- 1Innovation Research Institute of Chinese Medicine, Shandong University of Traditional Chinese Medicine, Jinan, China
- 2Northwestern Institute on Complex Systems, Northwestern University, Evanston, IL, United States
- 3College of Acupuncture and Massage, Shandong University of Traditional Chinese Medicine, Jinan, China
- 4Oxford Chinese Medicine Research Centre, University of Oxford, Oxford, United Kingdom
The therapeutic effects of traditional Chinese medicine (TCM) involve intricate interactions among multiple components and targets. Currently, computational approaches play a pivotal role in simulating various pharmacological processes of TCM. The application of network analysis in TCM research has provided an effective means to explain the pharmacological mechanisms underlying the actions of herbs or formulas through the lens of biological network analysis. Along with the advances of network analysis, computational science has coalesced around the core chain of TCM research: formula-herb-component-target-phenotype-ZHENG, facilitating the accumulation and organization of the extensive TCM-related data and the establishment of relevant databases. Nonetheless, recent years have witnessed a tendency toward homogeneity in the development and application of these databases. Advancements in computational technologies, including deep learning and foundation model, have propelled the exploration and modeling of intricate systems into a new phase, potentially heralding a new era. This review aims to delves into the progress made in databases related to six key entities: formula, herb, component, target, phenotype, and ZHENG. Systematically discussions on the commonalities and disparities among various database types were presented. In addition, the review raised the issue of research bottleneck in TCM computational pharmacology and envisions the forthcoming directions of computational research within the realm of TCM.
1 Introduction
Chinese herbal medicines have primarily originated from foods. Over long periods of practical living experience, the medicinal properties of many herbs were gradually established (Hou and Jiang, 2013; Gu and Pei, 2017). Subsequently, foods with therapeutic properties were progressively separated and designated for specialized use as medicines (Hou and Jiang, 2013; He et al., 2018; Long et al., 2022). Human foraging practices frequently entail the amalgamation of various food sources, a tendency that has played a significant role in the creation of Traditional Chinese Medicine (TCM) formulas (Hou and Jiang, 2013). From its inception, TCM may have involved the application of herbal combinations. Some of these herbal combinations were stable and clearly effective and were therefore documented and passed down as formulas through generations. This resulted in the creation of over 300,000 known formulas (Li et al., 2008), laying the foundation for clinical TCM treatments. However, pharmacological research of TCM formulas faces the significant challenge of analyzing combinations of 100 or more chemical compounds (which are also named components) per formula (Zhao et al., 2010). Statistics on the total amount of targets corresponding to each compounds in PubChem Bioassays database is 3.7 in average (Jalencas and Mestres, 2013; Hu et al., 2014). According to the number mentioned above, a given TCM formula could potentially regulate over 370 targets. Thus, the “one drug-one target” pharmacological research methodology is insufficient to explain the therapeutic effects and mechanisms of action associated with TCM formulas (Ding et al., 2020).
Deciphering the intricate pharmacological mechanisms associated with herbs and formulas is a monumental task for researchers in the field of TCM (Wang et al., 2021b; Li et al., 2022b). Due to the “black box” nature of complex biological systems, studies of formula efficacy would do well to take a more macroscopic approach (Yao et al., 2013; Huang et al., 2023), i.e., research needs be designed using a “system-to-system” framework for clinical and pharmacological investigations of entire formula instead of disassembling formulas and studying the components (Liang et al., 2012). This approach involves observing the relationships between formulas (input) and effects in biological systems (output). The research philosophy behind chemical drug development is fundamentally guided by reductionism, with antagonism serving as a primary principle (Saks et al., 2009). The key paradigm of drug discovery revolves around the creation of inhibitors or activators that specifically target particular molecular entities (Jendza et al., 2019; Gong et al., 2023). Over time, this approach has proven imperfect due to the discovery of off-target responses, which may have toxicological impacts or cause other side effects. Given the extensive range of enzymatic systems, classes, and isoforms that have been identified in biological systems, the development of many target-specific agents has relied on trial-and-error methodologies (Méndez-Lucio et al., 2016; Paydas, 2019). However, regulation of targets by formulas does not always require an extremely high level of specificity, and exceptionally high activity levels may not be necessary (Méndez-Lucio et al., 2016). Formulas themselves constitute complex systems, wherein synergistic interactions between components can lead to optimal effects to maximize impacts on the human biological systems (Chen et al., 2018). Research of the pharmacology associated with specific formulas therefore necessitates unveiling (or partially) of the “black box” that is synergistic interactions between components and their interactions with the human biological system. This requires accurate simulation of the alterations that occur in various nodes within the biological system due to regulation by a specific formula (Tan et al., 2019). The goal is to establish correlations (and ideally quantitative relationships) between changes in a formula and changes in clinical phenotypes.
Advances in computational biochemical analyses have ushered in a new age of TCM research (Barabási et al., 2011; Wang et al., 2022a). Cooperative regulation of multiple targets by multi-component medicine is an effective strategy for altering the output of complex systems (Csermely et al., 2005; Zhang et al., 2014; Ramsay et al., 2018). Mathematical models that reflect complex systems are exceptionally potent tools in systems biology research (Kitano, 2002; Liu and Barabási, 2016; Zhao et al., 2019). The advent of artificial intelligence (AI), particularly deep learning, has allowed the accumulation of TCM data with unprecedented depth and complexity (Chen et al., 2019). Studying a substantial number of effective formulas (rather than individual formulas) using phenotypes or clinical manifestations as outputs can allow elucidation of the intricate relationships among formulas, herbs, components, targets, phenotypes, and ZHENG. Computer science is a powerful tool that facilitates TCM research by allowing both establishment of relationships and large-scale collection of relevant data (Zhang et al., 2019a). However, it is crucial to exercise caution in utilizing such tools to ensure that results are grounded in reality. The establishment of trustworthy, accurate TCM databases will thus be a pivotal step in unraveling the complexities of herbs or formulas (Saks et al., 2009).
Research into the pharmacology underlying TCM necessitates the accumulation of extensive data for multiple parts of the TCM system: formulas, herbs, components, targets, phenotypes, and ZHENG (Han et al., 2017). Clear delineations of various relationships (e.g., formula-component, component-target, and target-phenotype relationships) are vital (Xu et al., 2021; Zhao et al., 2023b; Gan et al., 2023). Since 1960, databases have been developed and are now available for use in computational TCM research (Figure 1). In this review, we conduct a retrospective examination of the establishment of these databases, with a particular emphasis on comparison based on the inclusion of formula, herb, component, target, biological function, phenotype, and ZHENG data. We aim to consolidate and analyze the relationships between various entities within these databases, including formula-component, component-target, target-phenotype, and phenotype-ZHENG relationships. This review summarizes the trends, identifies gaps in the existing research, and suggests directions for future development of the databases related to systems pharmacology in TCM.
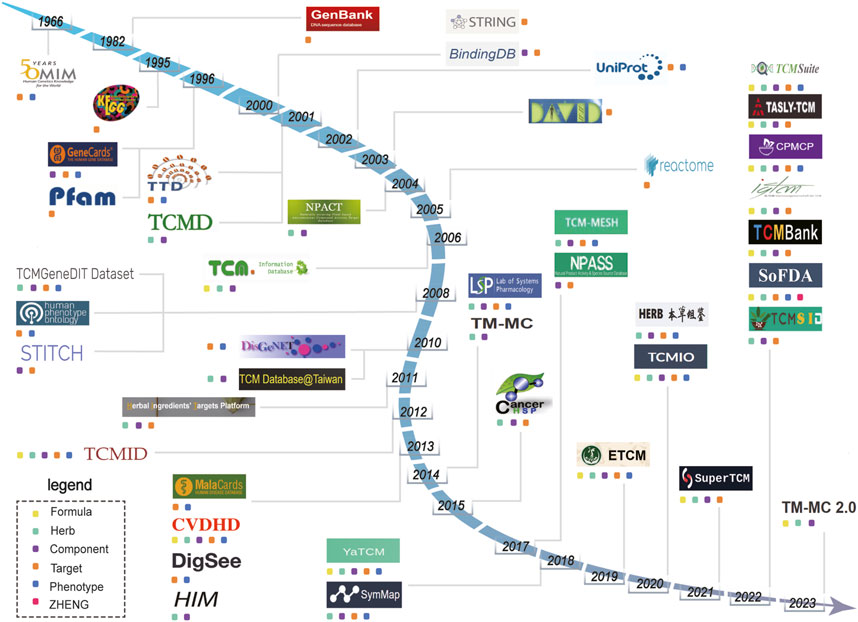
FIGURE 1. Timeline showing the establishment of databases related to traditional Chinese medicine (TCM) from 1966 to 2023. Different colored squares represent the main entity data contained in the database. The yellow squares represent “Formula”. The green squares represent “Herbs”. The purple squares represent “Components”. The orange squares represent “Target”. The blue squares represent “Phenotype”. The red squares represent “ZHENG”.
2 TCM formula databases
Initially, TCM formulas included only a small number of herbs. The herbs were consistently combined and administered in fixed proportions, which were documented and transmitted over time (Zha et al., 2015). With the evolution of medical practices, current formulas encompass not only ancient formulas but also modern empirical formulas and commercially prepared Chinese patent medicines. These formulas comprise the fundamental data within TCM formula databases, and there is a total of 21 such databases. These databases primarily contain fundamental information such as formula compositions, therapeutic functions, indications, and methods of use. Of the 21 databases, 18 are academic and 3 are commercial (Table 1). TCM-ID was one of the earliest TCM formula databases which brings the concept of formula-herb-component-target relationships in the form of databases (Chen et al., 2006). Chinese Medicine Think Tank (a big data analysis platform for TCM) houses the largest collection of TCM formula resources, including ∼300,000 formulas (Chinese Medicine Think Tank-a big data analysis platform for TCM, 2017). Over the Counter TCM Database and the Database of Standardized TCM Chinese Patent Drugs focus on marketed TCM formulas (Database of Standardized for Chinese patent drugs, 2017; OTC Chinese Herbal Medicine Database, 2017). These databases provide more comprehensive records of Chinese patent Drugs. DRUGDATAEXPY and Pharnexcloud are the major databases used in the pharmaceutical industry (DRUGDATAEXPY, 2009; Pharnexcloud, 2021). In addition to providing extensive formula resources, they also enable queries related to research, clinical trials, marketing, production inspections, and sales of specific formulas.
In 2016, with the emergence of databases like BATMAN-TCM that allow for customized predictive analysis of the pharmacological mechanisms of TCM formulas, databases for TCM were no longer limited to the functions of browsing and searching (Liu et al., 2016). The development of database functions became more geared towards analysis and prediction, and it was only then that TCM formula databases began to be utilized in a truly meaningful way. The principles of herbal compatibility are crucial for the effectiveness of TCM formulas. Formula databases not only allow for direct retrieval of herbal composition of formulas but also enable algorithmic-based analysis of the patterns governing herbal combinations. Through analysis of existing TCM formulas, CPMCP has summarized frequently used herbal combinations in TCM clinical practice. This functionality has helped to uncover the habitual pairings and contraindications between various herbs, shedding light on principles of herbal compatibility (Sun et al., 2022). Huabing data, an intelligent TCM big data platform, enables screening of disease-related formulas based on input herbal combinations. It dynamically calculates and analyzes the top 20 herbs, symptoms, diseases, and functions related to the treatment of certain diseases using formulas present in the database (Huabing data platform, 2023). TCMIP allows the selection of target TCM formula groups based on criteria such as formula composition and primary diseases treated. It calculates the frequency of herb usage, herb properties, inter-herb associations, and the frequency of formula targets within a selected formula group (Wang et al., 2021a). This approach facilitates innovative research of TCM formulas. For example, researchers have constructed a scoring system for the post-effects of drug combinations based on formula-herb relationships. The scoring system is utilized to recommend the most effective herbal combinations for certain diseases (Niu et al., 2023). The use of analytical algorithms on data from these databases can accelerate explorations of the intricate networks underlying TCM formula efficacy (Wang et al., 2021b).
One effective approach to simplifying the study of the pharmacological mechanisms of formulas is to make use of databases such as, ETCM, TCMIP, LTM-TCM, TCM-ID, which enable direct prediction of the pharmacological mechanisms of formulas (Chen et al., 2006; Xu et al., 2019; Wang et al., 2021a; Li et al., 2022a; Zhang et al., 2023). However, it's important to note that the quality of data and the dimensions covered vary among these databases, which can impact the accuracy of predictive results. Further evaluation is necessary for the data in these databases. Besides of that dosage and usage have varied significantly between regions and dynasties (Zha et al., 2015). Therefore, the results of the verification of the usage and dosage of individual herbs within formulas, as well as the sources of this information, should also be indispensable data within the database. The dosage of an herb significantly determines the concentrations of its components within the human body fluid and then may impacts the activity of component’s targets, which would be the key logic of both wet and dry experiments. However, most databases have not effectively cleaned dosage-related data during the inclusion of herbal formulas and data cleaning processes. Systematic research of ancient texts is essential to methodically organize and standardize ancient formulas. This highlights the needs of establishing uniform, rigorous standards, and quantitative dosage information for TCM data (Wang et al., 2021b).
3 Herb databases
Ancient Chinese people, through extensive medical practices over a long period of time, experimented with many medicinal substances derived from animals, plants, minerals, microorganisms, and other sources (Wang et al., 2017). These substances were documented, and their functions continuously verified over time. At present, TCM herbs encompass plant-based medicines, animal-derived medicines, and mineral-based medicines, with plant-based medicines being the predominant category. Herb databases are commonly used to compile fundamental information about herbs, such as properties, meridians, regions of usage, flavors, effects, and indications. This information came from various sources, including the Pharmacopoeia of the People’s Republic of China (ChP-TCM), the fourth national survey on Chinese Materia Medica Resources, books, literatures, and dictionaries. A total of 24 databases related to herbs have been identified, 21 of which are academic and 3 of which are commercial (Table 2). The Pharmacloud database contains the largest number of herb resources at −18,000 (Pharnexcloud, 2021). TCMID holds the distinction of being the academic database with the most extensive collection of herb resources, encompassing a total of 10,846 (Xue et al., 2012). China’s multi-ethnic composition means that various ethnic groups have discovered numerous herbs rooted in their own cultural practices. To enhance drug development, databases related to herbs from different ethnic groups have also been established, including databases for Tibetan, Mongolian, Uyghur, and Yao medicine.
The relationships between herbs and active components are currently key areas of focus in herb research (Fu et al., 2014; Zeng et al., 2022b). These linkages are included in herb databases. Active components are not only the primary materials that compose herbs but are also crucial for their therapeutic effects (Liu C. et al., 2018a). In 2018, following an update to the TCMID, there was a significant improvement in the coverage of herbs (Huang et al., 2018). Additionally, it introduced mass spectrometry (MS) data for these herbs, which served the purpose of distinguishing differences in the quality of herbs. Quantitative data for the characteristic components in each herb, as specified in the ChP-TCM, are available in the, ETCM and TCMIP databases (Wang et al., 2021a; Zhang et al., 2023). SymMap annotates components in four categories based on experimental MS data from ChP-TCM and from the literatures: quality control components, blood components, metabolite components, and other components (Wu et al., 2019). Utilizing herb-component relationship information from such a database, it is possible to construct more intricate features for herbs. This can be achieved, for example, by building heterogeneous herb-component-target networks. Such efforts enhance the accuracy of intelligent formula recommendation systems based on deep learning, such as FordNet (Zhou et al., 2021). Herb-component-target relationships in these databases also enable researchers to measure the effectiveness of specific herbs in treating diseases. This approach can then be used to identify herbs that are highly associated with specific diseases based on the importance of a particular target within a disease network (Wang et al., 2021d; Niu et al., 2023). For the identification of biological components in TCM, TCM-Suite gathered sequences and associated information for six marker genes: ITS2, matK, trnH-psbA, trnL, rpoC1, and ycf1 (Yang et al., 2022). Therapeutic efficacy of herbs is associated with the components and its content in the herb. A counterpart example in compound chemical drug is that there are fixed usage ratios for the synergistic effects of components (Ferrannini et al., 2022). For example, a fixed-ratio combination of insulin glargine and lixisenatide can better control the blood sugar levels in patients with diabetes (Aroda et al., 2016). Inappropriate ratios can lead to opposite effects (Létinier et al., 2023). In the context of components in herbs, the same principle holds true. Therefore, establishment of the herb-component relationships also requires the critical quantitative information-the content of components in herbs (Heinrich et al., 2022). Currently, there is a substantial accumulation of research on the identification and content measurements of components in herbs, including high-performance liquid chromatography, high-performance liquid chromatography-MS, and gas chromatography-MS, etc. (Arrizabalaga-Larrañaga et al., 2021; Papatheocharidou and Samanidou, 2023), but there is still a lack of databases for comprehensive aggregation and compilation of quantitative research data on components in herbs.
4 Component databases
Regardless of whether a so-called “herb” of interest is a plant, animal product, mineral-based medicine, the active components of which are chemical substances. Herbal component databases include information about the chemical components that have been extracted or isolated from single herbs or formulas. Such databases source their data from the literature, experimental data, and/or preexisting databases, encompassing essential details such as chemical structure, and CAS registry number of component. A total of 28 databases related to chemical components in TCM herbs have been identified (Table 3).TCM-Suite has the largest number of TCM chemical components at 704321 components, but it only 54,868 herb-component relationships (Yang et al., 2022). Some of these databases are more specialized: TCMIO, CancerHSP, and NPACT primarily focus on collecting information about active components related to tumors, whereas CVDHD contains data about active components associated with cardiovascular diseases (Mangal et al., 2013; Tao et al., 2015; 2015; Liu et al., 2020). Databases for herb components specifically offer a wealth of resources for modern drug development (Fu et al., 2016; Zhang et al., 2023).
The relationships between components and targets represent a key link connecting two intricate systems: herbs and human biological systems (Stitziel and Kathiresan, 2017). Data mining and computational chemistry approaches are currently being used to collect and organize known component-target relationships and to predict and validate previously unknown component-target relationships (Chen et al., 2016). Several component databases TCM provide both information about known component-target relationships and functionalities for predicting such relationships. The HIT and HERB databases contain information about component-target relationships obtained through text mining of the literature (Fang et al., 2021; Yan et al., 2022). HIT categorizes component-target relationships component into three types: “Directly inhibit/activate,” “Indirectly inhibit/activate,” and “Enzyme substrate”. Users can refer to the associated literature to learn more about specific component-target relationships. More importantly, HIT facilitates automatic target mining and curation of “My-target” information from newly released PubMed literature (Yan et al., 2022).
For components lacking reported relationships with a target, several computational chemistry approaches have been significantly developed. These approaches include ligand-based methods, target-based methods, and target-ligand methods, all of which aim to predict relationships between components and proteins (Sadybekov and Katritch, 2023). The SwissTargetPrediction is a widely used web tool, available online since 2014, designed to predict the most probable protein targets of small molecules. Predictions are made using the similarity principle through reverse screening. In the latest updated version, the models have been recalculated, achieving a success rate of at least one correct human target in the top 15 predictions for more than 70% of external compounds (Daina et al., 2019). BindingDB is a database that focuses on relationships between small molecules and their corresponding targets. The BindingDB website provides specialized tools that leverage its extensive data collection, allowing researchers to generate hypotheses for protein targets of a given bioactive component or to predict components that are bound by a particular protein. Additionally, the website offers virtual component screening using methods like maximal chemical similarity, binary kernel discrimination, and support vector machines(Gilson et al., 2016). To meet the demand for predicting targets of components, component databases have also started incorporating target prediction functionality. SysDT is a model that was designed to predict potential targets of components within the TCMSP database (Ru et al., 2014). SysDT has demonstrated remarkable predictive performance for drug-target relationships (Yu et al., 2012). ETCM v2.0 uses a target identification method that is based on a two-dimensional ligand similarity search module within the D3CARP platform and utilizes data from Binding DB (Zhang et al., 2023). To enhance the accuracy of target prediction, TCMSID employs multiple target prediction methods, including similarity ensemble approach, SwissTargetPrediction, HitpickV2, PPB, PPB2, and CHEMBL (Zhang al., 2022a). LTM-TCM integrates component-target information from various sources, including the BATMAN-TCM, ChEMBL, and STITCH databases. LTM-TCM retains target scores from different sources to enable personalized target screening based on user-defined thresholds (Li et al., 2022a).
The systematic collection and organization of herb components in databases forms the foundation of target prediction to decipher the multiple pharmacological actions of a given compound. Target prediction methods have the potential to significantly shorten drug development timelines, but the accuracy of computational studies remains relatively low. In practice, even the most successful virtual screening campaigns typically result in only 10%–40% of candidate hits being confirmed through experimental validation (Sadybekov and Katritch, 2023). A multitude of virtual screening efforts produced predominantly discouraging outcomes. For instance, the antimalarial drug ebselen, which had been identified through an early virtual screening process, ultimately proved unsuccessful in clinical trials (Sadybekov and Katritch, 2023). Therefore, it is essential to conduct more comprehensive in vitro and in vivo studies and develop improved methods for evaluating the above results. These results recorded in online databases should also have clear indications of their sources, to aid researchers in assessing the reliability of the data.
5 Target and target-related biofunction databases
Targets are the smallest functional units within an organism, serving as the internal nodes of complex systems (Turkarslan et al., 2014). They carry out various functions in numerous pathways and phenotypic responses, acting as bridges between medicines and the human biological system (Pfister and Ashworth, 2017; Santos et al., 2017). Drug mechanisms of action involve interactions between components and their targets. The initial paradigm in this area posited that a single component would act on a single target (Koeberle and Werz, 2014). However, further research revealed that nearly all natural and human-synthesized components interact with multiple targets (Plazas et al., 2022). Target databases primarily encompass genetic and protein-related information. Existing types of target databases include drug target databases, disease target databases, and specific target databases. These databases typically include basic information such as the target type, function, and origin, which are often sourced from the literature. UniProt, NCBI, and GeneCards are examples of target databases that provide comprehensive genetic and protein sequence information along with functional details (Table 4) (Safran et al., 2010; Brown et al., 2015; The UniProt Consortium, 2023). ETCM, TCMID, YaTCM, HIT, HERB, DisGeNET, and other databases also include information about targets, but these primarily focus on the relationships between targets and components or diseases (Piñero et al., 2017; Li et al., 2018a; Huang et al., 2018; Huang et al., 2018; Xu et al., 2019; Fang et al., 2021; Yan et al., 2022; Zhang et al., 2023). They often therefore have a decreased emphasis on the functional details of targets. Researchers have used target databases for purposes such as analysis of target-phenotype relationships (e.g., SymMap) (Wu et al., 2019; Lv et al., 2023). Target relationships in the TCMSP, TCMID, and TCM-ID databases have been used to map symptom-related genes and herb-related targets to human protein interaction networks (Chen et al., 2006; Xue et al., 2012; Ru et al., 2014; Huang et al., 2018). Through analysis of their topological relationships within a network, the distances between gene nodes can be calculated to infer distances between symptom modules, providing information about symptom co-occurrence and similarity. This approach has been employed to evaluate herb effectiveness for specific symptoms. It is a robust method for deciphering the mechanisms of herb and for predicting early-stage drug efficacy for diseases of interest (Gan et al., 2023).
Biological pathways can be considered as subsystems within complex systems. They serve as a framework for conducting pharmacological TCM research. These pathways can provide explanations for the complex mechanisms that link herbs to physiological changes. They often play significant roles in elucidation of interactions between drugs and biological functions (Wang et al., 2022b). Many target function databases integrate information about genes and genomes with higher-level functional annotations (Zeeshan et al., 2020). These data can then be used to systematically analyze gene functions based on known biological processes in an organism. Such databases are thus commonly utilized in conducting gene functional enrichment analyses, pathway-related analyses, and protein-protein interaction analyses. KEGG is a reference database for biological interpretation of genome sequences and other high-throughput data. The primary functionalities for biological process analyses are biochemical pathway mapping, metabolic network construction, genome comparison and merging, and enzyme database construction for target molecules (Kanehisa et al., 2017). BioCyc compiles and references genomes and metabolic pathways from thousands of sequenced organisms (Karp, 2005). Reactome systematically generates ordered molecular transformation networks, resulting in formation of classical metabolic maps. This database also associates human proteins with their molecular functions, offering a resource that serves as both a record of biological processes and a tool for discovering new functional relationships from data such as gene expression levels or mutations in tumor cells. Additionally, it can predict target biological processes of ion channels (Jassal et al., 2019). DAVID database consists of six tools: the functional annotation clustering, the functional annotation chart, the functional annotation table, gene functional classification, gene ID conversion, and gene name batch viewer (Sherman et al., 2022). The STRING database is used for analysis of protein-protein interactions. Individual protein queries generate a network composed of all proteins that interact with the queried protein (von Mering et al., 2003). This is particularly valuable for exploring interactions among input proteins; for example, it can be used to analyze the connections among differentially expressed proteins identified from proteomic data (Szklarczyk et al., 2021).
6 Phenotype databases
From the perspective of a complex system, the state of an organism corresponding to any abnormal phenotype is an abnormal steady state (Tyler et al., 2016). Such an abnormal steady state entails multiple nodes balance within the system. Likewise, interventions should target several nodes simultaneously to effectively restore the system to its normal steady state. Phenotype databases primarily focus on collecting data related to diseases, symptoms, and other phenotype-related entities. These databases provide robust datasets for those researching the mechanisms underlying TCM efficacy, primarily sourced from the literature and from other databases. Currently, a total of 13 databases have been compiled that provide detailed descriptions of diseases and symptoms (Table 5). TCMBank is the most comprehensive repository of disease-related resources, encompassing 32,529 data points (Lv et al., 2023).
The relationship between a target and the corresponding phenotype serves as a crucial bridge connecting a biological mechanism to the pathological manifestation in the human body. This connection was first established through the discovery of mutation-phenotype relationships. OMIM is a comprehensive repository that focuses on genetic and phenotypic data and interrelationships between the two. This database plays a pivotal role in naming and categorizing genetic phenotypes, thereby exerting a significant influence on the field of genetics (Funk et al., 2022). With the advent of the post-genomic era, the goal of deciphering the biological functions of target has evolved into the larger goal of delineating the intricate relationships between multiple genes and phenotypes. HPO and DisGeNET are comprehensive databases for analyzing and interpreting human gene-disease networks (Piñero et al., 2017; Köhler et al., 2021, 1). CPMCP and SymMap also include both TCM symptoms and modern medicine (MM) symptoms in an attempt to bridge TCM and modern medicine-based research through symptom associations (Wu et al., 2019; Sun et al., 2022).
7 ZHENG database
TCM involves a unique, intuitive understanding of physiological states. ZHENG differentiation and treatment (辩证论治) is the fundamental approach guiding clinical practice in TCM. Diagnoses and treatments are made by taking into account the individual differences between patients (Zhou et al., 2014; Wang and Zhang, 2017). ZHENG is a summary of the pathological and physiological discrepancies at each stage of a disease. It is also determined by factors such as the disease site and the nature of the disease (Wang et al., 2022a). TCM practitioners prescribe different formulas based on the ZHENG to achieve therapeutic efficacy. Explorations of ZHENG-formula and ZHENG-phenotype relationships represent a challenging area of research in both clinical practice and foundational TCM studies. A ZHENG database, SoFDA, has been constructed to record and collect ZHENG data (Zhang et al., 2022b). It includes both macroscopic data, such as ZHENG, phenotypes, and TCM formulas, and microscopic data (molecular mechanisms). Such databases promote a deeper understanding of ancient systematic medicine, TCM, and modern medicine. SoFDA implements two common association measures (Jaccard and Cosine similarity) to quantify relationships between clinical entities (e.g., ZHENG, phenotypes, and formulas). This allows users to compute the degree of indirect associations between the three entities in terms of six shared features: symptoms, genes, enriched gene ontology (GO) terms, enriched pathways, network modules, and network density. However, databases specifically focused on ZHENG are currently limited in number, and there are few comprehensive phenotypes analyses related to ZHENG.
8 Discussion
8.1 The emergence of network analysis triggered a surge in data generation and database construction
Database evolution is closely linked with current research trends and challenges over time (Sorokina and Steinbeck, 2020). Beyond serving as robust repositories for vast amounts of data, databases related to TCM systems pharmacology also represent pivotal milestones in summarizing the alternations of states in the TCM research. In this review, we retrospectively trace databases pertinent to computational analyses in TCM. Our primary focus is the detailed exploration and comparison of data structures within databases containing formula, herb, component, target, phenotype, and ZHENG data (Figure 1). Additionally, we delve into the intricate relationships between these entities within relevant databases. Systems biology is the cornerstone in the establishment of databases related to TCM systems pharmacology. It was until the emergence of component-target databases such as BindingDB, which summarize a large number of component-target relationships based on experimental data, allow mathematical simulation of component-target relationships, effectively addressing the challenge of identifying targets for numerous components (Gilson et al., 2016; Mendez et al., 2019). The emergence of component-target relationships as an area of study has bridged the gap between TCM and biological systems. In 2007, Yildirim et al. applied the principles of network biology by integrating and analyzing drug-gene and drug-protein interaction data. Their work revealed that the majority of drugs exert their effects through indirect modulation rather than direct targeting of disease-associated proteins (Csermely et al., 2005). Building upon this foundation, Hopkins proposed the research methodology of network analysis in pharmacology. He posited that drugs act on multiple targets and demonstrated enhanced efficacy and reduced toxicity through interactions among these multiple targets (Hopkins, 2008). The field of network analysis, which answers research questions from an inherently integrated standpoint, coincides remarkably well with the fundamental principles of TCM (Li and Zhang, 2013; Wang et al., 2021b). Over the course of Chinese history, thousands of herbs and over 300,000 formulas have been applied as medicines (Li et al., 2008). Often, the certain single herb appears in multiple formulas, each of which yields a distinct effect (Wang et al., 2021d). Compared to commercially available synthetic drugs, herbs exhibit a larger quantity of components with higher complexity. Consequently, there is a greater need to collect and organize information to uncover the patterns associated with herbal combinations and their therapeutic effects. Possibly driven by this rationale, the TCMID database was launched in 2012, including relationships between and among formulas, herbs, components, targets, and phenotypes. The inception of this database marked the emergence of the core chain of pharmacological research using herb (Xue et al., 2012; Huang et al., 2018). Subsequent databases related to TCM systems pharmacology have largely promoted establishment of relationships between and among the same entities. However, these newer databases have also offered enhanced capabilities for computational analyses.
In TCM, the stable coexistence of various clinical manifestations is defined as ZHENG, which is also the integrative description on the current status of complex biological system (Tang et al., 2008). Empirical explorations in TCM focus on establishing direct relationships between formulas and ZHENG. For patients sharing common pathological characteristics, TCM practice calls for the use of similar but not entirely identical formulas (Wang et al., 202a). Diagnosing and treating patients based on ZHENG differentiation can enhance the clinical effectiveness of the treatments. The integration of ZHENG and modern personalized medicine approaches could serve as a breakthrough for addressing current challenges in medical practice (He et al., 2008; Su et al., 2012; Chen et al., 2013). A significant amount of omics research is employed to uncover the physiological mechanisms of patients with different ZHENG (Wu et al., 2021; Akhoundova and Rubin, 2022). Experimental studies have revealed that patients with different ZHENG, but the same disease exhibit distinct biomarkers (Shang et al., 2022). The accumulation of this data can provide more accurate features for computational analysis of ZHENG. The rapid development of AI has enabled the training with and analysis of large datasets and led to advancements in personalized medicine. AI has been utilized to learn from tongue images and clinical diagnostic information, aiding in clinical diagnosis (Kanawong et al., 2012; Tang et al., 2021; Chen and He, 2022). Several computational studies have described the use of information about relationships between targets, phenotypes, and symptoms to recommend appropriate clinical formulas (Li et al., 2007; Kanawong et al., 2012; Zhou et al., 2014). It has become possible to reveal the essence of ZHENG based on a wealth of information, including phenotypes, and AI model. It thus appears that the process through which herbs exert their therapeutic effects follows the core formula-herb-component-target-phenotype-ZHENG chain (Figure 2). In fact, this core relationship chain built with extensive data may aid us in exploring from one entity to another, e.g., starting from a drug entity to explore its clinical applications, it offers an approach to uncover new clinical uses of existing drugs, thereby expanding our understanding and utilization of pharmaceutical resources. Similarly, application in an opposite direction is recommending personalized medications based on clinical phenotype entity. This interconnectivity, grounded in large-scale data, provides a robust framework for enhanced drug discovery and personalized medicine. It enables the identification of tailored therapeutic solutions catering to individual patient’s unique clinical presentations and needs (Zhang et al., 2019b). Indeed, there have been studies that utilize such relationship chains for recommendation of personalized medication. Researchers have created gene expression profiles for 189 diseases, then analyzed the perturbation characteristics of herbs based on the herb-component-target relationships within the database. Finally, they predicted the optimal combinations of herbs for treating diseases based on the mapping relationship between herbs and diseases (Chen et al., 2018). However, these studies are still in their early stages of research, and a substantial number of experiments are needed before they can be applied in clinical research.
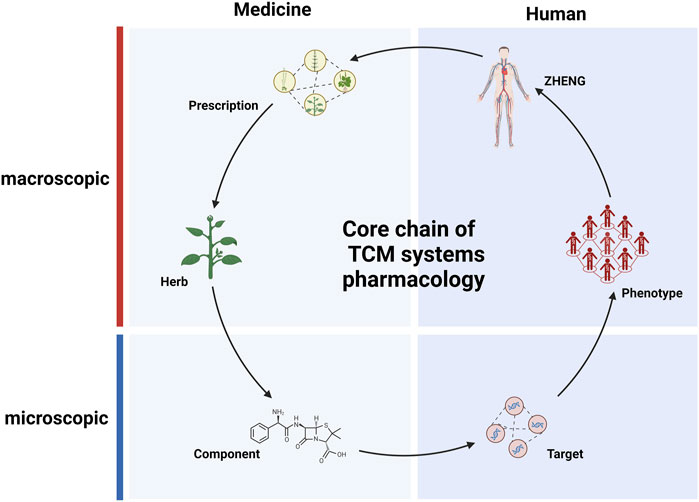
FIGURE 2. The central chain of pharmacological research in traditional Chinese medicine (TCM). Solid arrows represent primary relationships between entities within various hierarchical databases related to TCM.
Pharmacological research is concerned with the process of regulating biological systems through medications; TCM employs complex chemical systems to regulate biological systems (Liang et al., 2012). TCM research is based on the accumulation of extensive clinical experience, through which numerous associations between specific ZHENG and corresponding formula are established (Yang et al., 2020). Patients are primarily differentiated into subtypes to enable selection of suitable formulas (Wang et al., 2021c). Another aspect of TCM research involves discovering the efficacy of specific herbs for particular phenotypes. This allows for the incorporation of “specific herbs” into formulas, which are modified accordingly to address a specific patient’s disease state beyond the corresponding ZHENG. However, there is a lack of necessary research into the material bases and action mechanisms of formulas and herbs metioned above (Xu et al., 2019). This limitation has confined the development of new medical knowledge to the accumulation and extension of clinical experience. In contrast, modern medicine, which is based on chemistry and molecular biology, has be used to elucidate chemicalstructures, functions, and targets, providing modern pharmacology with an extremely precise perspective at the micro scale (Penrod et al., 2011; Zeeshan et al., 2020). However, the rate of new chemical drug production is slowing (Sadybekov and Katritch, 2023). During the development of modern pharmacology, a plethora of component-target relationships have been established (Santos et al., 2017). The establishment of these relationships has provided TCM research with numerous paradigms and methods. This, in turn, has endowed TCM pharmacology with the ability to unveil the formula systems regulating human biological systems, opening the “black box”. The core chain (formula-herb-component-target-phenotype-ZHENG) bridges the gap between macroscopic and microscopic levels; to some extent, it also explains the interactions between formula systems and the biological system at the molecular level (Figure 2). This framework has made it possible to conduct TCM systems pharmacology research.
8.2 Data accumulation encounters a research bottleneck in TCM computational pharmacology
To date, there has been a significant accumulation of data at various levels within the formula-herb-component-target-phenotype-ZHENG chain. The relationships between members of each level have been effectively organized and summarized in various databases. The research in TCM has leveraged the concept of networks, thereby advancing towards the approach with more characteristic’s systems science (Huang et al., 2017). However, in complex system, both formulas and modified herbal prescriptions are administered at specific quantities in practical applications, meaning that the herbal composition of formulas is quantitative, and the components within the herbs are quantitative (Luan et al., 2020). In the process of pharmacological research, the effective dosage of a drug is crucial and therefore carefully examined (Spencer and Jarvis, 1999). However, in the context of TCM databases and computational studies of TCM formulas, there are few quantitative calculations and little dosage information. Indeed, not only in TCM but also for active components in general, there have been few studies that provide absolute quantitative or relative quantitative (i.e., proportional) information. This approach raises doubts about the accuracy of computational predictions of the composition, efficacy, and mechanism of action associated with formulas. For instance, polyphenols could interact with multiple targets due to their unique nature of multiple hydrogen donor if not considering the effective concentration (Luca et al., 2020), but not all drug-target relationships identified through these methods necessarily translate into therapeutic effect, which presents one of the major limitations in in silico research. However, predictive research should apply “quantitative algorithm” to calculate the inhibition rate to the very target but not component-target relationship only; second, components must accumulate to a sufficient concentration around the target in cellular and animal experiments after passing through the cell membrane or even gastrointestinal tract and liver (Manukyan et al., 2019; Luca et al., 2020; Khojah et al., 2021).
Pharmacological research in TCM necessitates both qualitative and quantitative investigations of relationships between parameters in the “formula-herb-component-target-phenotype-ZHENG” chain. The relationships among these entities are highly intricate, constituting not one-to-one but rather many-to-many relationships. This complexity is reminiscent of neural networks, which are characterized by extensive intricate connections (Ma et al., 2014). Quantitative studies can be likened to parameters such as weights and biases in a neural network (Lu et al., 2022). In a previous study, the introduction of a novel coefficient aimed to replicate the proportional quantities of components relative to the weight of an herb of interest within a specific formula (Chu et al., 2020). This coefficient also serves to evaluate the pharmacological impact of antiarrhythmic herbal medicine Xin Su Ning capsule across various pertinent biological pathways (Wang et al., 2019b). However, the complex network of quantitative information requires systematic collection in relevant databases to facilitate systems pharmacology research of herbs.
8.3 Upcoming paradigm shifts in TCM pharmacological research
TCM databases offer a wealth of foundational data for pharmacological analyses of complex systems (e.g., formulas). They play pivotal roles in accelerating TCM-based computational science and pharmaceutical research. Moreover, these databases are essential for deciphering the intricate relationships among entities in the formula-herb-component-target-phenotype-ZHENG core chain. At present, such databases are primarily used for data retrieval rather than aiding in the discovery of new drugs/formulas or novel pharmacological mechanisms. However, many researchers have begun harnessing the extensive relationships described in databases such as those discussed here to simulate complex formulas. This approach aids in exploration of herb combination patterns (Niu et al., 2023), development of innovative drugs (Li et al., 2010), identification of mechanisms of disease intervention through herb (Gan et al., 2023), and enhancement of clinical research (Zhao et al., 2015; Wang et al., 2021c).
The essence of formulas and medicinal plants is a mixture of compounds. Referring to a single compound, the functionalities of chemical components are determined by their structures (Xiong et al., 2022). Chemical drugs exhibit limited structural diversity and target just over 700 different proteins. The constrained coverage of this chemical space is insufficient to address all modulable or pathological physiological mechanisms that occur in human disease states (Lipinski and Hopkins, 2004; Reymond, 2015; Stocker et al., 2020). Natural products, which are often referred to as single components in TCM, are numerous and display a wide range of chemical structures (Lachance et al., 2012). This diversity enables them to target a broader spectrum of receptors (Lipinski, 2016). These component therefore represent a valuable repository of potential therapeutic agents (Li et al., 2008). To date, a substantial body of research on TCM formulas has identified the key active components and core mechanisms of action (Zhang et al., 2017a; Wang et al., 2019a; Xu et al., 2020). This information continues to be instrumental in aiding the development of combination drugs composed of multiple components. Once the relationships between a significant number of components combinations and their therapeutic effects are understood, it becomes possible to create new formulas consist of those components based on specific requirements (Keith et al., 2005). This approach can minimize issues related to drug quality control and reduce the costs associated with drug development.
Advances in deep learning and foundation model (Hamet and Tremblay, 2017; Du et al., 2021) indicate that it is increasingly feasible to simulate the complex network encompassing the core chain. The emergence of foundation models is expected to provide tools with precise computational capabilities and entirely new perspectives on pharmacological calculations (Du et al., 2021; Zeng et al., 2022a). Additionally, foundation model-based generative AI has shown immense disruptive potential across various industries, including healthcare and medicine (Singhal et al., 2023b; Xiong et al., 2023). Currently, generative foundation models and medical models fine-tuned based on them have demonstrated strong general capabilities in many medical tasks (Singhal et al., 2023a). They have shown preliminary potential to simulate the corresponding relationships between the entities within the core chain. In the medical field, foundation models can be leveraged to perform various types of tasks, such as extracting key information from electronic health records and analyzing patient symptoms to make disease diagnoses (Xiong et al., 2023). These models can assist in automating data extraction and standardization procedures, leading to a substantial reduction in the time required to establish comprehensive medical databases (Singhal et al., 2023a). The integration of databases related to TCM with foundation models hold significant potential for establishing a knowledge graph in the field of TCM system pharmacology. This integration can enable the generation of knowledge graphs that encompass the relationships between various entities of TCM, formulas, herbs, ZHENG, and their pharmacological effects. It can facilitate the development of a question-and-answer system that provides relevant analytical solutions. Furthermore, the expansion of relevant data relationship dimensions in the systems pharmacology database may enhance the depth of computation in foundation models and improve the accuracy of computation. Using foundation model, a dataset comprising a substantial number of effective formulas and the corresponding phenotypes could be used to elucidate the intricate relationships among the entities of the core chain.
In summary, research on databases has made significant and substantial progress in recent times. A vast amount of data related to formula, herb, ZHENG, and diseases has been accumulated. A core chain of interrelated relationships has been established, linking the research entities. Furthermore, computational methods are now being employed to simulate and analyze the relationships between entities within this core chain. Currently, while there isn't a single database that can provide computational services to model the complex relationships among all entities in the core chain mentioned above, it's anticipated that with the advancement of technology, this stage is not too far off in the future. However, regarding the existing entities and relationships within the core chain, there are still numerous significant issues that cannot be overlooked. The presence of these issues poses a potential risk of failure in future computational pharmacological research (Sadybekov and Katritch, 2023). Most databases are interconnected resources, and even new databases are often updates or extensions of existing ones, with limited substantive changes to older data. An illustrative example is that some of these databases operate under the principle that if a specific chemical structure demonstrates activity, it's likely to have a similar effect on structurally similar sites. Therefore, we need more and better experiments for evaluation, and literature studies also contain many false positives/false negatives, so it's crucial to maintain clear data sources when incorporating them into the database. For the entire core chain, there is indeed the potential for quantitative calculations, which could enhance the rigor and accuracy of computational research in TCM. It's worth noting that there currently might not be corresponding databases or reports available to support this quantitative approach. It is hoped that in the future, databases will address this issue and foster greater collaboration between different domains, ultimately advancing the modernization and scientific exploration of TCM.
Author contributions
MF: Writing–original draft, Writing–review and editing. CJ: Writing–review and editing, Writing–original draft. DL: Writing–original draft. YD: Writing–original draft. LY: Writing–review and editing. YC: Writing–review and editing. Y-LM: Writing–review and editing. TW: Writing–review and editing.
Funding
The author(s) declare financial support was received for the research, authorship, and/or publication of this article. This work was supported by the National Natural Science Foundation of China (82374075 to TW), Taishan Scholar Youth Project of Shandong Province (TW). This work was also supported, in part, by National Natural Science Foundation of China (82274128 to LY), Joint Fund of Shandong Provincial Natural Science Foundation (ZR2021LZY020 to LY).
Conflict of interest
The authors declare that the research was conducted in the absence of any commercial or financial relationships that could be construed as a potential conflict of interest.
Publisher’s note
All claims expressed in this article are solely those of the authors and do not necessarily represent those of their affiliated organizations, or those of the publisher, the editors and the reviewers. Any product that may be evaluated in this article, or claim that may be made by its manufacturer, is not guaranteed or endorsed by the publisher.
References
Akhoundova, D., and Rubin, M. A. (2022). Clinical application of advanced multi-omics tumor profiling: shaping precision oncology of the future. Cancer Cell 40, 920–938. doi:10.1016/j.ccell.2022.08.011
Amberger, J. S., Bocchini, C. A., Schiettecatte, F., Scott, A. F., and Hamosh, A. (2015). OMIM.org: online Mendelian Inheritance in Man (OMIM®), an online catalog of human genes and genetic disorders. Nucleic Acids Res. 43, D789–D798. doi:10.1093/nar/gku1205
Aroda, V. R., Rosenstock, J., Wysham, C., Unger, J., Bellido, D., González-Gálvez, G., et al. (2016). Efficacy and safety of lixilan, a titratable fixed-ratio combination of insulin glargine plus lixisenatide in type 2 diabetes inadequately controlled on basal insulin and metformin: the lixilan-l randomized trial. Diabetes Care 39, 1972–1980. doi:10.2337/dc16-1495
Arrizabalaga-Larrañaga, A., Epigmenio-Chamú, S., Santos, F. J., and Moyano, E. (2021). Determination of banned dyes in red spices by ultra-high-performance liquid chromatography-atmospheric pressure ionization-tandem mass spectrometry. Anal. Chim. Acta 1164, 338519. doi:10.1016/j.aca.2021.338519
Barabási, A.-L., Gulbahce, N., and Loscalzo, J. (2011). Network medicine: a network-based approach to human disease. Nat. Rev. Genet. 12, 56–68. doi:10.1038/nrg2918
Benson, D. A., Cavanaugh, M., Clark, K., Karsch-Mizrachi, I., Ostell, J., Pruitt, K. D., et al. (2018). GenBank. Nucleic Acids Res. 46, D41–D47. doi:10.1093/nar/gkx1094
Brown, G. R., Hem, V., Katz, K. S., Ovetsky, M., Wallin, C., Ermolaeva, O., et al. (2015). Gene: a gene-centered information resource at NCBI. Nucleic Acids Res. 43, D36–D42. doi:10.1093/nar/gku1055
Chen, C. Y.-C. (2011). TCM Database@Taiwan: the world’s largest traditional Chinese medicine database for drug screening in silico. PloS One 6, e15939. doi:10.1371/journal.pone.0015939
Chen, H., and He, Y. (2022). Machine learning approaches in traditional Chinese medicine: a systematic review. Am. J. Chin. Med. 50, 91–131. doi:10.1142/S0192415X22500045
Chen, H.-Y., Chen, J.-Q., Li, J.-Y., Huang, H.-J., Chen, X., Zhang, H.-Y., et al. (2019). Deep learning and random forest approach for finding the optimal traditional Chinese medicine formula for treatment of alzheimer’s disease. J. Chem. Inf. Model. 59, 1605–1623. doi:10.1021/acs.jcim.9b00041
Chen, Q., Springer, L., Gohlke, B. O., Goede, A., Dunkel, M., Abel, R., et al. (2021). SuperTCM: a biocultural database combining biological pathways and historical linguistic data of Chinese materia medica for drug development. Biomed. Pharmacother. 144, 112315. doi:10.1016/j.biopha.2021.112315
Chen, X., Yan, C. C., Zhang, X., Zhang, X., Dai, F., Yin, J., et al. (2016). Drug–target interaction prediction: databases, web servers and computational models. Brief. Bioinform. 17, 696–712. doi:10.1093/bib/bbv066
Chen, X., Zheng, C., Wang, C., Guo, Z., Gao, S., Ning, Z., et al. (2018). Systems-mapping of herbal effects on complex diseases using the network-perturbation signatures. Front. Pharmacol. 9, 1174. doi:10.3389/fphar.2018.01174
Chen, X., Zhou, H., Liu, Y. B., Wang, J. F., Li, H., Ung, C. Y., et al. (2006). Database of traditional Chinese medicine and its application to studies of mechanism and to prescription validation. Br. J. Pharmacol. 149, 1092–1103. doi:10.1038/sj.bjp.0706945
Chen, X. Y., Ma, L. Z., Chu, N., Zhou, M., and Hu, Y. (2013). Classification and progression based on CFS-GA and C5.0 boost decision tree of TCM Zheng in chronic hepatitis B. Evid. Based Complement. Altern. Med. ECAM 2013, 695937. doi:10.1155/2013/695937
Chinese Herbal Formulas Database (2017). Available at: http://cintmed.cintcm.com/cintmed/searchIndex/basic.html?dbtype=zyfj&pdh=14 (Accessed September 1, 2023).
Chinese Medicine Formulae Image Database (2023). Available at: https://library.hkbu.edu.hk/electronic/libdbs/cmfid/index.html (Accessed September 1, 2023).
Chinese Medicine Think Tank- a big data analysis platform for TCM (2017). Available at: https://www.duguji.cn/login (Accessed September 1, 2023).
Chinese Traditional Medicine Chemical Ingredients Database (2017). Available at: https://cintmed.cintcm.com/cintmed/searchIndex/basic.html?dbtype=xdfj&pdh=7 (Accessed September 1, 2023).
Chu, X., Sun, B., Huang, Q., Peng, S., Zhou, Y., and Zhang, Y. (2020). Quantitative knowledge presentation models of traditional Chinese medicine (TCM): a review. Artif. Intell. Med. 103, 101810. doi:10.1016/j.artmed.2020.101810
CNKI-TCM formula knowledge base (2023). Available at: https://kb.tcm.cnki.net/TCM/TCM/Index?dbcode=ZYFF (Accessed August 30, 2023).
Csermely, P., Agoston, V., and Pongor, S. (2005). The efficiency of multi-target drugs: the network approach might help drug design. Trends Pharmacol. Sci. 26, 178–182. doi:10.1016/j.tips.2005.02.007
Daina, A., Michielin, O., and Zoete, V. (2019). SwissTargetPrediction: updated data and new features for efficient prediction of protein targets of small molecules. Nucleic Acids Res. 47, W357-W364–W364. doi:10.1093/nar/gkz382
Database of Standardized for Chinese patent drugs (2017). Available at: https://cintmed.cintcm.com/cintmed/searchIndex/basic.html?dbtype=xdfj&pdh=20 (Accessed September 1, 2023).
Ding, Z., Zhong, R., Yang, Y., Xia, T., Wang, W., Wang, Y., et al. (2020). Systems pharmacology reveals the mechanism of activity of Ge-Gen-Qin-Lian decoction against LPS-induced acute lung injury: a novel strategy for exploring active components and effective mechanism of TCM formulae. Pharmacol. Res. 156, 104759. doi:10.1016/j.phrs.2020.104759
Drugdataexpy, (2009). Available at: https://db.yaozh.com/fangji (Accessed September 1, 2023).
Du, Z., Qian, Y., Liu, X., Ding, M., Qiu, J., Yang, Z., et al. (2021). GLM: general language model pretraining with autoregressive blank infilling. doi:10.48550/arXiv.2103.10360
Fang, S., Dong, L., Liu, L., Guo, J., Zhao, L., Zhang, J., et al. (2021). HERB: a high-throughput experiment- and reference-guided database of traditional Chinese medicine. Nucleic Acids Res. 49, D1197–D1206. doi:10.1093/nar/gkaa1063
Fang, Y.-C., Huang, H.-C., Chen, H.-H., and Juan, H.-F. (2008). TCMGeneDIT: a database for associated traditional Chinese medicine, gene and disease information using text mining. BMC Complement. Altern. Med. 8, 58. doi:10.1186/1472-6882-8-58
Ferrannini, E., Niemoeller, E., Dex, T., Servera, S., and Mari, A. (2022). Fixed-ratio combination of insulin glargine plus lixisenatide (IGLARLIXI) improves ß-cell function in people with type 2 diabetes. Diabetes Obes. Metab. 24, 1159–1165. doi:10.1111/dom.14688
Fu, J., Wang, Z., Huang, L., Zheng, S., Wang, D., Chen, S., et al. (2014). Review of the botanical characteristics, phytochemistry, and pharmacology of astragalus membranaceus (Huangqi). Phytother. Res. 28, 1275–1283. doi:10.1002/ptr.5188
Fu, X.-M., Zhang, M.-Q., Shao, C.-L., Li, G.-Q., Bai, H., Dai, G.-L., et al. (2016). Chinese marine materia medica resources: status and Potential. Mar. Drugs 14, 46. doi:10.3390/md14030046
Funk, L., Su, K.-C., Ly, J., Feldman, D., Singh, A., Moodie, B., et al. (2022). The phenotypic landscape of essential human genes. Cell 185, 4634–4653.e22. doi:10.1016/j.cell.2022.10.017
Gan, X., Shu, Z., Wang, X., Yan, D., Li, J., Li, X., et al. (2023). Network medicine framework reveals generic herb-symptom effectiveness of traditional Chinese medicine. Sci. Adv. 9, eadh0215. doi:10.1126/sciadv.adh0215
Gilson, M. K., Liu, T., Baitaluk, M., Nicola, G., Hwang, L., and Chong, J. (2016). BindingDB in 2015: a public database for medicinal chemistry, computational chemistry and systems pharmacology. Nucleic Acids Res. 44, D1045–D1053. doi:10.1093/nar/gkv1072
Gong, G. Q., Bilanges, B., Allsop, B., Masson, G. R., Roberton, V., Askwith, T., et al. (2023). A small-molecule PI3Kα activator for cardioprotection and neuroregeneration. Nature 618, 159–168. doi:10.1038/s41586-023-05972-2
Gu, J., Gui, Y., Chen, L., Yuan, G., and Xu, X. (2013). CVDHD: a cardiovascular disease herbal database for drug discovery and network pharmacology. J. Cheminformatics 5, 51. doi:10.1186/1758-2946-5-51
Gu, S., and Pei, J. (2017). Innovating Chinese herbal medicine: from traditional health practice to scientific drug discovery. Front. Pharmacol. 8, 381. doi:10.3389/fphar.2017.00381
Hamet, P., and Tremblay, J. (2017). Artificial intelligence in medicine. Metabolism 69, S36-S40–S40. doi:10.1016/j.metabol.2017.01.011
Han, J.-Y., Li, Q., Ma, Z.-Z., and Fan, J.-Y. (2017). Effects and mechanisms of compound Chinese medicine and major ingredients on microcirculatory dysfunction and organ injury induced by ischemia/reperfusion. Pharmacol. Ther. 177, 146–173. doi:10.1016/j.pharmthera.2017.03.005
He, M., Yan, X., Zhou, J., and Xie, G. (2001). Traditional Chinese medicine database and application on the Web. J. Chem. Inf. Comput. Sci. 41, 273–277. doi:10.1021/ci0003101
He, Q., Huang, S., Wu, Y., Zhang, W., Wang, F., Cao, J., et al. (2018). Comparative study on the composition of free amino acids and derivatives in the two botanical origins of an edible Chinese herb "Xiebai", i.e., Allium chinense G. Don and Allium macrostemon Bunge species. i.e., Allium chinense G. Don Allium macrostemon Bunge species. Food Res. Int. 106, 446–457. doi:10.1016/j.foodres.2018.01.007
He, Y., Lu, A., Zha, Y., and Tsang, I. (2008). Differential effect on symptoms treated with traditional Chinese medicine and western combination therapy in RA patients. Complement. Ther. Med. 16, 206–211. doi:10.1016/j.ctim.2007.08.005
Heinrich, M., Jalil, B., Abdel-Tawab, M., Echeverria, J., Kulić, Ž., McGaw, L. J., et al. (2022). Best Practice in the chemical characterisation of extracts used in pharmacological and toxicological research-The ConPhyMP-Guidelines. Front. Pharmacol. 13, 953205. doi:10.3389/fphar.2022.953205
Hopkins, A. L. (2008). Network pharmacology: the next paradigm in drug discovery. Nat. Chem. Biol. 4, 682–690. doi:10.1038/nchembio.118
Hou, Y., and Jiang, J.-G. (2013). Origin and concept of medicine food homology and its application in modern functional foods. Food Funct. 4, 1727–1741. doi:10.1039/c3fo60295h
Hu, Y., Gupta-Ostermann, D., and Bajorath, J. (2014). Exploring compound promiscuity patterns and multi-target activity spaces. Comput. Struct. Biotechnol. J. 9, e201401003. doi:10.5936/csbj.201401003
Huabing data platform (2023). Available at: http://www.huabeing.com/pres/index (Accessed September 1, 2023).
Huang, J., Chen, T., Zhang, J., Ma, X., Wang, F., and Tang, X. (2023). Overlapping symptoms of functional gastrointestinal disorders: current challenges and the role of traditional Chinese medicine. Am. J. Chin. Med. 51, 833–857. doi:10.1142/S0192415X23500398
Huang, L., Xie, D., Yu, Y., Liu, H., Shi, Y., Shi, T., et al. (2018). TCMID 2.0: a comprehensive resource for TCM. Nucleic Acids Res. 46, D1117-D1120–D1120. doi:10.1093/nar/gkx1028
Huang, Z., Fan, X., Wang, Y., Liang, Q., Tong, X., Bai, Y., et al. (2017). A new method to evaluate the dose-effect relationship of a TCM formula Gegen Qinlian Decoction: "Focus" mode of integrated biomarkers. Acta Pharmacol. Sin. 38, 1141–1149. doi:10.1038/aps.2016.165
Jalencas, X., and Mestres, J. (2013). On the origins of drug polypharmacology. MedChemComm 4, 80–87. doi:10.1039/C2MD20242E
Jassal, B., Matthews, L., Viteri, G., Gong, C., Lorente, P., Fabregat, A., et al. (2019). The reactome pathway knowledgebase. Nucleic Acids Res. 48, D498-D503–D503. doi:10.1093/nar/gkz1031
Jendza, K., Kato, M., Salcius, M., Srinivas, H., De Erkenez, A., Nguyen, A., et al. (2019). A small-molecule inhibitor of C5 complement protein. Nat. Chem. Biol. 15, 666–668. doi:10.1038/s41589-019-0303-9
Kanawong, R., Obafemi-Ajayi, T., Ma, T., Xu, D., Li, S., and Duan, Y. (2012). Automated tongue feature extraction for ZHENG classification in traditional Chinese medicine. Evid. Based Complement. Altern. Med. 2012, 912852. doi:10.1155/2012/912852
Kanehisa, M., Furumichi, M., Tanabe, M., Sato, Y., and Morishima, K. (2017). KEGG: new perspectives on genomes, pathways, diseases and drugs. Nucleic Acids Res. 45, D353-D361–D361. doi:10.1093/nar/gkw1092
Kang, H., Tang, K., Liu, Q., Sun, Y., Huang, Q., Zhu, R., et al. (2013). HIM-herbal ingredients in-vivo metabolism database. J. Cheminformatics 5, 28. doi:10.1186/1758-2946-5-28
Karp, P. D., Ouzounis, C. A., Moore-Kochlacs, C., Goldovsky, L., Kaipa, P., Ahrén, D., et al. (2005). Expansion of the BioCyc collection of pathway/genome databases to 160 genomes. Nucleic Acids Res. 33, 6083–6089. doi:10.1093/nar/gki892
Keith, C. T., Borisy, A. A., and Stockwell, B. R. (2005). Multicomponent therapeutics for networked systems. Nat. Rev. Drug Discov. 4, 71–78. doi:10.1038/nrd1609
Khojah, A. A., Padilla-González, G. F., Bader, A., Simmonds, M. J. S., Munday, M., and Heinrich, M. (2021). Barbeya oleoides leaves extracts: in vitro carbohydrate digestive enzymes inhibition and phytochemical characterization. Molecules 26, 6229. doi:10.3390/molecules26206229
Koeberle, A., and Werz, O. (2014). Multi-target approach for natural products in inflammation. Drug Discov. Today 19, 1871–1882. doi:10.1016/j.drudis.2014.08.006
Köhler, S., Gargano, M., Matentzoglu, N., Carmody, L. C., Lewis-Smith, D., Vasilevsky, N. A., et al. (2021). The human phenotype ontology in 2021. Nucleic Acids Res. 49, D1207–D1217. doi:10.1093/nar/gkaa1043
Lachance, H., Wetzel, S., Kumar, K., and Waldmann, H. (2012). Charting, navigating, and populating natural product chemical space for drug discovery. J. Med. Chem. 55, 5989–6001. doi:10.1021/jm300288g
Létinier, L., Bezin, J., Jarne, A., and Pariente, A. (2023). Drug-drug interactions and the risk of emergency hospitalizations: a nationwide population-based study. Drug Saf. 46, 449–456. doi:10.1007/s40264-023-01283-7
Li, B., Ma, C., Zhao, X., Hu, Z., Du, T., Xu, X., et al. (2018a). YaTCM: yet another traditional Chinese medicine database for drug discovery. Comput. Struct. Biotechnol. J. 16, 600–610. doi:10.1016/j.csbj.2018.11.002
Li, S., and Zhang, B. (2013). Traditional Chinese medicine network pharmacology: theory, methodology and application. Chin. J. Nat. Med. 11, 110–120. doi:10.1016/S1875-5364(13)60037-0
Li, S., Zhang, B., Jiang, D., Wei, Y., and Zhang, N. (2010). Herb network construction and co-module analysis for uncovering the combination rule of traditional Chinese herbal formulae. BMC Bioinforma. 11, S6. doi:10.1186/1471-2105-11-S11-S6
Li, S., Zhang, Z. Q., Wu, L. J., Zhang, X. G., Wang, Y. Y., and Li, Y. D. (2007). Understanding ZHENG in traditional Chinese medicine in the context of neuro-endocrine-immune network. IET Syst. Biol. 1, 51–60. doi:10.1049/iet-syb:20060032
Li, W.-F., Jiang, J.-G., and Chen, J. (2008). Chinese medicine and its modernization demands. Arch. Med. Res. 39, 246–251. doi:10.1016/j.arcmed.2007.09.011
Li, X., Ren, J., Zhang, W., Zhang, Z., Yu, J., Wu, J., et al. (2022a). LTM-TCM: a comprehensive database for the linking of traditional Chinese medicine with modern medicine at molecular and phenotypic levels. Pharmacol. Res. 178, 106185. doi:10.1016/j.phrs.2022.106185
Li, Y., Lin, Z., Wang, Y., Ju, S., Wu, H., Jin, H., et al. (2022b). Unraveling the mystery of efficacy in Chinese medicine formula: new approaches and technologies for research on pharmacodynamic substances. Arab. J. Chem. 15, 104302. doi:10.1016/j.arabjc.2022.104302
Li, Y. H., Yu, C. Y., Li, X. X., Zhang, P., Tang, J., Yang, Q., et al. (2018b). Therapeutic target database update 2018: enriched resource for facilitating bench-to-clinic research of targeted therapeutics. Nucleic Acids Res. 46, D1121-D1127–D1127. doi:10.1093/nar/gkx1076
Liang, Q.-L., Liang, X.-P., Wang, Y.-M., Xie, Y.-Y., Zhang, R.-L., Chen, X., et al. (2012). Effective components screening and anti-myocardial infarction mechanism study of the Chinese medicine NSLF6 based on “system to system” mode. J. Transl. Med. 10, 26. doi:10.1186/1479-5876-10-26
Lipinski, C., and Hopkins, A. (2004). Navigating chemical space for biology and medicine. Nature 432, 855–861. doi:10.1038/nature03193
Lipinski, C. A. (2016). Rule of five in 2015 and beyond: target and ligand structural limitations, ligand chemistry structure and drug discovery project decisions. Adv. Drug Deliv. Rev. 101, 34–41. doi:10.1016/j.addr.2016.04.029
Liu, C., Guo, D., and Liu, L. (2018a). Quality transitivity and traceability system of herbal medicine products based on quality markers. Phytomedicine 44, 247–257. doi:10.1016/j.phymed.2018.03.006
Liu, Y.-Y., and Barabási, A.-L. (2016). Control principles of complex systems. Rev. Mod. Phys. 88, 035006. doi:10.1103/RevModPhys.88.035006
Liu, Z., Cai, C., Du, J., Liu, B., Cui, L., Fan, X., et al. (2020). TCMIO: a comprehensive database of traditional Chinese medicine on immuno-oncology. Front. Pharmacol. 11, 439. doi:10.3389/fphar.2020.00439
Liu, Z., Du, J., Yan, X., Zhong, J., Cui, L., Lin, J., et al. (2018b). TCMAnalyzer: a chemo-and bioinformatics web service for analyzing traditional Chinese medicine. J. Chem. Inf. Model. 58, 550–555. doi:10.1021/acs.jcim.7b00549
Liu, Z., Guo, F., Wang, Y., Li, C., Zhang, X., Li, H., et al. (2016). BATMAN-TCM: a bioinformatics analysis tool for molecular mechANism of traditional Chinese medicine. Sci. Rep. 6, 21146. doi:10.1038/srep21146
Long, Y., Li, D., Yu, S., Shi, A., Deng, J., Wen, J., et al. (2022). Medicine–food herb: Angelica sinensis, a potential therapeutic hope for alzheimer’s disease and related complications. Food Funct. 13, 8783–8803. doi:10.1039/D2FO01287A
Lu, Y., Lu, G., Li, J., Xu, Y., Zhang, Z., and Zhang, D. (2022). Multiscale conditional regularization for convolutional neural networks. IEEE Trans. Cybern. 52, 444–458. doi:10.1109/TCYB.2020.2979968
Luan, X., Zhang, L.-J., Li, X.-Q., Rahman, K., Zhang, H., Chen, H.-Z., et al. (2020). Compound-based Chinese medicine formula: from discovery to compatibility mechanism. J. Ethnopharmacol. 254, 112687. doi:10.1016/j.jep.2020.112687
Luca, S. V., Macovei, I., Bujor, A., Miron, A., Skalicka-Woźniak, K., Aprotosoaie, A. C., et al. (2020). Bioactivity of dietary polyphenols: the role of metabolites. Crit. Rev. Food Sci. Nutr. 60, 626–659. doi:10.1080/10408398.2018.1546669
Lv, Q., Chen, G., He, H., Yang, Z., Zhao, L., Zhang, K., et al. (2023). TCMBank-the largest TCM database provides deep learning-based Chinese-Western medicine exclusion prediction. Signal Transduct. Target. Ther. 8, 127. doi:10.1038/s41392-023-01339-1
Ma, Y., Sun, S., and Peng, C.-K. (2014). Applications of dynamical complexity theory in traditional Chinese medicine. Front. Med. 8, 279–284. doi:10.1007/s11684-014-0367-6
Mangal, M., Sagar, P., Singh, H., Raghava, G. P. S., and Agarwal, S. M. (2013). NPACT: naturally occurring plant-based anti-cancer compound-activity-target database. Nucleic Acids Res. 41, D1124–D1129. doi:10.1093/nar/gks1047
Manukyan, A., Lumlerdkij, N., and Heinrich, M. (2019). Caucasian endemic medicinal and nutraceutical plants: in-vitro antioxidant and cytotoxic activities and bioactive compounds. J. Pharm. Pharmacol. 71, 1152–1161. doi:10.1111/jphp.13093
Mendez, D., Gaulton, A., Bento, A. P., Chambers, J., De Veij, M., Félix, E., et al. (2019). ChEMBL: towards direct deposition of bioassay data. Nucleic Acids Res. 47, D930-D940–D940. doi:10.1093/nar/gky1075
Méndez-Lucio, O., Naveja, J. J., Vite-Caritino, H., Prieto-Martínez, F. D., and Medina-Franco, J. L. (2016). One drug for multiple targets: a computational perspective. J. Mex. Chem. Soc. 60, 168–181. doi:10.29356/jmcs.v60i3.100
Mistry, J., Chuguransky, S., Williams, L., Qureshi, M., Salazar, G. A., Sonnhammer, E. L. L., et al. (2021). Pfam: the protein families database in 2021. Nucleic Acids Res. 49, D412–D419. doi:10.1093/nar/gkaa913
Modern Application Database of Herbal Formulations (2017). Available at: http://cintmed.cintcm.com/cintmed/searchIndex/basic.html?dbtype=xdfj&pdh=15 (Accessed September 1, 2023).
Niu, Q., Li, H., Tong, L., Liu, S., Zong, W., Zhang, S., et al. (2023). TCMFP: a novel herbal formula prediction method based on network target’s score integrated with semi-supervised learning genetic algorithms. Brief. Bioinform. 24, bbad102. doi:10.1093/bib/bbad102
OTC Chinese Herbal Medicine Database (2017). Available at: https://cintmed.cintcm.com/cintmed/searchIndex/basic.html?dbtype=xdfj&pdh=21 (Accessed September 1, 2023).
Papatheocharidou, C., and Samanidou, V. (2023). Two-dimensional high-performance liquid chromatography as a powerful tool for bioanalysis: the paradigm of antibiotics. Molecules 28, 5056. doi:10.3390/molecules28135056
Paydas, S. (2019). Management of adverse effects/toxicity of ibrutinib. Crit. Rev. Oncol. Hematol. 136, 56–63. doi:10.1016/j.critrevonc.2019.02.001
Penrod, N. M., Cowper-Sal-lari, R., and Moore, J. H. (2011). Systems genetics for drug target discovery. Trends Pharmacol. Sci. 32, 623–630. doi:10.1016/j.tips.2011.07.002
Pfister, S. X., and Ashworth, A. (2017). Marked for death: targeting epigenetic changes in cancer. Nat. Rev. Drug Discov. 16, 241–263. doi:10.1038/nrd.2016.256
Pharnexcloud (2021). Available at: https://www.pharnexcloud.com/(Accessed September 1, 2023).
Piñero, J., Bravo, À., Queralt-Rosinach, N., Gutiérrez-Sacristán, A., Deu-Pons, J., Centeno, E., et al. (2017). DisGeNET: a comprehensive platform integrating information on human disease-associated genes and variants. Nucleic Acids Res. 45, D833-D839–D839. doi:10.1093/nar/gkw943
Plazas, E., Avila, M. C., Muñoz, D. R., and Cuca S, L. E. (2022). Natural isoquinoline alkaloids: pharmacological features and multi-target potential for complex diseases. Pharmacol. Res. 177, 106126. doi:10.1016/j.phrs.2022.106126
Ramsay, R. R., Popovic-Nikolic, M. R., Nikolic, K., Uliassi, E., and Bolognesi, M. L. (2018). A perspective on multi-target drug discovery and design for complex diseases. Clin. Transl. Med. 7, 3. doi:10.1186/s40169-017-0181-2
Rappaport, N., Twik, M., Plaschkes, I., Nudel, R., Iny Stein, T., Levitt, J., et al. (2017). MalaCards: an amalgamated human disease compendium with diverse clinical and genetic annotation and structured search. Nucleic Acids Res. 45, D877-D887–D887. doi:10.1093/nar/gkw1012
Rebhan, M., Chalifa-Caspi, V., Prilusky, J., and Lancet, D. (1997). GeneCards: integrating information about genes, proteins and diseases. Trends Genet. 13, 163. doi:10.1016/S0168-9525(97)01103-7
Reymond, J.-L. (2015). The chemical space project. Acc. Chem. Res. 48, 722–730. doi:10.1021/ar500432k
Ru, J., Li, P., Wang, J., Zhou, W., Li, B., Huang, C., et al. (2014). TCMSP: a database of systems pharmacology for drug discovery from herbal medicines. J. Cheminformatics 6, 13. doi:10.1186/1758-2946-6-13
Sadybekov, A. V., and Katritch, V. (2023). Computational approaches streamlining drug discovery. Nature 616, 673–685. doi:10.1038/s41586-023-05905-z
Safran, M., Dalah, I., Alexander, J., Rosen, N., Iny Stein, T., Shmoish, M., et al. (2010). GeneCards version 3: the human gene integrator. Database 2010, baq020. doi:10.1093/database/baq020
Saks, V., Monge, C., and Guzun, R. (2009). Philosophical basis and some historical aspects of systems biology: from hegel to noble - applications for bioenergetic research. Int. J. Mol. Sci. 10, 1161–1192. doi:10.3390/ijms10031161
Santos, R., Ursu, O., Gaulton, A., Bento, A. P., Donadi, R. S., Bologa, C. G., et al. (2017). A comprehensive map of molecular drug targets. Nat. Rev. Drug Discov. 16, 19–34. doi:10.1038/nrd.2016.230
Shang, H., Zhang, L., Xiao, T., Zhang, L., Ruan, J., Zhang, Q., et al. (2022). Study on the differences of gut microbiota composition between phlegm-dampness syndrome and qi-yin deficiency syndrome in patients with metabolic syndrome. Front. Endocrinol. 13, 1063579. doi:10.3389/fendo.2022.1063579
Sherman, B. T., Hao, M., Qiu, J., Jiao, X., Baseler, M. W., Lane, H. C., et al. (2022). DAVID: a web server for functional enrichment analysis and functional annotation of gene lists (2021 update). Nucleic Acids Res. 50, W216–W221. doi:10.1093/nar/gkac194
Singhal, K., Azizi, S., Tu, T., Mahdavi, S. S., Wei, J., Chung, H. W., et al. (2023a). Large language models encode clinical knowledge. Nature 620, 172–180. doi:10.1038/s41586-023-06291-2
Singhal, K., Tu, T., Gottweis, J., Sayres, R., Wulczyn, E., Hou, L., et al. (2023b). Towards expert-level medical question answering with large language models, 09617. doi:10.48550/arXiv.2305
Sorokina, M., and Steinbeck, C. (2020). Review on natural products databases: where to find data in 2020. J. Cheminformatics 12, 20. doi:10.1186/s13321-020-00424-9
Spencer, C. M., and Jarvis, B. (1999). Salmeterol/Fluticasone propionate combination. Drugs 57, 933–940. doi:10.2165/00003495-199957060-00010
Stitziel, N. O., and Kathiresan, S. (2017). Leveraging human genetics to guide drug target discovery. Trends cardiovasc. Med. 27, 352–359. doi:10.1016/j.tcm.2016.08.008
Stocker, S., Csányi, G., Reuter, K., and Margraf, J. T. (2020). Machine learning in chemical reaction space. Nat. Commun. 11, 5505. doi:10.1038/s41467-020-19267-x
Su, S.-B., Lu, A., Li, S., and Jia, W. (2012). Evidence-based ZHENG: a traditional Chinese medicine syndrome. Evid. Based Complement. Altern. Med. 2012, 246538. doi:10.1155/2012/246538
Sun, C., Huang, J., Tang, R., Li, M., Yuan, H., Wang, Y., et al. (2022). CPMCP: a database of Chinese patent medicine and compound prescription. Database J. Biol. Databases Curation 00, baac073–7. doi:10.1093/database/baac073
Szklarczyk, D., Gable, A. L., Nastou, K. C., Lyon, D., Kirsch, R., Pyysalo, S., et al. (2021). The STRING database in 2021: customizable protein–protein networks, and functional characterization of user-uploaded gene/measurement sets. Nucleic Acids Res. 49, D605–D612. doi:10.1093/nar/gkaa1074
Szklarczyk, D., Santos, A., von Mering, C., Jensen, L. J., Bork, P., and Kuhn, M. (2016). STITCH 5: augmenting protein–chemical interaction networks with tissue and affinity data. Nucleic Acids Res. 44, D380–D384. doi:10.1093/nar/gkv1277
Tan, A., Huang, H., Zhang, P., and Li, S. (2019). Network-based cancer precision medicine: a new emerging paradigm. Cancer Lett. 458, 39–45. doi:10.1016/j.canlet.2019.05.015
Tang, J.-L., Liu, B.-Y., and Ma, K.-W. (2008). Traditional Chinese medicine. Lancet 372, 1938–1940. doi:10.1016/S0140-6736(08)61354-9
Tang, Y., Li, Z., Yang, D., Fang, Y., Gao, S., Liang, S., et al. (2021). Research of insomnia on traditional Chinese medicine diagnosis and treatment based on machine learning. Chin. Med. 16, 2. doi:10.1186/s13020-020-00409-8
Tao, W., Li, B., Gao, S., Bai, Y., Shar, P. A., Zhang, W., et al. (2015). CancerHSP: anticancer herbs database of systems pharmacology. Sci. Rep. 5, 11481. doi:10.1038/srep11481
TCM Ancient Formulas Database (2023). Available at: http://zyygx.cintcm.ac.cn:9698/zyygx/(Accessed August 30, 2023).
The Chinese traditional medicine database (2017). Available at: https://cintmed.cintcm.com/cintmed/searchIndex/basic.html?dbtype=xdfj&pdh=5 (Accessed September 1, 2023).
The UniProt Consortium (2023). UniProt: the universal protein knowledgebase in 2023. Nucleic Acids Res. 51, D523–D531. doi:10.1093/nar/gkac1052
TM-MC 2.0 (2023). Available at: https://tm-mc.kr/index.jsp (Accessed September 1, 2023).
Turkarslan, S., Wurtmann, E. J., Wu, W.-J., Jiang, N., Bare, J. C., Foley, K., et al. (2014). Network portal: a database for storage, analysis and visualization of biological networks. Nucleic Acids Res. 42, D184–D190. doi:10.1093/nar/gkt1190
Tyler, A. L., Crawford, D. C., and Pendergrass, S. A. (2016). The detection and characterization of pleiotropy: discovery, progress, and promise. Brief. Bioinform. 17, 13–22. doi:10.1093/bib/bbv050
von Mering, C., Huynen, M., Jaeggi, D., Schmidt, S., Bork, P., and Snel, B. (2003). STRING: a database of predicted functional associations between proteins. Nucleic Acids Res. 31, 258–261. doi:10.1093/nar/gkg034
Wang, J., Duan, L., Li, H., Liu, J., and Chen, H. (2022a). Construction of an artificial intelligence traditional Chinese medicine diagnosis and treatment model based on syndrome elements and small-sample data. Engineering 8, 29–32. doi:10.1016/j.eng.2021.06.014
Wang, J., Li, Y., Yang, Y., Chen, X., Du, J., Zheng, Q., et al. (2017). A new strategy for deleting animal drugs from Traditional Chinese medicines based on modified yimusake formula. Sci. Rep. 7, 1504. doi:10.1038/s41598-017-01613-7
Wang, N., Xu, P., Wang, X., Yao, W., Yu, Z., Wu, R., et al. (2019a). Integrated pathological cell fishing and network pharmacology approach to investigate main active components of Er-Xian decotion for treating osteoporosis. J. Ethnopharmacol. 241, 111977. doi:10.1016/j.jep.2019.111977
Wang, P., Wang, S., Chen, H., Deng, X., Zhang, L., Xu, H., et al. (2021a). TCMIP v2.0 powers the identification of chemical constituents available in xinglou chengqi decoction and the exploration of pharmacological mechanisms acting on stroke complicated with tanre fushi syndrome. Front. Pharmacol. 12, 598200. doi:10.3389/fphar.2021.598200
Wang, T., Streeter, H., Wang, X., Purnama, U., Lyu, M., Carr, C., et al. (2019b). A network pharmacology study of the multi-targeting profile of an antiarrhythmic Chinese medicine xin su ning. Front. Pharmacol. 10, 1138. doi:10.3389/fphar.2019.01138
Wang, W., and Zhang, T. (2017). Integration of traditional Chinese medicine and Western medicine in the era of precision medicine. J. Integr. Med. 15, 1–7. doi:10.1016/S2095-4964(17)60314-5
Wang, X., Wang, Z.-Y., Zheng, J.-H., and Li, S. (2021b). TCM network pharmacology: a new trend towards combining computational, experimental and clinical approaches. Chin. J. Nat. Med. 19, 1–11. doi:10.1016/S1875-5364(21)60001-8
Wang, Y., Shi, X., Li, L., Efferth, T., and Shang, D. (2021c). The impact of artificial intelligence on traditional Chinese medicine. Am. J. Chin. Med. 49, 1297–1314. doi:10.1142/S0192415X21500622
Wang, Y., Yang, H., Chen, L., Jafari, M., and Tang, J. (2021d). Network-based modeling of herb combinations in traditional Chinese medicine. Brief. Bioinform. 00, bbab106–14. doi:10.1093/bib/bbab106
Wang, Y., Yuan, Y., Wang, W., He, Y., Zhong, H., Zhou, X., et al. (2022b). Mechanisms underlying the therapeutic effects of qingfeiyin in treating acute lung injury based on GEO datasets, network pharmacology and molecular docking. Comput. Biol. Med. 145, 105454. doi:10.1016/j.compbiomed.2022.105454
Wu, G., Zhao, J., Zhao, J., Song, N., Zheng, N., Zeng, Y., et al. (2021). Exploring biological basis of syndrome differentiation in coronary heart disease patients with two distinct syndromes by integrated multi-omics and network pharmacology strategy. Chin. Med. 16, 109. doi:10.1186/s13020-021-00521-3
Wu, Y., Zhang, F., Yang, K., Fang, S., Bu, D., Li, H., et al. (2019). SymMap: an integrative database of traditional Chinese medicine enhanced by symptom mapping. Nucleic Acids Res. 47, D1110-D1117–D1117. doi:10.1093/nar/gky1021
Xiong, H., Wang, S., Zhu, Y., Zhao, Z., Liu, Y., Huang, L., et al. (2023). DoctorGLM: fine-tuning your Chinese doctor is not a herculean task. doi:10.48550/arXiv.2304.01097
Xiong, W., Devkota, L., Zhang, B., Muir, J., and Dhital, S. (2022). Intact cells: “nutritional capsules” in plant foods. Compr. Rev. Food Sci. Food Saf. 21, 1198–1217. doi:10.1111/1541-4337.12904
Xu, H., Zhang, Y., Wang, P., Zhang, J., Chen, H., Zhang, L., et al. (2021). A comprehensive review of integrative pharmacology-based investigation: a paradigm shift in traditional Chinese medicine. Acta Pharm. Sin. B 11, 1379–1399. doi:10.1016/j.apsb.2021.03.024
Xu, H.-Y., Zhang, Y.-Q., Liu, Z.-M., Chen, T., Lv, C.-Y., Tang, S.-H., et al. (2019). ETCM: an encyclopaedia of traditional Chinese medicine. Nucleic Acids Res. 47, D976-D982–D982. doi:10.1093/nar/gky987
Xu, X., Gao, Z., Yang, F., Yang, Y., Chen, L., Han, L., et al. (2020). Antidiabetic effects of gegen qinlian decoction via the gut microbiota are attributable to its key ingredient berberine. Genomics Proteomics Bioinforma. 18, 721–736. doi:10.1016/j.gpb.2019.09.007
Xue, R., Fang, Z., Zhang, M., Yi, Z., Wen, C., and Shi, T. (2012). TCMID: traditional Chinese medicine integrative database for herb molecular mechanism analysis. Nucleic Acids Res. 41, D1089–D1095. doi:10.1093/nar/gks1100
Yan, D., Zheng, G., Wang, C., Chen, Z., Mao, T., Gao, J., et al. (2022). HIT 2.0: an enhanced platform for herbal ingredients’ targets. Nucleic Acids Res. 50, D1238–D1243. doi:10.1093/nar/gkab1011
Yang, J., Tian, S., Zhao, J., and Zhang, W. (2020). Exploring the mechanism of TCM formulae in the treatment of different types of coronary heart disease by network pharmacology and machining learning. Pharmacol. Res. 159, 105034. doi:10.1016/j.phrs.2020.105034
Yang, P., Lang, J., Li, H., Lu, J., Lin, H., Tian, G., et al. (2022). TCM-Suite: a comprehensive and holistic platform for traditional Chinese medicine component identification and network pharmacology analysis. iMeta 1, e47. doi:10.1002/imt2.47
Yao, Y., Zhang, X., Wang, Z., Zheng, C., Li, P., Huang, C., et al. (2013). Deciphering the combination principles of traditional Chinese medicine from a systems pharmacology perspective based on ma-huang decoction. J. Ethnopharmacol. 150, 619–638. doi:10.1016/j.jep.2013.09.018
Ye, Y.-N., Liang, D.-F., Yi, J.-H., Jin, S., and Zeng, Z. (2023). IGTCM: an integrative genome database of traditional Chinese medicine plants. Plant Genome 16, e20317. doi:10.1002/tpg2.20317
Yu, H., Chen, J., Xu, X., Li, Y., Zhao, H., Fang, Y., et al. (2012). A systematic prediction of multiple drug-target interactions from chemical, genomic, and pharmacological data. PLoS ONE 7, e37608. doi:10.1371/journal.pone.0037608
Zeeshan, S., Xiong, R., Liang, B. T., and Ahmed, Z. (2020). 100 years of evolving gene–disease complexities and scientific debutants. Brief. Bioinform. 21, 885–905. doi:10.1093/bib/bbz038
Zeng, A., Liu, X., Du, Z., Wang, Z., Lai, H., Ding, M., et al. (2022a). GLM-130B: an open bilingual pre-trained model. doi:10.48550/arXiv.2210.02414
Zeng, B., Wei, A., Zhou, Q., Yuan, M., Lei, K., Liu, Y., et al. (2022b). Andrographolide: a review of its pharmacology, pharmacokinetics, toxicity and clinical trials and pharmaceutical research. Phytother. Res. 36, 336–364. doi:10.1002/ptr.7324
Zha, L.-H., He, L.-S., Lian, F.-M., Zhen, Z., Ji, H.-Y., Xu, L.-P., et al. (2015). Clinical strategy for optimal traditional Chinese medicine (TCM) herbal dose selection in disease therapeutics: expert consensus on classic TCM herbal formula dose conversion. Am. J. Chin. Med. 43, 1515–1524. doi:10.1142/S0192415X1550086X
Zhang, A., Sun, H., and Wang, X. (2014). Potentiating therapeutic effects by enhancing synergism based on active constituents from traditional medicine: potentiating therapeutic effects by enhancing synergism. Phytother. Res. 28, 526–533. doi:10.1002/ptr.5032
Zhang, L.-X., Dong, J., Wei, H., Shi, S.-H., Lu, A.-P., Deng, G.-M., et al. (2022a). TCMSID: a simplified integrated database for drug discovery from traditional Chinese medicine. J. Cheminformatics 14, 89. doi:10.1186/s13321-022-00670-z
Zhang, M., Vrolijk, M., and Haenen, G. (2017a). The screening of anticholinergic accumulation by traditional Chinese medicine. Int. J. Mol. Sci. 19, 18. doi:10.3390/ijms19010018
Zhang, R., Zhu, X., Bai, H., and Ning, K. (2019a). Network pharmacology databases for traditional Chinese medicine: review and assessment. Front. Pharmacol. 10, 123. doi:10.3389/fphar.2019.00123
Zhang, R.-Z., Yu, S.-J., Bai, H., and Ning, K. (2017b). TCM-Mesh: the database and analytical system for network pharmacology analysis for TCM preparations. Sci. Rep. 7, 2821. doi:10.1038/s41598-017-03039-7
Zhang, W., Huai, Y., Miao, Z., Qian, A., and Wang, Y. (2019b). Systems pharmacology for investigation of the mechanisms of action of traditional Chinese medicine in drug discovery. Front. Pharmacol. 10, 743. doi:10.3389/fphar.2019.00743
Zhang, Y., Li, X., Shi, Y., Chen, T., Xu, Z., Wang, P., et al. (2023). ETCM v2.0: an update with comprehensive resource and rich annotations for traditional Chinese medicine. Acta Pharm. Sin. B 13, 2559–2571. doi:10.1016/j.apsb.2023.03.012
Zhang, Y., Wang, N., Du, X., Chen, T., Yu, Z., Qin, Y., et al. (2022b). SoFDA: an integrated web platform from syndrome ontology to network-based evaluation of disease–syndrome–formula associations for precision medicine. Sci. Bull. 67, 1097–1101. doi:10.1016/j.scib.2022.03.013
Zhao, C., Li, G.-Z., Wang, C., and Niu, J. (2015). Advances in patient classification for traditional Chinese medicine: a machine learning perspective. Evid. Based Complement. Altern. Med. 2015, 376716. doi:10.1155/2015/376716
Zhao, H., Yang, Y., Wang, S., Yang, X., Zhou, K., Xu, C., et al. (2023a). NPASS database update 2023: quantitative natural product activity and species source database for biomedical research. Nucleic Acids Res. 51, D621–D628. doi:10.1093/nar/gkac1069
Zhao, J., Jiang, P., and Zhang, W. (2010). Molecular networks for the study of TCM pharmacology. Brief. Bioinform. 11, 417–430. doi:10.1093/bib/bbp063
Zhao, J., Lv, C., Wu, Q., Zeng, H., Guo, X., Yang, J., et al. (2019). Computational systems pharmacology reveals an antiplatelet and neuroprotective mechanism of deng-zhan-xi-xin injection in the treatment of ischemic stroke. Pharmacol. Res. 147, 104365. doi:10.1016/j.phrs.2019.104365
Zhao, L., Zhang, H., Li, N., Chen, J., Xu, H., Wang, Y., et al. (2023b). Network pharmacology, a promising approach to reveal the pharmacology mechanism of Chinese medicine formula. J. Ethnopharmacol. 309, 116306. doi:10.1016/j.jep.2023.116306
Zhou, W., Yang, K., Zeng, J., Lai, X., Wang, X., Ji, C., et al. (2021). FordNet: recommending traditional Chinese medicine formula via deep neural network integrating phenotype and molecule. Pharmacol. Res. 173, 105752. doi:10.1016/j.phrs.2021.105752
Zhou, X., Li, Y., Peng, Y., Hu, J., Zhang, R., He, L., et al. (2014). Clinical phenotype network: the underlying mechanism for personalized diagnosis and treatment of traditional Chinese medicine. Front. Med. 8, 337–346. doi:10.1007/s11684-014-0349-8
Keywords: TCM databases, systems pharmacology, formula-ZHENG relationship, complex biological system, network analysis
Citation: Fan M, Jin C, Li D, Deng Y, Yao L, Chen Y, Ma Y-L and Wang T (2023) Multi-level advances in databases related to systems pharmacology in traditional Chinese medicine: a 60-year review. Front. Pharmacol. 14:1289901. doi: 10.3389/fphar.2023.1289901
Received: 06 September 2023; Accepted: 03 November 2023;
Published: 14 November 2023.
Edited by:
Rajeev K. Singla, Sichuan University, ChinaReviewed by:
Smith B. Babiaka, University of Tuebingen, GermanyManoj Kumar Sabnani, University of Texas at Arlington, United States
Rekha Khandia, Barkatullah University, India
Copyright © 2023 Fan, Jin, Li, Deng, Yao, Chen, Ma and Wang. This is an open-access article distributed under the terms of the Creative Commons Attribution License (CC BY). The use, distribution or reproduction in other forums is permitted, provided the original author(s) and the copyright owner(s) are credited and that the original publication in this journal is cited, in accordance with accepted academic practice. No use, distribution or reproduction is permitted which does not comply with these terms.
*Correspondence: Taiyi Wang, d2FuZ3RhaXlpQHNkdXRjbS5lZHUuY24=; Yu-Ling Ma, bWF5dWxpbmc5M0BnbWFpbC5jb20mI3gwMjAwYTs=