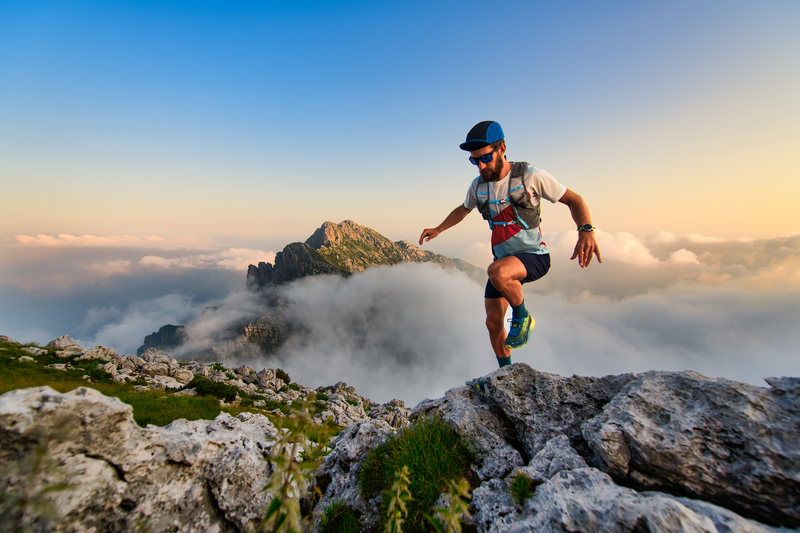
95% of researchers rate our articles as excellent or good
Learn more about the work of our research integrity team to safeguard the quality of each article we publish.
Find out more
ORIGINAL RESEARCH article
Front. Pharmacol. , 27 August 2024
Sec. Respiratory Pharmacology
Volume 15 - 2024 | https://doi.org/10.3389/fphar.2024.1407825
Objective: This study aimed to elucidate the prognostic significance of serum soluble thrombomodulin (sTM), lung ultrasound score (LUS), and lactate levels in patients with extrapulmonary acute respiratory distress syndrome (ARDS), with the goal of refining mortality risk prediction in this cohort.
Methods: In a prospective cohort of 95 patients with extrapulmonary ARDS admitted to the intensive care unit, we investigated the primary endpoint of 28-day mortality. Utilizing Lasso-Cox regression analysis, we identified independent prognostic factors for mortality. A predictive nomogram was developed incorporating these factors, and its performance was validated through several statistical measures, including the consistency index, calibration plot, internal validation curve, decision curve analysis, interventions avoided analysis, receiver operating characteristic curve analysis, and Kaplan-Meier survival analysis. We further conducted a subgroup analysis to examine the impact of prone positioning on patient outcomes.
Results: The study identified baseline serum sTM, LUS, and lactate levels as independent predictors of 28-day mortality in extrapulmonary ARDS patients. The predictive nomogram demonstrated superior prognostic accuracy compared to the use of sTM, LUS, or lactate levels alone, and outperformed traditional prognostic tools such as the Acute Physiology and Chronic Health Evaluation II score and the partial pressure of arterial oxygen to fractional inspired oxygen ratio. The subgroup analysis did not show a significant impact of prone positioning on the predictive value of the identified biomarkers.
Conclusion: Our study results support the development and validation of a novel prognostic nomogram that integrates key clinical biomarkers and ultrasound imaging scores to predict mortality in patients with extrapulmonary ARDS. While our research is preliminary, further studies and validation are required.
Acute respiratory distress syndrome (ARDS) is a clinical syndrome marked by an acute onset and refractory hypoxemia, primarily resulting from damage to capillary endothelial cells and alveolar epithelial cells (Suresh et al., 2000). ARDS poses a significant challenge in intensive care units (ICUs). Approximately 10.4% of ICU patients rapidly develop ARDS, with a mortality rate approaching 40% (Bellani et al., 2016), and an estimated 40% of ARDS cases may go undiagnosed (Laffey et al., 2016). Current therapeutic strategies for ARDS are limited, primarily relying on lung-protective ventilation (Peltan et al., 2023), fluid management (Ingelse et al., 2019), prone positioning (PP) (Al Hashim et al., 2023), and adjunctive anti-inflammatory and supportive therapies. While extracorporeal membrane oxygenation (ECMO) and lung transplantation are options for refractory ARDS (Cerier and Bharat, 2023; Majithia-Beet et al., 2023), they are costly and associated with high mortality rates. In recent years, stem cell therapy has shown promise for ARDS treatment, but it has not yet translated into effective clinical protocols (Lin et al., 2023). Consequently, early identification and proactive intervention for ARDS patients with poor prognosis are critical to improving survival rates.
ARDS is a highly heterogeneous syndrome, characterized by differences in etiology, pathology, pathophysiology, respiratory mechanics, and biomarkers (Viswan et al., 2020; Liao et al., 2023; Xu et al., 2023). Based on the underlying causes, ARDS can be categorized into pulmonary ARDS and extrapulmonary ARDS. Pulmonary ARDS typically results from direct lung injuries such as pneumonia or aspiration, whereas extrapulmonary ARDS is often caused by systemic diseases like pancreatitis, trauma, or sepsis (Bernard et al., 1994). The primary distinction between pulmonary and extrapulmonary ARDS lies in whether the injury originates from alveolar epithelial cells or vascular endothelial cells (Shaver and Bastarache, 2014). Studies have highlighted differences between these two types in terms of pathology (Negri et al., 2002; Menezes et al., 2005), respiratory mechanics (Gattinoni et al., 1998), response to positive end-expiratory pressure (PEEP) (Tugrul et al., 2003), response to PP (Lim et al., 2001), and radiographic imaging on chest CT (Goodman et al., 1999). The heterogeneity of ARDS complicates its diagnosis and treatment. Consequently, it is imperative to develop predictive models tailored to specific etiologies to enhance the accuracy and efficacy of clinical interventions.
Biomarkers are measurable indicators of normal or pathological processes or responses to therapeutic interventions, frequently used as auxiliary indices in clinical practice. Previous research has investigated the predictive and prognostic value of biomarkers in ARDS (Samanta et al., 2018; Leija-Martinez et al., 2020). ARDS can be induced by various pulmonary and extrapulmonary diseases, leading to distinct pathophysiological changes. Biomarkers may exhibit heterogeneity due to these differences in primary etiologies. For instance, biomarkers differ between pulmonary ARDS and extrapulmonary ARDS (Chen et al., 2013). Therefore, it is crucial to ensure that study populations are homogeneous when investigating ARDS biomarkers. Additionally, the pathogenesis of ARDS is extremely intricate, with various pathophysiological alterations frequently overlapping temporally and spatially. This overlap leads to considerable heterogeneity and variability in biomarkers (Cartin-Ceba et al., 2015), thereby constraining the relevance and value of research focusing on single biomarkers (Levitt and Rogers, 2016). Compared to single biomarkers, the combined use of multiple biomarkers is more advantageous for the early diagnosis, classification, and prognostic evaluation of ARDS (Cartin-Ceba et al., 2015; Bos et al., 2017; Negrin et al., 2017). However, studies on the combined use of biomarkers in ARDS are limited and have not yet reached clinical applicability (Ware et al., 2017; Ge et al., 2023). Additionally, some biomarkers are not routinely measured in clinical practice, and the feasibility of implementing multi-biomarker predictive methods in clinical settings is constrained. Therefore, targeting biomarkers most relevant to pathophysiology, combined with clinical diagnostic indicators and other clinical characteristic factors, may help predict and evaluate the prognosis of ARDS mediated by specific primary diseases and mechanisms.
This study aims to analyze patients with extrapulmonary ARDS, utilizing early clinical data that can be rapidly obtained to identify characteristic factors. These factors will be combined with protein biomarkers indicative of pulmonary endothelial injury and clinical diagnostic indicators reflecting pulmonary pathophysiological status. The objective of this study is to construct and validate a nomogram model to provide valuable references for the clinical diagnosis and treatment of ARDS.
This investigation enrolled participants admitted to the ICUs at the Central Theater General Hospital of the People’s Liberation Army of China and Hefei BOE Hospital from June 2019 to June 2022. We included individuals meeting the following criteria: 1) diagnosed with moderate to severe ARDS according to the 2012 Berlin criteria, displaying a partial pressure of arterial oxygen to fractional inspired oxygen (PaO2/FiO2) ratio ≤ 200 mmHg under a standardized ventilation regime (Force et al., 2012); 2) ARDS originating from indirect lung injury causes, including but not limited to non-pulmonary sepsis, trauma not involving the lungs, adverse reactions to blood transfusions, pancreatitis, among others; 3) diagnosis of ARDS confirmed within the initial 24 h following ICU admission. The exclusion criteria encompassed: 1) age below 18 or above 85 years; 2) pregnancy; 3) history of pulmonary tuberculosis, lung cancer, severe chronic obstructive pulmonary disease (COPD), chronic interstitial lung disease, or prior lung surgery; 4) presence of massive pleural effusion or pneumothorax; 5) cardiogenic pulmonary edema or hypoxemia primarily due to cardiac conditions; 6) inadequate acoustic window for ultrasound imaging; 7) inability to ascertain clinical outcomes within the study’s observation period.
This study was conducted in strict accordance with ethical principles and received approval from the review boards of the two participating hospital institutions (Approval No: 201910216; 20190010). Prior to enrollment, written informed consent was obtained from the legal guardians of the patients. All patient information was anonymized before analysis to ensure confidentiality.
ARDS was identified following the Berlin Definition criteria, which necessitate: an acute inception, evident bilateral infiltrates on chest radiographs not fully explained by effusions, lobar/lung collapse, or nodules; a non-cardiogenic origin as indicated by the absence of left atrial hypertension; and a PaO2/FiO2 ratio less than 300 mmHg (Force et al., 2012). Distinctively, extrapulmonary ARDS results from systemic factors that precipitate vascular endothelial damage, enhance pulmonary vascular permeability, lead to interstitial and alveolar exudation, and culminate in alveolar collapse, edema, and respiratory failure (Bernard et al., 1994).
Upon enrollment, we systematically collected comprehensive baseline clinical data for each participant. This encompassed demographic and physiological characteristics, the etiological factors contributing to ARDS, and any pre-existing health conditions. Within the first 24 h post-enrollment, we meticulously recorded vital signs, laboratory findings, assessed the severity of the disease using the Acute Physiology and Chronic Health Evaluation (APACHE) II scoring system, and determined the degree of oxygenation impairment via the PaO2/FiO2 ratio. These initial assessments provided insight into the patients’ baseline health status and the immediate therapeutic interventions initiated upon study entry.
Demographic data included age, sex, body mass index (BMI), and smoking history. The causative factors of ARDS were categorized into distinct groups: non-pulmonary sepsis, non-pulmonary trauma, complications arising from multiple blood transfusions, pancreatitis, and other causes. We also compiled an extensive list of pre-existing conditions, such as hypertension, coronary artery disease, diabetes mellitus, and cerebrovascular diseases.
Vital signs included mean arterial pressure, heart rate, respiratory rate, and body temperature. The laboratory parameters assessed encompassed hemoglobin concentration, white blood cell count, platelet count, serum creatinine, total bilirubin, albumin levels, troponin T, NT-proBNP, D-dimer, lactate, procalcitonin, C-reactive protein, and electrolytes (sodium and potassium). The APACHE II score was calculated to assess the initial severity of the illness (Knaus et al., 1985), while the PaO2/FiO2 ratio (Force et al., 2012) provided an index of the baseline level of pulmonary oxygenation dysfunction.
Therapeutic interventions initiated within the first 24 h of patient enrollment were meticulously recorded. These included the application of mechanical ventilation, the adoption of PP, the administration of vasoactive drugs, sedation, neuromuscular blocking agents, systemic corticosteroids, antibiotics, antifungal treatments, continuous renal replacement therapy, and venovenous ECMO (VV ECMO). These management strategies were individualized based on each patient’s clinical status and underlying conditions, in alignment with current best practice guidelines and clinical protocols.
All study participants received care in the ICU adhering to globally recognized ARDS management guidelines and consensus documents. Initial respiratory support was provided using non-invasive or invasive mechanical ventilation (NIV or IMV, respectively) upon patient enrollment. Transition from NIV to IMV was considered under specific conditions such as a high Simplified Acute Physiological Score (>34), PaO2/FiO2 ratio ≤ 175 mmHg (indicating severe hypoxemia) (Antonelli et al., 2007), lack of hypoxemia improvement after 1 h of NIV, or excessive spontaneous breathing leading to large tidal volumes (>9 mL/kg) (Frat et al., 2018).
For patients on IMV, a lung-protective ventilation strategy was employed aiming to optimize oxygenation and minimize ventilator-induced lung injury. Specific targets included maintaining peripheral capillary oxygen saturation between 88% and 95%, PaO2 levels of 55–80 mmHg, partial pressure of arterial carbon dioxide (PaCO2) ≤ 50 mmHg or pH ≥ 7.25, plateau pressure (Pplat) ≤ 30 cmH2O, and driving pressure (∆P) ≤ 15 cmH2O (Khemani et al., 2018). Tidal volume was initially set at 6 mL/kg of predicted body weight and adjusted based on Pplat and ∆P assessments. PEEP levels were set according to FiO2 requirements and subsequently fine-tuned based on Pplat, ∆P, and PaCO2 levels. Respiratory rate adjustments were made to manage PaCO2 and pH levels effectively. PP was implemented for durations exceeding 12 h per day in cases of persistent acidosis (pH < 7.25), PaCO2 > 50 mmHg, or when the PaO2/FiO2 ratio was ≤150 mmHg despite a PEEP ≥ 5 cmH2O, unless contraindicated (Fan et al., 2017; Fichtner et al., 2018). Neuromuscular blockade was utilized in scenarios of unresolving hypoxemia, during PP, or to prevent ventilator-induced injuries, in line with recent rapid practice guidelines (Alhazzani et al., 2020).
VV ECMO was considered for patients with severe respiratory failure unresponsive to mechanical ventilation if specific criteria were met, including a treatment duration under 7 days with FiO2 ≥ 0.80, a PaO2/FiO2 ratio < 50 mmHg for over 3 h or <80 mmHg for over 6 h, pH < 7.25 with PaCO2 ≥ 60 mmHg for more than 6 h, respiratory rates ≥ 35 min, or Pplat ≤ 32 cmH2O (Tonna et al., 2021).
Fluid management and vasoactive drug administration were tailored by the attending physician based on hemodynamic status, ARDS severity, and cardiac and renal function. In cases of concurrent sepsis, fluid and vasopressor strategies followed sepsis-specific guidelines (Singer et al., 2016). Corticosteroid use adhered to guidelines addressing critical illness-associated corticosteroid insufficiency and international sepsis guidelines (Singer et al., 2016; Pastores et al., 2018). Additionally, nutritional support and venous thromboembolism prophylaxis were provided in accordance with critical care guidelines and consensus statements (Di Nisio et al., 2016; Burgos et al., 2018).
LUS examinations were conducted within the initial 24 h post-enrollment. The pulmonary regions were systematically partitioned into twelve segments via anatomical delineations, which included the parasternal, anterior axillary, posterior axillary, posterior median lines, and the interscapular line. Utilizing a phased array convex transducer (frequency range: 3.5–10 MHz, manufactured by GE Healthcare, United States), scanning was meticulously performed across these predefined segments. Pulmonary ultrasound findings were quantified based on a four-point scale reflecting the extent of ventilatory compromise: 1) Score 0 indicating normal aeration evidenced by lung sliding and the presence of A-lines or fewer than three discrete B-lines; 2) Score 1 for moderate aeration loss, characterized by the appearance of three or more scattered B-lines; 3) Score 2 denoting severe ventilatory impairment with confluent B-lines; 4) Score 3 corresponding to pulmonary consolidation, identifiable by tissue-like echotexture and bronchogram signs. A composite score ranging from 0 to 36 was then computed, providing an aggregate measure of pulmonary aeration deficit (Mongodi et al., 2017).
Serum samples for sTM quantification were obtained within the first 24 h following patient enrollment. Concentrations of sTM were determined employing a dual-antibody sandwich enzyme-linked immunosorbent assay, specifically the kit supplied by Abcam (catalogue number ab46508, Shanghai, China). All procedures were rigorously conducted in adherence to the manufacturer-provided protocol.
The principal outcome was assessed as all-cause mortality within a 28-day interval post-admission. Patients were subsequently categorized into two distinct cohorts predicated on their 28-day survival outlook: those who survived (survival group) and those who did not (non-survival group).
Data analyses were executed using standardized statistical methodologies. Continuous variables underwent normality testing through the Shapiro-Wilk test, alongside Bartlett’s test for assessing variance homogeneity. Variables adhering to normal distribution were articulated as mean ± standard deviation, with inter-group discrepancies evaluated via the independent samples t-test. Conversely, variables deviating from normal distribution were presented as median (interquartile range, IQR), with the Mann-Whitney U test facilitating inter-group comparisons. Categorical variables were represented as percentages (%) and analyzed using the chi-square test. Univariate Cox regression analysis was employed for variables deemed clinically pertinent and demonstrating significant variances between survival outcomes. Lasso regression was utilized for risk factor identification, with the Schoenfeld residual test confirming the proportional hazard (PH) assumption post Lasso-Cox regression. A prognostic nomogram was formulated based on Lasso-Cox regression outcomes, its predictive accuracy gauged through the concordance index (C-index), calibration plots, internal validation, decision curve analysis (DCA), interventions avoided analysis (IAA), and receiver operating characteristic (ROC) analysis. The area under the ROC curve (AUC) was used to determine the predictive accuracy of each factor, and the DeLong test was employed to compare the AUCs. Patient survival probabilities were delineated via the Kaplan-Meier method. Data processing was accomplished using SPSS version 22.0 and STATA version 17.0, with statistical significance set at a p-value < 0.05 (two-tailed).
During the recruitment phase, 113 individuals were identified as eligible according to the inclusion criteria, as depicted in Figure 1. However, 18 participants were subsequently excluded due to reasons including age constraints (below 18 or above 85 years), the presence of severe pre-existing pulmonary conditions or recent thoracic surgeries, significant pleural effusion or pneumothorax, cardiogenic pulmonary edema, inadequate echogenicity for ultrasound examination, and the inability to ascertain clinical outcomes within the study’s observational timeframe. Consequently, the study proceeded with 95 participants, comprising 32 individuals in the non-survival cohort (33.68%) and 63 in the survival cohort (66.32%).
The baseline demographic and clinical characteristics, including the etiology of ARDS and pre-existing health conditions, are summarized in Table 1. Comparative analysis revealed no statistically significant disparities between survivors and non-survivors concerning age, gender distribution, BMI, and smoking history (p > 0.05 for all comparisons). Similarly, the underlying causes of ARDS, spanning non-pulmonary sepsis, trauma, multiple transfusions, pancreatitis, among others, showed no significant variance between the two groups (p > 0.05 for all etiologies). Furthermore, the prevalence of pre-existing conditions such as hypertension, coronary artery disease, diabetes, and cerebrovascular diseases was comparable between survivors and non-survivors (p > 0.05 for all conditions).
Table 1. General characteristics, ARDS etiology, and preexisting medical conditions of patients with extrapulmonary ARDS between survivors and non-survivors.
Table 2 elucidates the vital signs, laboratory findings, APACHE II scores, and PaO2/FiO2 ratios assessed within the initial 24 h post-enrollment. Noteworthy disparities were observed in platelet count (184.30 vs. 141.15, p = 0.017), serum creatinine levels (153.10 vs. 182.76, p = 0.046), and lactate levels (4.20 vs. 5.35, p = 0.001) between survivors and non-survivors, indicating a significantly more compromised physiological status in the latter group. Additionally, the APACHE II scores were significantly lower (21.52 ± 2.68 vs. 23.28 ± 2.63, p = 0.003), and PaO2/FiO2 ratios were notably higher (119.14 ± 14.02 vs. 109.47 ± 11.04, p = 0.001) in the survival cohort, suggesting a less severe clinical presentation and better oxygenation status at baseline.
Table 2. Vital signs, laboratory findings, APACHE II score, PaO2/FiO2 ratio, and treatments of patients with extrapulmonary ARDS between survivors and non-survivors.
An in-depth comparison of therapeutic interventions administered within the first 24 h of enrollment is presented in Table 2. There was no statistical significance in the application of mechanical ventilation, positive pressure support, vasoactive agents, sedation, neuromuscular blockade, systemic corticosteroids, antibiotic and antifungal treatments, continuous blood purification, and VV ECMO between the two groups (p > 0.05 for all treatment modalities).
The baseline levels of sTM and LUS were compared between patients who survived and those who did not survive in order to investigate potential differences related to patient outcomes. Table 3 illustrates that survivors exhibited significantly lower serum sTM levels and LUS compared to non-survivors (all p < 0.05).
Table 3. sTM levels and LUS of patients with extra-pulmonary ARDS between survivors and non-survivors.
Univariate Cox regression analysis was conducted to identify potential predictors for 28-day mortality. The analysis revealed that sTM level [odds ratio (OR) = 1.030, 95% confidence interval (CI) 1.018–1.042, p < 0.001], LUS (OR = 1.255, 95% CI 1.122–1.404, p < 0.001), APACHE II score (OR = 1.232, 95% CI 1.073–1.415, p = 0.003), PaO2/FiO2 ratio (OR = 0.959, 95% CI 0.934–0.985, p = 0.002), platelet count (OR = 0.994, 95% CI 0.988–0.999, p = 0.028), creatinine (OR = 1.005, 95% CI 1.001–1.009, p = 0.026), and lactate (OR = 1.212, 95% CI 1.073–1.369, p = 0.002) were significantly associated with 28-day mortality (Table 4).
Table 4. Univariate Cox regression analysis of risk factors for 28-day mortality in patients with extra-pulmonary ARDS.
Subsequently, Lasso regression analysis was employed to further refine the predictive variables. The parameter path was estimated over a range of values for λ, as depicted in Figure 2A. Through iterative analysis and 10-fold crossvalidation, the optimal value of λ was determined to be 0.095, resulting in a model with exceptional performance and minimal variables (Figure 2B). The final screened variables included sTM, LUS, and lactate.
Figure 2. Identification and screening of prognostic variables. (A) The parameter path was estimated over a range of values for λ. (B) The selection process of the optimal value of the parameter λ in the Lasso regression model using cross-validation. The number of variables selected was three. (C) sTM, LUS, and lactate met the PH assumption. sTM, serum soluble thrombomodulin; LUS, lung ultrasound score.
To ensure the validity of the model, the baseline levels of sTM, LUS, and lactate were subjected to the proportional risk hypothesis test based on Schoenfeld residuals (Figure 2C). The results showed that all variables met the PH assumptions (sTM, p = 0.407; LUS, p = 0.672; lactate, p = 0.066). Therefore, all three variables were utilized in the predictive modeling for 28-day mortality.
The constructed nomogram visually represents the impact of sTM, LUS, and lactate on patient survival outcomes. The “total points” projection provides an estimation of the probability of 28-day mortality based on the combination of these variables (Figure 3A), facilitating informed clinical decision-making. The median C-index for the nomogram was 0.807 (0.740–0.873), indicating excellent predictive accuracy. Calibration curve analysis demonstrated strong agreement between predicted and observed outcomes (Figure 3B), reinforcing the reliability of the nomogram. Internal validation of the nomogram yielded a C-index of 0.875, confirming its robustness (Figure 3C).
Figure 3. Development and validation of a predictive nomogram for forecasting 28-day overall survival. (A) The nomogram for predicting the 28-day survival probability of patients with extrapulmonary ARDS. The nomogram comprised three variables, namely sTM, LUS, and lactate. (B) The calibration curve of the nomogram. (C) The internal validation of the nomogram. sTM, serum soluble thrombomodulin; LUS, lung ultrasound score; CI, confidence interval.
To evaluate the clinical utility of the predictive model, DCA and IAA were performed. Figure 4A illustrates the clinical effectiveness of the nomogram predictive model as assessed by DCA, demonstrating a higher net benefit for clinical decision-making within a threshold probability range of 0–0.71. Figure 4B shows that the IAA analysis indicates a net reduction in unnecessary interventions within a threshold probability range of 0–0.73. Table 5 presents the ROC analysis of the model’s predictive performance. The nomogram demonstrated significantly superior predictive ability for 28-day mortality compared to individual factors such as sTM, LUS, and lactate, with an AUC of 0.873 (95% CI 0.789–0.932). The DeLong test confirmed that the AUC differences between the nomogram and each individual factor were statistically significant (all p < 0.05). Additionally, the nomogram exhibited higher sensitivity (87.50%) and specificity (77.78%) in predicting 28-day mortality compared to these individual factors. Figure 4C visually illustrates the AUC values obtained from the ROC analysis. Furthermore, the Kaplan-Meier survival analysis, depicted in Table 6, indicated a significantly lower overall survival rate in the high-risk group compared to the low-risk group (66.67% vs. 7.55%, p < 0.001). The mean survival time was also substantially shorter for the high-risk group (17.69 days vs. 27.13 days, 95% CI 15.19–20.19 days vs. 26.29–27.97 days, p < 0.001). Figure 4D illustrates the Kaplan-Meier survival curves for the high-risk and low-risk groups.
Figure 4. Validation of a predictive nomogram for forecasting 28-day overall survival. (A) Decision curve analysis of sTM, LUS, lactate, and the nomogram. (B) Analysis of avoided interventions for sTM, LUS, lactate, and the nomogram. (C) ROC curves of sTM, LUS, lactate, and the nomogram for predicting 28-day mortality. (D) Kaplan-Meier 28-day survival curve of patients with varying cut-off values of the nomogram. sTM, serum soluble thrombomodulin; LUS, lung ultrasound score; AUC, area under curve.
Table 5. Youden index analyses of sTM, LUS, lactate, and the nomogram for predicting 28-day mortality.
Table 6. Comparison of 28-day mortality and survival status in patients with varying cut-off values of the nomogram.
In clinical practice, the APACHE II score is a widely accepted prognostic tool for assessing the severity of critical illness, while the PaO2/FiO2 ratio is a reliable indicator for evaluating the severity of ARDS. This study conducted a comparative analysis to assess the predictive accuracy of the combined nomogram versus traditional models, particularly the APACHE II score and PaO2/FiO2 ratio.
ROC analysis was employed to evaluate and compare the predictive accuracy of these models. As shown in Table 7, the nomogram achieved a higher AUC than both the APACHE II score (AUC 0.676, 95% CI 0.572–0.768) and the PaO2/FiO2 ratio (AUC 0.704, 95% CI 0.602–0.794). The DeLong test confirmed that the differences in AUC between the nomogram and each individual factor were statistically significant (all p < 0.05). Additionally, the nomogram showed superior sensitivity and specificity for predicting 28-day mortality compared to the APACHE II score (sensitivity 50.00%, specificity 76.19%) and the PaO2/FiO2 ratio (sensitivity 78.12%, specificity 60.32%). Figure 5 provides a visual representation of the AUC values for these models.
Table 7. Youden index analyses of APACHE II score, PaO2/FiO2 ratio, and nomogram for predicting 28-day mortality in patients with extra-pulmonary ARDS.
Figure 5. Comparison of AUC between the combined predictive nomogram and the traditional predictive models, including APACHE II score and PaO2/FiO2 ratio. APACHE II score, Acute Physiology and Chronic Health Evaluation II score; PaO2/FiO2 ratio, partial pressure of arterial oxygen to fractional inspired oxygen ratio; AUC, area under curve.
The efficacy of PP in enhancing the clinical outcomes of patients afflicted with ARDS has been well-documented in the literature (Guerin et al., 2013). Nonetheless, the introduction of medical interventions during the observation period can potentially introduce confounding factors, thereby affecting the predictive accuracy of our combined nomogram. To mitigate this issue and elucidate the specific impact of PP on clinical outcomes, we conducted a subgroup analysis, categorizing patients based on their exposure to PP during the study period. Patients were thus classified into two groups: those who did not undergo PP treatment (non-PP group) and those who received PP treatment (PP group).
At the commencement of the study, comparative analysis of baseline characteristics, specifically sTM, LUS, and lactate levels, between the PP and non-PP groups revealed no statistically significant differences, as delineated in Table 8. Subsequent stratification based on PP exposure facilitated a more nuanced analysis of the relationship between these biomarkers and patient outcomes. Table 9 delineates the differential baseline levels of sTM, LUS, and lactate between patients who survived and those who did not, following stratification by PP status.
Table 8. sTM, LUS, and lactate of patients with extra-pulmonary ARDS between non-PP and PP patients.
Moreover, Table 10 articulates the findings derived from both univariate and multivariate Cox regression analyses, examining the association between sTM, LUS, lactate levels, and 28-day mortality subsequent to stratification by PP. These analyses were meticulously adjusted for potential confounders, including the APACHE II score, PaO2/FiO2 ratio, platelet count, and creatinine levels. Intriguingly, our analysis revealed that, upon adjusting for these confounding factors, the associations of sTM, LUS, and lactate levels with 28-day mortality were no longer statistically significant among patients stratified by PP.
Table 10. Univariate and multivariate Cox regression analysis of sTM, LUS and lactate for predicting 28-day mortality in subgroups.
Due to the highly heterogeneous nature of ARDS, developing reliable diagnostic tests and specific drug treatments is significantly challenging. To enhance the predictive accuracy for extrapulmonary ARDS, this study aims to establish a multiparameter predictive model. We selected sTM as a protein biomarker indicative of pulmonary endothelial injury and the LUS as a clinical diagnostic indicator of pulmonary pathophysiology. Additionally, we gathered a comprehensive set of clinical characteristics, including demographics, underlying causes of ARDS, comorbidities, physical findings, laboratory results, clinical scores, and therapeutic interventions. Using univariate Cox regression and Lasso regression analyses, we identified sTM and LUS as independent risk factors for ARDS mortality. Among the various clinical characteristics evaluated, only lactate levels were found to be an independent risk factor for ARDS mortality.
Thrombomodulin (TM) is an endothelial-specific transmembrane protein that can be cleaved into its soluble form (sTM) during endothelial activation or injury (Boehme et al., 1996). TM plays a critical role in the negative regulation of coagulation and inflammation (Ito and Maruyama, 2011). Given the abundant expression of sTM in the lungs and its detectability in serum (Kawanami et al., 2000), sTM serves as a biomarker for endothelial cell damage in the lungs. Elevated serum sTM levels indicate a reduction in the anticoagulant and anti-inflammatory capacities of pulmonary microvascular endothelial cells (Hofstra et al., 2008; Yang et al., 2014), which may exacerbate endothelial cell damage and create a vicious cycle. The potential of sTM as a serum biomarker for ARDS has garnered significant attention, with recent studies exploring its utility in predicting the onset and prognosis of ARDS (Orwoll et al., 2015; Sapru et al., 2015). Further research indicates that patients with extrapulmonary ARDS exhibit significantly elevated levels of sTM compared to those with pulmonary ARDS (Orwoll et al., 2015), suggesting that circulating sTM is more relevant for this subgroup rather than the predominantly pulmonary ARDS cases found in ICUs. Additionally, within the subcategories of extrapulmonary ARDS, those associated with non-pulmonary sepsis show markedly higher sTM levels compared to trauma-induced ARDS or ARDS of other etiologies (Garcia-Alvarez et al., 2014). This increase in sTM is also linked to higher mortality rates. Notably, although COVID-19-related ARDS is classified as pulmonary ARDS, it also presents with elevated serum sTM levels, with critically ill patients exhibiting significant and sustained increases (Sb et al., 2024). This indicates that endothelial injury is a prominent feature in the pathogenesis of severe COVID-19-related ARDS. These findings suggest that sTM, which reflects pulmonary endothelial activation, may serve as a valuable biomarker for identifying specific ARDS biophenotypes. This can aid in recognizing subgroups with similar pathophysiological characteristics. It is important to note that sTM is not routinely measured in clinical practice, and not all laboratories have the capability to quantify its levels.
Lung ultrasound has proven to be highly valuable in diagnosing ARDS. The global definition of ARDS incorporates ultrasound diagnostic criteria from the Kigali definition (Matthay et al., 2024). Study by Smit et al. (2023) has shown that the LUS method demonstrates greater accuracy in diagnosing and excluding ARDS compared to the modified Kigali method. LUS involves dividing both lungs into sections, with the commonly used method being the 12-zone technique. This approach assesses the extent of ventilation loss in both lungs by calculating the total score for all zones. Since lung heat dilution evaluates LUS in direct relation to extravascular lung water and quantitative computed tomography assesses LUS in direct relation to overall lung tissue density (Corradi et al., 2013), LUS serves as a semi-quantitative indicator of aeration status. An elevated LUS reflects alveolar ventilation loss and increased pulmonary shunting, the primary causes of severe hypoxemia in ARDS patients. LUS has been utilized in evaluating the diagnosis, treatment, and prognosis of ARDS patients (Pisani et al., 2019; Sayed et al., 2022; Smit et al., 2023; Huai and Ye, 2024). Due to its non-invasive and bedside nature, ultrasound presents unique advantages in resource-limited settings and during the implementation of prone positioning ventilation (Rousset et al., 2021; Mayo et al., 2022). However, comprehensive lung ultrasound training is essential before it can be effectively used as a predictive tool in clinical practice.
Lactate levels are often regarded as a reliable indicator of disease severity in critically ill patients (Zhang et al., 2022). Lactate is a metabolic byproduct of anaerobic glycolysis, and elevated lactate levels typically indicate increased production under hypoxic or ischemic conditions, reflecting a mismatch between production and clearance (Wardi et al., 2020). In the ICU, various types of shock often lead to elevated lactate levels due to reduced systemic tissue perfusion, an imbalance between oxygen supply and demand, and cellular hypoxia (Vincent et al., 2016). Additionally, in critically ill patients, elevated catecholamine levels and the production of multiple cytokines can promote glycolysis even under non-hypoxic conditions, resulting in hyperlactatemia (Haji-Michael et al., 1999). It is noteworthy that during ARDS, the lungs can release lactate at rates exceeding 60 mmol/h, far surpassing normal levels (Opdam and Bellomo, 2000; Iscra et al., 2002). This release is significantly correlated with the severity of lung injury, likely due to the promotion of glycolysis in lung tissues by inflammatory cells and cytokines (Brown et al., 1996; Haji-Michael et al., 1999). Therefore, when interpreting elevated serum lactate levels in the presence of systemic tissue hypoperfusion and ARDS, it is essential to consider the contribution of pulmonary lactate release alongside systemic hypoxia. Given that lactate levels are influenced by multiple factors, including hypoxia, tissue perfusion deficits, glucose metabolism disturbances, and liver and kidney function (Li et al., 2022), a single measurement of lactate concentration can be challenging (Weinberger et al., 2021). Currently, research on the prognostic value of lactate in ARDS patients is limited.
Using Lasso-Cox regression analysis, our study developed a nomogram based on sTM, LUS, and lactate levels. The analysis demonstrated that the nomogram incorporating these factors had higher predictive value for 28-day mortality in extrapulmonary ARDS patients compared to models using single indicators. Additionally, the nomogram outperformed traditional models such as APACHE II score and PaO2/FiO2 ratio. The combined use of sTM, LUS, and lactate provides a comprehensive assessment of pulmonary vascular endothelial damage, uneven dead space ventilation, pulmonary shunting, and the overall severity of the patient’s condition.
Several limitations of our study should be acknowledged. Firstly, the relatively small sample size is insufficient for robustly testing the performance of the predictive score and may have restricted the identification of other potential confounding factors that could impact the predictive value of our combined model for 28-day mortality. Secondly, the lack of external validation limits the applicability of our findings. Future studies should include external validation cohorts to verify the robustness of our predictive model. Thirdly, there may have been selection bias in identifying factors related to patient prognosis, which could have influenced the results.
In summary, we identified three significant factors distinguishing survivors from non-survivors among patients with extrapulmonary ARDS admitted to the ICU. We developed a composite nomogram aimed at predicting the 28-day mortality rate in patients with extrapulmonary ARDS. Further studies with larger sample sizes are required to validate these findings and refine the predictive model.
The raw data supporting the conclusions of this article will be made available by the authors, without undue reservation.
The studies involving humans were approved by the Ethics Committee of Hefei BOE Hospital and Central Theater General Hospital. The studies were conducted in accordance with the local legislation and institutional requirements. The participants provided their written informed consent to participate in this study.
YY: Conceptualization, Data curation, Investigation, Writing–original draft. YW: Investigation, Methodology, Project administration, Writing–original draft. GZ: Data curation, Investigation, Methodology, Writing–review and editing. SX: Data curation, Software, Writing–review and editing. JL: Project administration, Resources, Supervision, Writing–review and editing. ZT: Resources, Software, Validation, Visualization, Writing–review and editing.
The author(s) declare that no financial support was received for the research, authorship, and/or publication of this article.
Thanks to Professor Hongwei Jiang in the Department of statistics, Tongji Medical College, Huazhong University of Science and Technology for his comments and advice on this study. He has no responsibility for the content of the manuscript.
Authors YY, JL and YW were employed by Hefei BOE Hospital Co., Ltd.
The remaining authors declare that the research was conducted in the absence of any commercial or financial relationships that could be construed as a potential conflict of interest.
All claims expressed in this article are solely those of the authors and do not necessarily represent those of their affiliated organizations, or those of the publisher, the editors and the reviewers. Any product that may be evaluated in this article, or claim that may be made by its manufacturer, is not guaranteed or endorsed by the publisher.
Al Hashim, A. H., Al-Zakwani, I., Al Jadidi, A., Al Harthi, R., Al Naabi, M., Biyappu, R., et al. (2023). Early prone versus supine positioning in moderate to severe coronavirus disease 2019 patients with acute respiratory distress syndrome. Oman Med. J. 38, e465. doi:10.5001/omj.2023.52
Alhazzani, W., Belley-Cote, E., Moller, M. H., Angus, D. C., Papazian, L., Arabi, Y. M., et al. (2020). Neuromuscular blockade in patients with ARDS: a rapid practice guideline. Intensive Care Med. 46, 1977–1986. doi:10.1007/s00134-020-06227-8
Antonelli, M., Conti, G., Esquinas, A., Montini, L., Maggiore, S. M., Bello, G., et al. (2007). A multiple-center survey on the use in clinical practice of noninvasive ventilation as a first-line intervention for acute respiratory distress syndrome. Crit. Care Med. 35, 18–25. doi:10.1097/01.CCM.0000251821.44259.F3
Bellani, G., Laffey, J. G., Pham, T., Fan, E., Brochard, L., Esteban, A., et al. (2016). Epidemiology, patterns of care, and mortality for patients with acute respiratory distress syndrome in intensive care units in 50 countries. JAMA 315, 788–800. doi:10.1001/jama.2016.0291
Bernard, G. R., Artigas, A., Brigham, K. L., Carlet, J., Falke, K., Hudson, L., et al. (1994). The American-European Consensus Conference on ARDS. Definitions, mechanisms, relevant outcomes, and clinical trial coordination. Am. J. Respir. Crit. Care Med. 149, 818–824. doi:10.1164/ajrccm.149.3.7509706
Boehme, M. W., Deng, Y., Raeth, U., Bierhaus, A., Ziegler, R., Stremmel, W., et al. (1996). Release of thrombomodulin from endothelial cells by concerted action of TNF-alpha and neutrophils: in vivo and in vitro studies. Immunology 87, 134–140.
Bos, L. D., Schouten, L. R., Van Vught, L. A., Wiewel, M. A., Ong, D. S. Y., Cremer, O., et al. (2017). Identification and validation of distinct biological phenotypes in patients with acute respiratory distress syndrome by cluster analysis. Thorax 72, 876–883. doi:10.1136/thoraxjnl-2016-209719
Brown, S. D., Clark, C., and Gutierrez, G. (1996). Pulmonary lactate release in patients with sepsis and the adult respiratory distress syndrome. J. Crit. Care 11, 2–8. doi:10.1016/s0883-9441(96)90014-3
Burgos, R., Breton, I., Cereda, E., Desport, J. C., Dziewas, R., Genton, L., et al. (2018). ESPEN guideline clinical nutrition in neurology. Clin. Nutr. 37, 354–396. doi:10.1016/j.clnu.2017.09.003
Cartin-Ceba, R., Hubmayr, R. D., Qin, R., Peters, S., Determann, R. M., Schultz, M. J., et al. (2015). Predictive value of plasma biomarkers for mortality and organ failure development in patients with acute respiratory distress syndrome. J. Crit. Care 30, 219.e1–219.e2197. doi:10.1016/j.jcrc.2014.09.001
Cerier, E. J., and Bharat, A. (2023). Lung transplantation for coronavirus disease 2019 acute respiratory distress syndrome/fibrosis: silver lining of a global pandemic. Curr. Opin. Organ Transpl. 28, 157–162. doi:10.1097/MOT.0000000000001068
Chen, X., Shan, Q., Jiang, L., Zhu, B., and Xi, X. (2013). Quantitative proteomic analysis by iTRAQ for identification of candidate biomarkers in plasma from acute respiratory distress syndrome patients. Biochem. Biophys. Res. Commun. 441, 1–6. doi:10.1016/j.bbrc.2013.09.027
Corradi, F., Ball, L., Brusasco, C., Riccio, A. M., Baroffio, M., Bovio, G., et al. (2013). Assessment of extravascular lung water by quantitative ultrasound and CT in isolated bovine lung. Respir. Physiol. Neurobiol. 187, 244–249. doi:10.1016/j.resp.2013.04.002
Di Nisio, M., Van Es, N., and Buller, H. R. (2016). Deep vein thrombosis and pulmonary embolism. Lancet 388, 3060–3073. doi:10.1016/S0140-6736(16)30514-1
Fan, E., Del Sorbo, L., Goligher, E. C., Hodgson, C. L., Munshi, L., Walkey, A. J., et al. (2017). An official American thoracic society/European society of intensive care medicine/society of critical care medicine clinical practice guideline: mechanical ventilation in adult patients with acute respiratory distress syndrome. Am. J. Respir. Crit. Care Med. 195, 1253–1263. doi:10.1164/rccm.201703-0548ST
Fichtner, F., Moerer, O., Laudi, S., Weber-Carstens, S., Nothacker, M., Kaisers, U., et al. (2018). Mechanical ventilation and extracorporeal membrane oxygena tion in acute respiratory insufficiency. Dtsch. Arztebl Int. 115, 840–847. doi:10.3238/arztebl.2018.0840
Force, A. D. T., Ranieri, V. M., Rubenfeld, G. D., Thompson, B. T., Ferguson, N. D., Caldwell, E., et al. (2012). Acute respiratory distress syndrome: the Berlin Definition. JAMA 307, 2526–2533.
Frat, J. P., Ragot, S., Coudroy, R., Constantin, J. M., Girault, C., Prat, G., et al. (2018). Predictors of intubation in patients with acute hypoxemic respiratory failure treated with a noninvasive oxygenation strategy. Crit. Care Med. 46, 208–215. doi:10.1097/CCM.0000000000002818
Garcia-Alvarez, M., Marik, P., and Bellomo, R. (2014). Sepsis-associated hyperlactatemia. Crit. Care 18, 503. doi:10.1186/s13054-014-0503-3
Gattinoni, L., Pelosi, P., Suter, P. M., Pedoto, A., Vercesi, P., and Lissoni, A. (1998). Acute respiratory distress syndrome caused by pulmonary and extrapulmonary disease. Different syndromes? Am. J. Respir. Crit. Care Med. 158, 3–11. doi:10.1164/ajrccm.158.1.9708031
Ge, R., Wang, F., and Peng, Z. (2023). Advances in biomarkers for diagnosis and treatment of ARDS. Diagn. (Basel) 13, 3296. doi:10.3390/diagnostics13213296
Goodman, L. R., Fumagalli, R., Tagliabue, P., Tagliabue, M., Ferrario, M., Gattinoni, L., et al. (1999). Adult respiratory distress syndrome due to pulmonary and extrapulmonary causes: CT, clinical, and functional correlations. Radiology 213, 545–552. doi:10.1148/radiology.213.2.r99nv42545
Guerin, C., Reignier, J., Richard, J. C., Beuret, P., Gacouin, A., Boulain, T., et al. (2013). Prone positioning in severe acute respiratory distress syndrome. N. Engl. J. Med. 368, 2159–2168. doi:10.1056/NEJMoa1214103
Haji-Michael, P. G., Ladriere, L., Sener, A., Vincent, J. L., and Malaisse, W. J. (1999). Leukocyte glycolysis and lactate output in animal sepsis and ex vivo human blood. Metabolism 48, 779–785. doi:10.1016/s0026-0495(99)90179-8
Hofstra, J. J., Juffermans, N. P., Schultz, M. J., and Zweers, M. M. (2008). Pulmonary coagulopathy as a new target in lung injury--a review of available pre-clinical models. Curr. Med. Chem. 15, 588–595. doi:10.2174/092986708783769696
Huai, J., and Ye, X. (2024). Lung ultrasound evaluation of aeration changes in response to prone positioning in acute respiratory distress syndrome (ARDS) patients requiring venovenous extracorporeal membrane oxygenation: an observational study. Cureus 16, e55554. doi:10.7759/cureus.55554
Ingelse, S. A., Juschten, J., Maas, M. W., Matute-Bello, G., Juffermans, N. P., Van Woensel, J. B. M., et al. (2019). Fluid restriction reduces pulmonary edema in a model of acute lung injury in mechanically ventilated rats. PLoS One 14, e0210172. doi:10.1371/journal.pone.0210172
Iscra, F., Gullo, A., and Biolo, G. (2002). Bench-to-bedside review: lactate and the lung. Crit. Care 6, 327–329. doi:10.1186/cc1519
Ito, T., and Maruyama, I. (2011). Thrombomodulin: protectorate God of the vasculature in thrombosis and inflammation. J. Thromb. Haemost. 9 (Suppl. 1), 168–173. doi:10.1111/j.1538-7836.2011.04319.x
Kawanami, O., Jin, E., Ghazizadeh, M., Fujiwara, M., Jiang, L., Nagashima, M., et al. (2000). Heterogeneous distribution of thrombomodulin and von Willebrand factor in endothelial cells in the human pulmonary microvessels. J. Nippon. Med. Sch. 67, 118–125. doi:10.1272/jnms.67.118
Khemani, R. G., Parvathaneni, K., Yehya, N., Bhalla, A. K., Thomas, N. J., and Newth, C. J. L. (2018). Positive end-expiratory pressure lower than the ARDS network protocol is associated with higher pediatric acute respiratory distress syndrome mortality. Am. J. Respir. Crit. Care Med. 198, 77–89. doi:10.1164/rccm.201707-1404OC
Knaus, W. A., Draper, E. A., Wagner, D. P., and Zimmerman, J. E. (1985). Apache II: a severity of disease classification system. Crit. Care Med. 13, 818–829. doi:10.1097/00003246-198510000-00009
Laffey, J. G., Bellani, G., Pham, T., Fan, E., Madotto, F., Bajwa, E. K., et al. (2016). Potentially modifiable factors contributing to outcome from acute respiratory distress syndrome: the LUNG SAFE study. Intensive Care Med. 42, 1865–1876. doi:10.1007/s00134-016-4571-5
Leija-Martinez, J. J., Huang, F., Del-Rio-Navarro, B. E., Sanchez-Munoz, F., Munoz-Hernandez, O., Giacoman-Martinez, A., et al. (2020). IL-17A and TNF-α as potential biomarkers for acute respiratory distress syndrome and mortality in patients with obesity and COVID-19. Med. Hypotheses 144, 109935. doi:10.1016/j.mehy.2020.109935
Levitt, J. E., and Rogers, A. J. (2016). Proteomic study of acute respiratory distress syndrome: current knowledge and implications for drug development. Expert Rev. Proteomics 13, 457–469. doi:10.1586/14789450.2016.1172481
Li, X., Yang, Y., Zhang, B., Lin, X., Fu, X., An, Y., et al. (2022). Lactate metabolism in human health and disease. Signal Transduct. Target Ther. 7, 305. doi:10.1038/s41392-022-01151-3
Liao, Q., Pu, Y., Jin, X., Zhuang, Z., Xu, X., Ren, X., et al. (2023). Physiological and clinical variables identify ARDS classes and therapeutic heterogeneity to glucocorticoids: a retrospective study. BMC Pulm. Med. 23, 92. doi:10.1186/s12890-023-02384-w
Lim, C. M., Kim, E. K., Lee, J. S., Shim, T. S., Lee, S. D., Koh, Y., et al. (2001). Comparison of the response to the prone position between pulmonary and extrapulmonary acute respiratory distress syndrome. Intensive Care Med. 27, 477–485. doi:10.1007/s001340000848
Lin, K. C., Fang, W. F., Sung, P. H., Huang, K. T., Chiang, J. Y., Chen, Y. L., et al. (2023). Early and dose-dependent xenogeneic mesenchymal stem cell therapy improved outcomes in acute respiratory distress syndrome rodent through ameliorating inflammation, oxidative stress, and immune reaction. Cell Transpl. 32, 9636897231190178. doi:10.1177/09636897231190178
Majithia-Beet, G., Naemi, R., and Issitt, R. (2023). Efficacy of outcome prediction of the respiratory ECMO survival prediction score and the predicting death for severe ARDS on VV-ECMO score for patients with acute respiratory distress syndrome on extracorporeal membrane oxygenation. Perfusion 38, 1340–1348. doi:10.1177/02676591221115267
Matthay, M. A., Arabi, Y., Arroliga, A. C., Bernard, G., Bersten, A. D., Brochard, L. J., et al. (2024). A new global definition of acute respiratory distress syndrome. Am. J. Respir. Crit. Care Med. 209, 37–47. doi:10.1164/rccm.202303-0558WS
Mayo, P. H., Chew, M., Doufle, G., Mekontso-Dessap, A., Narasimhan, M., and Vieillard-Baron, A. (2022). Machines that save lives in the intensive care unit: the ultrasonography machine. Intensive Care Med. 48, 1429–1438. doi:10.1007/s00134-022-06804-z
Menezes, S. L., Bozza, P. T., Neto, H. C., Laranjeira, A. P., Negri, E. M., Capelozzi, V. L., et al. (2005). Pulmonary and extrapulmonary acute lung injury: inflammatory and ultrastructural analyses. J. Appl. Physiol. (1985) 98, 1777–1783. doi:10.1152/japplphysiol.01182.2004
Mongodi, S., Bouhemad, B., Orlando, A., Stella, A., Tavazzi, G., Via, G., et al. (2017). Modified lung ultrasound score for assessing and monitoring pulmonary aeration. Ultraschall Med. 38, 530–537. doi:10.1055/s-0042-120260
Negri, E. M., Hoelz, C., Barbas, C. S., Montes, G. S., Saldiva, P. H., and Capelozzi, V. L. (2002). Acute remodeling of parenchyma in pulmonary and extrapulmonary ARDS. An autopsy study of collagen-elastic system fibers. Pathol. Res. Pract. 198, 355–361. doi:10.1078/0344-0338-00266
Negrin, L. L., Halat, G., Kettner, S., Gregori, M., Ristl, R., Hajdu, S., et al. (2017). Club cell protein 16 and cytokeratin fragment 21-1 as early predictors of pulmonary complications in polytraumatized patients with severe chest trauma. PLoS One 12, e0175303. doi:10.1371/journal.pone.0175303
Opdam, H., and Bellomo, R. (2000). Oxygen consumption and lactate release by the lung after cardiopulmonary bypass and during septic shock. Crit. Care Resusc. 2, 181–187. doi:10.1016/s1441-2772(23)02156-7
Orwoll, B. E., Spicer, A. C., Zinter, M. S., Alkhouli, M. F., Khemani, R. G., Flori, H. R., et al. (2015). Elevated soluble thrombomodulin is associated with organ failure and mortality in children with acute respiratory distress syndrome (ARDS): a prospective observational cohort study. Crit. Care 19, 435. doi:10.1186/s13054-015-1145-9
Pastores, S. M., Annane, D., and Rochwerg, B.Corticosteroid Guideline Task Force of SCCM and ESICM (2018). Guidelines for the diagnosis and management of critical illness-related corticosteroid insufficiency (CIRCI) in critically ill patients (Part II): society of critical care medicine (SCCM) and European society of intensive care medicine (ESICM) 2017. Crit. Care Med. 46, 146–148. doi:10.1097/CCM.0000000000002840
Peltan, I. D., Knighton, A. J., Barney, B. J., Wolfe, D., Jacobs, J. R., Klippel, C., et al. (2023). Delivery of lung-protective ventilation for acute respiratory distress syndrome: a hybrid implementation-effectiveness trial. Ann. Am. Thorac. Soc. 20, 424–432. doi:10.1513/AnnalsATS.202207-626OC
Pisani, L., Vercesi, V., Van Tongeren, P. S. I., Lagrand, W. K., Leopold, S. J., Huson, M. M., et al. (2019). The diagnostic accuracy for ARDS of global versus regional lung ultrasound scores - a post hoc analysis of an observational study in invasively ventilated ICU patients. Intensive Care Med. Exp. 7, 44. doi:10.1186/s40635-019-0241-6
Rousset, D., Sarton, B., Riu, B., Bataille, B., and Silva, S.PLUS Study Group (2021). Bedside ultrasound monitoring of prone position induced lung inflation. Intensive Care Med. 47, 626–628. doi:10.1007/s00134-021-06347-9
Samanta, J., Singh, S., Arora, S., Muktesh, G., Aggarwal, A., Dhaka, N., et al. (2018). Cytokine profile in prediction of acute lung injury in patients with acute pancreatitis. Pancreatology 18, 878–884. doi:10.1016/j.pan.2018.10.006
Sapru, A., Calfee, C. S., Liu, K. D., Kangelaris, K., Hansen, H., Pawlikowska, L., et al. (2015). Plasma soluble thrombomodulin levels are associated with mortality in the acute respiratory distress syndrome. Intensive Care Med. 41, 470–478. doi:10.1007/s00134-015-3648-x
Sayed, M. S., Elmeslmany, K. A., Elsawy, A. S., and Mohamed, N. A. (2022). The validity of quantifying pulmonary contusion extent by lung ultrasound score for predicting ARDS in blunt thoracic trauma. Crit. Care Res. Pract. 2022, 3124966. doi:10.1155/2022/3124966
Sb, B. M., Chacko, B., Selvarajan, S., Peter, J. V., Geevar, T., Dave, R. G., et al. (2024). Biomarkers of coagulation, endothelial, platelet function, and fibrinolysis in patients with COVID-19: a prospective study. Sci. Rep. 14, 2011. doi:10.1038/s41598-024-51908-9
Shaver, C. M., and Bastarache, J. A. (2014). Clinical and biological heterogeneity in acute respiratory distress syndrome: direct versus indirect lung injury. Clin. Chest Med. 35, 639–653. doi:10.1016/j.ccm.2014.08.004
Singer, M., Deutschman, C. S., Seymour, C. W., Shankar-Hari, M., Annane, D., Bauer, M., et al. (2016). The third international consensus definitions for sepsis and septic shock (Sepsis-3). JAMA 315, 801–810. doi:10.1001/jama.2016.0287
Smit, M. R., Hagens, L. A., Heijnen, N. F. L., Pisani, L., Cherpanath, T. G. V., Dongelmans, D. A., et al. (2023). Lung ultrasound prediction model for acute respiratory distress syndrome: a multicenter prospective observational study. Am. J. Respir. Crit. Care Med. 207, 1591–1601. doi:10.1164/rccm.202210-1882OC
Suresh, R., Kupfer, Y., and Tessler, S. (2000). Acute respiratory distress syndrome. N. Engl. J. Med. 343, 660–661. doi:10.1056/NEJM200008313430914
Tonna, J. E., Abrams, D., Brodie, D., Greenwood, J. C., Rubio Mateo-Sidron, J. A., Usman, A., et al. (2021). Management of adult patients supported with venovenous extracorporeal membrane oxygenation (VV ECMO): guideline from the extracorporeal life support organization (ELSO). ASAIO J. 67, 601–610. doi:10.1097/MAT.0000000000001432
Tugrul, S., Akinci, O., Ozcan, P. E., Ince, S., Esen, F., Telci, L., et al. (2003). Effects of sustained inflation and postinflation positive end-expiratory pressure in acute respiratory distress syndrome: focusing on pulmonary and extrapulmonary forms. Crit. Care Med. 31, 738–744. doi:10.1097/01.CCM.0000053554.76355.72
Vincent, J. L., Quintairos, E. S. A., Couto, L., and Taccone, F. S. (2016). The value of blood lactate kinetics in critically ill patients: a systematic review. Crit. Care 20, 257. doi:10.1186/s13054-016-1403-5
Viswan, A., Singh, C., Kayastha, A. M., Azim, A., and Sinha, N. (2020). An NMR based panorama of the heterogeneous biology of acute respiratory distress syndrome (ARDS) from the standpoint of metabolic biomarkers. NMR Biomed. 33, e4192. doi:10.1002/nbm.4192
Wardi, G., Brice, J., Correia, M., Liu, D., Self, M., and Tainter, C. (2020). Demystifying lactate in the emergency department. Ann. Emerg. Med. 75, 287–298. doi:10.1016/j.annemergmed.2019.06.027
Ware, L. B., Zhao, Z., Koyama, T., Brown, R. M., Semler, M. W., Janz, D. R., et al. (2017). Derivation and validation of a two-biomarker panel for diagnosis of ARDS in patients with severe traumatic injuries. Trauma Surg. Acute Care Open 2, e000121. doi:10.1136/tsaco-2017-000121
Weinberger, J., Klompas, M., and Rhee, C. (2021). What is the utility of measuring lactate levels in patients with sepsis and septic shock? Semin. Respir. Crit. Care Med. 42, 650–661. doi:10.1055/s-0041-1733915
Xu, H., Sheng, S., Luo, W., Xu, X., and Zhang, Z. (2023). Acute respiratory distress syndrome heterogeneity and the septic ARDS subgroup. Front. Immunol. 14, 1277161. doi:10.3389/fimmu.2023.1277161
Yang, S. M., Ka, S. M., Wu, H. L., Yeh, Y. C., Kuo, C. H., Hua, K. F., et al. (2014). Thrombomodulin domain 1 ameliorates diabetic nephropathy in mice via anti-NF-κB/NLRP3 inflammasome-mediated inflammation, enhancement of NRF2 antioxidant activity and inhibition of apoptosis. Diabetologia 57, 424–434. doi:10.1007/s00125-013-3115-6
Keywords: extrapulmonary acute respiratory distress syndrome, mortality, soluble thrombomodulin, lung ultrasound score, lactate, nomogram
Citation: Yang Y, Wang Y, Zhu G, Xu S, Liu J and Tang Z (2024) Developing a predictive nomogram for mortality in patients with extrapulmonary acute respiratory distress syndrome: the prognostic value of serum soluble thrombomodulin, lung ultrasound score, and lactate. Front. Pharmacol. 15:1407825. doi: 10.3389/fphar.2024.1407825
Received: 27 March 2024; Accepted: 02 August 2024;
Published: 27 August 2024.
Edited by:
Riccardo Inchingolo, Fondazione Policlinico Universitario A. Gemelli IRCCS, ItalyReviewed by:
Santosh Prajapati, University of South Florida, United StatesCopyright © 2024 Yang, Wang, Zhu, Xu, Liu and Tang. This is an open-access article distributed under the terms of the Creative Commons Attribution License (CC BY). The use, distribution or reproduction in other forums is permitted, provided the original author(s) and the copyright owner(s) are credited and that the original publication in this journal is cited, in accordance with accepted academic practice. No use, distribution or reproduction is permitted which does not comply with these terms.
*Correspondence: Jie Liu, MTM3MjAzMzU3OTBAMTYzLmNvbQ==; Zhongzhi Tang, ancxMjkzOTEzNjEyQDE2My5jb20=
†These authors have contributed equally to this work and share first authorship
Disclaimer: All claims expressed in this article are solely those of the authors and do not necessarily represent those of their affiliated organizations, or those of the publisher, the editors and the reviewers. Any product that may be evaluated in this article or claim that may be made by its manufacturer is not guaranteed or endorsed by the publisher.
Research integrity at Frontiers
Learn more about the work of our research integrity team to safeguard the quality of each article we publish.