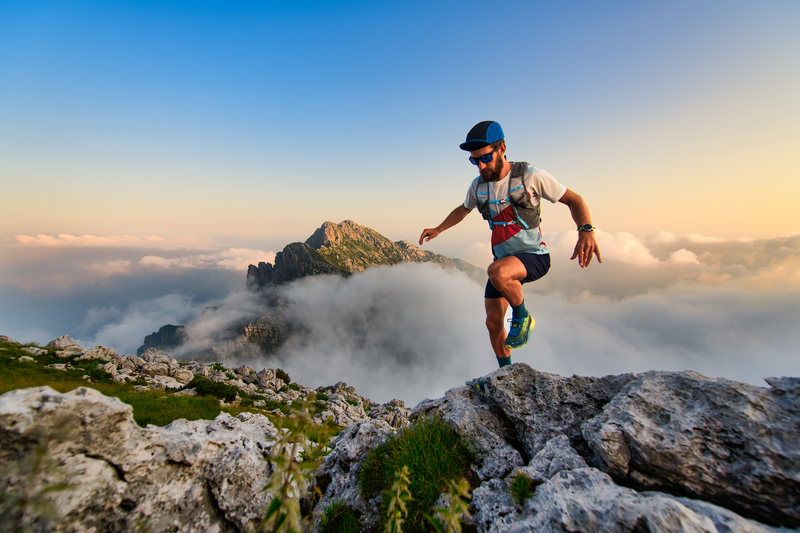
94% of researchers rate our articles as excellent or good
Learn more about the work of our research integrity team to safeguard the quality of each article we publish.
Find out more
ORIGINAL RESEARCH article
Front. Pharmacol. , 02 July 2024
Sec. Experimental Pharmacology and Drug Discovery
Volume 15 - 2024 | https://doi.org/10.3389/fphar.2024.1360226
Introduction: Multiple sclerosis (MS) is an autoimmune and inflammatory disease that destroys the protective coating of central nervous system (CNS) nerve fibers and affects over 2.8 million people worldwide. Despite several studies on new therapeutic targets and lead compounds, MS disease has limited treatment options. This condition may be caused by a complicated interaction of environmental and genetic variables. Studies showed that MS-associated microglial cells’ increased MAPKERK activity may cause CNS inflammation and oligodendrocyte damage. Thus, screening for lead compounds that inhibit MAPKERK may protect brain cells and slow disease progression.
Methods: The study aims to discover compounds that may inhibit MAPKERK as a novel approach for protecting the nervous system in managing MS. The study includes in silico methods, such as virtual screening, molecular docking, Density-functional theory (DFT) investigations (using the B3LYP/6–31++G(d,p) basis set in a gas phase environment), drug likeness scores, and molecular dynamic (MD) simulations.
Results and Discussion:During the docking process with the MAPKERK protein, it was shown that the ligand L12 receptor had the best binding affinity, with a docking score of -6.18 kcal/mol. To investigate the stability of the binding, a 100 ns MD simulation was performed on the complex formed by the MAPKERK protein and L12. The receptor-ligand combination exhibited significant stability throughout the duration of the MD simulation. Additionally, the pharmacokinetic and drug-likeness properties of these ligands suggest that they have the potential to be considered viable candidates for future development in MS management.
MS is an autoimmune condition that causes inflammatory demyelinating lesions in the CNS, affecting both white and gray matter (Kolahdouzan et al., 2019) and leading to progressive disability. The increasing frequency of MS is expected to rise, perhaps reaching a worldwide total of 2.8 million people (Soiza et al., 2018). The cause of MS is not completely understood; however, it is believed to be the consequence of a complicated interaction of environmental and genetic variables. Environmental variables include geographical location during pre-adult years, smoking behaviors, and the age of exposure to the Epstein-Barr virus, whereas genetic susceptibility entails the existence of 52 known alleles linked to the development of MS (Alrouji et al., 2023). An empirical finding indicates that increased MAPKERK activity in microglial cells linked to MS might be a factor in the development of localized inflammation in the CNS, in addition to regional oligodendrocyte dysfunction. Moreover, a clear causal connection has been established between the pathological characteristics of MS, such as inflammation, neurodegeneration, and demyelination, and the clinical manifestations of the disease. The excessive activity of MAPKERK in microglial cells, which can be observed in MS, indicates that these dysregulated microglia may have a harmful effect on oligodendrocytes, particularly in terms of myelination. The connection between microglia and oligodendrocytes highlights the importance of their interaction in the pathogenesis of MS (Peferoen et al., 2014). Prior studies have shown that impaired microglial activity negatively affects nearby oligodendrocytes (Pang et al., 2012). Oligodendrocytes are susceptible to substances generated by microglia due to MAPKERK activation (Li et al., 2008). Microglial MAPKERK activation leads to the induction of cytokines such as IL-1β and TNF-α (Kaur et al., 2013), which are associated with causing damage to adjacent oligodendrocytes and resulting in hypomyelination. Because oligodendrocytes play a vital role in supporting axons, any harm to oligodendrocytes is expected to affect axonal function (Fünfschilling et al., 2012). The negative impact of microglia on oligodendrocytes may explain the occurrence of local demyelination when microglia with an overexpression of MAPKERK are present in certain areas. Moreover, the activation of MAPKERK in microglia has been linked to the production of several pro-inflammatory mediators (Benveniste, 1997), which worsens sclerosis in the afflicted areas of the central nervous system. Although there have been many studies on prospective therapeutic targets and lead compounds for MS, the therapy choices for MS patients are still restricted. Hence, identifying lead molecules that may target the MAPKERK signaling pathway is crucial for therapeutic development. Pharmacological inhibitors that target this system have shown strong anti-inflammatory effects and provide potential for safeguarding neuronal cells from injury, which might help slow down the course of the illness. This study seeks to discover possible inhibitors of MAPKERK as a new neuroprotective approach for managing MS. As shown in Figure 1, trametinib (Pratt et al., 2020), selumetinib (Ciombor and Bekaii-Saab, 2015), cobimetinib (Han et al., 2016), binimetinib (Woodfield et al., 2016), PD0325901 (Suo et al., 2019), amantadine (Generali and Cada, 2014), and memantine (Turalde et al., 2021) have been identified as inhibitors of MAPKERK.
The present study aimed to investigate the binding of these compounds to the MAPKERK receptor via molecular docking analysis, elucidating the interaction mechanism, and studying binding stability through MD simulation analysis. Additionally, ADMET characteristics were evaluated to identify pharmacological candidates with minimal toxicity.
A subset of 137,972 natural products obtained from the ZINC12 database (Sterling and Irwin, 2012) underwent preparation procedures at a pH of 7.4, mirroring the physiological pH found in the human body. This procedure included the generation of every possible tautomer and ionized state (Release Schrödinger Suite, 2012). Following this, a meticulous screening protocol was implemented to assess each compound based on predefined criteria associated with drug-likeness properties, such as molecular weight (MW) not surpassing 500 Da, a LogP value of 5 or lower, a maximum of 10 hydrogen bond acceptors (HBA), no more than 5 hydrogen bond donors (HBD), a limitation of 10 rotatable bonds (RB), and a total polar surface area (TPSA) constrained to 140 Å2 or less. Following this screening, 15,000 compounds that met these criteria were screened.
The ligands used as input for the docking investigation were obtained from the virtual screening hits of the natural databases. Subsequently, ligands were integrated into the workstation, and energy was minimized by utilizing the OPLS3e force field in the LigPrep module of the program. This minimization aids in assigning bond order, adding hydrogens to ligands, and converting 2D structures to 3D structures for performing docking studies. The generated output file, including the best conformations of the ligands, was then used for docking studies (Schrödinger Release, 2017).
The MAPKERK protein’s crystal structure (PDB ID: 4qte) underwent refinement via Schrödinger’s protein preparation wizard (Protein Preparation Wizard, 2017). This meticulous procedure ensured an elevated level of confidence in the accuracy of the protein structure. By converting it from its raw state to a refined form, the protein was ensured for subsequent molecular docking and dynamic studies, enhancing its suitability and reliability for comprehensive studies. The protein preparation process consisted of correcting bond orders, removing water molecules and other non-specific components from the crystal structure, and adding hydrogen atoms to the protein structure to modify the tautomeric and ionization states of amino acid residues. After adding the missing hydrogen, the system underwent restricted energy reduction using the OPLS 2005 force field to ensure high accuracy.
The MAPKERK protein and its prepared ligand underwent docking using Schrödinger’s GLIDE module (Glide, 2017). This process aimed to improve binding affinity by aiding in the identification of specific structural motifs. The docking results were analyzed using XP descriptors, which provided useful details on a variety of intermolecular interactions. The Glide XP descriptor data facilitated the determination of energy associated with each pose, aiding in the optimization of the ligand conformation within the ligand-receptor complex. Before initiating the docking protocol, Schrödinger’s Glide Grid generation was employed to create a specific Glide Grid surrounding the co-crystallized ligand. Subsequently, this grid served as the framework for the docking procedure, facilitating the precise positioning and interaction analysis between the prepared ligand and the protein structures within the designated spatial coordinates.
The physico-chemical parameters were utilizing the Swiss ADME service (Daina et al., 2017) and ADMET lab 2.0 (ADMETlab 2.0, 2021). The anticipated parameters encompass several key molecular attributes: MW, HBD, HBA, the octanol/water partition coefficient (log P), aqueous solubility (Log S), predicted apparent Caco-2 cell permeability, human intestinal absorption propensity (HIA), plasma protein binding (PPB), and the tally of RB. These parameters collectively serve as crucial indicators for assessing the physicochemical properties, drug-likeness, and potential bioavailability of the compounds under investigation.
Validation of the docking study is necessary to ensure precise molecule docking. The precision of the molecular docking process is assessed using the Root Mean Square Deviation (RMSD). In order to calculate the RMSD, the co-crystallized ligand of 4qte was removed from it and then subjected to XP glide docking using the matching receptor grid. The obtained RMSD value, which was less than 2.0 Å, indicates the reliability of the docking study (Gheidari et al., 2023).
MD simulation was conducted utilizing Desmond through the Schrödinger-Maestro interface (Desmond, 2017). This enables the precise identification and prediction of ligand-receptor interactions and the validation of molecular docking results. MD simulations were performed by solving a substance in an explicit orthorhombic water box using the SPC water model. Sufficient Cl ions were added to the system in order to counterbalance the overall charge of the complex. The duration of the simulation was 100 ns. The NPT ensemble was utilized, maintaining a constant number of atoms, a pressure of 1.01325 bar, and a temperature of 300 K. The default thermostat was the 1.0-ps interval Nose-Hoover chain method, and the default barostat was the 2.0-ps interval Martyna-Tobias-Klein. The maestro simulation interaction diagram was used to assess the MD simulation.
The DFT is used to ascertain the electron’s density and energy properties. The Gaussian 09W program performs calculations that illustrate the estimated structure of atoms, molecules, crystals, and surfaces, as well as their interactions (Frisch et al., 2009). The wavenumbers of the vibrations were determined by the use of the B3LYP method and a 6-31++G (d,p) basis set. The B3LYP functional is a valuable approach for accurately characterizing harmonic vibrational frequencies in molecules of small to medium size. The output verification files were analyzed using GuassView 6.0. Molecular orbital (MO) analysis is essential in quantum chemistry and has been used to thoroughly characterize chemical behavior. The highest occupied molecular orbital (HOMO) and lowest unoccupied molecular (LUMO) of a molecule are employed to characterize chemical properties, encompassing reactivity, stability, kinetics, hardness, softness, and electronegativity.
The optimization of ligands (L1-L18) was initially conducted using the B3LYP/6-31++G (d,p) basis set in the gas phase, and the results obtained from this optimization are presented in Table 1.
The geometries of the selected ligands underwent optimization until reaching the lowest energy gradient, confirming their status as true local minima, as no imaginary frequencies were detected. Figure 2 illustrates the optimized structures of the selected ligands.
The study of MO is very important in quantum chemistry, significantly enhancing our understanding and knowledge of chemical behavior. The HOMO and LUMO that are the chief molecular orbitals in a ligand are shown in Figure 3. The red and green color distributions represent the positive and negative phases, respectively, in the MO wave function.
The parameter hardness (h) quantifies the degree of hardness or softness exhibited by a molecule. Greater molecular softness correlates with enhanced reactivity. The atom’s electronegativity (X) indicates its ability to attract electron pairs towards its nucleus. The ligand L4, exhibiting the smallest HOMO–LUMO energy gap value of 2.4884 eV, suggests a heightened potential for chemical reactivity. Also, L4 demonstrates a low hardness value of 1.2442, positioning it as the softest ligand among all ligands. The greater electronegativity value of ligand L4 indicates that L4 has a strong ability to attract electrons and operates as a superior electrophile compared to other ligands. Ligands L3 and L10 exhibited favorable reactivity after L4, with energy gap values of 3.7621 eV and 4.7434 eV, respectively. Similarly, after L3, the ligands L11, L4, and L5 exhibit significant polarizability, with corresponding values of 236.316, 239.453, and 240.025. The energetic parameters of ligands (L1-L18) are shown in Table 2.
Schrödinger’s Ligand docking module utilizes grid-based ligand docking with energetics (GLIDE) (Glide, 2017) in three specific docking modes: high-throughput virtual screening (HTVS), standard precision (SP), and extra precision (XP) for docking and scoring. 15,000 compounds underwent screening by HTVS docking and scoring. A total of 50 ligands that had the highest G-Score with HTVS were further evaluated using SP docking and scoring. This was done to ensure that the docking postures were reliable and proper. To decrease false-positive findings, 18 ligands that were completely thriving were screened using XP docking and scoring. An analysis was conducted on the interactions of these protein-ligand complexes, and the outcomes are shown in Table 3. MAPKERK’s active site’s amino acid residues, both bonding and non-bonding interactions with ligand L1–L18, included Asn154, Ser153, Asp111, Tyr113, Gly32, Glu33, Gly34, Asp167, Leu156, Ile31, Cys166, Val39, Lys54, Tyr30, Lys114, Gln105, Ile84, Lys151, Asp111, Asp149, Ala35, Tyr36, Glu71, Ala52, and Lys117.
L15 ranked second out of the three top-scoring ligands due to its strong binding interactions with the active site of the MAPKERK protein. Ligand L15 has six carbon-hydrogen bonds, corresponding to the amino acid residues Ser153 and Glu33, with bond lengths of 2.42 and 2.74, respectively. Additionally, it interacts with Asp167 at a bond length of 3.02 and with Asn154 at bond lengths of 2.18 and 2.23. Furthermore, five hydrophobic interactions have been observed between the ligand L15 and the MAPKERK protein. These include bond lengths of 4.88 Å for Lys54, 3.82 Å for Cys166, 4.56 Å for Leu156, and two band lengths of 4.60 and 4.85 Å for Val39. Also, ligand L15 interacts with Gly134, Lys151, Gln105, Ile84, Ala52, Ile31, Gly32, and Asp111 in five van der Waals interactions. Figure 5 displays the 2D and 3D binding interactions of L15 within MAPKERK’s active pocket.
Ligand L17 has five carbon-hydrogen bonds, two of which are associated with amino acid Ser153 and have bond lengths of 2.21 and 2.79, respectively; the other two are related to amino acid Asn154 and have bond lengths of 2.31 and 2.72, respectively; and another is related to Glu33 and has a bond length of 2.48. In addition, it has four alkyl interactions with the residues Leu156, Cys166, and Val39, and nine van der Waals interactions with Gly34, Asp167, Gln105, Asp111, Lys54, Gly32, Lys151, and Ile31. Figure 6 displays the 2D and 3D binding interactions of L17 within MAPKERK’s active pocket. In summary, Table 3 illustrates that the two main interactions in all complexes are hydrophobic intermolecular interactions and carbon-hydrogen bonds.
In order to enhance the reliability of the obtained results and confirm the stability of the system, we combined the docking methods with MD simulation. This allowed us to investigate the changes in the ligand-receptor complex’s conformation during the simulation time (Caporuscio et al., 2011). Simulations offer a detailed investigation of the exact movement of each atom over time, allowing us to investigate changes and fluctuations in protein patterns. MD simulation was conducted on the best-scoring L12 complex for 100 ns. The dynamic stability of the complexes was assessed by measuring the RMSD of the complex backbone atoms during the whole trajectory to confirm their stability (Figure 7). The RMSD curves of the ligand (Lig fit Prot) and the protein backbone (Cα) showed that our simulation had converged and equilibrated after an initial period of disturbance. Throughout the 100 ns simulation, variations in the RMSD of L12 in the binding pocket ranged from 1.70 to 4.20 Å. The MAPKERK altered its maximum RMSD from 0.85 Å in the starting frame to 3.20 Å. Higher fluctuations were observed between 22 and 30 ns, after which the RMSD remained constant until the last 100 ns. The convergence of RMSD values indicated that L15 and MAPKERK maintained their interaction through the simulation.
Root Mean Square Fluctuation (RMSF) serves as a metric quantifying the average deviation of each atom’s position from its mean position within a specified simulation or ensemble of structures. The RMSF values for the catalytic region of the MAPKERK of all the complexes exhibited stability. There were no considerable fluctuations observed where the ligand binds to the protein.The comprehensive analysis of the interaction between L12 and the binding site residues of MAPKERK is illustrated in Figure 8. The residues that interact with L12 are as follows: Ile31,Glu33,Ala35,Val39,Ala52,Lys54,Ile84,Gln105,Leu107,Leu112,Lys114,Lys151,Ser153,Asn154,Leu156,Cys166,Asp167. The residue interacting with L15 is shown in green, while the protein’s secondary structures, helices and β-strands, are represented by orange and blue bands, respectively. The RMSF values for the residues in the binding site were found to be less than 2 Å.
Ligand-protein interactions can be monitored throughout the simulation. These interactions can be categorized as hydrogen bonds, hydrophobic, ionic, and water bridges, and summarized. As depicted in Figure 9, Ala52, Ile84, and Leu156 engaged in hydrophobic interactions with the ligand for approximately 24%, 4%, and 7% of the simulation duration. Moreover, over at least 3% of the simulation period, Lys54, Gln105, and Asp111, Lys151, Asn154, and Asp167 formed water bridge interactions with the ligand. Significantly, Ser153 demonstrated a variety of interactions, encompassing water-bridged and hydrogen-bond interactions with the ligand. Consequently, this residue experienced numerous interactions throughout the simulation.
Throughout the 100-ns MD simulation, ligand properties were examined. The RMSD of a ligand with respect to its reference conformation (time t = 0) was calculated to be between 0.56 and 0.85 Å. The radius of gyration (Rg) provided a means to evaluate the variation in compactness of the ligand-protein complex within the range of 2.97–3.14 Å. The molecular surface area (MolSA) was computed using the van der Waals surface area between 250 and 260 Å. No intramolecular hydrogen bond (intra-HB) was detected. The solvent accessible surface area (SASA) analysis examines the interaction between a ligand and solvents during a 100 ns MD simulation, yielding values ranging from 40 to 158 Å. The analysis also included the examination of the polar surface area (PSA), which provides information about the solvent’s ability to access the surface area given by oxygen and nitrogen atoms. The PSA value ranged from 29 to 40 Å (Figure 10).
The physicochemical, pharmacokinetic, and medicinal chemistry features mentioned for our top 18 candidates are shown in Table 4. The above 18 lead ligands were found to have desirable drug-like properties based on Lipinski’s rule of five. The pharmacokinetic parameters revealed that all compounds are highly absorbed after oral administration through the gastrointestinal tract (GI). Structural alarms and pan-assay interference (PAINS) have been utilized in medicinal chemistry to forecast the presence of unstable, reactive, and toxic fragments in a compound’s structure (Baell and Holloway, 2010). All ligands in PAINS descriptors have zero alarms. The synthetic accessibility score (SA score) is a metric used to evaluate the ease of synthesizing drug-like molecules. It was observed that all the compounds possess a favorable SA score, indicating that they can be readily synthesized. The use of CaCo-2 cells, which are generated from human colon epithelial cells, is a widely accepted approach for studying the intestinal absorption of medicines in people. The CaCo-2 cell permeability findings showed that all ligands except L2, L9, L10, L11, L12, L13, L14, L15, L17, and L18 were in the satisfactory range, indicating that these ligands have favorable membrane permeability. In terms of plasma glycoprotein (PGP) substrate, it was observed that, except for ligands L3, L4, L5, and L11, other ligands have inhibitory effects on PGP. As well, all ligands exhibit PGP substrate activity. The computed values for HIA indicate that all ligands except L12, L14, and L18 possess a high likelihood of being effectively absorbed through the intestinal membrane. The assessment of PPB has significance in the evaluation of drug safety. Drugs exhibiting a high PPB value (>90%) are associated with a narrow therapeutic index, whereas those with a low PPB value are considered to be comparatively safer. In the current investigation, it was shown that all ligands exhibited low PPB values. This finding suggests that these particular compounds have a wide therapeutic index, indicating a favorable safety profile. A substance with a positive blood-brain barrier value has a higher lipophilicity profile and may be easily absorbed from plasma membranes. It was noticed that ligand L1 had a higher lipophilicity profile, with values as high as BBB+++. Ligands L11, L12, L13, L14, and L16 are shown to be carcinogenic, while the other ligands are non-carcinogenic. The AMES toxicity profile showed that all ligands have the potential to be toxic. Overall, all ligands demonstrated better ADMET profiles; all values are shown in Table 5.
Table 4. Physicochemical, pharmacokinetics, and medicinal chemistry properties of the compounds (L1-L18).
The study aims to find prospective compounds for MS by targeting the MAPKERK protein using a computational method to expedite the drug development process and reduce costs. Studies show that heightened MAPKERK activity in microglial cells associated with MS can lead to inflammation in the CNS and harm oligodendrocytes. In the current study, the findings of molecular docking, ADMET, MD simulation, and DFT calculations suggested that diazaadamantan derivatives can be a suitable scaffold for developing efficient leads capable of inhibiting MAPKERK and aiding in the battle against MS. During docking with MAPKERK, the L12 receptor exhibited the best binding affinity. A 100 ns MD simulation of the MAPKERK protein and L12 complex assessed binding stability. The MD simulation showed potent receptor-ligand stability. These ligands’ pharmacokinetic and drug-like properties suggest they can be potential MS therapy options. However, to confirm the biological activity of the selected ligands and assess the value of pharmacological inhibition of MAPKERK, more in vitro and in vivo research is necessary.
The original contributions presented in the study are included in the article/Supplementary material, further inquiries can be directed to the corresponding authors.
DG: Conceptualization, Data curation, Investigation, Methodology, Software, Supervision, Writing–original draft. MM: Conceptualization, Data curation, Investigation, Methodology, Software, Supervision, Writing–original draft. FH: Data curation, Methodology, Writing–review and editing, Data curation, Methodology, Writing–review and editing.
The author(s) declare that no financial support was received for the research, authorship, and/or publication of this article.
The authors declare that the research was conducted in the absence of any commercial or financial relationships that could be construed as a potential conflict of interest.
All claims expressed in this article are solely those of the authors and do not necessarily represent those of their affiliated organizations, or those of the publisher, the editors and the reviewers. Any product that may be evaluated in this article, or claim that may be made by its manufacturer, is not guaranteed or endorsed by the publisher.
ADMETlab 2.0 (2021). ADMETlab 2.0. https://admetmesh.scbdd.com/(Accessed September 29, 2021).
Alrouji, M., Manouchehrinia, A., Aram, J., Alotaibi, A., Alhajlah, S., Almuhanna, Y., et al. (2023). Investigating the effect of cigarette smoking on serum uric acid levels in multiple sclerosis patients: a cross sectional study. Brain. Scien 13, 800. doi:10.3390/brainsci13050800
Baell, J. B., and Holloway, G. A. (2010). New substructure filters for removal of Pan assay interference compounds (PAINS) from screening libraries and for their exclusion in bioassays. J. Med. Chem. 53, 2719–2740. doi:10.1021/jm901137j
Benveniste, E. N. (1997). Role of macrophages/microglia in multiple sclerosis and experimental allergic encephalomyelitis. J. Mol. Med. 75, 165–173. doi:10.1007/s001090050101
Caporuscio, F., Rastelli, G., Imbriano, C., and Del Rio, A. (2011). Structure-based design of potent aromatase inhibitors by high-throughput docking. J. Med. Chem. 54, 4006–4017. doi:10.1021/jm2000689
Ciombor, K. K., and Bekaii-Saab, T. (2015). Selumetinib for the treatment of cancer. Expert. Opin. Invest. Drugs. 24, 111–123. doi:10.1517/13543784.2015.982275
Daina, A., Michielin, O., and Zoete, V. (2017). SwissADME: a free web tool to evaluate pharmacokinetics, drug-likeness and medicinal chemistry friendliness of small molecules. Sci. Rep. 7, 42717. doi:10.1038/srep42717
Frisch, M. J., Trucks, G. W., Schlegel, H. B., Scuseria, G. E., Robb, M. A., Cheeseman, J. R., et al. (2009). Gaussian 09, revision A.02. Wallingford, CT, USA: Gaussian, Inc.
Fünfschilling, U., Supplie, L. M., Mahad, D., Boretius, S., Saab, A. S., Edgar, J., et al. (2012). Glycolytic oligodendrocytes maintain myelin and long-term axonal integrity. Nature 485, 517–521. doi:10.1038/nature11007
Generali, J. A., and Cada, D. J. (2014). Amantadine: multiple sclerosis–related fatigue. Hosp. Pharm. 49, 710–712. doi:10.1310/hpj4908-710
Gheidari, D., Mehrdad, M., and Bayat, M. (2023). Synthesis, docking, MD simulation, ADMET, drug likeness, and DFT studies of novel furo[2,3-b]indol-3a-ol as promising Cyclin-dependent kinase 2 inhibitors. Sci. Rep. 14, 3084. doi:10.1038/s41598-024-53514-1
Han, L., Zhang, Q., Shi, C., Cavazos, A., Ruvolo, V., Leverson, J., et al. (2016). Targeting MAPK signaling pathway with cobimetinib (GDC-0973) enhances anti-leukemia efficacy of venetoclax (ABT-199/GDC-0199) in acute myeloid leukemia models. Blood 128, 97. doi:10.1182/blood.V128.22.97.97
Kaur, C., Rathnasamy, G., and Ling, E. A. (2013). Roles of activated microglia in hypoxia induced neuroinflammation in the developing brain and the retina. J. Neuroi. Pharm. 8, 66–78. doi:10.1007/s11481-012-9347-2
Kolahdouzan, M., Futhey, N. C., Kieran, N. W., and Healy, L. M. (2019). Novel molecular leads for the prevention of damage and the promotion of repair in neuroimmunological disease. Front. Immunol. 10, 1657. doi:10.3389/fimmu.2019.01657
Li, J., Ramenaden, E. R., Peng, J., Koito, H., Volpe, J. J., and Rosenberg, P. A. (2008). Tumor necrosis factor alpha mediates lipopolysaccharide-induced microglial toxicity to developing oligodendrocytes when astrocytes are present. J. Neuros 28, 5321–5330. doi:10.1523/JNEUROSCI.3995-07.2008
Pang, Y., Zheng, B., Kimberly, S. L., Cai, Z., Rhodes, P. G., and Lin, R. C. (2012). Neuron-oligodendrocyte myelination Co-culture derived from embryonic rat spinal cord and cerebral cortex. Brain. Behav. 2, 53–67. doi:10.1002/brb3.33
Peferoen, L., Kipp, M., van der Valk, P., van Noort, J. M., and Amor, S. (2014). Oligodendrocyte-microglia cross-talk in the central nervous system. Immun 141, 302–313. doi:10.1111/imm.12163
Pratt, E. C., Isaac, E., Stater, E. P., Yang, G., Ouerfelli, O., Pillarsetty, N., et al. (2020). Synthesis of the PET tracer 124I-trametinib for MAPK/ERK kinase distribution and resistance monitoring. J. Nucl. Med. 61, 1845–1850. doi:10.2967/jnumed.120.241901
Protein Preparation Wizard (2017). Schrödinger suite 2017-1: protein preparation wizard. New York: Schrödinger, LLC.
Soiza, R. L., Donaldson, A. I. C., and Myint, P. K. (2018). Rising prevalence of multiple sclerosis worldwide: insights from the Atlas of MS, third edition. Ther. Adv. Vaccines 26 (14), 1816–1821. doi:10.1177/1352458520970841
Sterling, , and Irwin, J. (2012). ZINC 15 – Ligand Discovery for Everyone. Chem. Inf. Model. 55 (11), 2324–2337. doi:10.1021/acs.jcim.5b00559 (Accessed March 26, 2024).
Suo, N., Guo, Y. E., He, B., Gu, H., and Xie, X. (2019). Inhibition of MAPK/ERK pathway promotes oligodendrocytes generation and recovery of demyelinating diseases. Glia 67, 1320–1332. doi:10.1002/glia.23606
Turalde, C. W. R., Espiritu, A. I., and Anlacan, V. M. M. (2021). Memantine for multiple sclerosis: a systematic review and meta-analysis of randomized trials. Front. Neurol. 11, 574748. doi:10.3389/fneur.2020.574748
Keywords: virtual screening, molecular docking, MAPKERK, Diaza-adamantane, MD simulation
Citation: Gheidari D, Mehrdad M and Hoseini F (2024) Virtual screening, molecular docking, MD simulation studies, DFT calculations, ADMET, and drug likeness of Diaza-adamantane as potential MAPKERK inhibitors. Front. Pharmacol. 15:1360226. doi: 10.3389/fphar.2024.1360226
Received: 12 January 2024; Accepted: 07 March 2024;
Published: 02 July 2024.
Edited by:
Mithun Rudrapal, Vignan’s Foundation for Science, Technology and Research, IndiaReviewed by:
Anas Shamsi, Ajman University, United Arab EmiratesCopyright © 2024 Gheidari, Mehrdad and Hoseini. This is an open-access article distributed under the terms of the Creative Commons Attribution License (CC BY). The use, distribution or reproduction in other forums is permitted, provided the original author(s) and the copyright owner(s) are credited and that the original publication in this journal is cited, in accordance with accepted academic practice. No use, distribution or reproduction is permitted which does not comply with these terms.
*Correspondence: Davood Gheidari, ZGF2b29kZ2hlaWRhcmlAZ21haWwuY29t; Morteza Mehrdad, bV9tZWhyZGFkNEB5YWhvby5jb20=
Disclaimer: All claims expressed in this article are solely those of the authors and do not necessarily represent those of their affiliated organizations, or those of the publisher, the editors and the reviewers. Any product that may be evaluated in this article or claim that may be made by its manufacturer is not guaranteed or endorsed by the publisher.
Research integrity at Frontiers
Learn more about the work of our research integrity team to safeguard the quality of each article we publish.