- 1Center for Research in Medical Pharmacology, Varese, Italy
- 2PhD Program in Clinical and Experimental Medicine and Medical Humanities, University of Insubria, Varese, Italy
- 3General Psychiatry Service, Hopitaux Universitaires de Genève, Geneva, Switzerland
- 4Unit of Pharmacogenetics and Clinical Psychopharmacology, Centre for Psychiatric Neuroscience, Department of Psychiatry, Lausanne University Hospital, University of Lausanne, Prilly, Switzerland
- 5Service of General Psychiatry, Department of Psychiatry, Lausanne University Hospital, University of Lausanne, Prilly, Switzerland
- 6Les Toises Psychiatry and Psychotherapy Center, Lausanne, Switzerland
- 7Service of Old Age Psychiatry, Department of Psychiatry, Lausanne University Hospital, University of Lausanne, Prilly, Switzerland
- 8School of Pharmaceutical Sciences, University of Geneva, University of Lausanne, Geneva, Switzerland
- 9Center for Research and Innovation in Clinical Pharmaceutical Sciences, University of Lausanne, Lausanne, Switzerland
- 10Institute of Pharmaceutical Sciences of Western Switzerland, University of Geneva, University of Lausanne, Lausanne, Switzerland
Background: Response to antipsychotics is subject to a wide interindividual variability, due to genetic and non-genetic factors. Several single nucleotide polymorphisms (SNPs) have been associated with response to antipsychotics in genome-wide association studies (GWAS). Polygenic risk scores (PRS) are a powerful tool to aggregate into a single measure the small effects of multiple risk alleles.
Materials and methods: We studied the association between a PRS composed of SNPs associated with response to antipsychotics in GWAS studies (PRSresponse) in a real-world sample of patients (N = 460) with different diagnoses (schizophrenia spectrum, bipolar, depressive, neurocognitive, substance use disorders and miscellaneous). Two other PRSs composed of SNPs previously associated with risk of schizophrenia (PRSschizophrenia1 and PRSschizophrenia2) were also tested for their association with response to treatment.
Results: PRSresponse was significantly associated with response to antipsychotics considering the whole cohort (OR = 1.14, CI = 1.03–1.26, p = 0.010), the subgroup of patients with schizophrenia, schizoaffective disorder or bipolar disorder (OR = 1.18, CI = 1.02–1.37, p = 0.022, N = 235), with schizophrenia or schizoaffective disorder (OR = 1.24, CI = 1.04–1.47, p = 0.01, N = 176) and with schizophrenia (OR = 1.27, CI = 1.04–1.55, p = 0.01, N = 149). Sensitivity and specificity were sub-optimal (schizophrenia 62%, 61%; schizophrenia spectrum 56%, 55%; schizophrenia spectrum plus bipolar disorder 60%, 56%; all patients 63%, 58%, respectively). PRSschizophrenia1 and PRSschizophrenia2 were not significantly associated with response to treatment.
Conclusion: PRSresponse defined from GWAS studies is significantly associated with response to antipsychotics in a real-world cohort; however, the results of the sensitivity-specificity analysis preclude its use as a predictive tool in clinical practice.
Introduction
Antipsychotics represent the mainstay in the treatment of schizophrenia and schizophrenia spectrum disorders and are among the principal therapeutic options for bipolar disorder (National institute for Heath and Care excellence NICE, 2014; Taylor et al., 2018; Yatham et al., 2018). Some antipsychotics can also be used as add-ons for treatment resistant unipolar major depression (Cantù et al., 2021) and many are extensively prescribed off-label for anxiety disorders (Pies, 2009; Hershenberg et al., 2014; Pignon et al., 2017), personality disorders (Rosenbluth and Sinyor, 2012; Wasylyshen and Williams, 2016; Yadav, 2020) and organic mental disorders (Elie and Rej, 2018). In the treatment of psychosis, response rates range from 47% for individuals who have received prior treatment to 66% for antipsychotic-naive individuals (Haddad and Correll, 2018), while 1-year discontinuation rates may be as high as 74% due to poor tolerability and/or lack of efficacy (Lieberman et al., 2005). Inter-individual variability in efficacy and side effects of antipsychotics is large and unpredictable, with several contributing factors, including nongenetic (e.g., lack of compliance, variability in pharmacokinetics, drug-drug interactions, environmental factors), genetic and epigenetic factors (Mackenzie et al., 2010; Pouget et al., 2014).
Among genetic factors, single nucleotide polymorphisms (SNPs) are relevant, contributing from 25% up to 50% of inappropriate drug responses, both in terms of efficacy and side effects (Allen and Bishop, 2019; Martin et al., 2019). Individual SNPs can be used according to a candidate gene approach to estimate their influence on drug response. More interestingly, they can be used together to build a polygenic risk score (PRS), combining multiple SNPs previously found to be associated to drug response in genome-wide association studies (GWAS), integrating into a single measure the sum of genetic influences (Martin et al., 2019). Of note, many GWAS have been published, aiming at elucidating the relationship between genetics and response to antipsychotic treatment, with the most relevant being systematically reviewed recently (Allen and Bishop, 2019; Koromina et al., 2020).
Thus, two studies managed to establish a positive relationship between response to treatment and a PRS (Zhang et al., 2019; Chen et al., 2020), while another did not (Santoro et al., 2018). Other studies focused on the relationship between a PRS and treatment-resistant schizophrenia (i.e., the clinical condition defined as the persistence of symptoms despite ≥2 trials of antipsychotic medications of adequate dose and duration with documented adherence (Demjaha et al., 2017)). Results were contradictory as well, since two studies established an association (Werner et al., 2020; Pardiñas et al., 2022) but another did not (Wimberley et al., 2017).
Studies including only highly selected and controlled patients determines a lack of generalizability to real-world clinical practice; for this reason, studies with a real-world design are becoming increasingly popular (Sherman et al., 2016).
The hypothesis of the study relies on the important genetic component in schizophrenia etiology and response to treatment, and on their polygenic nature; accordingly, we expected that a polygenic risk score could account for a significant proportion of variability in the individual response to antipsychotics.
The objective of the present study was to evaluate if a PRS, composed of 11SNPs previously associated with response to antipsychotic medications in GWAS studies (Allen and Bishop, 2019; Koromina et al., 2020), is associated with response to antipsychotic treatment in a real-world cohort (Allen and Bishop, 2019; Koromina et al., 2020).
Methods
Sample description
Four-hundred-and-sixty patients of European descent treated with antipsychotic(s) in the psychiatric service of the Lausanne University hospital as outpatients, inpatients or both, from 16.12.2006 to 09.09.2020, and with extensive genetic data available were selected in the cohort study. They are part of the PsyMetab study, an ongoing longitudinal cohort of over 3,000 psychiatric patients taking psychotropic medication, focusing on risks of weight gain and metabolic problems (PsyMetab, 2021; Sjaarda et al., 2023). Selected patients for the present study were the ones treated with an antipsychotic as primary therapy (see Table 1), combined in some cases with other antipsychotic(s), mood stabilizer(s), antidepressant(s) and/or benzodiazepine(s); 71% of the patients received an antipsychotic monotherapy.
Demographic covariates (i.e., sex, age and ethnicity) as well as history of treatment (e.g., psychotropic treatment duration) were obtained from medical files. Psychiatric clinical status was evaluated through Clinical Global Impressions-Severity (CGI-S) and Clinical Global Impressions-Improvement (CGI-I) rating scales, obtained through the PsyMetab database. Response to treatment was based on CGI-I and CGI-S scores and/or on the need for dose increases, a switch to another antipsychotic or mood stabilizer, an add-on and/or discontinuation of the treatment according to criteria described in Table 2. The clinical evaluation for each participant was performed for the first year after inclusion in the PsyMetab cohort.
Psychiatric diagnoses were made according to ICD-10 classification criteria. Diagnoses were proposed by a senior psychiatrist by means of a semi-structured interview, and/or by means of a prolonged longitudinal evaluation. The main diagnostic groups were [F20.0-F24.9] & [F28-F29]: psychotic disorders; [F25.0-F25.9]: schizoaffective disorders; [F30.0-F31.9]: bipolar disorders; [F32.00-F33.9]: depression; [F03.0-F03.9]: dementia; [F10.0-F19.99]: substance use disorder; miscellaneous. The PsyMetab study was approved by the Ethics Committee of the Canton of Vaud (CER-VD) Lausanne University Hospital (approval number 2017–01301); written informed consents, also for genetic analysis, were obtained from all the participants.
SNP selection, genotyping and construction of PRSs
Genetic variants were determined by standard genotyping or imputation methods. DNA samples from all patients were genotyped using the Global Screening Array (GSA) v2 with multiple disease option processed on an iScan equipped platform (Illumina, San Diego, CA) at the iGE3 genomics platform of the University of Geneva (https://www.ige3.unige.ch/). Quality control methods were applied as follows: SNPs were excluded on the basis of low call rate (<99%), deviation from HW-equilibrium (p < 1 × 10−6), or low minor allele frequency (MAF<0.05). Sex mismatches or cryptic relatedness were also removed. All quality control steps were performed using PLINK (https://www.cog-genomics.org/plink/2.0/). Finally, all autosomal variants were submitted to the Michigan imputation server (https://imputationserver.sph.umich.edu/index.html#!). The server uses SHAPEIT2 (v2. r790) to phase data and imputation to the reference panel (1000G phase 1 version 3) was performed with Minimac 3.7.8.
Polygenic risk scores were built with the “standard weighted allele” method implemented in PRSice-2 (Chen et al., 2018). Linkage disequilibrium (LD) clumping was performed to retain only data for independent SNPs (r2 < 0.1, clump-p = 1, 250 kb windows).
The main PRS (PRSresponse) was built using 11 SNPs (see Table 3). The rationale was to combine SNPs previously associated with response to antipsychotics in GWAS studies, as from two recent reviews (Allen and Bishop, 2019; Koromina et al., 2020), and having a minor allele frequency in the European descent population >5%. We aimed to replicate findings from the source studies, testing each individual SNP for association with response, and to determine if the combination of SNPs in a PRS could improve the prediction of response to antipsychotics.
Four other SNPs obtained from these studies were ruled out for the following reasons: rs17390445 was not used because the risk allele for response to treatment was not available from the original author; rs11725502 and rs10023464 were in total linkage disequilibrium, as well as rs17742120, rs2164660 and rs17382202: rs11725502, rs17742120 and rs2164660 were therefore not included in the PRS.
In addition, 2 PRSs composed on SNPs related to the risk of schizophrenia were built. PRSschizophrenia1 used 94 SNPs from a 2014 study realized by the Psychiatric Genomic consortium (Schizophrenia Working Group of the Psychiatric Genomics Consortium, 2014); PRSschizophrenia2 used 313 SNPs from a novel study performed by the same group in 2022 (Trubetskoy et al., 2022).
The aim of using these two PRSs was to have a control to confirm the non-random nature of the results eventually obtained with PRSresponse, and to explore the hypothesis that SNPs conferring a risk of schizophrenia could also be linked to response to antipsychotic treatment. PRSresponse had no SNP in common with PRSschizophrenia1 and PRSschizophrenia2.
Building PRSresponse, the effect of each SNP could not be weighted, since beta-coefficients for response to treatment were unknown; a dummy beta-value of 1 was used, and genotypes from each SNP were coded as 0, 1 or 2 according to the number of risk alleles for non-response to antipsychotic treatment (PRSresponse). For PRSschizophrenia1 and PRSschizophrenia2 the influence of each SNPs was weighted based on odds-ratios.
Statistics
Ethnicity was assessed by the patient’s reported ethnicity and confirmed by genotyping using a principal component analysis with the EIGENSTRAT algorithm implemented in GCTA software. The majority of the variance was explained by the first two vectors and the European descent was arbitrarily selected when pca1<0.0025 and pca2>−0.0125, values which gave the highest concordance with the patient’s reported ethnicity. For further precision, principal components PC1, PC2, PC3, PC4 and PC5 were used in the statistical model described below.
Hardy-Weinberg Equilibrium (HWE) was determined for each polymorphism by a chi-square test. Descriptive analysis of quantitative data are presented as median and range unless otherwise specified whereas qualitative data are expressed as percentages. The chi-squared test or rank sum test were used for association studies within categorical data or non-parametric continuous variables, respectively. p-values equal to or less than 0.05 were considered statistically significant.
Influence of possible confounders on the variable of interest (response to treatment) were analyzed through a univariate analysis based on a linear regression model. We derived a list of prognostic factors for response to antipsychotic treatment which includes premorbid global functioning, education, age of onset, sex, psychiatric comorbidities (especially substance abuse), duration of untreated psychosis (DUP), duration of disease, baseline severity and response to treatment in the first 2 weeks (Chandola and Jenkinson, 2000; Carbon and Correll, 2014; Bozzatello et al., 2019). According to data available in our database we studied the possible effect of age (used as a proxy for age of onset and duration of untreated psychosis), duration of disease, sex, socioeconomic status (used as a proxy for premorbid global functioning and education), and of comorbid substance abuse. Socioeconomic status was obtained with a GPS-based classification system, as previously described (Takeuchi et al., 2019; Dubath et al., 2020). Other variables of interest such as duration of untreated psychosis and treatment response in the first 2 weeks were not available in our dataset.
Moreover, relapses of psychotic episodes are known to cause resistance to antipsychotic treatment (Ruopp et al., 2008; Taipale et al., 2022). In order to account for this possible confounder we evaluated the relationship between the number of admissions to a psychiatric unit and response to treatment in the cohort.
In univariate analysis, age was significantly associated with response to treatment (p < 0.004, data not shown) in the whole cohort, a result confirmed in multivariate analysis (data not shown). Other variables listed above showed no associations with response to treatment and were therefore not included in multivariate analysis. A logistic regression model linking each PRS (PRSresponse, PRSschizophrenia1 and PRSschizophrenia2) with response to treatment was performed, including the whole group and specific diagnostic subgroups. In the logistic regression model, PRS, age and the 5 principal genetic components (PC1-PC5) were used as predictor variables, and response to antipsychotics was the outcome variable. Sensitivity, specificity, positive predictive value (PPV) and negative predictive value (NPV) were calculated for PRS, predicting response to treatment. Cut-off values for the sensitivity-specificity analysis were chosen in order to maximize the Youden index (Wray et al., 2007).
Results
Population description
A flow chart describing patient selection is shown in Figure 1.
Table 4 presents the characteristics of the included patients, consisting of 460 individuals of european descent, of which 249 were responders and 211 non-responders to antipsychotic treatment. Non-responders were significantly older than responders (48.4 years vs. 42.2 years, p < 0.002), while other factors known to predict a worse response to antipsychotics, such as male gender, low socioeconomic status, longer duration of illness, higher baseline severity of the illness and substance abuse (Chandola and Jenkinson, 2000; Bozzatello et al., 2019; Dubath et al., 2020) and the number of admissions to a psychiatry unit were similarly distributed in both groups.
Association of individual SNPs with response to AP
The relationship between response to treatment and each individual SNP composing PRSresponse was first considered. No statistically significant associations were found, both considering the whole cohort (see Table 5) and subgroups of patients (i.e., schizophrenia spectrum plus bipolar disorder, schizophrenia spectrum, schizophrenia, schizoaffective disorder, bipolar disorder, others; data not shown).
Association of PRSresponse, PRSschizophrenia1 and PRSschizophrenia2 with response to APs
PRSresponse was significantly associated with response to antipsychotic treatment considering all patients without differentiation based on diagnostic category (OR = 1.14, CI = 1.03–1.26, N = 460, see Table 6), but also in the group of patients with schizophrenia, schizoaffective disorder or bipolar disorder (OR = 1.18, CI = 1.02–1.37, N = 235) and in the group of patients with schizophrenia and schizoaffective disorder (OR = 1.27, CI = 1.04–1.47, N = 176). When considering single diagnosis, a significant association was observed for schizophrenia (OR = 1.27, CI = 1.04–1.55, N = 149) but not for schizoaffective disorder (OR = 1.78, CI = 0.91–3.52, N = 27), bipolar disorder (OR = 1.00, CI = 0.71–1.41, N = 59) or another diagnosis of minor interest for antipsychotic prescription (OR = 0.98, CI = 0.76–1.26, N = 96), each represented by few patients (depression, N = 42; neurocognitive disorders, N = 17; substance abuse disorder, N = 9; others, N = 28). The most relevant results for PRS predicting the response to treatment were as follows: for the whole cohort of patients sensitivity was 63%, specificity 58%, PPV 64%, NPV 57%; for the group of patients with schizophrenia sensitivity was 62%, specificity 61%, PPV 66%, NPV 57%.
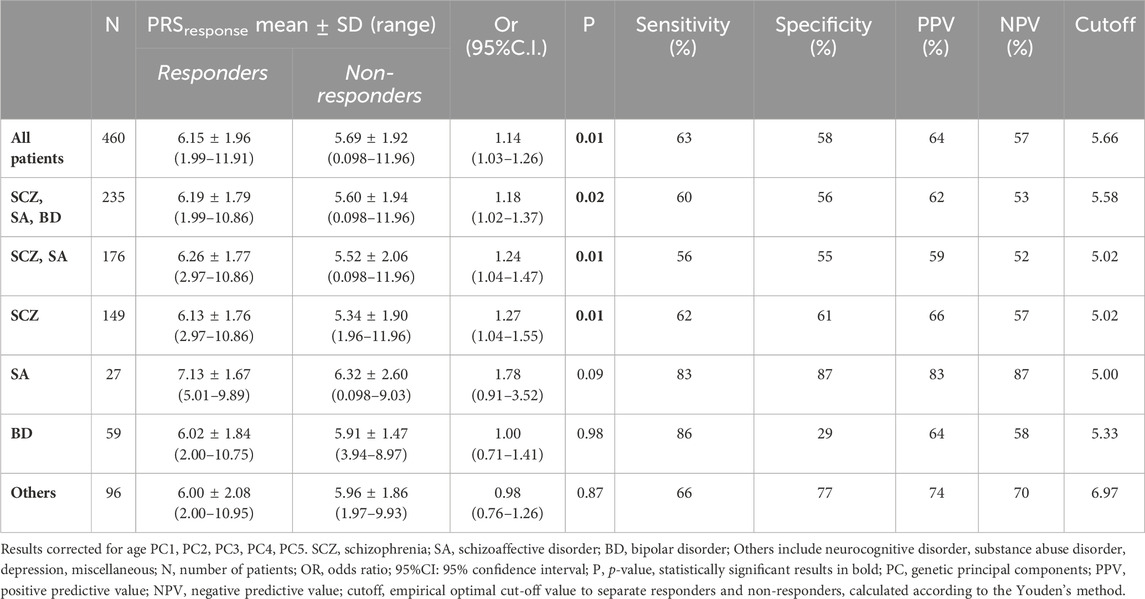
TABLE 6. PRSresponse values in responders and non-responders and logistic multivariate regression analysis between PRSresponse and response to treatment.
Figure 2 shows the distribution of PRSresponse in responders and non-responders to treatment.
Using a logistic multivariate regression controlling for age, PRSschizophrenia1 was not associated with response to treatment either in the whole group, nor in any of the abovementioned diagnoses (see Table 7).
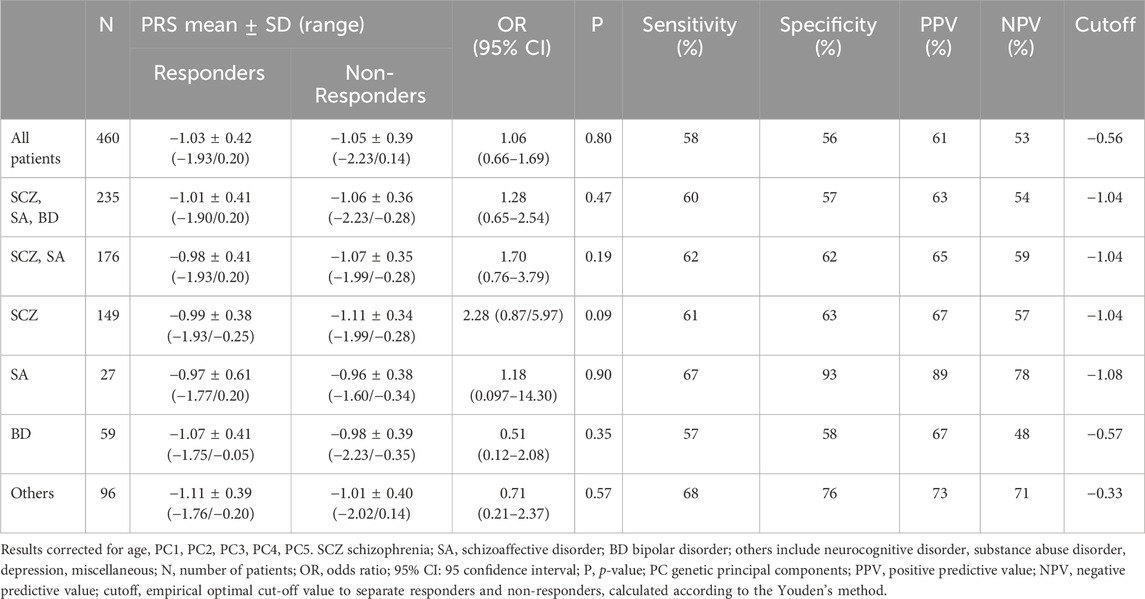
TABLE 7. PRSschizophrenia1 values in responders and non-responders and logistic multivariate regression analysis between PRSschizophrenia1 and response to treatment.
Similarly, PRSschizophrenia2 showed no relationship with response to antipsychotics, in any diagnostic group (see Table 8).
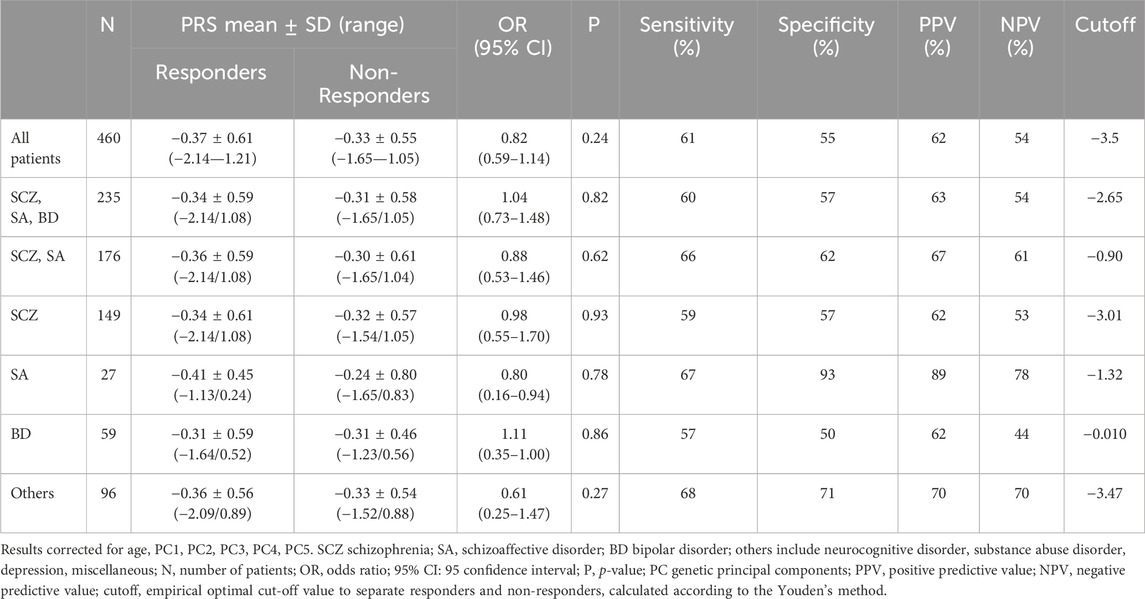
TABLE 8. PRSschizophrenia2 values in responders and non-responders and logistic multivariate regression analysis between PRSschizophrenia and response to treatment.
Discussion
The present study aimed to determine whether PRSresponse, integrating SNPs previously associated with response to antipsychotics, predict the likelihood of response to antipsychotics in a naturalistic cohort including multiple diagnostic categories. As a secondary aim, we also evaluated the relationship of response to antipsychotics with PRSschizophrenia1 and PRSschizophrenia2, PRSresponse was significantly associated with response to antipsychotics when considering the whole cohort and 3 subgroups: one composed of patients with schizophrenia; one of patients with schizophrenia or schizoaffective disorder (i.e., the schizophrenia spectrum); and one of patients with schizophrenia, schizoaffective disorder or bipolar disorder. No association was found in subgroups of patients with schizoaffective disorder, with bipolar disorder, with depression, or with another diagnosis, which could be also due to the smaller number of patients.
As indicated by p-values when considering diagnostic sub-groups, the statistical significance in the whole cohort and in multiple-diagnoses subgroups seemed driven by patients with schizophrenia, while relevant relationships were absent for the other diagnoses. However, odds-ratio were similar among different diagnostic groups, and the sample sizes were smaller for diagnosis other than schizophrenia; as a consequence, due to low statistical power, the diagnosis-specific character of PRSresponse remains to be determined.
Subsequent analysis for all diagnostic groups revealed modest sensitivity, specificity, PPV and NPV; these findings indicate that PRSresponse cannot be translated into a predictive tool for clinical practice. One exception is represented by the group with schizoaffective patients, in which we values of sensitivity and specificity were notheworthy. However, the corresponding p-value and odds ratios were not significant, cancelling the relevance of the sensitivity-specificity values.
Of note, when considering individually each single SNP composing PRSresponse, no statistically significant association was found with response to treatment, indicating that PRSresponse has a stronger relationship with response to treatment than single SNPs.
No significant associations with response to treatment were found for both PRSschizophrenia1, and PRSschizophrenia2, indicating that, at least in the present cohort, genes involved in response to antipsychotic treatment are stronger determinants of response than genes involved in risk of schizophrenia. We explored the relationship of PRSschizophrenia1 and PRSschizophrenia2 with response to treatment in order to embrace a different approach, not considering candidate genes but instead the summary statistics of a large GWAS to build the polygenic risk score. Present results are in contrast with a previous study that discovered a significant relationship between PRSschizophrenia and response to antipsychotics (Zhang et al., 2019). However, many differences in methods can explain the contrasting outcome: the latter study included a higher number of patients (N = 510), all patients were diagnosed with a first episode of schizophrenia; all 108 loci from the seminal study of the Psychiatric Genomic Consortium (Schizophrenia Working Group of the Psychiatric Genomics Consortium, 2014) were used to build PRSschizophrenia, while 14 were missing in our dataset; PRSschizophrenia was the weighted sum of SNPs; a different definition of response to treatment was used; first-generation antipsychotics were used more often than in the present cohort; and multiple ethnicities were considered, while the present study focused on patients of European descent.
Two other studies examined the relationship between PRSschizophrenia and treatment-resistant schizophrenia, with one (Werner et al., 2020) positive and one negative result (Wimberley et al., 2017). Also, these studies differed from the present one in several ways (number of patients, rating scale used to evaluate response, duration of the antipsychotic trial, and the weighting of SNPs included in the PRS).
Three other studies on the relationship between a PRS and response to antipsychotics have been published, in which PRS was based on a case-control GWAS design, using all loci available from a large cohort’s summary statistics. Therefore, the primary objective of GWAS analysis performed in these studies was to build a PRS and not to find single loci significantly associated with response to antipsychotics, which was the case of the source studies used to build PRSresponse in the present one. The underlying differences in methodology leaded to different SNP selection (in particular, p-value thresholds for statistical significance), with a lower number of SNPs included in the PRSs in the present study. A case-control study of patients with first-episode psychosis treated with risperidone failed to establish a significant association between a PRS and response (evaluated with the positive and negative syndrome scale), even though significant associations were found with some secondary outcomes (Santoro et al., 2018). A second study classified schizophrenia into distinct subtypes with different dimensions of genetic risk, using an ensemble of 10 GWAS-based PRSs for comorbid traits. The analysis produced 5 clusters of patients differing for positive, negative and cognitive symptom improvement (Chen et al., 2020). A third study found a significant association between a PRS and treatment-resistant schizophrenia, even if the proportion of variance explained was low (Pardiñas et al., 2022). Differences of methodology (e.g., construction of the PRS) and clinical characteristics (e.g., number of patients, age, diagnosis, number of psychotic episodes, type of antipsychotics) between the present and the abovementioned studies (Pardiñas et al., 2019; Chen et al., 2020; Pardiñas et al., 2022) make it difficult to compare results. However, no PRSresponse has a sufficient predictive power to be used in clinical practice, genetic factors explaining the variability in response to antipsychotics s only to a limited extent.
Of note, even if the selection of SNPs in the present study is based on GWAS studies, which is a probabilistic approach making no assumptions on the function of selected genetic variants, it is noteworthy that most of the SNPs are in genes linked to the pathogenesis of psychosis and/or to response to antipsychotics. Only rs10023464, rs7668556 and rs7395555 are located in intergenic regions, with no known regulatory role (Clark et al., 2013; Ensembl, 2024). Rs6688363 is in ATP1A2 (Seabra et al., 2020), coding for a Na/K ATPase expressed at multiple levels in the central nervous system (Ensembl, 2024), including the basal ganglia (Yue et al., 2009) and, therefore, possibly influencing the neuronal electrical activity. Rs10170310 is in SPOPL (Ensembl, 2024), involved in the Hedgehog pathway and in the ubiquitin-proteasome system (Seabra et al., 2020), possibly affected by antipsychotic administration (Sacchetti et al., 2017). Rs2133450 is in the glutamate metabotropic receptor GRM7 (Stevenson et al., 2016) and rs1875705 in the NMDA receptor subunit GRID2 (Stahl, 2018), likely modulating glutamatergic neurotransmission, whose dysfunction contributes to schizophrenia (Schwartz et al., 2012; Smith et al., 2020). Rs17382202 is in the phosphodiesterase 4D (PDE4D) gene (Ensembl, 2024), encoding for a key enzyme in nitric oxide neurotransmission (Yue et al., 2009), whose role in the pathogenesis of schizophrenia is under investigation. Rs711355 is in the gene coding for TJP1, a protein involved in the formation of brain tight junctions (Ensembl, 2024). Rs12767583 (Leucht and Engel, 2006) is in the coding region of CYP2C19 (Jürgens et al., 2020), and can therefore influence the response to treatment modulating the availability of its substrate clozapine (Najjar et al., 2013). Rs2980976 is located on TNFRSF1A (Ensembl, 2024), coding for a protein belonging to the family of TNF-alfa receptors, expressed in the brain and playing a role in the pathway of NFkB (Yue et al., 2009), a protein involved in immune response, neuroinflammation being an established feature of many mental disorders, including schizophrenia and bipolar disorder (Leucht et al., 2007).
The present study has several limitations. The number of patients is modest, and the antipsychotics under study are heterogeneous for mechanisms of actions and doses prescribed. Included patients had a hetegenous history of medication, from first antipsychotic treatment to multiple treatments and treatment resistance. However, a careful preliminary univariate statistical analysis was conducted to assess the influence of confounders on response to treatment. Only patients of European descent were included and results cannot be extrapolated to other ethnicities. Diagnostic categories evaluated were heterogeneous, including not only schizophrenia and bipolar disorder but also other categories in which antipsychotics are often prescribed off-label. Even if this choice introduced further variability, it aimed to expand the knowledge on antipsychotics pharmacogenomics beyond schizophrenia and bipolar disorder. Moreover, to address this possible limitations, the analyses were conducted not only at the whole cohort level but also in single-diagnosis subgroups. Major limitations of this study concern the criteria for response to antipsychotics, not based on the standard use of Positive and Negative Syndrome Scale (PANSS) or of Brief Psychiatric Rating Scale (BPRS) (Leucht and Engel, 2006). However, CGI demonstrated a high level of concordance with PANSS and BPRS in evaluating psychosis severity and antipsychotic treatment outcome and proved to be an effective tool to these ends (LEON et al., 1993; Rabinowitz et al., 2006; Rabinowitz et al., 2010). In addition, compared to PANSS and BPRS, CGI offers the advantage of being applicable to all mental disorders, fitting the present study design. Lastly, CGI measures were shown to have good internal consistency and concurrent validity (Purgato and Barbui, 2013). Indirect indicators of treatment efficacy (i.e., switch to a different antipsychotic, add-on of a novel antipsychotic or mood stabilizer, long duration of an antipsychotic treatment without dose increase) were used when CGI was not available. Even if their use as a criteria for response is to our knowledge unprecedented, according to our clinical experience, they are strongly related to efficacy of treatment, especially when side effects as a cause for treatment discontinuation have been ruled out, as in the present study.
A possible issue related to response criteria was the fact that response to treatment was a dichotomic outcome. Dichotomizing a variable as response to treatment poses several issues such as an underestimate of the extent of variation in outcome between groups, a loss of statistical power and an increase in the risk of a type 1 error. Moreover, the definition of a cut-point could be arbitrary, and produces large differences in proportions. On another side, keeping data continuous forces to express results in terms of mean and standard deviations, while in clinical practice to label individuals as having or not having an outcome makes the interpretation of results easier. Moreover, dichotomization allows to use measures such as risk difference, relative risk and odds ratio and it simplifies statistical analysis (Altman and Royston, 2006; Leucht and Lasser, 2006). As a matter of fact, gold standard criteria to determine response to antipsychotics revolve around a binary definition of response as a 50% reduction in a rating scale score such as BPRS or PANSS (Leucht et al., 2007; Cole et al., 2019). In our criteria for response a variation in the score of CGI-S and CGI-I was used in a way mirroring the change in BPRS or PANSS indicated above (LEON et al., 1993; Rabinowitz et al., 2006).
Age range was wide, and represented a relevant source of heterogeneity. In fact, an earlier onset of the disease is a negative prognostic factor for schizophrenia and for response to treatment (Chandola and Jenkinson, 2000; Carbon and Correll, 2014; Bozzatello et al., 2019); since our population included young patients, age of onset was mirrored by age at the evaluation. Moreover, older patients have on the average a longer disease duration and received many different medications. This is relevant since the probability of response to antipsychotics is higher at the first episode of psychosis, and decreases after multiple acute episodes (Haddad and Correll, 2018). Then, changes in biochemistry, functional connectivity, vasculature, synapsis remodeling and neuronal death occurs during brain aging and maturation, possibly provoking differences in AP pharmacodynamics (Shi and Klotz, 2011) Last, changes in pharmacokinetics related to age can influence the bioavailability of AP in the brain and the other bodily compartments63. However, our target was to realize a real-world study including heterogeneous patients, in order to have results to extend to all subjects prescribed with antipsychotics. In order to control the possibly confounding effect of age, it was included in the regression analysis model.
In conclusion, the present study shows that genetic factors previously associated in GWAS studies to response to antipsychotics but not to schizophrenia are associated with response to treatment in schizophrenic patients from a naturalistic cohort study. On the other hand, this result cannot be translated into a clinical tool to predict response.
Data availability statement
Due to the sensitivity of the data and the absence of informed consent for public data depository, the datasets analyzed during this study are not available to the public. The dataset supporting the conclusions of this article were obtained from PsyMetab study. Requests to access the datasets should be directed to: cmVzZWFyY2gucHN5bWV0YWJAY2h1di5jaA==.
Ethics statement
The studies involving humans were approved by Ethics Committee of the Canton of Vaud (CER-VD) Lausanne University Hospital. The studies were conducted in accordance with the local legislation and institutional requirements. The participants provided their written informed consent to participate in this study.
Author contributions
MD: Conceptualization, Data curation, Formal Analysis, Investigation, Methodology, Writing–original draft, Writing–review and editing. MF: Methodology, Supervision, Writing–review and editing. GP: Formal Analysis, Writing–review and editing. AV: Supervision, Validation, Writing–review and editing. MC: Methodology, Validation, Writing–review and editing. FM: Validation, Supervision, Writing–review and editing. FG: Supervision, Validation, Methodology, Visualization, Writing–review and editing. PC: Methodology, Supervision, Validation, Writing–review and editing. C-BE: Supervision, Validation, Conceptualization, Data curation, Formal Analysis, Methodology, Project administration, Writing–review and editing.
Funding
The author(s) declare financial support was received for the research, authorship, and/or publication of this article. This work has been funded in part by the Swiss National Research Foundation (CE and PC: 320030-120686, 324730-144064, and 320030-173211; CBE, PC and KJP: 320030-200602).
Acknowledgments
Claire Grosu, Marianna Piras, Celine Dubath, and Nermine Laaboub for their contributions in data handling and statistical analysis.
Conflict of interest
The authors declare that the research was conducted in the absence of any commercial or financial relationships that could be construed as a potential conflict of interest.
Publisher’s note
All claims expressed in this article are solely those of the authors and do not necessarily represent those of their affiliated organizations, or those of the publisher, the editors and the reviewers. Any product that may be evaluated in this article, or claim that may be made by its manufacturer, is not guaranteed or endorsed by the publisher.
References
Allen, J. D., and Bishop, J. R. (2019). A systematic review of genome-wide association studies of antipsychotic response. Pharmacogenomics 20 (4), 291–306. doi:10.2217/pgs-2018-0163
Altman, D. G., and Royston, P. (2006). The cost of dichotomising continuous variables. BMJ 332 (7549), 1080–1081. doi:10.1136/bmj.332.7549.1080
Bozzatello, P., Bellino, S., and Rocca, P. (2019). Predictive factors of treatment resistance in first episode of psychosis: a systematic review. Front. Psychiatry 10, 67. doi:10.3389/fpsyt.2019.00067
Cantù, F., Ciappolino, V., Enrico, P., Moltrasio, C., Delvecchio, G., and Brambilla, P. (2021). Augmentation with atypical antipsychotics for treatment-resistant depression. J. Affect Disord. 280, 45–53. doi:10.1016/j.jad.2020.11.006
Carbon, M., and Correll, C. U. (2014). Clinical predictors of therapeutic response to antipsychotics in schizophrenia. Dialogues Clin. Neurosci. 16 (4), 505–524. doi:10.31887/DCNS.2014.16.4/mcarbon
Chandola, T., and Jenkinson, C. (2000). The new UK national statistics socio-economic classification (NS-SEC); investigating social class differences in self-reported health status. J. Public Health (Bangkok). 22 (2), 182–190. doi:10.1093/pubmed/22.2.182
Chen, J., Mize, T., Wu, J. S., Hong, E., Nimgaonkar, V., Kendler, K. S., et al. (2020). Polygenic risk scores for subtyping of schizophrenia. Schizophr. Res. Treat. 2020, 1638403–1638413. doi:10.1155/2020/1638403
Chen, Y. L., Chen, K. P., Chiu, C. C., Tai, M. H., and Lung, F. W. (2018). Early predictors of poor treatment response in patients with schizophrenia treated with atypical antipsychotics. BMC Psychiatry 18, 376. Published online. doi:10.1186/s12888-018-1950-1
Clark, S. L., Souza, R. P., Adkins, D. E., Aberg, K., Bukszár, J., McClay, J. L., et al. (2013). Genome-wide association study of patient-rated and clinician-rated global impression of severity during antipsychotic treatment. Pharmacogenet Genomics 23 (2), 69–77. doi:10.1097/FPC.0b013e32835ca260
Cole, J. H., Marioni, R. E., Harris, S. E., and Deary, I. J. (2019). Brain age and other bodily ‘ages’: implications for neuropsychiatry. Mol. Psychiatry 24 (2), 266–281. doi:10.1038/s41380-018-0098-1
Demjaha, A., Lappin, J. M., Stahl, D., Patel, M. X., MacCabe, J. H., Howes, O. D., et al. (2017). Antipsychotic treatment resistance in first-episode psychosis: prevalence, subtypes and predictors. Psychol. Med. 47 (11), 1981–1989. doi:10.1017/S0033291717000435
Dubath, C., Delacrétaz, A., Glatard, A., Vollenweider, P., Preisig, M., Richard-Lepouriel, H., et al. (2020). Evaluation of cardiometabolic risk in a large psychiatric cohort and comparison with a population-based sample in Switzerland. J. Clin. Psychiatry 81 (3), 19m12796. doi:10.4088/JCP.19m12796
Elie, D., and Rej, S. (2018). Pharmacological management of neuropsychiatric symptoms in patients with major neurocognitive disorders. J. Psychiatry & Neurosci. 43 (1), 69–70. doi:10.1503/jpn.170117
Ensembl (2024). Ensembl. https://asia.ensembl.org/index.html.
Haddad, P. M., and Correll, C. U. (2018). The acute efficacy of antipsychotics in schizophrenia: a review of recent meta-analyses. Ther. Adv. Psychopharmacol. 8 (11), 303–318. doi:10.1177/2045125318781475
Hershenberg, R., Gros, D. F., and Brawman-Mintzer, O. (2014). Role of atypical antipsychotics in the treatment of generalized anxiety disorder. CNS Drugs 28 (6), 519–533. doi:10.1007/s40263-014-0162-6
Jürgens, G., Andersen, S. E., Rasmussen, H. B., Werge, T., Jensen, H. D., Kaas-Hansen, B. S., et al. (2020). Effect of routine cytochrome P450 2D6 and 2C19 genotyping on antipsychotic drug persistence in patients with schizophrenia: a randomized clinical trial. JAMA Netw. Open 3 (12), e2027909. doi:10.1001/jamanetworkopen.2020.27909
Koromina, M., Koutsilieri, S., and Patrinos, G. P. (2020). Delineating significant genome-wide associations of variants with antipsychotic and antidepressant treatment response: implications for clinical pharmacogenomics. Hum. Genomics 14 (1), 4. doi:10.1186/s40246-019-0254-y
Leon, A. C., Shear, M. K., Klerman, G. L., Portera, L., Rosenbaum, J. F., and Goldenberg, I. (1993). A comparison of symptom determinants of patient and clinician global ratings in patients with panic disorder and depression. J. Clin. Psychopharmacol. 13 (5), 327–331. doi:10.1097/00004714-199310000-00005
Leucht, S., Davis, J. M., Engel, R. R., Kane, J. M., and Wagenpfeil, S. (2007). Defining ‘response’ in antipsychotic drug trials: recommendations for the use of scale-derived cutoffs. Neuropsychopharmacology 32 (9), 1903–1910. doi:10.1038/sj.npp.1301325
Leucht, S., and Engel, R. R. (2006). The relative sensitivity of the clinical global impressions scale and the Brief psychiatric rating scale in antipsychotic drug trials. Neuropsychopharmacology 31 (2), 406–412. doi:10.1038/sj.npp.1300873
Leucht, S., and Lasser, R. (2006). The concepts of remission and recovery in schizophrenia. Pharmacopsychiatry 39 (5), 161–170. doi:10.1055/s-2006-949513
Lieberman, J. A., Stroup, T. S., McEvoy, J. P., Swartz, M. S., Rosenheck, R. A., Perkins, D. O., et al. (2005). Effectiveness of antipsychotic drugs in patients with chronic schizophrenia. N. Engl. J. Med. 353 (12), 1209–1223. doi:10.1056/NEJMoa051688
Mackenzie, B., Souza, R., Likhodi, O., Tiwari, A., Zai, C., Sturgess, J., et al. (2010). Pharmacogenetics of antipsychotic treatment response and side effects. Therapy 7 (2), 191–198. doi:10.2217/thy.10.3
Martin, A. R., Daly, M. J., Robinson, E. B., Hyman, S. E., and Neale, B. M. (2019). Predicting polygenic risk of psychiatric disorders. Biol. Psychiatry 86 (2), 97–109. doi:10.1016/j.biopsych.2018.12.015
Najjar, S., Pearlman, D. M., Alper, K., Najjar, A., and Devinsky, O. (2013). Neuroinflammation and psychiatric illness. J. Neuroinflammation 10 (1), 43. doi:10.1186/1742-2094-10-43
National institute for Heath and Care excellence (NICE) (2014). Psychosis and schizophrenia in adults: prevention and management. Published online.
Pardiñas, A. F., Nalmpanti, M., Pocklington, A. J., Legge, S. E., Medway, C., King, A., et al. (2019). Pharmacogenomic variants and drug interactions identified through the genetic analysis of clozapine metabolism. Am. J. Psychiatry 176 (6), 477–486. doi:10.1176/appi.ajp.2019.18050589
Pardiñas, A. F., Smart, S. E., Willcocks, I. R., Holmans, P. A., Dennison, C. A., Lynham, A. J., et al. (2022). Interaction testing and polygenic risk scoring to estimate the association of common genetic variants with treatment resistance in schizophrenia. JAMA Psychiatry 79 (3), 260–269. doi:10.1001/jamapsychiatry.2021.3799
Pies, R. (2009). Should psychiatrists use atypical antipsychotics to treat nonpsychotic anxiety? Psychiatry 6, 29–37. Published online.
Pignon, B., Tezenas du Montcel, C., Carton, L., and Pelissolo, A. (2017). The place of antipsychotics in the therapy of anxiety disorders and obsessive-compulsive disorders. Curr. Psychiatry Rep. 19 (12), 103. doi:10.1007/s11920-017-0847-x
Pouget, J. G., Shams, T. A., Tiwari, A. K., and Muller, D. J. (2014). Pharmacogenetics and outcome with antipsychotic drugs. Dialogues Clin. Neurosci. 16 (4), 555–566. doi:10.31887/DCNS.2014.16.4/jpouget
PsyMetab (2021). PsyMetab. https://www.chuv.ch/fr/psychiatrie/dp-home/recherche/centres-et-unites-de-recherche/centre-de-neurosciences-psychiatriques-cnp/unite-de-pharmacogenetique-et-de-psychopharmacologie-clinique-uppc/psymetab.
Purgato, M., and Barbui, C. (2013). Dichotomizing rating scale scores in psychiatry: a bad idea? Epidemiol. Psychiatr. Sci. 22 (1), 17–19. doi:10.1017/S2045796012000613
Rabinowitz, J., Levine, S., and Martinez, G. (2010). Concordance between measures of functioning, symptoms, and change: examining the GAF, CGI-S, CGI-C, and PANSS. J. Clin. Psychopharmacol. 30 (4), 478–480. doi:10.1097/JCP.0b013e3181e7145f
Rabinowitz, J., Mehnert, A., and Eerdekens, M. (2006). To what extent do the PANSS and CGI-S overlap? J. Clin. Psychopharmacol. 26 (3), 303–307. doi:10.1097/01.jcp.0000218407.10362.6e
Rosenbluth, M., and Sinyor, M. (2012). Off-label use of atypical antipsychotics in personality disorders. Expert Opin. Pharmacother. 13 (11), 1575–1585. doi:10.1517/14656566.2011.608351
Ruopp, M. D., Perkins, N. J., Whitcomb, B. W., and Schisterman, E. F. (2008). Youden index and optimal cut-point estimated from observations affected by a lower limit of detection. Biometrical J. 50 (3), 419–430. doi:10.1002/bimj.200710415
Sacchetti, E., Magri, C., Minelli, A., Valsecchi, P., Traversa, M., Calza, S., et al. (2017). The GRM7 gene, early response to risperidone, and schizophrenia: a genome-wide association study and a confirmatory pharmacogenetic analysis. Pharmacogenomics J. 17 (2), 146–154. doi:10.1038/tpj.2015.90
Santoro, M. L., Ota, V., de Jong, S., Noto, C., Spindola, L. M., Talarico, F., et al. (2018). Polygenic risk score analyses of symptoms and treatment response in an antipsychotic-naive first episode of psychosis cohort. Transl. Psychiatry 8 (1), 174. doi:10.1038/s41398-018-0230-7
Schizophrenia Working Group of the Psychiatric Genomics Consortium (2014). Biological insights from 108 schizophrenia-associated genetic loci. Nature 511 (7510), 421–427. doi:10.1038/nature13595
Schwartz, T. L., Sachdeva, S., and Stahl, S. M. (2012). Glutamate neurocircuitry: theoretical underpinnings in schizophrenia. Front. Pharmacol. 3, 195. doi:10.3389/fphar.2012.00195
Seabra, G., de Almeida, V., Reis-de-Oliveira, G., Crunfli, F., Antunes, ASLM, and Martins-de-Souza, D. (2020). Ubiquitin–proteasome system, lipid metabolism and DNA damage repair are triggered by antipsychotic medication in human oligodendrocytes: implications in schizophrenia. Sci. Rep. 10 (1), 12655. doi:10.1038/s41598-020-69543-5
Sherman, R. E., Anderson, S. A., Dal Pan, G. J., Gray, G. W., Gross, T., Hunter, N. L., et al. (2016). Real-world evidence — what is it and what can it tell us? N. Engl. J. Med. 375 (23), 2293–2297. doi:10.1056/NEJMsb1609216
Shi, S., and Klotz, U. (2011). Age-related changes in pharmacokinetics. Curr. Drug Metab. 12 (7), 601–610. doi:10.2174/138920011796504527
Sjaarda, J., Delacrétaz, A., Dubath, C., Laaboub, N., Piras, M., Grosu, C., et al. (2023). Identification of four novel loci associated with psychotropic drug-induced weight gain in a Swiss psychiatric longitudinal study: a GWAS analysis. Mol. Psychiatry 28, 2320–2327. Published online May 12. doi:10.1038/s41380-023-02082-3
Smith, R. L., O’Connell, K., Athanasiu, L., Djurovic, S., Kringen, M. K., Andreassen, O. A., et al. (2020). Identification of a novel polymorphism associated with reduced clozapine concentration in schizophrenia patients—a genome-wide association study adjusting for smoking habits. Transl. Psychiatry 10 (1), 198. doi:10.1038/s41398-020-00888-1
Stahl, S. M. (2018). Beyond the dopamine hypothesis of schizophrenia to three neural networks of psychosis: dopamine, serotonin, and glutamate. CNS Spectr. 23 (3), 187–191. doi:10.1017/S1092852918001013
Stevenson, J. M., Reilly, J. L., Harris, M. S. H., Patel, S. R., Weiden, P. J., Prasad, K. M., et al. (2016). Antipsychotic pharmacogenomics in first episode psychosis: a role for glutamate genes. Transl. Psychiatry 6 (2), e739. doi:10.1038/tp.2016.10
Taipale, H., Tanskanen, A., Correll, C. U., and Tiihonen, J. (2022). Real-world effectiveness of antipsychotic doses for relapse prevention in patients with first-episode schizophrenia in Finland: a nationwide, register-based cohort study. Lancet Psychiatry 9 (4), 271–279. doi:10.1016/S2215-0366(22)00015-3
Takeuchi, H., Siu, C., Remington, G., Fervaha, G., Zipursky, R. B., Foussias, G., et al. (2019). Does relapse contribute to treatment resistance? Antipsychotic response in first-vs. second-episode schizophrenia. Neuropsychopharmacology 44 (6), 1036–1042. doi:10.1038/s41386-018-0278-3
Taylor, D. M., Barnes, T. R. E., and Young, A. H. (2018). The maudsley prescribing guidelines in psychiatry. 13. Wiley-Blackwell.
Trubetskoy, V., Pardiñas, A. F., Qi, T., Panagiotaropoulou, G., Awasthi, S., Bigdeli, T. B., et al. (2022). Mapping genomic loci implicates genes and synaptic biology in schizophrenia. Nature 604 (7906), 502–508. doi:10.1038/s41586-022-04434-5
Wasylyshen, A., and Williams, A. M. (2016). Second-generation antipsychotic use in borderline personality disorder: what are we targeting? Ment. Health Clin. 6 (2), 82–88. doi:10.9740/mhc.2016.03.82
Werner, M. C. F., Wirgenes, K. V., Haram, M., Bettella, F., Lunding, S. H., Rødevand, L., et al. (2020). Indicated association between polygenic risk score and treatment-resistance in a naturalistic sample of patients with schizophrenia spectrum disorders. Schizophr. Res. 218, 55–62. doi:10.1016/j.schres.2020.03.006
Wimberley, T., Gasse, C., Meier, S. M., Agerbo, E., MacCabe, J. H., and Horsdal, H. T. (2017). Polygenic risk score for schizophrenia and treatment-resistant schizophrenia. Schizophr. Bull. 43 (5), 1064–1069. doi:10.1093/schbul/sbx007
Wray, N. R., Goddard, M. E., and Visscher, P. M. (2007). Prediction of individual genetic risk to disease from genome-wide association studies. Genome Res. 17 (10), 1520–1528. doi:10.1101/gr.6665407
Yadav, D. (2020). Prescribing in borderline personality disorder – the clinical guidelines. Prog. Neurol. Psychiatry 24 (2), 25–30. doi:10.1002/pnp.667
Yatham, L. N., Kennedy, S. H., Parikh, S. v., Schaffer, A., Bond, D. J., Frey, B. N., et al. (2018). Canadian network for mood and anxiety treatments (CANMAT) and international society for bipolar disorders (ISBD) 2018 guidelines for the management of patients with bipolar disorder. Bipolar Disord. 20 (2), 97–170. doi:10.1111/bdi.12609
Yue, S., Chen, Y., and Cheng, S. Y. (2009). Hedgehog signaling promotes the degradation of tumor suppressor Sufu through the ubiquitin–proteasome pathway. Oncogene 28 (4), 492–499. doi:10.1038/onc.2008.403
Keywords: antipsychotics, personalized medicine, GWAS, response to treatment, polygenic risk score, single nucleotide polimorphism (SNP)
Citation: De Pieri M, Ferrari M, Pistis G, Gamma F, Marino F, Von Gunten A, Conus P, Cosentino M and Eap C-B (2024) Prediction of antipsychotics efficacy based on a polygenic risk score: a real-world cohort study. Front. Pharmacol. 15:1274442. doi: 10.3389/fphar.2024.1274442
Received: 08 August 2023; Accepted: 26 January 2024;
Published: 08 March 2024.
Edited by:
Vijay Suppiah, University of South Australia, AustraliaReviewed by:
Urs Heilbronner, LMU Munich University Hospital, GermanyCarolina Muniz Carvalho, Federal University of São Paulo, Brazil
Copyright © 2024 De Pieri, Ferrari, Pistis, Gamma, Marino, Von Gunten, Conus, Cosentino and Eap. This is an open-access article distributed under the terms of the Creative Commons Attribution License (CC BY). The use, distribution or reproduction in other forums is permitted, provided the original author(s) and the copyright owner(s) are credited and that the original publication in this journal is cited, in accordance with accepted academic practice. No use, distribution or reproduction is permitted which does not comply with these terms.
*Correspondence: Marco De Pieri, bWFyY28uZGVwaWVyaUBoY3VnZS5jaA==