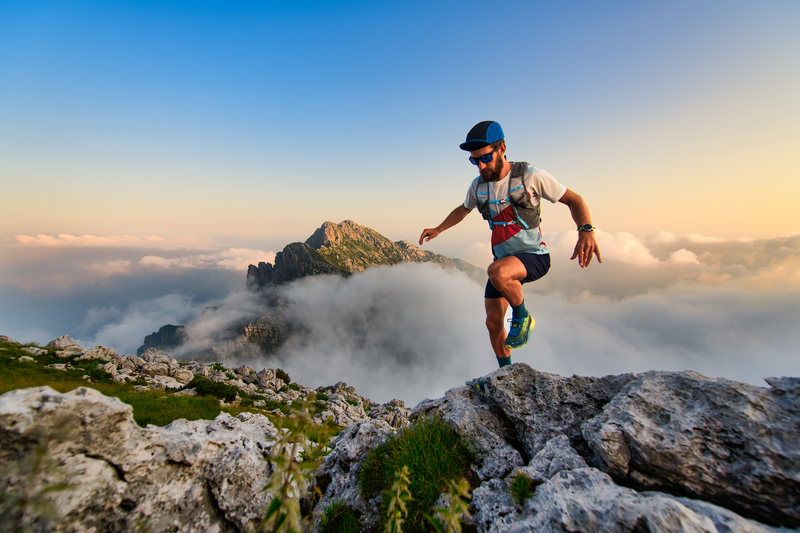
95% of researchers rate our articles as excellent or good
Learn more about the work of our research integrity team to safeguard the quality of each article we publish.
Find out more
ORIGINAL RESEARCH article
Front. Pharmacol. , 26 June 2024
Sec. Ethnopharmacology
Volume 15 - 2024 | https://doi.org/10.3389/fphar.2024.1408304
This article is part of the Research Topic Pine Bark Extract: Nutrition and Metabolism View all 6 articles
Introduction: Pycnogenol (PYC), a standardized extract from French maritime pine, has traditionally been used to treat inflammation. However, its primary active components and their mechanisms of action have not yet been determined.
Methods: This study employed UPLC-MS/MS (Ultra-high performance liquid chromatography-tandem mass spectrometry) and network pharmacology to identify the potential active components of PYC and elucidate their anti-inflammatory mechanisms by cell experiments.
Results: 768 PYC compounds were identified and 19 anti-inflammatory compounds were screened with 85 target proteins directly involved in the inflammation. PPI (protein-protein interaction) analysis identified IL6, TNF, MMP9, IL1B, AKT1, IFNG, CXCL8, NFKB1, CCL2, IL10, and PTGS2 as core targets. KEGG (Kyoto Encyclopedia of Genes and Genomes) enrichment analysis suggested that the compound in PYC might exert anti-inflammatory effects through the IL17 and TNF signal pathways. Cell experiments determined that PYC treatment can reduce the expression of IL6 and IL1β to relieve inflammation in LPS (lipopolysaccharide)-induced BV2 cells.
Conclusion: PYC could affect inflammation via multi-components, -targets, and -mechanisms.
Inflammation is a critical physiological and pathological process that prevents diseases, removes necrotic and damaged tissues, and targets infectious pathogens (Rafiyan and Sadeghmousavi, 2023; D'Arcy, 2019). Normally, inflammation resolves quickly and benefits the host; however, when acute inflammation becomes chronic, it can lead to a harmful cycle of aberrant inflammatory responses and prolonged tissue damage, contributing to the development of conditions such as diabetes, neurological disorders, heart disease, and cancer (Alderton and Scanlon, 2021; Langworth-Green et al., 2023; Nigam et al., 2023). Therefore, it is crucial to control inflammation through effective therapeutic strategies.
Pycnogenol (PYC) is a standardized extract from French maritime pine (Pinus maritime) comprising 65%–75% procyanidins. These procyanidins consist of varying chain-length subunits of epicatechin and catechin, along with other constituents such as flavonoids, polyphenolic monomers, and cinnamic or phenolic acids and their glycosides (Rohdewald, 2002). PYC exhibits immuno-modulatory, anti-inflammatory, antioxidant, and anti-carcinogenic effects (Maimoona et al., 2011). Furthermore, PYC has been traditionally used to treat various inflammatory diseases, including chronic, circulatory, and neurological conditions (Norouzy and Malekahmadi, 2023). Many in vitro studies have elucidated PYC’s anti-inflammatory mechanisms, including inhibition of proinflammatory cytokines and matrix metalloproteases, reduction of histamine release from mast cells, and blocking of inflammatory transcription factors (Cordova et al., 2013; Nattagh-Eshtivani et al., 2022).
Existing literature suggests that PYC significantly enhances antioxidant mediators, indicating that procyanidins may be its primary anti-inflammatory component (Shin et al., 2013). However, PYC also contains 25%–35% of other compounds, and the association of these ingredients with PYC’s anti-inflammatory effects remains unclear. Moreover, identifying and understanding the mechanisms of PYC’s anti-inflammatory compounds pose significant challenges. UPLC-MS/MS (Ultra-high performance liquid chromatography-tandem mass spectrometry) has high sensitivity, resolution, and selectivity; therefore, it has been utilized to characterize unknown trace components in herbal medicines rapidly (Wang et al., 2021). Network pharmacology, a novel interdisciplinary tool, merges aspects of pharmacology, computational and systems biology, network analysis, and bioinformatics to explore the relationships between traditional herbs and various diseases, facilitating the identification of signaling pathways and targets to enhance drug discovery efficiency holistically (Xian X et al., 2023). Therefore, integrating UHPLC-MS and network pharmacology offers a robust approach to assessing the anti-inflammatory components of PYC and their mechanisms.
This investigation employed the UPLC-MS/MS to assess the active PYC compounds. Then, network pharmacology was used to identify key active targets, components, and potential signaling pathways related to the anti-inflammatory effects, enabling a detailed analysis of PYC’s anti-inflammatory mechanism. This research aims to provide evidence supporting the comprehensive evaluation and clinical application of PYC for treating inflammation. The experimental procedures of this study are depicted in Figure 1.
PYC was acquired from the Horphag Research Ltd. (Geneva, Switzerland). UNIQ-10 column total RNA purification kit and CCK8 (Cell Counting Kit-8) were bought from Sangon Biotech Co., Ltd. (Shanghai, China). MagicSYBR Mixture and HiFiScript cDNA Synthesis Kit were sourced from Jiangsu Cowin Biotech Co., Ltd (Nanjing, China). The BV 2 cell line and its specified media were brought from Procell Life Science &Technology Co., Ltd. (Wuhan, China). Lipopolysaccharides (LPS), Micro NO (nitric oxide) content and Malondialdehyde (MDA) content kits were obtained from Solarbio Science and Technology Co., Ltd. (Beijing, China).
The PYC powder (2 g) was macerated with 50 mL double distilled H2O (dd H2O) in a 250 mL sample bottle and stored at 4°C for 12 h. This mixture was sonicated in an ice-cold water bath for 1 h and centrifuged at 4°C for 10 min at 10,000 × g to separate the buoyant fraction as sample (A), which was subsequently stored at 4°C. The remaining PYC residue was mixed with 30 mL of dd H2O, then sonicated, centrifuged, and collected as sample (B) using the abovementioned parameters. The extracted supernatant was then mixed and diluted to 100 mL with dd H2O as the final PYC sample for subsequent chemical analyses.
For chemical analysis, the UHPLC system (Vanquish, Thermo Fisher Scientific) fitted with a UPLC HSS T3 column (2.1 mm × 100 mm, 1.8 μm) coupled to an Orbitrap Exploris 120 mass spectrometer (Orbitrap MS, Thermo) was employed. The mobile phases included 5 mM/L acetic acid in acetonitrile and 5 mM/L ammonium acetate in water. The analysis was conducted at 40°C with a 0.4 mL/min flow rate.
With the help of the mass spectrometer, MS/MS spectra were obtained using the information-dependent acquisition (IDA) mode. The ESI source parameters were: 50 Arb flow rate of sheath gas, full MS resolution was 60000, 15 Arb flow rate of Aux gas, MS/MS resolution was 15000 collision energy as 10/30/60 in NCE mode, 320°C capillary temperature, spray Voltage was −3.4 kV (negative) or 3.8 kV (positive).
For 30 s, the extract supernatant (0.3 mL) and methanol extract solution (1 mL) were vortexed, then for 10 min was sonicated on ice-cold temperature before incubation for 1 h at −40°C and centrifugation at −40°C for 15 min at 12,000 rpm. The acquired supernatant was utilized for product assessment. The raw data extraction and chemical identification were based on the secondary and primary spectral data interpreted against the MS2 database. The UPLC-MS/MS analysis were commissioned by a professional biological company (Biotree DB, Shanghai).
The chemical components of PYC were characterized using UHPLC-MS. The compounds with anti-inflammatory bioactivity in PYC were identified using the Inflammatory Natural Products Database and Predictor (http://www.inflamnat.com/#/main/home). Compounds were further filtered based on ADME (absorption, distribution, metabolism, and excretion) properties from the Traditional Chinese Medicine Systems Pharmacology Database and Analysis Platform (TCMSP, https://tcmsp-e.com/index.php) with the criteria: drug-likeness (DL) ≥ 0.18, oral bioavailability (OB) ≥ 25%, and rotatable bond number (RBN) ≤ 10 (Li et al., 2022). Target predictions for these anti-inflammatory compounds were obtained from the TCMSP, High-throughput Experiment- and Reference-guided database of TCM (HERB, http://herb.ac.cn), and the Comparative Toxicogenomics Database (CTD, http://ctdbase.org). The human genes associated with the inflammation were screened from the Genecards database (https://www.genecards.org/) using the keyword “inflammation,” and to ensure results credibility, the relevance score was set to ≥5. The potential targets of the final anti-inflammatory compounds were assessed and compared with disease targets to acquire potential target genes for treating inflammation. Using the CTD, the common overlapping genes were depicted through the Venn diagram. Furthermore, these genes were uploaded to the STRING database (https://www.string-db.org/) to establish a protein-protein interaction (PPI) network and were graphically visualized using the Cytoscape (version 3.9.1). Moreover, Gene Ontology (GO) and Kyoto Encyclopedia of Genes and Genomes (KEGG) pathway enrichment assessments were conducted using the Database for Annotation, Visualization, and Integrated Discovery (DAVID, https://david.ncifcrf.gov/). The target organism was Homo sapiens, the false discovery rate (FDR)-adjusted p-value was employed for elucidating the enrichment analysis, and a p-value < 0.05 was termed significant.
Under the influence of various inflammatory factors, the BV2 microglial cell line can be over-activation and subsequently release inflammatory mediators such as TNFα, IL1β, and IL6, so here the BV2 microglial cell line were chosen for further study and was cultured at 37 °C in the BV2 cell specified media containing DMEM with 10% FBS (Fetal Bovine Serum) and 1% Penicillin-Streptomycin Solution, which is also the culture media for BV2 cells. Meanwhile, Both LPS and PYC were dissolved in BV2 cell specific media to obtain stocking solutions with concentrations of 10 μg/mL and 200 μg/mL, respectively.
BV2 microglial cells were seeded at 5,000 cells per well in a 96-well plate and cultured overnight in 100 μL of BV2 special medium. Then, for 24 h, the cells were treated with the PYC (at 100, 80, 60, 40, 20, 10, and 5 μg/mL concentrations) with and without LPS (1 μg/mL). The model group only received LPS (1 μg/mL) treatment, and the blank group received no treatment. For viability analysis, 10 μL of CCK-8 solution containing WST-8 (2-(2- methoxy-4-nitrophenyl)-3-(4-nitrophenyl)-5-(2,4-disulfophenyl)-2H-tetrazolium) was added to each well, and cells were incubated for 1 h at 37°C. The optical density (OD) was assessed using the Spark Multimode Reader Platform (Tecan, Switzerland) at 450 nm. The cell survival rate was elucidated as follows: Cell viability (%) = [(OD Sample-OD Blank)/(OD Control- OD Blank)] × 100%.
BV2 cells were cultured at 37°C in a 6-well plate at a density of 2 × 105 per well overnight in 5% CO2 using. Those cells were categorized into three groups: the blank (Control; received no treatment, the LPS (received BV2 special medium augmented with 1 μg/mL LPS), and the treatment (received BV2 special medium augmented with 80 μg/mL of PYC). The treatment time was 24 h. Each group contained four replicates.
The NO and MDA levels were quantified using commercial assay kits from Solarbio. The concentration of NO was determined using the Griess reagent. In short, 100 μL of cell lysis supernatant was combined with an equal volume of Griess reagent, containing a final concentration of 0.1% N-[1-naphthyl]ethylenediamine dihydrochloride in distilled water and 1% sulfanilamide in 5% phosphoric acid, in a 96-well flat-bottom plate. The absorbance was then measured at 550 nm after a 10-min incubation period. Finally, the content of NO was calculated from a standard curve plotted using sodium nitrite. The MDA concentration was assessed by TBA (Thiobarbituric Acid) method, and the reaction mixture included 100 μL of a cell lysis supernatant, 300 μL of MDA test fluid (containing TBA) and 100 μL of acetic acid. The mixed solution was incubated in a water bath at 100°C for 60 min, then tightly covered to prevent moisture loss. It was then cooled in an ice bath, centrifuged at 10,000 g for 10 min at room temperature. 200μL of supernatant was transferred to 96-well plate, and the absorbance of each sample at 532 nm was measured and the content of MDA can be estimated.
The total cellular RNA was obtained with the help of the UNIQ-10 column total RNA purification kit, per the manufacturer’s guide. cDNA was prepared using the HiFiScript cDNA synthesis kit, followed by qPCR assessment via a CFX Connect Real-Time PCR Detection System (Bio-Rad, United States) using using a total 20 μL reaction volume containing 10 μL of Magic SYBR Mixture (2×), 4 μL of each primer (2 μM), and 2 μL of cDNA. The -ΔΔCq formula was applied to identify the target genes (IL6 and IL1β) expression. β-actin was set as an endogenous control. All qPCR primers are as follows, β-actin (Sense: GGCTGTATTCCCCTCCATCG, Antisense: CCAGTTGGTAACAATGCCATGT), IL1β (Sense: GCAACTGTTCCTGAACTCAACT, Antisense: ATCTTTTGGGGTCCGTCAACT), IL6 (Sense: CTGCAAGAGACTTCCATCCAG, Antisense: AGTGGTATAGACAGGTCTGTTGG).
All assays were performed in quadruplicate, and the data are presented as mean ± standard error of the mean (SEM). The data assessment and statistical measurements were performed using the GraphPad Prism (9.0 version) software. The inter-group statistically significant variations were assessed using the one-way ANOVA followed by Duncan’s multiple range test. The significance threshold was set as p < 0.05.
The chemical components of PYC were characterized using UHPLC-MS/MS. The mixed quality control sample’s total ions current was the sum of all ion’s intensities in the mass spectrum at each time point versus time (Figure 2). A total of 768 compounds were analyzed (Supplementary Table SA1). The InflamNat database indicated >1,351 (up to 2021) natural compounds with anti-inflammatory activity (Zhang et al., 2022). Using the InflamNat database, 53 chemical components in PYC were identified with anti-inflammatory bioactivity. Components were screened using the TCMSP database based on the following criteria: DL ≥ 0.18, RBN ≤10, and OB ≥ 25%. After eliminating repetitions, 19 anti-inflammatory compounds in PYC were screened (Table 1; Figure 3).
HERB database identified 630 targets associated with the 19 anti-inflammatory compounds (Supplementary Table SA2). After duplicate removal, 251 unique targets remained for further analysis. From the GeneCards database, 607 inflammation-related targets were identified among 13,622 entries with a relevance score >5.0 (Supplementary Table SA3). Venn analysis identified 85 genes associated with PYC’s anti-inflammatory compounds and inflammation (Figure 4A), highlighting potential treatment targets. A network of 375 edges and 106 nodes was constructed to depict PYC-target-inflammation relationships (Figure 4B).
Figure 4. Targets of PYC’s anti-inflammatory compounds and inflammation. (A) Venn diagram of overlapping target genes between PYC’s anti-inflammatory compounds and inflammation. (B) PYC-compound-target-inflammation network. The yellow round rectangle nodes indicate the PYC’s anti-inflammatory compounds, the red hexagon node depicts inflammation, the green diamond node displays PYC, and the blue round rectangle nodes represent predicted PYC targets for treating inflammation.
The PPI data for the specified targets were retrieved from the STRING database and imported into Cytoscape 3.9.1 to construct a function-related PPI network. Figure 5A shows the PPI network, which consists of 2,193 edges and 85 nodes. The average node degree was 51.6, and the edge referred to the interaction of nodes. After assessing each node’s degree value (Supplementary Table SA4), the gene with a degree value ≥54 (median) was identified as the key gene target, and a total of 43 genes were screened out. The top 18 targets with ≥70-degree value included IL6, TNF, MMP9, IL1B, AKT1, IFNG, CXCL8, NFKB1, CCL2, IL10, PTGS2, ICAM1, IL1A, TGFB1, CASP3, HIF1A, JUN, STAT3 (Figure 5B; the horizontal coordinate is the degree value of each target point).
Figure 5. PPI network analysis. (A) PPI network reconstructed by Cytoscape. The node color and size are proportional to the target degree in the PPI network. (B) The degree values (≥70) of targets in the PPI network.
Enrichment analysis using the DAVID database identified 85 PYC targets involved in treating inflammation. GO enrichment comprises cellular component (CC), biological process (BP), and molecular function (MF). Bonferroni-corrected p-values < 0.05 revealed 114 BP, 14 CC, and 22 MF (Supplementary Table SA5). Each category’s top 10 GO entries were selected according to the FDR <0.05 (Figure 6). The BPs enrichment items included positive transcription regulation, inflammatory response, positive regulation of transcription from RNA polymerase II promoter, positive gene expression regulation, negative apoptosis regulation, positive DNA-template modulation of pri-miRNA transcription from RNA polymerase II promoter, etc. The CCs enrichment items comprised extracellular region, macromolecular complex, cytoplasm, extracellular space, membrane raft, etc. The MFs enrichment items contained enzyme binding, cytokine activity, identical protein binding, protein homodimerization activity, protease binding, etc.
KEGG pathway enrichment analysis identified 105 significant pathways with Bonferroni-corrected p-value < 0.05 (Supplementary Table SA6). These pathways were further classified into four major groups: six pathways of cellular processes, 21 of organismal systems, 13 of environmental information processing, and 65 were related to human disease (Figure 7). The pathways from the environmental information processing, organismal systems, and cellular processes groups were selected for the Sankey and dot plot chart: the IL17, TNF, C-type lectin receptor, Toll-like receptor, and NF-kappa B were the main signaling pathways associated with inflammation (Figure 8).
Figure 7. Classified enriched KEGG pathways of PYC candidate targets against inflammation. The pathways were classified into four major groups: human diseases, organismal systems, cellular processes, and environmental information processing.
To further elucidate and understand the PYC pharmacological mechanism against inflammation, a network of 19 anti-inflammatory compounds, 85 anti-inflammatory targets, and 40 signaling pathways was constructed (Figure 9). This network indicated that the PYC’s components could regulate various targets, which regulates different signaling pathways.
Figure 9. Network of compound-target-pathway. Green triangles, blue circles, and purple boxes represent compounds, targets, and pathways, respectively.
The cell viability of BV2 cells is depicted in Figure 10. When treating BV2 cells with different concentrations of PYC, compared to the blank, there was no significant difference in cell viability, with the highest viability occurring at 80 μg/mL. Compared to the blank group (100% viability), the viability of the model cells significantly decreased to 30.96% (p < 0.001) but improved following PYC treatment, as shown in the PYC group (Figure 10B).
MDA levels were significantly higher in the model cells than the controls but were markedly reduced after PYC and aspirin treatment (Figure 11A). Estimation of NO content and cytokines (IL1β and IL6) mRNA levels revealed significantly higher values in the model group compared to the control group. Furthermore, these levels were substantially decreased in the treatment group than in the model group (Figures 11B–D).
Figure 11. The effect of PYC on the levels of (A) Malondialdehyde (MDA), (B) NO, (C) IL1β mRNA, and (D) IL6 mRNA.
Inflammation is a physiological response of the immune system to irritation, injury, or infection by pathogens, autoimmune processes, or radiation exposure. Controlled inflammation is beneficial as it helps remove irritants, damaged cells, and pathogens (Nakkala et al., 2021). However, dysregulated uncontrolled inflammation can cause several chronic diseases, even death (Ma and Willey, 2022). Glucocorticoids, broad-spectrum anti-inflammatory agents, are used to treat inflammatory diseases but are associated with several adverse effects, including high blood pressure, hyperglycemia, and adrenal insufficiency (Sun et al., 2022). Aspirin is a nonsteroidal anti-inflammatory drug that is known to act by blocking cyclooxygenase activity (Nyambuya et al., 2023), but a variety of side effects can be associated with long-term aspirin use, which include varying degrees of damage to the gastrointestinal tract, liver, kidney, and cardiovascular system (Shi et al., 2023). Thus, finding agents with substantial anti-inflammatory effects and few side effects is important. As an herb, pine bark extract is traditionally used as an anti-inflammatory in Europe with few side effects (D'Andrea, 2010).
Like macrophages, microglia are vital immune system components, stimulating proinflammatory cytokines involved in inflammatory responses (Xiong et al., 2016; Zhang et al., 2021). In order to better demonstrate the anti-inflammatory activity of PYC, based on our previous cytotoxicity experiments, we chose the concentration of PYC as 80 μg/mL, which is above any concentration that can be expected in vivo (except for concentrations in the intestine). This study identified the inhibitory effects of PYC on the levels of NO and proinflammatory IL6 and IL1β cytokines in LPS-stimulated microglia (Figure 11). Network pharmacology analysis identified 19 anti-inflammatory compounds in PYC. PYC is recognized as one of the most powerful natural antioxidants, which is rich in flavonoids, phenolic acids and proanthocyanidins. The aqueous extract of PYC contains (+)-catechin, (−)-epicatechin, (+)-taxifolin, the benzoic acids protocatechuic acid, vanillic acid, gallic acid, the cinnamic acids, ferulic acid, caffeic acid, p-coumaric acid and higher molecular weight procyanidins predominate (Rohdewald, 2002; Bayer and Högger, 2024). Except for (+)-catechin, (−)-epicatechin, (+)-taxifolin and procyanidin b1, the other fifteen molecules we listed here are novel. While the compounds first reported here such as ellagic acid and quercetin which were a typical polyphenolic compound and flavonoid found in various plants (Vattem and Shetty, 2005; Singh et al., 2021). PYC is a bark extract of the French maritime pine (Pinus pinaster), hence the detection of these compounds is reasonable. The compounds listed here are related to anti-inflammatory ingredients after network pharmacology integrating and other detected chemicals of PYC extraction supernatant are not presented. Among them, 12 compounds (3′,4′,5,7-tetrahydroxyflavone, 3-methylquercetin, eriodictyol, procyanidin b1, epicatechin, (+) catechin, diosmetin, taxifolin, kaempferol, quercetin, oroxylin-a, and tricin) were flavonoids, tectorigenin, and formononetin were iso-flavonoids. Flavonoids comprise two benzene rings linked with a heterocyclic pyran or pyrone ring. These naturally occurring polyphenolic compounds have diverse biological activities. Compounds such as eriodictyol, procyanidin B1, epicatechin, (+)-catechin, taxifolin, kaempferol, quercetin, and tricin have demonstrated anti-inflammatory properties (Morikawa et al., 2006; Moscatelli et al., 2006; Hämäläinen et al., 2007). 3′,4′,5,7-tetrahydroxyflavone, known as luteolin, suppresses LPS-mediated inflammatory responses by modulating NF-κB/AP-1/PI3K-Akt signaling cascades (Park and song, 2013). Oroxylin-a suppresses LPS-mediated gene expression of COX-2 and iNOS by inhibiting NF-κB activation (Chen et al., 2000). Tectorigenin and formononetin, as isoflavonoids, can also display the inhibitory effects of NO release in LPS-activated mouse peritoneal macrophages (Matsuda et al., 2003). Ellagic acid, a phenolic acid, significantly inhibited NO in RAW 264.7 cells (Yang et al., 2011). Furthermore, Rhein can effectively suppress NO release and substantially reduce the expression of iNOS and COX-2 proteins after 18 h of LPS treatment (Choi et al., 2013). In RAW264.7 cells, cryptotanshinone significantly suppressed LPS-mediated NO generation and cytokines release like IL6 and TNFα (Gao et al., 2018). Auraptene and beta-sitosterol also showed anti-inflammatory effects (Morikawa et al., 2006; Nguyen et al., 2016). These studies further confirm the potential of the 19 anti-inflammatory compounds identified in PYC.
For the 19 identified anti-inflammatory compounds, 85 target genes were identified. PPI analysis and network pharmacology revealed core targets including IL6, TNF, MMP9, IL1B, AKT1, IFNG, CXCL8, NFKB1, CCL2, IL10, and PTGS2. Of these, IL6, TNF, IL1B, IFNG, CXCL8, and IL10 are the proinflammatory cytokines usually employed for assessing the anti-inflammatory effects of drugs (Xia et al., 2023). Studies have shown that levels of IL1β, IL6, and TNF are significantly associated with the intensity of systemic inflammation (Del Valle et al., 2020). MMP9, usually expressed in inflammatory cells, is a known activator of inflammatory cytokines like TNF and IL1β (Cheeran and Munuswamy-Ramanujam, 2020). AKT1, a significant subtype of serine/threonine kinase (AKT), regulates macrophage innate immunity by producing mitochondrial H2O2, and its levels are crucial for stimulating inflammatory reactions (Tang et al., 2017). NFKB1 is a pleiotropic transcription factor in almost all cell types and has been indicated as a crucial inflammatory regulator (Tu et al., 2020). CCL2 exerts its effect as an inflammatory chemokine, essential for recruiting leukocytes and memory T cells during inflammation (Ranjbar et al., 2022). PTGS2 expression is promoted during inflammation and regulates inflammatory responses by generating prostaglandins (Li et al., 2022). Usually, PYC can reduce inflammation by acting as a blocker of inflammatory transcription factors NF-kB and lowering the levels of MMP-9 (Grimm et al., 2006), which is consistent with our analysis results for the PYC’s target. When pycnogenol is administered, are lowered, displaying the inhibitory effect of pycnogenol on NF-kB All identified core targets were closely associated with the inflammation.
The KEGG and GO enrichment tests predicted the potential functions of the 85 targets and signaling pathways associated with the anti-inflammatory response of PYC. The GO analysis revealed that the BP of the targets was mainly involved in the inflammatory response, transcription regulation, and cell response; the CC affected by the targets included extracellular structures, and the MF of the targets was binding and cytokine activity (Figure 5A). Furthermore, it was indicated that PYC’s anti-inflammatory compounds are involved in various biological processes, cellular components, and molecular functions as defined in the GO categories. KEGG enrich analysis suggested that the anti-inflammatory compounds in PYC might exert their anti-inflammatory effect through the IL17 and TNF signal pathways. Interestingly, among the 11 core targets (IL6, TNF, MMP9, IL1B, AKT1, IFNG, CXCL8, NFKB1, CCL2, IL10 and PTGS2) analyzed by PPI, 10 genes except IL10 participated in IL17 signaling pathway. This pathway is initiated after the interaction of cytokine (IL17A/F, IL17F/F, or IL17A/A) with their receptors IL17RC and IL17RA, which activates TRAF proteins, which in turn stimulate C/EBPβ, NF-κB, C/EBPδ, and MAPK pathways, aggravating inflammation (Amatya et al., 2017). Contrasting with the IL17 signaling pathway, the TNF signaling pathway, a traditional proinflammatory pathway, involves six core targets: IL6, TNF, MMP9, IL1B, AKT1, and CCL2. Furthermore, it was found that PYC treatment reduced the expression of IL6 and IL1B, thereby alleviating inflammation.
Traditional anti-inflammatory agents usually act on a single target, which can lead to drug resistance during long-term use. For example, dexamethasone binds to the cytoplasmic GC receptor to form a heterodimer that translocated from the cytosol to the nucleus, where it transactivates or transrepresses target genes, leading to cell-cycle arrest and apoptosis (Zhang et al., 2023); aspirin is known to block cyclooxygenase activity (Nyambuya et al., 2023). Therefore, treatment of the chronic inflammation, dexamethasone and aspirin showed no effect (Thuo et al., 2022). Different from traditional anti-inflammatory agents, PYC could affect inflammation via multi-components, -targets and -mechnism, which avoid drug resistance and is suitable for long-term use. Moreover, The results shown in this in vitro study demonstrate that PYC was observed to be non-toxic shown by the cytotoxicity analyses in BV2 cells at the conctration below 100 μg/mL. Other studies also shown that no acute toxicity was identified with intraplantar injection of P. brutia bark extract into rat at a dose of 2000 mg/kg body wt (Ince et al., 2009). Therefore, PYC can be utilized as an anti-inflammatory agent.
Nevertheless, the study still presents certain limitations. Our research primarily centers on investigating the anti-inflammatory properties of the compounds found in PYC. We have solely examined the direct anti-inflammatory effects of these compounds in PYC, neglecting to explore the potential anti-inflammatory effects of their metabolites post-assimilation in the body. Consequently, a comprehensive understanding of the overall anti-inflammatory potential of PYC remains elusive, and and further in vivo and in vitro research need to be conducted.
This study systematically analyzed 768 chemical components in PYC using UPLC-MS/MS. After that, 19 anti-inflammatory compounds targeting 85 proteins directly involved in inflammation were identified using the InflamNat and TCMSP databases. Furthermore, PPI analysis indicated the core targets, including IL6, TNF, MMP9, IL1B, AKT1, IFNG, CXCL8, NFKB1, CCL2, IL10, and PTGS2. GO analysis indicated that these targets were involved in the BP, such as inflammatory response, transcription regulation, and cell response; they affected the CC, mainly the extracellular structures, and their MF included cytokine activity and binding. KEGG pathway analysis suggested that the anti-inflammatory compounds in PYC may exert their effects through the IL17 and TNF signaling pathways. Furthermore, it was found that PYC treatment reduced the expression of IL6 and IL1B, thereby alleviating inflammation. However, further research is required to elucidate the underlying mechanisms fully.
The original contributions presented in the study are included in the article/Supplementary Material, further inquiries can be directed to the corresponding author.
Ethical approval was not required for the studies on animals in accordance with the local legislation and institutional requirements because only commercially available established cell lines were used.
HL: Data curation, Investigation, Methodology, Writing–original draft. JS: Data curation, Investigation, Writing–original draft. FL: Data curation, Funding acquisition, Investigation, Methodology, Writing–original draft. LZ: Conceptualization, Methodology, Writing–original draft, Writing–review and editing.
The author(s) declare that financial support was received for the research, authorship, and/or publication of this article. This investigation was supported by the Natural Science Foundation of Shandong Province (Grant No. ZR2021QC020) and Shandong Provincial University Youth Innovation Team (2022KJ102).
The authors would like to thank all the reviewers who participated in the review and MJEditor (www.mjeditor.com) for their linguistic assistance while preparing this manuscript.
The authors declare that the research was conducted in the absence of any commercial or financial relationships that could be construed as a potential conflict of interest.
All claims expressed in this article are solely those of the authors and do not necessarily represent those of their affiliated organizations, or those of the publisher, the editors and the reviewers. Any product that may be evaluated in this article, or claim that may be made by its manufacturer, is not guaranteed or endorsed by the publisher.
The Supplementary Material for this article can be found online at: https://www.frontiersin.org/articles/10.3389/fphar.2024.1408304/full#supplementary-material
PYC, Pycnogenol; UPLC-MS/MS, Ultra-high performance liquid chromatography-tandem mass spectrometry; InflamNat, Inflammatory Natural Products Database and Predictor; TCMSP, Traditional Chinese Medicine Systems Pharmacology Database and Analysis Platform; PPI, Protein-Protein Interaction; KEGG, Kyoto Encyclopedia of Genes and Genomes; LPS, Lipopolysaccharide; CCK8, Cell Counting Kit 8; MDA, Malondialdehyde; NO, Nitric Oxide; DL, Drug-Likeness; OB, Oral Bioavailability; RBN, Rotatable Bond Number; HERB, High-throughput Experiment- and Reference-guided database of TCM; CTD, Comparative Toxicogenomics Database; GO, Gene Ontology; DAVID, Database for Annotation, Visualization, and Integrated Discovery; FDR, False Discovery Rate; OD, optical density; TBA, Thiobarbituric Acid; SEM, Standard Error Of The Mean.
Alderton, G., and Scanlon, S. T. (2021). Inflammation. Science 374, 1068–1069. doi:10.1126/science.abn1721
Amatya, N., Garg, A. V., and Gaffen, S. L. (2017). IL-17 signaling: the yin and the yang. Trends Immunol. 38, 310–322. doi:10.1016/j.it.2017.01.006
Bayer, J., and Högger, P. (2024). Review of the pharmacokinetics of French maritime pine bark extract (Pycnogenol®) in humans. Front. Nutr. 11, 1389422. doi:10.3389/fnut.2024.1389422
Cheeran, V., and Munuswamy-Ramanujam, G. (2020). Sesquiterpene lactone Zaluzanin D alters MMP-9 promoter methylation in differentiated THP-1 monocytic cells and down regulates inflammatory cytokines IL-1β and TNF-α. Int. Immunopharmacol. 87, 106803. doi:10.1016/j.intimp.2020.106803
Chen, Y. C., Yang, L. L., and Lee, T. J. F. (2000). Oroxylin A inhibition of lipopolysaccharide-induced iNOS and COX-2 gene expression via suppression of nuclear factor-kappaB activation. Biochem. Pharmacol. 59, 1445–1457. doi:10.1016/S0006-2952(00)00255-0
Choi, R. J., Ngoc, T. M., Bae, K., Cho, H. J., Kim, D. D., Chun, J., et al. (2013). Anti-inflammatory properties of anthraquinones and their relationship with the regulation of p-glycoprotein function and expression. Eur. J. Pharm. Sci. 48, 272–281. doi:10.1016/j.ejps.2012.10.027
Cordova, F. M., Marks, M. B. F., and Watson, R. R. (2013). “Chapter 39 - anti-inflammatory actions of pycnogenol: diabetes and arthritis,” in Bioactive food as dietary interventions for diabetes. Editors R. R. Watson, and V. R. Preedy (San Diego: Academic Press), 495–501.
D'Andrea, G. (2010). Pycnogenol: a blend of procyanidins with multifaceted therapeutic applications? Fitoterapia 81, 724–736. doi:10.1016/j.fitote.2010.06.011
D'Arcy, M. S. (2019). Cell death: a review of the major forms of apoptosis, necrosis and autophagy. Cell Biol. Int. 43, 582–592. doi:10.1002/cbin.11137
Del Valle, D. M., Kim-Schulze, S., Huang, H. H., Beckmann, N. D., Nirenberg, S., Wang, B., et al. (2020). An inflammatory cytokine signature predicts COVID-19 severity and survival. Nat. Med. 26, 1636–1643. doi:10.1038/s41591-020-1051-9
Gao, H. W., Huang, L. T., Ding, F., Yang, K., Feng, Y., Tang, H., et al. (2018). Simultaneous purification of dihydrotanshinone, tanshinone I, cryptotanshinone, and tanshinone IIA from Salvia miltiorrhiza and their anti-inflammatory activities investigation. Sci. Rep. 8, 8460. doi:10.1038/s41598-018-26828-0
Grimm, T., Chovanová, Z., Muchová, J., Sumegová, K., Liptáková, A., Duracková, Z., et al. (2006). Inhibition of NF-kappaB activation and MMP-9 secretion by plasma of human volunteers after ingestion of maritime pine bark extract (Pycnogenol). J. Inflamm. 3, 1–6. doi:10.1186/1476-9255-3-1
Hämäläinen, M., Nieminen, R., Vuorela, P., Heinonen, M., and Moilanen, E. (2007). Anti-inflammatory effects of flavonoids: genistein, kaempferol, quercetin, and daidzein inhibit STAT-1 and NF-kappaB activations, whereas flavone, isorhamnetin, naringenin, and pelargonidin inhibit only NF-kappaB activation along with their inhibitory effect on iNOS expression and NO production in activated macrophages. Mediat. Inflamm. 2007, 45673. doi:10.1155/2007/45673
Ince, I., Yesil-Celiktas, O., Karabay-Yavasoglu, N. U., and Elgin, G. (2009). Effects of Pinus brutia bark extract and Pycnogenol in a rat model of carrageenan induced inflammation. Phytomedicine 16, 1101–1104. doi:10.1016/j.phymed.2009.05.004
Langworth-Green, C., Patel, S., Jaunmuktane, Z., Jabbari, E., Morris, H., Thom, M., et al. (2023). Chronic effects of inflammation on tauopathies. Lancet. Neurology 22, 430–442. doi:10.1016/S1474-4422(23)00038-8
Li, R. L., Xie, J. R., Xu, W., Zhang, L., Lin, H., and Huang, W. (2022). LPS-induced PTGS2 manipulates the inflammatory response through trophoblast invasion in preeclampsia via NF-κB pathway. Reprod. Biol. 22, 100696. doi:10.1016/j.repbio.2022.100696
Ma, L., and Willey, J. (2022). The interplay between inflammation and thrombosis in COVID-19: mechanisms, therapeutic strategies, and challenges. Thromb. Update 8, 100117. doi:10.1016/j.tru.2022.100117
Maimoona, A., Naeem, I., Saddiqe, Z., and Jameel, K. (2011). A review on biological, nutraceutical and clinical aspects of French maritime pine bark extract. J. Ethnopharmacol. 133, 261–277. doi:10.1016/j.jep.2010.10.041
Matsuda, H., Morikawa, T., Ando, S., Toguchida, I., and Yoshikawa, M. (2003). Structural requirements of flavonoids for nitric oxide production inhibitory activity and mechanism of action. Bioorgan. Med. Chem. 11, 1995–2000. doi:10.1016/s0968-0896(03)00067-1
Morikawa, T., Xu, F. M., Matsuda, H., and Yoshikawa, M. (2006). Structures of new flavonoids, erycibenins D, E, and F, and NO production inhibitors from Erycibe expansa originating in Thailand. Chem. Pharm. Bull. 54, 1530–1534. doi:10.1248/cpb.54.1530
Moscatelli, V., Hnatyszyn, O., Acevedo, C., Megías, J., Alcaraz, M. J., and Ferraro, G. (2006). Flavonoids from Artemisia copa with anti-inflammatory activity. Planta. Med. 72, 72–74. doi:10.1055/s-2005-873177
Nakkala, J. R., Li, Z. M., Ahmad, W., Wang, K., and Gao, C. (2021). Immunomodulatory biomaterials and their application in therapies for chronic inflammation-related diseases. Acta. Biomater. 123, 1–30. doi:10.1016/j.actbio.2021.01.025
Nattagh-Eshtivani, E., Gheflati, A., Barghchi, H., Rahbarinejad, P., Hachem, K., Shalaby, M. N., et al. (2022). The role of Pycnogenol in the control of inflammation and oxidative stress in chronic diseases: molecular aspects. Phytother. Res. 36, 2352–2374. doi:10.1002/ptr.7454
Nguyen, P. H., Zhao, B. T., Kim, O., Lee, J. H., Choi, J. S., Min, B. S., et al. (2016). Anti-inflammatory terpenylated coumarins from the leaves of Zanthoxylum schinifolium with α-glucosidase inhibitory activity. J. Nat. Med.-Tokyo 70, 276–281. doi:10.1007/s11418-015-0957-x
Nigam, M., Mishra, A. P., Deb, V. K., Dimri, D. B., Tiwari, V., Bungau, S. G., et al. (2023). Evaluation of the association of chronic inflammation and cancer: insights and implications. Biomed. Pharmacother. 164, 115015. doi:10.1016/j.biopha.2023.115015
Norouzy, A., and Malekahmadi, M. (2023). “Chapter 10 - French maritime pine bark extract and neurological disorders,” in Treatments, nutraceuticals, supplements, and herbal medicine in neurological disorders. Editors C. R. Martin, V. B. Patel, and V. R. Preedy (Academic Press), 181–192.
Nyambuya, T. M., Dludla, P. V., Mxinwa, V., Mokgalaboni, K., Ngcobo, S. R., Tiano, L., et al. (2023). The impact of metformin and aspirin on T-cell mediated inflammation: a systematic review of in vitro and in vivo findings. Life Sci. 255, 117854. doi:10.1016/j.lfs.2020.117854
Park, C. M., and Song, Y. S. (2013). Luteolin and luteolin-7-O-glucoside inhibit lipopolysaccharide-induced inflammatory responses through modulation of NF- kappa B/AP-1/PI3K-Akt signaling cascades in RAW 264.7 cells. Nutr. Res. Pract. 7, 423–429. doi:10.4162/nrp.2013.7.6.423
Rafiyan, M., Sadeghmousavi, S., Akbarzadeh, M., and Rezaei, N. (2023). Experimental animal models of chronic inflammation. Curr. Res. Immunol. 4, 100063. doi:10.1016/j.crimmu.2023.100063
Ranjbar, M., Rahimi, A., Baghernejadan, Z., Ghorbani, A., and Khorramdelazad, H. (2022). Role of CCL2/CCR2 axis in the pathogenesis of COVID-19 and possible treatments: all options on the table. Int. Immunopharmacol. 113, 109325. doi:10.1016/j.intimp.2022.109325
Rohdewald, P. (2002). A review of the French maritime pine bark extract (Pycnogenol), a herbal medication with a diverse clinical pharmacology. Int. J. Clin. Pharm. 40, 158–168. doi:10.5414/cpp40158
Shi, Y., Zhang, R., Da, N., Wang, Y., Yang, J., Li, B., et al. (2023). Aspirin loaded extracellular vesicles inhibit inflammation of macrophages via switching metabolic phenotype in periodontitis. Biochem. Bioph. Res. Co. 667, 25–33. doi:10.1016/j.bbrc.2023.05.024
Shin, I. S., Shin, N. R., Jeon, C. M., Hong, J. M., Kwon, O. K., Kim, J. C., et al. (2013). Inhibitory effects of Pycnogenol® (French maritime pine bark extract) on airway inflammation in ovalbumin-induced allergic asthma. Food Chem. Toxicol. 62, 681–686. doi:10.1016/j.fct.2013.09.032
Singh, P., Arif, Y., Bajguz, A., and Hayat, S. (2021). The role of quercetin in plants. Plant Physiol. bioch. 166, 10–19. doi:10.1016/j.plaphy.2021.05.023
Sun, L., Wang, B. X., Sun, T., Zhou, F., Zhu, B., Li, C., et al. (2022). Investigation on the mechanism of 2,3,4 ',5-Tetrahydroxystilbene 2-o-D-glucoside in the treatment of inflammation based on network pharmacology. Comput. Biol. Med. 145, 105448. doi:10.1016/j.compbiomed.2022.105448
Tang, B. F., Li, X. C., Ren, Y. L., Wang, J., Xu, D., Hang, Y., et al. (2017). MicroRNA-29a regulates lipopolysaccharide (LPS)-induced inflammatory responses in murine macrophages through the Akt1/NF-kappa B pathway. Exp. Cell Res. 360, 74–80. doi:10.1016/j.yexcr.2017.08.013
Thuo, J. K. N., Towett, P. K., Kanui, T. I., and Abelson, K. S. P. (2022). Effects of dexamethasone and acetylsalicylic acid on inflammation caused by Complete Freund's adjuvant in the naked mole rat (Heterocephalus glaber). Heliyon 8 (2), e08920. doi:10.1016/j.heliyon.2022.e08920
Tu, Y. B., Wang, K., Tan, L. H., Han, B., Hu, Y., Ding, H., et al. (2020). Dolichosin A, a coumestan isolated from Glycine tabacina, inhibits IL-1β-induced inflammation in SW982 human synovial cells and suppresses RANKL-induced osteoclastogenesis: from network pharmacology to experimental pharmacology. J. Ethnopharmacol. 258, 112855. doi:10.1016/j.jep.2020.112855
Vattem, D. A., and Shetty, K. (2005). Biological functionality of ellagic acid: a review. J. Food Biochem. 29, 234–266. doi:10.1111/j.1745-4514.2005.00031.x
Wang, X., Zhou, W., Wang, Q., Zhang, Y., Ling, Y., Zhao, T., et al. (2021). A novel and comprehensive strategy for quality control in complex Chinese medicine formula using UHPLC-Q-Orbitrap HRMS and UHPLC-MS/MS combined with network pharmacology analysis: take Tangshen formula as an example. J. Chromatogr. B 1183, 122889. doi:10.1016/j.jchromb.2021.122889
Xia, Z. X., Li, Q., and Tang, Z. Y. (2023). Network pharmacology, molecular docking, and experimental pharmacology explored Ermiao wan protected against periodontitis via the PI3K/AKT and NF-Kappa B/MAPK signal pathways. J. Ethnopharmacol. 303, 115900. doi:10.1016/j.jep.2022.115900
Xian, X., Chunyan, L., Canyu, W., Huiqing, Z., Liaofan, S., Xiaolong, L., et al. (2023). Hypoglycemic effect and active ingredients screening of Isodon Japonicus based on network pharmacology and experimental validation. Arab. J. Chem. 16, 105108. doi:10.1016/j.arabjc.2023.105108
Xiong, X. Y., Liu, L., and Yang, Q. W. (2016). Functions and mechanisms of microglia/macrophages in neuroinflammation and neurogenesis after stroke. Prog. Neurobiol. 142, 23–44. doi:10.1016/j.pneurobio.2016.05.001
Yang, Z. G., Jia, L. N., Shen, Y., Ohmura, A., and Kitanaka, S. (2011). Inhibitory effects of constituents from Euphorbia lunulata on differentiation of 3T3-L1 cells and nitric oxide production in RAW264.7 cells. Molecules 16, 8305–8318. doi:10.3390/molecules16108305
Zhang, R. H., Ren, S. P., Dai, Q., Shen, T., Li, X., Li, J., et al. (2022). InflamNat: web-based database and predictor of anti-inflammatory natural products. J. Cheminformatics 14, 30. doi:10.1186/s13321-022-00608-5
Zhang, W., Tian, T., Gong, S. X., Huang, W. Q., Zhou, Q. Y., Wang, A. P., et al. (2021). Microglia-associated neuroinflammation is a potential therapeutic target for ischemic stroke. Neural. Regen. Res. 16, 6–11. doi:10.4103/1673-5374.286954
Keywords: anti-inflammation, network pharmacology, pycnogenol, UPLC-MS/MS, molecular mechainsm
Citation: Liu H, Shi J, Liu F and Zhang L (2024) Integrating network pharmacology and experimental verification to reveal the anti-inflammatory ingredients and molecular mechanism of pycnogenol. Front. Pharmacol. 15:1408304. doi: 10.3389/fphar.2024.1408304
Received: 28 March 2024; Accepted: 06 June 2024;
Published: 26 June 2024.
Edited by:
Ying-Yu Cui, Tongji University, ChinaReviewed by:
Laiba Arshad, Forman Christian College, PakistanCopyright © 2024 Liu, Shi, Liu and Zhang. This is an open-access article distributed under the terms of the Creative Commons Attribution License (CC BY). The use, distribution or reproduction in other forums is permitted, provided the original author(s) and the copyright owner(s) are credited and that the original publication in this journal is cited, in accordance with accepted academic practice. No use, distribution or reproduction is permitted which does not comply with these terms.
*Correspondence: Litao Zhang, emhhbmdsaXRhb0BtYWlsLmpubWMuZWR1LmNu
Disclaimer: All claims expressed in this article are solely those of the authors and do not necessarily represent those of their affiliated organizations, or those of the publisher, the editors and the reviewers. Any product that may be evaluated in this article or claim that may be made by its manufacturer is not guaranteed or endorsed by the publisher.
Research integrity at Frontiers
Learn more about the work of our research integrity team to safeguard the quality of each article we publish.