doi:10.1158/1078-0432.CCR-22-3116
Cortellini, A., Di Maio, M., Nigro, O., Leonetti, A., Cortinovis, D. L., Aerts, JGJV, et al. (2021). Differential influence of antibiotic therapy and other medications on oncological outcomes of patients with non-small cell lung cancer treated with first-line pembrolizumab versus cytotoxic chemotherapy. J. Immunother. cancer 9 (4), e002421. doi:10.1136/jitc-2021-002421
Daina, A., Michielin, O., and Zoete, V. (2019). SwissTargetPrediction: updated data and new features for efficient prediction of protein targets of small molecules. Nucleic acids Res. 47 (W1), W357–W364. doi:10.1093/nar/gkz382
Davis, A. P., Wiegers, T. C., Johnson, R. J., Sciaky, D., Wiegers, J., and Mattingly, C. J. (2023). Comparative toxicogenomics database (CTD): update 2023. Nucleic acids Res. 51 (D1), D1257–D1262. doi:10.1093/nar/gkac833
Emens, L. A., Romero, P. J., Anderson, A. C., Bruno, T. C., Capitini, C. M., Collyar, D., et al. (2024). Challenges and opportunities in cancer immunotherapy: a Society for Immunotherapy of Cancer (SITC) strategic vision. J. Immunother. Cancer 12 (6), e009063. doi:10.1136/jitc-2024-009063
Gaucher, L., Adda, L., Séjourné, A., Joachim, C., Guillaume, C., Poulet, C., et al. (2021). Associations between dysbiosis-inducing drugs, overall survival and tumor response in patients treated with immune checkpoint inhibitors. Ther. Adv. Med. Oncol. 13, 17588359211000591. doi:10.1177/17588359211000591
Guo, H., Li, Y., Lin, J., Li, D., Yang, J., Wang, J., et al. (2024). A novel investigation into the negative impact of opioid use on the efficacy of immune checkpoint inhibitors in advanced non-small cell lung cancer patients. Int. Immunopharmacol. 129, 111611. doi:10.1016/j.intimp.2024.111611
Guo, Z., Qi, X., Li, Z., Yang, J., Sun, Z., Li, P., et al. (2023). Development and validation of an immune-related gene signature for prognosis in Lung adenocarcinoma. IET Syst. Biol. 17 (1), 27–38. doi:10.1049/syb2.12057
Holder, A. M., Dedeilia, A., Sierra-Davidson, K., Cohen, S., Liu, D., Parikh, A., et al. (2024). Defining clinically useful biomarkers of immune checkpoint inhibitors in solid tumours. Nat. Rev. Cancer 24 (7), 498–512. doi:10.1038/s41568-024-00705-7
Jama, M., Tabana, Y., and Barakat, K. H. (2024). Targeting cytotoxic lymphocyte antigen 4 (CTLA-4) in breast cancer. Eur. J. Med. Res. 29 (1), 353. doi:10.1186/s40001-024-01901-9
Júnior, A. D. C., Bragagnoli, A. C., Costa, F. O., and Carvalheira, J. B. C. (2021). Repurposing metformin for the treatment of gastrointestinal cancer. World J. gastroenterology 27 (17), 1883–1904. doi:10.3748/wjg.v27.i17.1883
Kang, S., Khalil, L., McCook-Veal, A., Liu, Y., Galvin, J., Draper, A., et al. (2023). Impact of metformin on clinical outcomes in advanced hepatocellular carcinoma treated with immune checkpoint inhibitors. Liver Cancer Int. 4 (2), 77–88. doi:10.1002/lci2.71
Kemnade, J. O., Florez, M., Sabichi, A., Zhang, J., Jhaveri, P., Chen, G., et al. (2023). Phase I/II trial of metformin as a chemo-radiosensitizer in a head and neck cancer patient population. Oral Oncol. 145, 106536. doi:10.1016/j.oraloncology.2023.106536
Kim, Y., Vagia, E., Viveiros, P., Kang, C. Y., Lee, J. Y., Gim, G., et al. (2021). Overcoming acquired resistance to PD-1 inhibitor with the addition of metformin in small cell lung cancer (SCLC). Cancer Immunol. Immunother. 70 (4), 961–965. doi:10.1007/s00262-020-02703-8
Kim, Y. J., Hong, S.-E., Jang, S.-K., Park, K. S., Kim, C.-H., Park, I.-C., et al. (2022). Knockdown of NUPR1 enhances the sensitivity of non-small-cell lung cancer cells to metformin by AKT inhibition. Anticancer Res. 42 (7), 3475–3481. doi:10.21873/anticanres.15834
Knox, C., Wilson, M., Klinger, C. M., Franklin, M., Oler, E., Wilson, A., et al. (2024). DrugBank 6.0: the DrugBank knowledgebase for 2024. Nucleic acids Res. 52 (D1), D1265–D1275. doi:10.1093/nar/gkad976
Linkeviciute-Ulinskiene, D., Dulskas, A., Patasius, A., Zabuliene, L., Urbonas, V., and Smailyte, G. (2020). Response to letter to the editor: metformin in colorectal cancer: a match ruled by MiR26b? Cancer Epidemiol. 64, 101626. doi:10.1016/j.canep.2019.101626
Liu, C., Guo, H., Mao, H., Tong, J., Yang, M., and Yan, X. (2022). An up-to-date investigation into the correlation between proton pump inhibitor use and the clinical efficacy of immune checkpoint inhibitors in advanced solid cancers: a systematic review and meta-analysis. Front. Oncol. 12, 753234. doi:10.3389/fonc.2022.753234
Najafi, F., Rajati, F., Sarokhani, D., Bavandpour, M., and Moradinazar, M. (2023). The Relationship between metformin consumption and cancer risk: an updated umbrella review of systematic reviews and meta-analyses. Int. J. Prev. Med. 14, 90. doi:10.4103/ijpvm.ijpvm_62_21
O’connor, L., Bailey-Whyte, M., Bhattacharya, M., Butera, G., Hardell, K. N. L., Seidenberg, A. B., et al. (2024). Association of metformin use and cancer incidence: a systematic review and meta-analysis. JNCI J. Natl. Cancer Inst. 116 (4), 518–529. doi:10.1093/jnci/djae021
Panaampon, J., Zhou, Y., and Saengboonmee, C. (2023). Metformin as a booster of cancer immunotherapy. Int. Immunopharmacol. 121, 110528. doi:10.1016/j.intimp.2023.110528
Park, S. H., Lee, J., Yun, H. J., Kim, S.-H., and Lee, J.-H. (2024). Metformin suppresses both PD-L1 expression in cancer cells and cancer-induced PD-1 expression in immune cells to promote antitumor immunity. Ann. Laboratory Med. 44 (5), 426–436. doi:10.3343/alm.2023.0443
Pei, L., Liu, H., Ouyang, S., Zhao, C., Liu, M., Wang, T., et al. (2020). Discovering novel lung cancer associated antigens and the utilization of their autoantibodies in detection of lung cancer. Immunobiology 225 (2), 151891. doi:10.1016/j.imbio.2019.11.026
Shen, J., Ye, X., Hou, H., and Wang, Y. (2024). Clinical evidence for the prognostic impact of metformin in cancer patients treated with immune checkpoint inhibitors. Int. Immunopharmacol. 134, 112243. doi:10.1016/j.intimp.2024.112243
Sonal, S., Boudreau, C., Kunitake, H., Goldstone, R. N., Lee, G. C., Cauley, C. E., et al. (2024). Metformin does not affect outcomes in patients with locally advanced rectal cancer treated with neoadjuvant therapy and resection. Am. Surgeon 90 (4), 858–865. doi:10.1177/00031348231198106
Svaton, M., Zemanova, M., Zemanova, P., Kultan, J., Fischer, O., Skrickova, J., et al. (2020). Impact of concomitant medication administered at the time of initiation of nivolumab therapy on outcome in non-small cell lung cancer. Anticancer Res. 40 (4), 2209–2217. doi:10.21873/anticanres.14182
Takei, R., Miyashita, T., Takada, S., Tajima, H., Ninomiya, I., Takamura, H., et al. (2022). Dynamic switch of immunity and antitumor effects of metformin in rat spontaneous esophageal carcinogenesis. Cancer Immunol. Immunother. 71 (4), 777–789. doi:10.1007/s00262-021-03027-x
Tsakiridis, T., Pond, G. R., Wright, J., Ellis, P. M., Ahmed, N., Abdulkarim, B., et al. (2021). Metformin in combination with chemoradiotherapy in locally advanced non–small cell lung cancer: the OCOG-ALMERA randomized clinical trial. JAMA Oncol. 7 (9), 1333–1341. doi:10.1001/jamaoncol.2021.2328
Urbonas, V., Rutenberge, J., Patasius, A., Dulskas, A., Burokiene, N., and Smailyte, G. (2020). The impact of metformin on survival in patients with melanoma—national cohort study. Ann. Epidemiol. 52, 23–25. doi:10.1016/j.annepidem.2020.09.010
van Eijck, C. W. F., Vadgama, D., van Eijck, C. H. J., Wilmink, J. W., Dutch Pancreatic Cancer Group DPCG, , van der Harst, E., et al. (2024). Metformin boosts antitumor immunity and improves prognosis in upfront resected pancreatic cancer: an observational study. JNCI J. Natl. Cancer Inst., djae070. doi:10.1093/jnci/djae070
Wang, J., Guo, H., Yang, J., Mao, J., Wang, Y., Yan, X., et al. (2024). Identification of C-PLAN index as a novel prognostic predictor for advanced lung cancer patients receiving immune checkpoint inhibitors. Front. Oncol. 14, 1339729. doi:10.3389/fonc.2024.1339729
Wang, Y., Yang, M., Tao, M., Liu, P., Kong, C., Li, H., et al. (2021). Corticosteroid administration for cancer-related indications is an unfavorable prognostic factor in solid cancer patients receiving immune checkpoint inhibitor treatment. Int. Immunopharmacol. 99, 108031. doi:10.1016/j.intimp.2021.108031
Xian, H., Li, Y., Zou, B., Chen, Y., Yin, H., Li, X., et al. (2022). Identification of TIMELESS and RORA as key clock molecules of non-small cell lung cancer and the comprehensive analysis. BMC cancer 22 (1), 107. doi:10.1186/s12885-022-09203-1
Xu, W., Li, Y., Wang, X., Wang, C., Zhao, W., and Wu, J. (2010). Anti-tumor activity of gene transfer of the membrane-stable CD40L mutant into lung cancer cells. Int. J. Oncol. 37 (4), 935–941. doi:10.3892/ijo_00000744
Yan, X., Liu, P., Li, D., Hu, R., Tao, M., Zhu, S., et al. (2022). Novel evidence for the prognostic impact of β-blockers in solid cancer patients receiving immune checkpoint inhibitors. Int. Immunopharmacol. 113 (Pt A), 109383. doi:10.1016/j.intimp.2022.109383
Yan, X., Wang, J., Mao, J., Wang, Y., Wang, X., Yang, M., et al. (2023). Identification of prognostic nutritional index as a reliable prognostic indicator for advanced lung cancer patients receiving immune checkpoint inhibitors. Front. Nutr. 10, 1213255. doi:10.3389/fnut.2023.1213255
Yang, J., Kim, S. H., Jung, E. H., Kim, S. A., Suh, K. J., Lee, J. Y., et al. (2023). The effect of metformin or dipeptidyl peptidase 4 inhibitors on clinical outcomes in metastatic non-small cell lung cancer treated with immune checkpoint inhibitors. Thorac. Cancer 14 (1), 52–60. doi:10.1111/1759-7714.14711
Yang, M., Wang, Y., Yuan, M., Tao, M., Kong, C., Li, H., et al. (2020). Antibiotic administration shortly before or after immunotherapy initiation is correlated with poor prognosis in solid cancer patients: an up-to-date systematic review and meta-analysis. Int. Immunopharmacol. 88, 106876. doi:10.1016/j.intimp.2020.106876
Yao, Z.-J., Dong, J., Che, Y.-J., Zhu, M.-F., Wen, M., Wang, N.-N., et al. (2016). TargetNet: a web service for predicting potential drug–target interaction profiling via multi-target SAR models. J. computer-aided Mol. Des. 30 (5), 413–424. doi:10.1007/s10822-016-9915-2
Zhang, B., Qian, R., and Li, X. (2024). METTL3 suppresses invasion of lung cancer via SH3BP5 m6A modification. Archives Biochem. Biophysics 752, 109876. doi:10.1016/j.abb.2023.109876
Zhang, Z., Li, F., Tian, Y., Cao, L., Gao, Q., Zhang, C., et al. (2020). Metformin enhances the antitumor activity of CD8+ T lymphocytes via the AMPK–miR-107–Eomes–PD-1 pathway. J. Immunol. 204 (9), 2575–2588. doi:10.4049/jimmunol.1901213
Keywords: immune checkpoint inhibitor, cancer, metformin, prognosis, biomarker
Citation: Wang J, Lin J, Guo H, Wu W, Yang J, Mao J, Fan W, Qiao H, Wang Y, Yan X and Guo H (2024) Prognostic impact of metformin in solid cancer patients receiving immune checkpoint inhibitors: novel evidences from a multicenter retrospective study. Front. Pharmacol. 15:1419498. doi: 10.3389/fphar.2024.1419498
Received: 18 April 2024; Accepted: 15 July 2024;
Published: 29 July 2024.
Edited by:
Olesya A. Kharenko, Syantra Inc., CanadaReviewed by:
Duoyi Zhao, Fourth Affiliated Hospital of China Medical University, ChinaAudrius Dulskas, National Cancer Institute, Lithuania
Copyright © 2024 Wang, Lin, Guo, Wu, Yang, Mao, Fan, Qiao, Wang, Yan and Guo. This is an open-access article distributed under the terms of the Creative Commons Attribution License (CC BY). The use, distribution or reproduction in other forums is permitted, provided the original author(s) and the copyright owner(s) are credited and that the original publication in this journal is cited, in accordance with accepted academic practice. No use, distribution or reproduction is permitted which does not comply with these terms.
*Correspondence: Ying Wang, WYingzl@163.com; Xuebing Yan, yyxxbb8904@163.com; Hong Guo, guohong7666@163.com
†These authors have contributed equally to this work