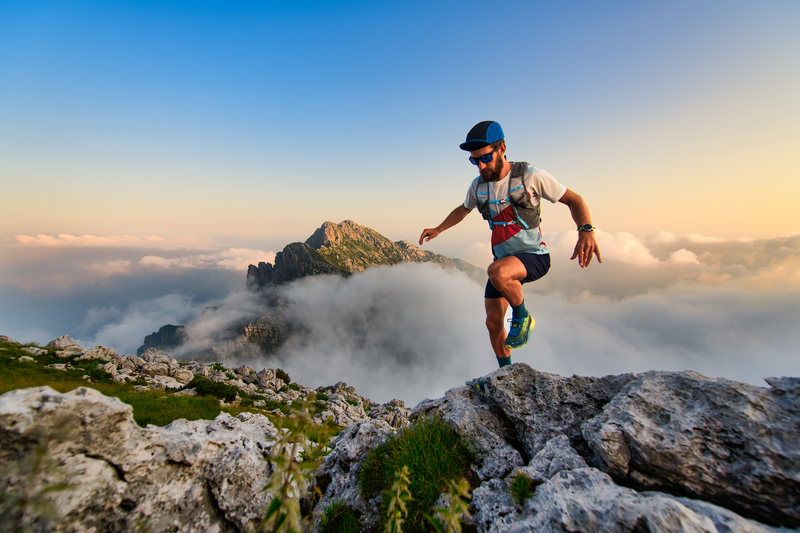
95% of researchers rate our articles as excellent or good
Learn more about the work of our research integrity team to safeguard the quality of each article we publish.
Find out more
MINI REVIEW article
Front. Pharmacol. , 18 July 2024
Sec. Experimental Pharmacology and Drug Discovery
Volume 15 - 2024 | https://doi.org/10.3389/fphar.2024.1419110
This article is part of the Research Topic Network Polypharmacology of ATP-binding Cassette (ABC) and Solute Carrier (SLC) Transporters View all 7 articles
Medicinal polypharmacology is one answer to the complex reality of multifactorial human diseases that are often unresponsive to single-targeted treatment. It is an admittance that intrinsic feedback mechanisms, crosstalk, and disease networks necessitate drugs with broad modes-of-action and multitarget affinities. Medicinal polypharmacology grew to be an independent research field within the last two decades and stretches from basic drug development to clinical research. It has developed its own terminology embedded in general terms of pharmaceutical drug discovery and development at the intersection of medicinal chemistry, chemical biology, and clinical pharmacology. A clear and precise language of critical terms and a thorough understanding of underlying concepts is imperative; however, no comprehensive work exists to this date that could support researchers in this and adjacent research fields. In order to explore novel options, establish interdisciplinary collaborations, and generate high-quality research outputs, the present work provides a first-in-field glossary to clarify the numerous terms that have originated from various individual disciplines.
Drug development has evolved over the last decades and is increasingly acknowledging integral approaches to achieve clinical effectiveness of drugs, specifically taking target combinations into account (Morphy and Rankovic, 2007; Zimmermann et al., 2007; Proschak et al., 2019). Although the majority of academic research and industrial efforts still adhere to the Specificity paradigm (One drug-one target philosophy) (Morphy and Rankovic, 2007; Jalencas and Mestres, 2013a; Anighoro et al., 2014), the general perception of human pathology is changing to a more integral point of view acknowledging the existence of complex coherences in malignant, metabolic, or neurological diseases that may be engaged simultaneously (Morphy and Rankovic, 2007; Anighoro et al., 2014; Proschak et al., 2019) to overcome ineffective pharmacotherapy (Anighoro et al., 2014; Proschak et al., 2019).
This vastly growing research field, which we designated as “medicinal polypharmacology” (Stefan and Rafehi, 2023; Rafehi et al., 2024), expands into various individual research fields, allowing for novel concepts to emerge.
(i) The clinically observed effects of drugs are generally a result of multiple individual interactions with multiple interaction partners (Paolini et al., 2006; Vulpetti et al., 2012; Jalencas and Mestres, 2013b; Anighoro et al., 2014; Schmidt et al., 2014);
(ii) Prevalent human diseases are often multifactorial with far-reaching disease networks that result in feedback, crosstalk, and, subsequently, therapy resistance (Morphy and Rankovic, 2007; Azmi and Mohammad, 2014; Keith et al., 2005);
(iii) Multitargeticity is an inherent character of small molecules that has molecular-structural limits (Paolini et al., 2006; Hu and Bajorath, 2010; Jalencas and Mestres, 2013a; Anighoro et al., 2014; Namasivayam et al., 2022a);
(iv) Phylogenetically distant proteins have common and reoccurring structural motifs [Superfolds (Orengo et al., 1994; Russell et al., 1998; Grishin, 2001; Koch, 2011)] which can form Supersites that bind related, multitarget ligands (Russell et al., 1998; Namasivayam et al., 2021a);
(v) The multitarget inheritance of multitarget drugs allows for superior clinical effectiveness and, in parallel, exploration of yet undruggable targets of the future [Privileged ligands (Jalencas and Mestres, 2013a; Kim et al., 2014) and Target repurposing (Paolini et al., 2006; Pollastri and Campbell, 2011; Klug et al., 2016; Singh et al., 2020)].
Medicinal polypharmacology is an attractive multidisciplinary research field that bears a certain linguistic complexity. Multitarget paradigm, Supersites, Privileged ligands, and Target repurposing—these terms are nothing but words unless an interconnected explanation is provided. In order to discuss medicinal polypharmacology and the individual aspects involved, an understanding of the terminology and developed concepts is necessary. This is particularly true with respect to how these terms and concepts are embedded in the broader context of medicinal chemistry, chemical biology, and clinical pharmacology.
Instances in the past have demonstrated that incorrect terminologies can lead to misleading interpretations in medical life sciences. These include, for example, the use of incorrect terms and units (Zavorsky, 2021), incorrect physiological and anatomical descriptions (Franz-Odendaal, 2023), or erroneous histopathological interpretations that can lead to false diagnoses (Brunye et al., 2023). These and other instances have resulted in article corrections (Judge et al., 2020), commentaries and letters to the editor (Zavorsky, 2021), entire review articles addressing specific incorrect terminology (Tao et al., 2023), and subject-specific online glossaries. Although it is undoubtedly important to clarify misconceptions, it would even be better to avoid them altogether. Clear and precise language is important for communication, discussion, and awareness, which prompted us to provide a first-in-field glossary of terms and concepts used in medicinal polypharmacology. This glossary takes the pioneering and contemporary literature, the standardized nomenclature as outlined by IUPAC (Proudfoot et al., 2011) and IUPHAR, as well as definitions from medical subject headings (MeSH; https://www.ncbi.nlm.nih.gov/mesh) into account:
An Off-target effect that leads to severe adverse events and/or toxicity (McKie, 2016; Ravikumar and Aittokallio, 2018; Polanski and Bak, 2019).
Chemical and biological space toward which (computer-driven) predictions are feasible and reliable based on a training set of ligands/small-molecule drugs; in polypharmacology, the multiplicity of data (origin) and controversial/contradicting data (e.g., Promiscuity cliffs) as well as publication bias and data pollution hamper the prediction capabilities and limit the applicability domain of computational prediction methodologies (Taula et al., 2009; Stefan and Rafehi, 2024).
Experimental verification that the measured output (readout) of an assay is in association with, and correlates to, the biological activity of the anticipated target (consistent reflection); assay validation is a valuable part of a Target validation and subsequent Lead identification processes at the very beginning of the drug development pipeline (Proudfoot et al., 2011).
Compound that interferes with assay readouts and causes artifacts and, thus, leads to its identification as a false-positive hit (Stork and Kirchmair, 2018).
Modulating the biological activity of a polypharmacological ligand/small-molecule drug against two or more targets to achieve an optimal ratio of activities, and thus, a preferable polypharmacological profile; optimized polypharmacological ligands/small-molecule drugs are also referred to as “balanced”; balancing is achieved by Designing-in drug design and Designing-out drug design (Morphy et al., 2004; Morphy and Rankovic, 2006; Korcsmaros et al., 2007; Morphy and Rankovic, 2007; Morphy and Rankovic, 2009; Zhan and Liu, 2009; Peters, 2013; Proschak et al., 2019).
see Space.
Networks of genes, the cytoskeleton, the metabolism, organelles, proteins, and signaling connected by bridges, hubs, links, and critical nodes offering potential druggable binding sites; also referred to as Interactome; cellular networks are studied in the discipline of Network biology (Barabasi and Oltvai, 2004; Hopkins et al., 2006; Korcsmaros et al., 2007; Morphy and Rankovic, 2009; McKie, 2016).
see Promiscuity.
see Space.
Ligand/small-molecule drug designed or developed to modulate and analyze the function of the target(s) of interest; chemical tools are important factors in the Target validation process at the very beginning of the drug development pipeline (Hopkins et al., 2006).
Computer-aided data mining and acquisition to comprehend and address current problems in chemistry; cheminformatics is strongly related to Chemometrics.
see Space.
The property of structurally, functionally, and/or phylogenetically distant proteins to interact with the same chemical fragments/entities/scaffolds; related to Supersites (Russell et al., 1998; Jalencas and Mestres, 2013b; Anighoro et al., 2014).
Chemical discipline to extract and/or maximize chemical information and knowledge in chemical systems by (computer-driven) mathematical and statistical methodologies; chemometrics is strongly related to Cheminformatics and overlaps with the discipline of Systems chemistry; chemometrics is used, for example, for Quantitative structure-activity relationships (QSARs) (Proudfoot et al., 2011; Joshi, 2023).
Chemical class/structure/scaffold; Virtual screening and High-throughput screening (HTS) anticipate the discovery of molecular-structurally and/or functionally novel chemotypes, particularly in medicinal polypharmacology; chemotypes are also referred to as “topologically equivalent scaffolds” that result in the same carbon skeleton (Hopkins et al., 2006; Zhan and Liu, 2009; Anighoro et al., 2014; Proschak et al., 2019).
Organic synthesis approach to generate a virtually unlimited number of novel, drug-like molecules out of a limited set of starting materials.
see Polypharmacy (Kim et al., 2014).
Two or more targets share a defined number of ligands/small-molecule drugs; these Privileged ligands provide the basis for novel drug Target identification and Target validation; CBTRs are the structure-based counterpart of Structure–activity relationships (SARs) (Bajorath, 2021).
A computational fragment-based methodology for 1) the extraction of Multitarget fragments and, subsequently, Multitarget fingerprints from a set of compounds that were functionally evaluated against two or more targets, and 2) the application of these Multitarget fragments and Multitarget fingerprints against chemical space to predict polypharmacological ligands; also referred to as Computer-aided pattern scoring (C@PS) (Namasivayam et al., 2021a; Namasivayam et al., 2021b; Namasivayam et al., 2022b; Stefan et al., 2022; Stefan et al., 2023; Stefan et al., 2024).
see Computer-aided pattern analysis (C@PA).
The approach to uncover Target constellations (Wang and Yang, 2022).
Ability of two or more phylogenetically distant targets to share the same set of ligands/small-molecule drugs; also referred to as Distant polypharmacology (Jalencas and Mestres, 2013a; Jalencas and Mestres, 2013b; Anighoro et al., 2014; Antolin and Mestres, 2015).
see Unselectivity.
see High-throughput screening (HTS).
A group of compounds that has extensively been evaluated without notable biological activity and which may lead to hit molecules toward yet unknown or barely established novel drug targets by exceptional, unexpected, and/or unprecedented bioactivity, modes-of-action, and Specificity (Wassermann et al., 2015).
An approach to explore Orphan targets (Franchini and Orlandi, 2023).
see Designing-out drug design.
Ligand/small-molecule drug designed to address multiple targets (Morphy and Rankovic, 2005; Morphy and Rankovic, 2009).
Knowledge-based (Framework combination) design of a novel molecular-structural entity out of two or more selective (and potent) ligands/small-molecule drugs against one or more independent targets to obtain simultaneous biological activity against all targets of interest within one molecule; also referred to as Design-in approach (Morphy et al., 2004; Zhan and Liu, 2009; Proschak et al., 2019).
Design of a novel molecular-structural entity without undesired biological activity from a template molecule that has both the desired biological activity against one or more targets as well as undesired biological effects; also referred to as Design-out approach (Morphy and Rankovic, 2009; Zhan and Liu, 2009; Proschak et al., 2019).
see Orphan target.
A drug that exerts multiple Off-target effect, potentially leading to adverse events; like Promiscuity, the term Dirty drug has a negative connotation (Azmi and Mohammad, 2014).
Disease-related Interactome (Wang and Yang, 2022).
The polypharmacological nature of a ligand/small-molecule drug against two or more phylogenetically distant targets (Jalencas and Mestres, 2013b; Antolin and Mestres, 2015).
The accessibility of a target by ligands/small-molecule drugs (Vulpetti et al., 2012).
see Drug profiling.
see Polypharmacy.
Preferable molecular-structural and physicochemical properties originally defined by Lipinski et al. (2001); also referred to as Lipinski-rule-of-five. According to the authors’ original definition, developed drugs should anticipate a calculated octanol-water partition coefficient (CLogP) ≤ 5, a molecular weight (MW) ≤ 500 g mol−1, not more than five hydrogen-(H)-bond donors, and not more than 10 H-bond acceptors; molecular-structural and physicochemical properties are particularly important in the design of polypharmacological ligands/small-molecule drugs (Lipinski et al., 2001; Namasivayam et al., 2022a).
Annotation of a drug (Drug annotation) to physical, chemical, and/or biological attributes; in the drug development pipeline, drug profiling is used to uncover unknown and/or unwanted Off-target effects; many automated design tools exist to predict and map polypharmacological profiles (Besnard et al., 2012; Bowes et al., 2012; Schmidt et al., 2014; Ravikumar and Aittokallio, 2018; Popovic et al., 2019).
see Drug repurposing.
see Drug repurposing.
see Drug repurposing.
see Drug repurposing.
Deliberate and methodical exploration of novel therapeutic purposes and/or indications for existing/approved drugs; also referred to as Drug repositioning, Drug reprofiling, Drug redirecting, Drug retasking, Drug rediscovering, or Rescue of drugs (Peters, 2013; Anighoro et al., 2014; Azmi and Mohammad, 2014; McKie, 2016; Santos et al., 2016; Bajorath, 2022; Wang and Yang, 2022).
see Drug repurposing.
Drug that constitutes the first member of a chemical or pharmacological class of bioactive agents (Kim et al., 2014).
Knowledge-based approach for designing polypharmacological ligands/small-molecule drugs based on the combination of molecular-structural frameworks and pharmacophores of selective (and potent) molecules toward the targets of interest; also referred to as Designing-in drug design or Design-in approach (Morphy et al., 2004; Morphy and Rankovic, 2006; Morphy and Rankovic, 2007; Morphy and Rankovic, 2009; Zhan and Liu, 2009; Proschak et al., 2019).
Compound with a higher-than-expected hit rate in various assays (Hopkins et al., 2006; Jalencas and Mestres, 2013a; Stork and Kirchmair, 2018; Wang and Yang, 2022).
see Promiscuity.
see Phenotypic screening.
Large-scale (random) screening of preferably multiple (and diverse) analogous/physical compound libraries in a short time frame; in Polypharmacology, these screenings are carried out against multiple targets (Cross-screening) in order to identify molecular-structurally (and/or functionally) novel hit molecules (Chemotypes) with polypharmacological profiles; HTS is based on Serendipity (Morphy et al., 2004; Morphy and Rankovic, 2006; Morphy and Rankovic, 2009; Zhan and Liu, 2009; Proudfoot et al., 2011; Ravikumar and Aittokallio, 2018).
The physical molecular interactions between ligands and targets, including associated proteins and/or genes, as well as biochemical cascades, crosstalk, and feedback; also referred to as Cellular network; the interactome is studied in the discipline of Network biology (Barabasi and Oltvai, 2004; Hopkins et al., 2006; Korcsmaros et al., 2007; Morphy and Rankovic, 2009; McKie, 2016).
Defined and charted inventory of ligands/small-molecule drugs, partial structures and functional groups, targets, and/or bioactivities (Anighoro et al., 2014; Namasivayam et al., 2022b; Stefan et al., 2022; Wu et al., 2022; Puri et al., 2023).
Identification of a ligand for (a) particular target(s) of interest that fulfills the requirements for the subsequent drug development stage of Lead optimization; lead molecules are usually discovered via High-throughput screening (HTS) (Proudfoot et al., 2011).
Improvement of the biological activities and other relevant properties (e.g., safety profile, drug likeness, etc.) of a lead molecule with medicinal chemistry methodologies; in Polypharmacology, lead optimization is achieved by Balancing, Designing-in drug design, and Designing-out drug design (Proudfoot et al., 2011):
Exploration of the extended pharmacological profile of a lead compound of the early drug development stage initially designed/discovered to target one particular target (class) only (Klug et al., 2016; Singh et al., 2020).
Experimental setup using immobilized targets of interest to identify novel binding partners by, for example, mass spectrometry, quantitative proteomics, or microarrays; ligand fishing is an integral part of the drug development pipeline and can be seen as reciprocal to Target fishing, in vitro (Chen et al., 2020).
Computer-aided extraction of information about descriptors and fingerprints present in a set of ligands/small-molecule drugs with demonstrated interaction with, or biological activity against, the target(s) of interest; ligand-based drug design is supported by Cheminformatics (Proudfoot et al., 2011; Proschak et al., 2019).
see Drug likeness.
Obsolete synonym for a highly potent, specific, and selective ligand/small-molecule drug that interacts with one defined target only; the opposite of Magic shotgun (Morphy et al., 2004; Terstappen et al., 2007; Anighoro et al., 2014; Bansal and Silakari, 2014; Ravikumar and Aittokallio, 2018).
Obsolete synonym for a polypharmacological ligand/small-molecule drug; the opposite of Magic bullet (Ravikumar and Aittokallio, 2018).
see Promiscuity cliffs.
Target-independent screening methodology based on cell painting assays using various dyes to image the morphological size, shape, and texture of cellular and organellar components with high-throughput microscopy under exposure of ligands/small-molecule drug candidates; morphological profiling provides spectral fingerprints that may be compared to reference compounds for the identification of similar (or distinctive) targets and/or modes-of-action (Bray et al., 2016; Schneidewind et al., 2020).
see Polypharmacy.
Hybridization of individual ligands/small-molecule drugs to a hybrid drug (“conjugated”) or chimeric drug (“fused”) (Bansal and Silakari, 2014).
Multiple monomeric ligands (individual ligands/small-molecule drugs) attached to a single backbone (small-)molecule; very close to the definition of a conjugate (see Polypharmacology) (Handl et al., 2004).
The ability of a ligand/small-molecule drug to address more than one target; also referred to as Multitargeting; it is commonly accepted that multitargeticity is given when targets of different, phylogenetically distant target (super)families are addressed (Stefan, 2019).
see Multitargeticity.
A sum of measured properties and/or mathematically calculated descriptors that conserve chemical, molecular-structural, and/or physicochemical attributes of molecules/partial structures/functional groups that are associated with biological effects toward multiple targets; ultimately distinguished from single-target descriptors/fingerprints (Polanski and Bak, 2019).
see Multitarget paradigm.
Molecular-structural attribute of partial structures/functional groups that are associated with biological effects toward multiple targets (Brunst et al., 2021).
The concept that a ligand/small-molecule drug interacts with multiple targets to exert its biological effect(s); also referred to as the Multitarget drug (MTD) concept (Medina-Franco et al., 2013; Santos et al., 2016; Ravikumar and Aittokallio, 2018; Wang and Yang, 2022).
Discipline addressing the biological organization of cellular components, such as protein–protein interactions, metabolic, signaling, and transcription regulation, as well as Cellular networks; also covered by the term Interactome (Barabasi and Oltvai, 2004; Hopkins et al., 2006; Morphy and Rankovic, 2009; McKie, 2016).
Pharmacological intervention with a small-molecule drug under consideration and understanding of the relevant Cellular network and Interactome; network pharmacology also anticipates the prediction, discovery, and identification of optimal target combinations; also referred to as Systems pharmacology (Hopkins, 2008; Proschak et al., 2019; Wang and Yang, 2022).
see Unselectivity.
see Unspecific effect.
Low-quality compound that interferes with assay readouts, consumes precious scientific resources, and leads to publication bias (Stork and Kirchmair, 2018; Dahlin et al., 2021).
Unintended effect of a ligand/small-molecule drug on a target irrelevant to the respective disease; off-target effects are believed to be a major reason for (secondary) adverse events (also called Side effects) and toxicity (Hopkins et al., 2006; Morphy and Rankovic, 2006; Morphy and Rankovic, 2007; Bowes et al., 2012; Vulpetti et al., 2012; Peters, 2013; Schmidt et al., 2014; McKie, 2016; Polanski and Bak, 2019; Proschak et al., 2019; Wang and Yang, 2022).
Intended effect of a ligand/small-molecule drug on an anticipated drug target; polypharmacology anticipates multiple on-targets (Carragher et al., 2012; Wang and Yang, 2022).
see Specificity paradigm.
see Specificity paradigm.
see Specificity paradigm.
Undruggable target for which no ligands/small-molecule drugs are available; also referred to as Difficult-to-drug target or Yet-to-be-drugged target (Wassermann et al., 2009; Coleman and Rodon, 2021; Franchini and Orlandi, 2023).
Pan-assay interference compounds that result in false-positive hits in various assays; PAINS are, to a certain extent, predictable (Ravikumar and Aittokallio, 2018; Stork and Kirchmair, 2018; Wang and Yang, 2022):
see Redundant pathways.
The modulating [e.g., activating/agonizing, inhibiting/antagonizing, (up-/down-)regulating, etc.], nature of a ligand/small-molecule drug toward a panel of related targets belonging to a common target (sub/super)family (Namasivayam et al., 2021c).
Three-dimensional molecular-structural motif of a small molecule necessary to act as a ligand of the target(s) of interest.
Screening of potential ligands/small-molecule drugs for particular in vitro and/or in vivo biological effects with multidimensional readouts; also referred to as High-content screening (Proudfoot et al., 2011; Kim et al., 2014; Proschak et al., 2019).
Analysis of the therapeutic effectiveness of multiple drugs on a biological system (Wang and Yang, 2022).
Analysis of absorption, distribution, metabolism, and excretion of multiple drugs in a biological system (Wang and Yang, 2022).
The interaction of a single (polyvalent) drug with multiple targets and/or disease pathways to treat a pathological condition; polypharmacology is related to the positive connotation of promiscuity; the single drug can be a hybrid/chimeric compound (conjugate) of individual drugs connected by a (potentially cleavable) linker or a genuinely multitarget single agent that is either fused (pharmacophore overlap) or merged (pharmacophore integration); polypharmacological ligands/small-molecule drugs can also be referred to as Multimeric ligands or Multifactorial compounds; the anticipated development of polypharmacological ligands/small-molecule drugs is referred to as Targeted polypharmacology (Handl et al., 2004; Morphy et al., 2004; Morphy and Rankovic, 2005; Hopkins et al., 2006; Morphy and Rankovic, 2006; Korcsmaros et al., 2007; Vulpetti et al., 2012; Jalencas and Mestres, 2013b; Medina-Franco et al., 2013; Peters, 2013; Anighoro et al., 2014; Bansal and Silakari, 2014; Santos et al., 2016; Polanski and Bak, 2019; Proschak et al., 2019; Wang and Yang, 2022).
The opportunity space of molecular interactions between the structural-biological limitation of target proteins (Structural conservatism of nature) and the molecular-structural limitation of multitarget ligands/small-molecule drugs (Multitarget fingerprint; Superpatterns) (Stefan and Rafehi, 2023; Rafehi et al., 2024).
Combined use of multiple (single-targeted) drugs to treat a pathological condition with combined effects; also referred to as Combination drug therapy (CTD), Drug cocktail (individual drugs), or Multicomponent drug (coformulated drugs); polypharmacy anticipates the use of drugs with different modes-of-action to achieve Selective synergy (increased efficacy at reduced adverse effects); also referred to as Polypharmacotherapy (Morphy and Rankovic, 2005; Korcsmaros et al., 2007; Carragher et al., 2012; Jalencas and Mestres, 2013a; Peters, 2013; McKie, 2016; Wang and Yang, 2022).
see Polypharmacy.
see Promiscuous target.
Molecular frameworks and entities (Superpatterns) and/or high-quality compound collections that conserve diverse biological activities, which “cross-over” target protein (super)families, and may be used to address yet undruggable targets; often referred to as “rich sources of chemical diversity” with “broad-range biological activity”; furthermore, privileged ligands can be defined as high-quality compound collections of functionally diverse drugs with diverse molecular targets to obtain an optimized Combination drug therapy (CTD) in Phenotypic screenings (High-content screenings) to overcome resistance and/or to acknowledge and address the multifactoricity of diseases (Morphy et al., 2004; Duarte et al., 2007; Zimmermann et al., 2007; Proudfoot et al., 2011; Jalencas and Mestres, 2013a; Kim et al., 2014; Stork and Kirchmair, 2018; Li et al., 2022; Wang and Yang, 2022; Stefan and Rafehi, 2023; Tolomeu and Fraga, 2023).
see Superpatterns.
see Superpatterns.
A multitarget character of a ligand/small-molecule drug that includes both the therapeutic effects through interaction with the intended targets and the adverse events from Off-target effects; in polypharmacological drug design, it is also referred to as Chemical promiscuity, Functional promiscuity, and Strategic promiscuity; promiscuity is nowadays considered to be a rather negative characteristic (Stark, 2004; Hopkins et al., 2006; Vulpetti et al., 2012; Jalencas and Mestres, 2013a; Peters, 2013; Anighoro et al., 2014; Polanski and Bak, 2019; Bajorath, 2022; Wang and Yang, 2022).
Strong difference in activities between molecules of close molecular-structural similarity (Matched molecular pair) toward multiple targets (McKie, 2016).
A target with susceptibility to be addressed by various different compound classes; also referred to as Polyspecificity (Hopkins et al., 2006; Degiacomi et al., 2020; Wang and Yang, 2022).
Clustering of secondary protein structures around the ligand-sensing cores (secondary protein structure of binding sites and/or catalytic centers) to identify common supra-structural motifs and Supersites (Russell et al., 1998; Koch et al., 2004; Koch, 2011).
Computer-aided prediction of biological activity of small molecules based on Structure–activity relationships (SARs) data.
Pathways and/or signaling cascades that consist of proteins from the same protein family with similar biological outcomes; also referred to as Pan-targets (Hopkins, 2008; Vulpetti et al., 2012; Stefan et al., 2020; Wang and Yang, 2022).
Network of repurposed drugs, including their annotated targets and bioactivities (Cavalla and Crichton, 2023).
see Drug repurposing.
Establishment of assay parameters as determined in the Assay validation in a High-content screening format (Proudfoot et al., 2011).
see Polypharmacy.
Ability of a ligand/small-molecule drug to exclusively modulate the target of interest compared to other, phylogenetically close or distant (alternative) targets or target classes; it is dependent on Target profiling assays with large numbers of assessed targets; it shall be noted that true (global) selectivity does not exist (Proudfoot et al., 2011; Jalencas and Mestres, 2013b; Antolin and Mestres, 2015; Bajorath, 2021).
Unintended but fortunate discovery; to date, most discoveries in Polypharmacology were serendipitous, with retrospective elucidation of modes-of-modulation (Morphy et al., 2004; Morphy and Rankovic, 2005; Morphy and Rankovic, 2007; Ravikumar and Aittokallio, 2018; Proschak et al., 2019; Bajorath, 2022; Wang and Yang, 2022).
see Off-target effect.
see Specificity paradigm.
Includes both the charted and uncharted inventory of ligands/small-molecule drugs, partial structures and functional groups, targets, and/or bioactivities; space refers to what is available and what could (potentially) be available on the chemical (Chemical space) and biological levels (Target space and Bioactivity space); often referred to as Chemogenomic space in which chemical and binding site similarities/conserved target motifs are linked (Koch, 2011; Vulpetti et al., 2012; Fechner et al., 2013; Mousavian and Masoudi-Nejad, 2014; Namasivayam et al., 2022b; Stefan et al., 2022; Wu et al., 2022; Puri et al., 2023).
The degree by which the observed effect in a biological testing system is caused by the specific interaction between a ligand/small-molecule drug and the target (class) of interest.
The concept that a drug (candidate) should address one target only; also referred to as One target-one drug model, Single-target drug (STD) concept, One target-one disease philosophy, or One molecule-one target-one disease philosophy (Morphy et al., 2004; Morphy and Rankovic, 2005; Hopkins et al., 2006; Zimmermann et al., 2007; Morphy and Rankovic, 2009; Jalencas and Mestres, 2013a; Medina-Franco et al., 2013; Peters, 2013; Santos et al., 2016; Bajorath, 2022; Wang and Yang, 2022).
see Promiscuity.
The principle that spatial structure is more conserved than amino acid sequences in nature, and that the number of fold types for protein domains is limited (Russell et al., 1998; Grishin, 2001; Koch et al., 2004).
Correlation between molecular-structural specificities of ligands/small-molecule drugs and their exerted biological effects (Proudfoot et al., 2011).
Computer-aided extraction of information about descriptors and fingerprints deduced from ligands/small-molecule drugs co-crystallized/bound to the target(s) of interest in (a) resolved target structure(s), for example, determined by crystallography or cryo-EM (Proschak et al., 2019).
Similar folding of chain topologies of proteins with low sequence similarity; superfolds may form the basis for Supersites (Orengo et al., 1994; Russell et al., 1998).
Molecular-structural frameworks, entities, and/or elements that reoccur in Privileged ligands and form the molecular-structural basis of polypharmacology toward a set of structurally, functionally, and/or phylogenetically (un)related targets, particularly regarding the binding to Superfolds and Supersites (Stefan and Rafehi, 2023).
Supra-structural motifs within Superfolds that bind similar ligands/small-molecule drugs despite phylogenetic distance of the proteins considered; related to Chemoisosterism; supersites can be explored by Protein structure similarity clustering (Russell et al., 1998; Koch et al., 2004; Koch, 2011; Jalencas and Mestres, 2013b; Anighoro et al., 2014).
Definition and mapping of entire biochemical regulation mechanisms, including monitoring the responses of Cellular networks and the Interactome to perturbations to understand complex interactions in biological systems (Azmi and Mohammad, 2014; McKie, 2016; Wang and Yang, 2022; Wu et al., 2022).
Definition and mapping of networks of interacting small-molecular components that form new functions and obtain emergent properties at different hierarchical levels; systems chemistry emphasizes the boundary between prebiotic and biotic systems focusing autocatalytic systems, compartmentalized chemical systems, dynamic (or constitutional) Combinatorial chemistry, kinetic stability, self-assembly, self-maintaining, self-organization, self-replicating, self-reproducing (non-)metabolic networks, and thermodynamic equilibria (Altamura and Fiore, 2022).
see Network pharmacology.
Similar to Target repurposing, but the actual target of interest is unknown, and it is only assumed to belong to a similar target class for which pharmacological tools exist; the term Target repurposing is often used in the pharmacological translation between species/organisms; related to Target hopping, target class repurposing is also used in the context of a transfer of knowledge from one protein family to another functionally and/or phylogenetically distant (known) protein family (Klug et al., 2016; Singh et al., 2020; Haupenthal et al., 2024).
A group of functionally, inter-cellularly, and/or cell-type-linked proteins that elicit a physiological function (Wang and Yang, 2022).
Retrospective Target identification after Phenotypic screening (High-content screening) (Terstappen et al., 2007; Bowes et al., 2012; Proschak et al., 2019).
Computational prediction of compound–target interactions based on the chemical structure of a ligand/small-molecule drug using biologically annotated chemical databases; in silico target fishing is an integral part of Target identification at the very beginning of the drug development pipeline; several web tools exist to predict novel drug targets (Jenkins et al., 2006; Vulpetti et al., 2012; Wang and Xie, 2014; McKie, 2016; Ji et al., 2023).
Experimental setup using immobilized ligands/small-molecule drugs to identify novel binding partners by, for example, mass spectrometry, quantitative proteomics, or microarrays; in vitro target fishing is an integral part of Target identification at the beginning of the drug development pipeline; in vitro target fishing can be seen as reciprocal to Ligand fishing (Chen et al., 2020).
The interaction between two or more targets through chemical space by sharing a similar set of ligands/small-molecule drugs without further interactions in physical or phylogenetic space and subsequent use of this set of ligands/small-molecule drugs to explore these drug targets (Paolini et al., 2006).
Discovery of a particular protein (family) or pathway with clear association to a pathological condition. Target identification represents the very beginning of the drug development pipeline.
The organization of phylogenetically related protein superfamily members within a broad spectrum of evolutionary variation and functional diversity (McKie, 2016).
Discipline which analyzes the likelihood of a ligand/small-molecule drug, which binds to one particular target protein, to also bind to phylogenetically related target proteins (Anighoro et al., 2014).
In silico methodology to predict interactions between a panel of phylogenetically close and/or distant target proteins and (a) virtual ligand(s)/small-molecule drug(s); in the drug development pipeline, target profiling is used to discover unknown and/or unwanted Off-target effects; target profiling can be seen as reciprocal to Drug profiling (Jalencas and Mestres, 2013b; Antolin and Mestres, 2015).
Translation of knowledge (e.g., ligands) derived from a target (class) or group of targets to a known, functionally, and/or phylogenetically related target of interest; this term is often used in the pharmacological translation between species/organisms. Related to Target hopping and Target class repurposing (Paolini et al., 2006; Pollastri and Campbell, 2011; Klug et al., 2016; Singh et al., 2020).
see Space.
Exploration and assessment of newly identified potential drug target(s) for critical aspects in drug discovery, such as assayability and druggability (Emmerich et al., 2021).
Anticipated development of ligands/small-molecule drugs that engage multiple targets with the aim of enhancing efficacy and safety (Morphy and Rankovic, 2006).
The opposite of Druggability (Coleman and Rodon, 2021).
The opposite of Selectivity; also referred to as Non-selectivity or Cross-reactivity (Morphy et al., 2004; Morphy and Rankovic, 2005; Morphy and Rankovic, 2006; Ravikumar and Aittokallio, 2018).
Biological effects that do not relate to the specific drug-target interaction but to other, unspecific interactions leading to the same experimental outcome/observation; also referred to as Non-specific effect.
Screening of diverse (Chemical space) or focused virtual compound libraries applying descriptors and/or fingerprints to obtain novel Chemotypes in the context of the anticipated targets; several virtual screening approaches have been developed to uncover polypharmacological ligands/small-molecule drugs, such as Computer-aided pattern analysis (C@PA) or Computer-aided pattern scoring (C@PS) (Peters, 2013; Namasivayam et al., 2021a; Namasivayam et al., 2021b; Namasivayam et al., 2022b, Stefan et al., 2024).
see Orphan target.
As sophisticated and mature the above terminology seems, there is no doubt that medicinal polypharmacology, with all its facets, is still at an early stage despite two decades of evolution (Anighoro et al., 2014; Proschak et al., 2019). Medicinal polypharmacology has attracted the attention of medicinal chemists, chemical biologists, clinical pharmacologists, and researchers from various other disciplines; however, the fact that it is still a small and young field limits its advancement.
Nevertheless, medicinal polypharmacology has an inherently multidisciplinary and translational character, bearing the unique chance to promote more integral and sophisticated strategies in modern drug development through international collaboration to 1) biologically assess, 2) structurally explore, and 3) clinically evaluate yet hidden drug targets and drug target combinations of the future.
SS: conceptualization, funding acquisition, project administration, resources, writing–original draft, and writing–review and editing. MR: conceptualization, funding acquisition, project administration, resources, writing–original draft, and writing–review and editing.
The author(s) declare that financial support was received for the research, authorship, and/or publication of this article. SS was supported by the Research Grant program of the German Research Foundation (Deutsche Forschungsgemeinschaft, DFG, Germany; #504079349 [PANABC]). MR received funding from the DFG (#437446827) and the research program of the University Medical Center Göttingen.
The authors declare that the research was conducted in the absence of any commercial or financial relationships that could be construed as a potential conflict of interest.
All claims expressed in this article are solely those of the authors and do not necessarily represent those of their affiliated organizations, or those of the publisher, the editors and the reviewers. Any product that may be evaluated in this article, or claim that may be made by its manufacturer, is not guaranteed or endorsed by the publisher.
Altamura, E., and Fiore, M. (2022). The origin and early evolution of life: (prebiotic) systems chemistry perspective. Life (Basel) 12 (5), 710. doi:10.3390/life12050710
Anighoro, A., Bajorath, J., and Rastelli, G. (2014). Polypharmacology: challenges and opportunities in drug discovery. J. Med. Chem. 57 (19), 7874–7887. doi:10.1021/jm5006463
Antolin, A. A., and Mestres, J. (2015). Distant polypharmacology among MLP chemical probes. ACS Chem. Biol. 10 (2), 395–400. doi:10.1021/cb500393m
Azmi, A. S., and Mohammad, R. M. (2014). Rectifying cancer drug discovery through network pharmacology. Future Med. Chem. 6 (5), 529–539. doi:10.4155/fmc.14.6
Bajorath, J. (2021). Minimal screening requirements for identifying highly promiscuous kinase inhibitors. Future Med. Chem. 13 (13), 1083–1085. doi:10.4155/fmc-2021-0077
Bajorath, J. (2022). Explainable machine learning for medicinal chemistry: exploring multi-target compounds. Future Med. Chem. 14 (16), 1171–1173. doi:10.4155/fmc-2022-0122
Bansal, Y., and Silakari, O. (2014). Multifunctional compounds: smart molecules for multifactorial diseases. Eur. J. Med. Chem. 76, 31–42. doi:10.1016/j.ejmech.2014.01.060
Barabasi, A. L., and Oltvai, Z. N. (2004). Network biology: understanding the cell's functional organization. Nat. Rev. Genet. 5 (2), 101–113. doi:10.1038/nrg1272
Besnard, J., Ruda, G. F., Setola, V., Abecassis, K., Rodriguiz, R. M., Huang, X. P., et al. (2012). Automated design of ligands to polypharmacological profiles. Nature 492 (7428), 215–220. doi:10.1038/nature11691
Bowes, J., Brown, A. J., Hamon, J., Jarolimek, W., Sridhar, A., Waldron, G., et al. (2012). Reducing safety-related drug attrition: the use of in vitro pharmacological profiling. Nat. Rev. Drug Discov. 11 (12), 909–922. doi:10.1038/nrd3845
Bray, M. A., Singh, S., Han, H., Davis, C. T., Borgeson, B., Hartland, C., et al. (2016). Cell Painting, a high-content image-based assay for morphological profiling using multiplexed fluorescent dyes. Nat. Protoc. 11 (9), 1757–1774. doi:10.1038/nprot.2016.105
Brunst, S., Kramer, J. S., Kilu, W., Heering, J., Pollinger, J., Hiesinger, K., et al. (2021). Systematic assessment of fragment identification for multitarget drug design. ChemMedChem 16 (7), 1088–1092. doi:10.1002/cmdc.202000858
Brunye, T. T., Balla, A., Drew, T., Elmore, J. G., Kerr, K. F., Shucard, H., et al. (2023). From image to diagnosis: characterizing sources of error in histopathologic interpretation. Mod. Pathol. 36 (7), 100162. doi:10.1016/j.modpat.2023.100162
Carragher, N. O., Unciti-Broceta, A., and Cameron, D. A. (2012). Advancing cancer drug discovery towards more agile development of targeted combination therapies. Future Med. Chem. 4 (1), 87–105. doi:10.4155/fmc.11.169
Cavalla, D., and Crichton, G. (2023). Drug repurposing: known knowns to unknown unknowns - network analysis of the repurposome. Drug Discov. Today 28 (7), 103639. doi:10.1016/j.drudis.2023.103639
Chen, X., Wang, Y., Ma, N., Tian, J., Shao, Y., Zhu, B., et al. (2020). Target identification of natural medicine with chemical proteomics approach: probe synthesis, target fishing and protein identification. Signal Transduct. Target Ther. 5 (1), 72. doi:10.1038/s41392-020-0186-y
Coleman, N., and Rodon, J. (2021). Taking aim at the undruggable. Am. Soc. Clin. Oncol. Educ. Book 41, 1–8. doi:10.1200/EDBK_325885
Dahlin, J. L., Auld, D. S., Rothenaigner, I., Haney, S., Sexton, J. Z., Nissink, J. W. M., et al. (2021). Nuisance compounds in cellular assays. Cell Chem. Biol. 28 (3), 356–370. doi:10.1016/j.chembiol.2021.01.021
Degiacomi, G., Belardinelli, J. M., Pasca, M. R., De Rossi, E., Riccardi, G., and Chiarelli, L. R. (2020). Promiscuous targets for antitubercular drug discovery: the paradigm of DprE1 and MmpL3. Appl. Sci. 10 (2), 623. doi:10.3390/app10020623
Duarte, C. D., Barreiro, E. J., and Fraga, C. A. (2007). Privileged structures: a useful concept for the rational design of new lead drug candidates. Mini Rev. Med. Chem. 7 (11), 1108–1119. doi:10.2174/138955707782331722
Emmerich, C. H., Gamboa, L. M., Hofmann, M. C. J., Bonin-Andresen, M., Arbach, O., Schendel, P., et al. (2021). Improving target assessment in biomedical research: the GOT-IT recommendations. Nat. Rev. Drug Discov. 20 (1), 64–81. doi:10.1038/s41573-020-0087-3
Fechner, N., Papadatos, G., Evans, D., Morphy, J. R., Brewerton, S. C., Thorner, D., et al. (2013). ChEMBLSpace--a graphical explorer of the chemogenomic space covered by the ChEMBL database. Bioinformatics 29 (4), 523–524. doi:10.1093/bioinformatics/bts711
Franchini, L., and Orlandi, C. (2023). Probing the orphan receptors: tools and directions. Prog. Mol. Biol. Transl. Sci. 195, 47–76. doi:10.1016/bs.pmbts.2022.06.011
Franz-Odendaal, T. A. (2023). The elusive scleral cartilages: comparative anatomy and development in teleosts and avians. Anat. Rec. Hob., doi:10.1002/ar.25345
Grishin, N. V. (2001). Fold change in evolution of protein structures. J. Struct. Biol. 134 (2-3), 167–185. doi:10.1006/jsbi.2001.4335
Handl, H. L., Vagner, J., Han, H., Mash, E., Hruby, V. J., and Gillies, R. J. (2004). Hitting multiple targets with multimeric ligands. Expert Opin. Ther. Targets 8 (6), 565–586. doi:10.1517/14728222.8.6.565
Haupenthal, J., Rafehi, M., Kany, A. M., Lespine, A., Stefan, K., Hirsch, A. K. H., et al. (2024). Target repurposing unravels avermectins and derivatives as novel antibiotics inhibiting energy-coupling factor transporters (ECFTs). Arch. Pharm. doi:10.1002/ardp.202400267
Hopkins, A. L. (2008). Network pharmacology: the next paradigm in drug discovery. Nat. Chem. Biol. 4 (11), 682–690. doi:10.1038/nchembio.118
Hopkins, A. L., Mason, J. S., and Overington, J. P. (2006). Can we rationally design promiscuous drugs? Curr. Opin. Struct. Biol. 16 (1), 127–136. doi:10.1016/j.sbi.2006.01.013
Hu, Y., and Bajorath, J. (2010). Polypharmacology directed compound data mining: identification of promiscuous chemotypes with different activity profiles and comparison to approved drugs. J. Chem. Inf. Model 50 (12), 2112–2118. doi:10.1021/ci1003637
Jalencas, X., and Mestres, J. (2013a). On the origins of drug polypharmacology. Med. Chem. Comm. 4, 80–87. doi:10.1039/c2md20242e
Jalencas, X., and Mestres, J. (2013b). Identification of similar binding sites to detect distant polypharmacology. Mol. Inf. 32 (11-12), 976–990. doi:10.1002/minf.201300082
Jenkins, J. L., Bender, A., and Davies, J. W. (2006). In silico target fishing: predicting biological targets from chemical structure. Drug Discov. Today Technol. 3 (4), 413–421. doi:10.1016/j.ddtec.2006.12.008
Ji, K. Y., Liu, C., Liu, Z. Q., Deng, Y. F., Hou, T. J., and Cao, D. S. (2023). Comprehensive assessment of nine target prediction web services: which should we choose for target fishing? Brief. Bioinform 24 (2), bbad014. doi:10.1093/bib/bbad014
Joshi, P. B. (2023). Navigating with chemometrics and machine learning in chemistry. Artif. Intell. Rev. 56, 9089–9114. doi:10.1007/s10462-023-10391-w
Judge, D. P., Kristen, A. V., Grogan, M., Maurer, M. S., Falk, R. H., Hanna, M., et al. (2020). Correction to: phase 3 multicenter study of revusiran in patients with hereditary transthyretin-mediated (hATTR) amyloidosis with cardiomyopathy (ENDEAVOUR). Cardiovasc Drugs Ther. 34 (6), 889. doi:10.1007/s10557-020-07023-8
Keith, C. T., Borisy, A. A., and Stockwell, B. R. (2005). Multicomponent therapeutics for networked systems. Nat. Rev. Drug Discov. 4 (1), 71–78. doi:10.1038/nrd1609
Kim, J., Kim, H., and Park, S. B. (2014). Privileged structures: efficient chemical “navigators” toward unexplored biologically relevant chemical spaces. J. Am. Chem. Soc. 136 (42), 14629–14638. doi:10.1021/ja508343a
Klug, D. M., Gelb, M. H., and Pollastri, M. P. (2016). Repurposing strategies for tropical disease drug discovery. Bioorg Med. Chem. Lett. 26 (11), 2569–2576. doi:10.1016/j.bmcl.2016.03.103
Koch, M. A., Wittenberg, L. O., Basu, S., Jeyaraj, D. A., Gourzoulidou, E., Reinecke, K., et al. (2004). Compound library development guided by protein structure similarity clustering and natural product structure. Proc. Natl. Acad. Sci. U. S. A. 101 (48), 16721–16726. doi:10.1073/pnas.0404719101
Koch, O. (2011). Use of secondary structure element information in drug design: polypharmacology and conserved motifs in protein-ligand binding and protein-protein interfaces. Future Med. Chem. 3 (6), 699–708. doi:10.4155/fmc.11.26
Korcsmaros, T., Szalay, M. S., Bode, C., Kovacs, I. A., and Csermely, P. (2007). How to design multi-target drugs. Expert Opin. Drug Discov. 2 (6), 799–808. doi:10.1517/17460441.2.6.799
Li, D., Li, Y., Jiang, X., Liu, W., and Zhao, Q. (2022). Evodiamine: a privileged structure with broad-ranging biological activities. Mini Rev. Med. Chem. 22 (21), 2680–2701. doi:10.2174/1389557522666220404090835
Lipinski, C. A., Lombardo, F., Dominy, B. W., and Feeney, P. J. (2001). Experimental and computational approaches to estimate solubility and permeability in drug discovery and development settings. Adv. Drug Deliv. Rev. 46 (1-3), 3–26. doi:10.1016/s0169-409x(00)00129-0
McKie, S. A. (2016). Polypharmacology: in silico methods of ligand design and development. Future Med. Chem. 8 (5), 579–602. doi:10.4155/fmc-2015-0006
Medina-Franco, J. L., Giulianotti, M. A., Welmaker, G. S., and Houghten, R. A. (2013). Shifting from the single to the multitarget paradigm in drug discovery. Drug Discov. Today 18 (9-10), 495–501. doi:10.1016/j.drudis.2013.01.008
Morphy, R., Kay, C., and Rankovic, Z. (2004). From magic bullets to designed multiple ligands. Drug Discov. Today 9 (15), 641–651. doi:10.1016/S1359-6446(04)03163-0
Morphy, R., and Rankovic, Z. (2005). Designed multiple ligands. An emerging drug discovery paradigm. J. Med. Chem. 48 (21), 6523–6543. doi:10.1021/jm058225d
Morphy, R., and Rankovic, Z. (2006). The physicochemical challenges of designing multiple ligands. J. Med. Chem. 49 (16), 4961–4970. doi:10.1021/jm0603015
Morphy, R., and Rankovic, Z. (2007). Fragments, network biology and designing multiple ligands. Drug Discov. Today 12 (3-4), 156–160. doi:10.1016/j.drudis.2006.12.006
Morphy, R., and Rankovic, Z. (2009). Designing multiple ligands - medicinal chemistry strategies and challenges. Curr. Pharm. Des. 15 (6), 587–600. doi:10.2174/138161209787315594
Mousavian, Z., and Masoudi-Nejad, A. (2014). Drug-target interaction prediction via chemogenomic space: learning-based methods. Expert Opin. Drug Metab. Toxicol. 10 (9), 1273–1287. doi:10.1517/17425255.2014.950222
Namasivayam, V., Silbermann, K., Pahnke, J., Wiese, M., and Stefan, S. M. (2021a). Scaffold fragmentation and substructure hopping reveal potential, robustness, and limits of computer-aided pattern analysis (C@PA). Comput. Struct. Biotechnol. J. 19, 3269–3283. doi:10.1016/j.csbj.2021.05.018
Namasivayam, V., Silbermann, K., Wiese, M., Pahnke, J., and Stefan, S. M. (2021b). C@PA: computer-aided pattern analysis to predict multitarget ABC transporter inhibitors. J. Med. Chem. 64 (6), 3350–3366. doi:10.1021/acs.jmedchem.0c02199
Namasivayam, V., Stefan, K., Gorecki, L., Korabecny, J., Soukup, O., Jansson, P. J., et al. (2022a). Physicochemistry shapes bioactivity landscape of pan-ABC transporter modulators: anchor point for innovative Alzheimer's disease therapeutics. Int. J. Biol. Macromol. 217, 775–791. doi:10.1016/j.ijbiomac.2022.07.062
Namasivayam, V., Stefan, K., Pahnke, J., and Stefan, S. M. (2021c). Binding mode analysis of ABCA7 for the prediction of novel Alzheimer's disease therapeutics. Comput. Struct. Biotechnol. J. 19, 6490–6504. doi:10.1016/j.csbj.2021.11.035
Namasivayam, V., Stefan, K., Silbermann, K., Pahnke, J., Wiese, M., and Stefan, S. M. (2022b). Structural feature-driven pattern analysis for multitarget modulator landscapes. Bioinformatics 38 (5), 1385–1392. doi:10.1093/bioinformatics/btab832
Orengo, C. A., Jones, D. T., and Thornton, J. M. (1994). Protein superfamilies and domain superfolds. Nature 372 (6507), 631–634. doi:10.1038/372631a0
Paolini, G. V., Shapland, R. H., van Hoorn, W. P., Mason, J. S., and Hopkins, A. L. (2006). Global mapping of pharmacological space. Nat. Biotechnol. 24 (7), 805–815. doi:10.1038/nbt1228
Peters, J. U. (2013). Polypharmacology - foe or friend? J. Med. Chem. 56 (22), 8955–8971. doi:10.1021/jm400856t
Polanski, J., and Bak, A. (2019). Ligand potency - an essential estimator for drug design: between intuition, misinterpretation and serendipity. Future Med. Chem. 11 (14), 1827–1843. doi:10.4155/fmc-2018-0230
Pollastri, M. P., and Campbell, R. K. (2011). Target repurposing for neglected diseases. Future Med. Chem. 3 (10), 1307–1315. doi:10.4155/fmc.11.92
Popovic, A., Morelato, M., Roux, C., and Beavis, A. (2019). Review of the most common chemometric techniques in illicit drug profiling. Forensic Sci. Int. 302, 109911. doi:10.1016/j.forsciint.2019.109911
Proschak, E., Stark, H., and Merk, D. (2019). Polypharmacology by design: a medicinal chemist's perspective on multitargeting compounds. J. Med. Chem. 62 (2), 420–444. doi:10.1021/acs.jmedchem.8b00760
Proudfoot, J., Nosjean, O., Blanchard, J., Wang, J., Besson, D., Crankshaw, D., et al. (2011) Glossary of terms used in biomolecular screening. Research Triangle Park, NC, USA: IUPAC Standards Online.
Puri, S., Stefan, K., Khan, S. L., Pahnke, J., Stefan, S. M., and Juvale, K. (2023). Indole derivatives as new structural class of potent and antiproliferative inhibitors of monocarboxylate transporter 1 (MCT1; SLC16A1). J. Med. Chem. 66 (1), 657–676. doi:10.1021/acs.jmedchem.2c01612
Rafehi, M., Moller, M., Ismail Al-Khalil, W., and Stefan, S. M. (2024). Medicinal polypharmacology in the clinic - translating the polypharmacolome into therapeutic benefit. Pharm. Res. 41, 411–417. doi:10.1007/s11095-024-03656-8
Ravikumar, B., and Aittokallio, T. (2018). Improving the efficacy-safety balance of polypharmacology in multi-target drug discovery. Expert Opin. Drug Discov. 13 (2), 179–192. doi:10.1080/17460441.2018.1413089
Russell, R. B., Sasieni, P. D., and Sternberg, M. J. (1998). Supersites within superfolds. Binding site similarity in the absence of homology. J. Mol. Biol. 282 (4), 903–918. doi:10.1006/jmbi.1998.2043
Santos, M. A., Chand, K., and Chaves, S. (2016). Recent progress in repositioning Alzheimer's disease drugs based on a multitarget strategy. Future Med. Chem. 8 (17), 2113–2142. doi:10.4155/fmc-2016-0103
Schmidt, F., Matter, H., Hessler, G., and Czich, A. (2014). Predictive in silico off-target profiling in drug discovery. Future Med. Chem. 6 (3), 295–317. doi:10.4155/fmc.13.202
Schneidewind, T., Brause, A., Pahl, A., Burhop, A., Mejuch, T., Sievers, S., et al. (2020). Morphological profiling identifies a common mode of action for small molecules with different targets. Chembiochem 21 (22), 3197–3207. doi:10.1002/cbic.202000381
Singh, B., Bernatchez, J. A., McCall, L. I., Calvet, C. M., Ackermann, J., Souza, J. M., et al. (2020). Scaffold and parasite hopping: discovery of new Protozoal proliferation inhibitors. ACS Med. Chem. Lett. 11 (3), 249–257. doi:10.1021/acsmedchemlett.9b00453
Stark, H. (2004). Turning from monogamy to strategic promiscuity. Drug Discov. Today 9 (17), 736–737. doi:10.1016/S1359-6446(04)03208-8
Stefan, K., Wen Leck, L. Y., Namasivayam, V., Bascuñana, P., Huang, M. L.-H., Riss, P. J., et al. (2020). Vesicular ATP-binding cassette transporters in human disease: relevant aspects of their organization for future drug development. Future Drug Discov. 2 (4), FDD51. doi:10.4155/fdd-2020-0025
Stefan, S. M. (2019). Multi-target ABC transporter modulators: what next and where to go? Future Med. Chem. 11 (18), 2353–2358. doi:10.4155/fmc-2019-0185
Stefan, S. M., Jansson, P. J., Pahnke, J., and Namasivayam, V. (2022). A curated binary pattern multitarget dataset of focused ATP-binding cassette transporter inhibitors. Sci. Data 9 (1), 446. doi:10.1038/s41597-022-01506-z
Stefan, S. M., Pahnke, J., and Namasivayam, V. (2023). HD_BPMDS: a curated binary pattern multitarget dataset of Huntington's disease-targeting agents. J. Cheminform 15 (1), 109. doi:10.1186/s13321-023-00775-z
Stefan, S. M., and Rafehi, M. (2023). Medicinal polypharmacology: exploration and exploitation of the polypharmacolome in modern drug development. Drug Dev. Res. 85, e22125. doi:10.1002/ddr.22125
Stefan, S. M., and Rafehi, M. (2024). The big data challenge - and how polypharmacology supports the translation from pre-clinical research into clinical use against neurodegenerative diseases and beyond. Neural Regen. Res. 19 (8), 1647–1648. doi:10.4103/1673-5374.387984
Stefan, K., Namasivayam, V., and Stefan, S. M. (2024) Computer-aided pattern scoring-a multitarget dataset-driven workflow to predict ligands of orphan targets. Sci. Data. 11 (1), 530. doi:10.1038/s41597-024-03343-8
Stork, C., and Kirchmair, J. (2018). PAIN(S) relievers for medicinal chemists: how computational methods can assist in hit evaluation. Future Med. Chem. 10 (13), 1533–1535. doi:10.4155/fmc-2018-0116
Tao, B., Vosoughi, A., Margolin, E., and Micieli, J. A. (2023). Inappropriate use of the term “papilledema” in the medical literature: a systematic review of case reports across specialties. Ophthalmology 130 (2), 129–136. doi:10.1016/j.ophtha.2022.09.017
Terstappen, G. C., Schlupen, C., Raggiaschi, R., and Gaviraghi, G. (2007). Target deconvolution s trategies in drug discovery. Nat. Rev. Drug Discov. 6 (11), 891–903. doi:10.1038/nrd2410
Tolomeu, H. V., and Fraga, C. A. M. (2023). Imidazole: synthesis, functionalization and physicochemical properties of a privileged structure in medicinal chemistry. Molecules 28 (2), 838. doi:10.3390/molecules28020838
Vulpetti, A., Kalliokoski, T., and Milletti, F. (2012). Chemogenomics in drug discovery: computational methods based on the comparison of binding sites. Future Med. Chem. 4 (15), 1971–1979. doi:10.4155/fmc.12.147
Wang, L., and Xie, X. Q. (2014). Computational target fishing: what should chemogenomics researchers expect for the future of in silico drug design and discovery? Future Med. Chem. 6 (3), 247–249. doi:10.4155/fmc.14.5
Wassermann, A. M., Geppert, H., and Bajorath, J. (2009). Ligand prediction for orphan targets using support vector machines and various target-ligand kernels is dominated by nearest neighbor effects. J. Chem. Inf. Model 49 (10), 2155–2167. doi:10.1021/ci9002624
Wassermann, A. M., Lounkine, E., Hoepfner, D., Le Goff, G., King, F. J., Studer, C., et al. (2015). Dark chemical matter as a promising starting point for drug lead discovery. Nat. Chem. Biol. 11 (12), 958–966. doi:10.1038/nchembio.1936
Wu, J., Möhle, L., Brüning, T., Eiriz, I., Rafehi, M., Stefan, K., et al. (2022). A novel huntington’s disease assessment platform to support future drug discovery and development. Int. J. Mol. Sci. 23 (23), 14763. doi:10.3390/ijms232314763
Zavorsky, G. S. (2021). Incorrect terminology confuses article's purpose and usefulness. Med. Sci. Sports Exerc 53 (6), 1315. doi:10.1249/MSS.0000000000002650
Zhan, P., and Liu, X. (2009). Designed multiple ligands: an emerging anti-HIV drug discovery paradigm. Curr. Pharm. Des. 15 (16), 1893–1917. doi:10.2174/138161209788453266
Keywords: polypharmacolome, drug repurposing, target repurposing, privileged structures, privileged ligands, network pharmacology, chemogenomic space, superfolds
Citation: Stefan SM and Rafehi M (2024) Medicinal polypharmacology—a scientific glossary of terminology and concepts. Front. Pharmacol. 15:1419110. doi: 10.3389/fphar.2024.1419110
Received: 17 April 2024; Accepted: 30 April 2024;
Published: 18 July 2024.
Edited by:
Yuxiang Dong, University of Nebraska Medical Center, United StatesReviewed by:
Elena Puris, Heidelberg University, GermanyCopyright © 2024 Stefan and Rafehi. This is an open-access article distributed under the terms of the Creative Commons Attribution License (CC BY). The use, distribution or reproduction in other forums is permitted, provided the original author(s) and the copyright owner(s) are credited and that the original publication in this journal is cited, in accordance with accepted academic practice. No use, distribution or reproduction is permitted which does not comply with these terms.
*Correspondence: Sven Marcel Stefan, c3Zlbi5zdGVmYW5AdW5pLWx1ZWJlY2suZGU=, c3Zlbi5zdGVmYW5AdW1sdWIucGw=; Muhammad Rafehi, bXVoYW1tYWQucmFmZWhpQG1lZC51bmktZ29ldHRpbmdlbi5kZQ==, bXVoYW1tYWQucmFmZWhpQG1lZC51bmktYXVnc2J1cmcuZGU=
†These authors have contributed equally to this work
Disclaimer: All claims expressed in this article are solely those of the authors and do not necessarily represent those of their affiliated organizations, or those of the publisher, the editors and the reviewers. Any product that may be evaluated in this article or claim that may be made by its manufacturer is not guaranteed or endorsed by the publisher.
Research integrity at Frontiers
Learn more about the work of our research integrity team to safeguard the quality of each article we publish.