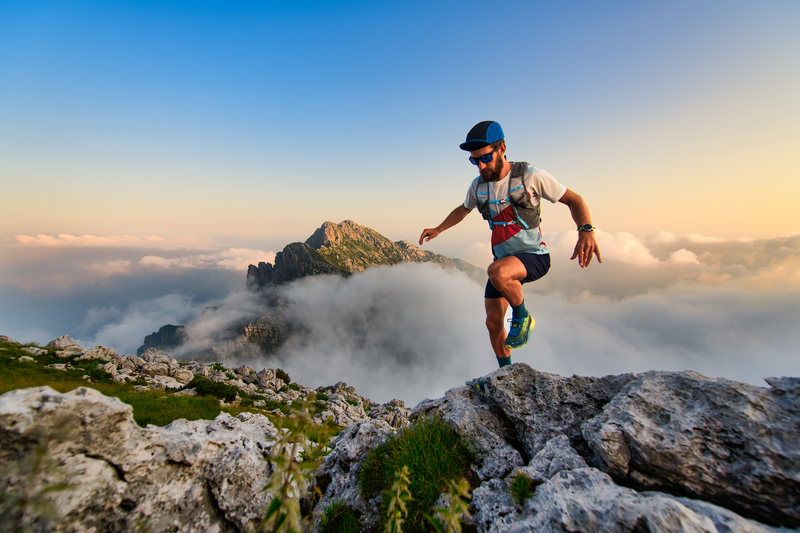
94% of researchers rate our articles as excellent or good
Learn more about the work of our research integrity team to safeguard the quality of each article we publish.
Find out more
REVIEW article
Front. Pharmacol. , 09 January 2024
Sec. Pharmacogenetics and Pharmacogenomics
Volume 14 - 2023 | https://doi.org/10.3389/fphar.2023.1260276
This article is part of the Research Topic Exploring the Impact of Genetics on New Drugs and Potential Drug Targets: A Multi-Omics Approach to Improve Personalized Therapeutics. View all 10 articles
Over the past two decades, Next-Generation Sequencing (NGS) has revolutionized the approach to cancer research. Applications of NGS include the identification of tumor specific alterations that can influence tumor pathobiology and also impact diagnosis, prognosis and therapeutic options. Pharmacogenomics (PGx) studies the role of inheritance of individual genetic patterns in drug response and has taken advantage of NGS technology as it provides access to high-throughput data that can, however, be difficult to manage. Machine learning (ML) has recently been used in the life sciences to discover hidden patterns from complex NGS data and to solve various PGx problems. In this review, we provide a comprehensive overview of the NGS approaches that can be employed and the different PGx studies implicating the use of NGS data. We also provide an excursus of the ML algorithms that can exert a role as fundamental strategies in the PGx field to improve personalized medicine in cancer.
Pharmacogenetics is a branch of molecular biology and pharmacology that studies the relationships between the genetic background of individuals and the effects of a particular treatment (Nebert, 1999). In recent years, thanks to rapid access to high-throughput sequencing technologies, commonly referred to as Next-Generation Sequencing (NGS), pharmacogenetic studies have seen an upsurge in the identification of variants associated with differential patient response to drugs, leading to an evolution from pharmacogenetics to pharmacogenomics (Auwerx et al., 2022). Although these terms have subtle differences, they are generally used as synonyms and will be referred to as PGx in the following.
In many clinical trials, the primary endpoint is not met because of inadequate patient cohort selection, stratification criteria, or genotype and phenotype characterization, which in turn can introduce confounding factors and reduce the statistical power of the study (Fogel, 2018). Despite demonstrated benefit for a few patients, failure to meet the primary endpoint may reduce success rates of anticancer drugs entering clinical practice, with only about 5% of drugs approved by the Food and Drugs Administration (FDA) (Harrison, 2016). These issues are critical in cancer therapy because drugs can be ineffective for a variety of reasons, including altered expression of target genes by cancer cells and development of resistance to treatment, as well as inappropriate selection for clinical study design. As a result, many patients with advanced disease may lose access to potentially effective treatments. For these reasons, identifying the genetic factors responsible for drug response and resistance in cancer is mandatory for better patient management and treatment.
The Human Genome Project is considered a milestone in the context of sequencing experiments, and has contributed to an upsurge in both the genetic characterization of tumors and the development of sequencing technologies where NGS has become mainstream. The application of NGS technology has enabled the detection of multiple genetic mutations or altered gene expression in many samples and in a few runs, providing a large amount of data in a short turnaround time and at a competitive cost (Hussen et al., 2022). In addition, NGS allows researchers to identify somatic and germline variants within the same experiment, both of which are important in the context of cancer, drug response, and drug toxicity. Somatic variants are mutations that arise de novo in tissue-specific cells due to environmental stress and errors in DNA replication, and are divided into driver and passenger mutations. Driver mutations have the effect of conferring proliferative advantages, whereas passenger mutations occur in cells that already carry a driver mutation and are a consequence of genomic instability (Bozic et al., 2010). In contrast, germline mutations are inherited changes that affect reproductive cells and are present in all somatic cells; they may be common or rare in a given population. Of note, not only are somatic variants important for cancer treatment and mainly used as molecular targets, but germline mutations may also contribute, at least in part, to tumor development, progression, and resistance (Chen X et al., 2023; Wang et al., 2023).
Genetic variations in genes related to pharmacokinetic processes (PK, i.e., absorption, distribution, metabolism and excretion), or in genes related to pharmacodynamics (PD, mechanisms of action and post-target signaling), can lead to drug inefficacy or toxicity, making treatment unavailable to patients. The most commonly inherited genetic variants include single nucleotide polymorphisms (SNPs), insertions/deletions (INDELs), copy number variations (CNVs) and a variable number of tandem repeats (Ismail and Essawi, 2012; Yu et al., 2021). The frequency of these variants also plays a role in adverse drug reactions and inefficacy, as not only common variants, but also low-frequency and rare variants should be considered for drug-specific functional alterations (Lauschke et al., 2018). Processing the high-throughput data obtained by NGS in PGx approaches is challenging. To cope with this huge amount of omics data, numerous bioinformatics pipelines have been developed.
Machine learning (ML) is a branch of artificial intelligence based on statistical learning that is able to predict a response or recognize relationships between complex data structures. Thanks to its flexibility, ML is also used in medical and biological sciences (Handelman et al., 2018). So far, many efforts have been made to improve ML algorithms for cancer diagnosis, prognosis and treatment.
Existing reviews mainly address the use of omics data in cancer and pharmacogenomics, but to our knowledge very few of them focus on the use of machine learning in cancer pharmacogenomics. For these reasons, although the topics covered here are quite extensive and are not addressed in detail, this review aims to highlight emerging research trends and underlying critical issues that may be encountered by new researchers approaching ML and pharmacogenomics in cancer. In this review, we provide a comprehensive overview of NGS applications and PGx studies in personalized medicine. The first chapters are dedicated to sequencing applications (e.g., genome, exome, transcriptome), with a focus on targeted and whole sequencing approaches. We also provide an overview of omics data generated by NGS in cancer research and its application to PGx studies, focusing on targeted therapy, efficacy, and toxicity. We next analyze the types of ML algorithms and their application in cancer research. Finally, we discuss about the challenges faced by the ML approach in PGx studies and make suggestions for further improvements.
It is widely acknowledged that NGS has been groundbreaking in cancer research. Over the past two decades, many NGS technologies have been developed to meet multiple needs and have become even more sophisticated. Figure 1 provides an overview of NGS technologies and strategies for investigating the molecular background of cancer.
FIGURE 1. Omic data. Overview of NGS technology and applications in cancer research. Abbreviation: Ac, acetylation; ADP-ribo, ADP ribosylation; CNV, copy number variation; Glyco, glycosylation; Lipid, lipidation; lncRNA, long non-coding RNA; Me, methylation; miRNA, microRNA; ncRNA, non-coding RNA; OH, oxidrylic group; P, phosphorylation; SNV, single nucleotide variants; S-S, disulphide; Sumo, Sumoylation; TFs, transcription factors; Ub, ubiquitination.
From a theoretical standpoint, characterization of the entire genetic background of the tumor should be considered the most comprehensive strategy to gain insight into tumor biology. Whole genome sequencing (WGS) and whole exome sequencing (WES) are NGS approaches in which virtually the entire genome (WGS) or the protein-coding regions (exons) of the genome (WES) are sequenced. Both approaches can be used in cancer research to sequence normal tissue (e.g., blood) and tumor tissue to discover new targets for therapies and biomarkers of cancer stage, predisposition, and response to therapy. In addition, sequencing of paired tumor and normal tissues allows unambiguous identification of individual germline and somatic variants as well as loss of heterozygosity and the “second hit” mutations (Mandelker and Ceyhan-Birsoy, 2020).
In the clinical setting, somatic and germline variants can be identified using both WGS and WES approaches but some important aspects should be highlighted. There are two main advantages of WGS: first, the discovery of novel genomic variants, including single nucleotide variants (SNVs) and structural variants (SVs) such as CNVs, INDELs, variable stretches in tandem repeats and balanced chromosomal translocations; second, the sequencing result includes coding, non-coding and mitochondrial DNA (Sims et al., 2014). On the other hand, WES highlights coding variants that are easier to study and whose phenotypic effects are more functional to assess.
Although it could be considered an advantage to sequence the whole genome at once, since different types of variants can be found with the same sequencing library, some limitations should be considered. First, we need to distinguish between two key parameters in sequencing, coverage depth and coverage itself. Coverage depth is a measure of how often a particular base in a sequence is seen during sequencing and can be an indicator of the reliability of the results, while coverage is the percentage of the genome that is sequenced during the experiment. These parameters are closely related and must be weighed when researchers define the goal of their studies (Sims et al., 2014; Meienberg et al., 2016). Although the coverage of WGS is higher than that of WES, the average depth of coverage in WGS experiments may be low. In contrast, WES has a higher average depth of coverage compared to WGS because WES only covers the exons that account for about 2% of the genome. Another limitation is related to the data generated by sequencing, as WGS data are very huge, and processing and storing such amount of data requires adequate computational resources, which may be a limit in some contexts. Finally, the cost of WGS experiments is usually higher than WES, especially in clinical settings.
For these reasons, WES has long been considered the gold standard for detecting genetic variants. However, comparative WGS and WES studies have recently shown that WGS is more powerful than WES in exome variant detection, providing broader coverage and better variant detection, and costs are now decreasing (Belkadi et al., 2015). In addition, the latest WGS library preparation methods are PCR-free, while the WES library preparation methods still rely on PCR amplification. This could lead to GC content bias and misidentification of variants (Meienberg et al., 2016). In addition, WES does not really cover the whole exome, so some deleterious coding SNVs might be missed. As mentioned earlier, WES is not validated for the detection of structural variants, including CNVs and translocations, and finally, by definition, it does not include non-coding intron regions. Therefore, WGS has become more attractive than WES for diagnostic purposes in recent years (Belkadi et al., 2015; Lionel et al., 2018; Hou et al., 2022).
Whole transcriptome sequencing (WTS) is an RNA-based sequencing strategy that captures the transcriptome repertoire, and its applications include quantification of gene expression, detection of alternative transcripts resulting from splice variants, detection of chromosomal rearrangements leading to chimeric gene fusions, and identification of the ever-growing family of non-coding RNAs (Lakhotia et al., 2020; Li and Wang, 2021). Depending on the research interest, total RNA extracted from samples should be treated to remove unwanted RNA species, which may be a limitation, especially in terms of time. On the other hand, the loss of valuable reads and the management of background noise are problems faced when no depletion is performed. Bulked WTS has been used in cancer research to identify pathways and genes involved in cancer development and, thanks to spatial transcriptomics and single-cell sequencing, also to understand tumor organization and interactions with the microenvironment (Chen TY et al., 2023).
Many companies that have developed high-throughput technologies have now launched numerous tumor-specific panels to study cancer. Targeted panels sequence only a small part of the genome because they are designed with probes targeting specifically regions of interest, such as sets of genes, in a specific/custom fashion. Depending on the size of the panel, they can achieve the depth of coverage required to highlight specific pathogenic variants (Lenahan et al., 2023). Targeted panels offer many advantages over WGS and WES approaches, including reduced hands-on time, ease of translation of raw data, profiling of specific tumor-associated genes and customization of the panel. These advantages can support the therapeutic decision-making process while reducing the time required (Bewicke-Copley et al., 2019).
For their part, WGS and WES approaches can be extremely useful in exploratory research and clinical trials, as they do not require “a priori” knowledge of disease mechanisms and can reveal novel molecular biomarkers. In this sense, the COGNITION study has shown that comprehensive molecular profiling using WGS and WES identifies a genomic signature in a subset of breast cancer patients at high risk of recurrence after neoadjuvant treatment, for whom targeted therapy solutions may be available (Pixberg et al., 2022).
On the other hand, targeted panels can also be employed in clinical trial design. In this case, panels could be used for many goals. First, to stratify the cohort according to known biomarkers, as in the case of the REGISTRI phase II clinical trial, in which a customized DNA panel was developed to specifically identify KIT/PDGFRA wildtype GIST patients eligible for regorafenib therapy (Martin-Broto et al., 2023); second, to support the discovery of new specific positive biomarkers, associated with response to therapy, as in the RELAY phase III trial, in which a targeted approach was used to assess ctDNA mutations and EGFR mutation dynamics after erlotinib with or without ramucirumab treatment in NSCLC patients (Garon et al., 2023); and finally, to identify actionable tumor alterations and candidate genes for molecular targeted therapies, as demonstrated in the MATCH study (Parsons et al., 2022). Of note, many targeted panels, known as PanCancer panels, are designed to cover many cancer-related genes, so the applications of these panels are widespread for many different goals.
Whole and targeted sequencing approaches can also be combined in clinical trials, as in the EVOLVE phase II study in which WES of tumor tissue and a targeted panel of cell-free DNA from blood were matched to discover novel genomic alterations responsible for resistance to PARP inhibitors in high-grade serous ovarian cancer (Lheureux et al., 2023).
In the clinical setting, things are different, as the cost of analysis is one of the limiting factors for sequencing. In this scenario, targeted panels are preferred because they have a lower cost per sample and an easier data management (Bewicke-Copley et al., 2019). Targeted panels are often designed to provide information on known biomarkers such as genomic instability score (GIS), loss of heterozygosity (LOH), microsatellite instability (MSI), and tumor mutation burden (TMB). The latter biomarker is of great interest in clinical practice, as pembrolizumab is the first FDA-approved agnostic cancer therapy that can be used in tumors with high TMB (Marcus et al., 2021); however, the sequencing method used to assess TMB may impact clinical outcomes, by excluding patients who might otherwise benefit from this treatment. Indeed, WGS, WES, and targeted-based panels have been used to measure TMB in cancer patients, with not only primary tumors but also circulating DNA in blood proposed as an alternative source material. In this context, WES of tumor and paired normal tissues is currently considered the most accurate approach to determine TMB, although this approach is both costly and time-consuming in the clinical setting (McGrail et al., 2021). On the other hand, an approach that uses targeted sequencing assays enriched in genes known to be involved in cancer appears to be more feasible in the clinic, particularly because these assays do not require paired tumor and normal samples to determine TMB. However, the type of cancer tested and the type of panel used to assess TMB can significantly affect the outcome (Merino et al., 2020). Among the many examples of targeted panels used in the clinical setting there are MyeloSeq, a 40-gene targeted panel used to determine variant and allele frequencies in patients with suspected hematologic malignancies (Barnell et al., 2021), the Oncomine Precision Assay, which tests 45 cancer-related genes (such as EGFR, KRAS, ALK, RET, BRAF, and others) used in screening for genomic alterations that can be treated with targeted therapy in NSCLC, colorectal cancer, melanoma, breast cancer, and other malignancies (Werner et al., 2022; Nindra et al., 2023), and other targeted panels such as the TruSight Oncology, the AmpliSeq, the FusionPlex, the QIASeq Multimodal Lung, which have demonstrated expertise in identifying genetic variants, and the NTRK gene fusion panel used to identify tumors sensitive to larotrectinib, an NTRK inhibitor (Drilon et al., 2018; Stockley et al., 2023).
In addition, there is also an ethical aspect to consider, as sequencing a larger portion of the genome may lead to the identification of unsolicited findings that should be better communicated to physicians and patients (Schoot et al., 2021). Targeted panels therefore have a lower chance of discovering new unsolicited findings, which facilitates clinical reports.
In summary, although WGS and WES sequencing are accurate and do not require “a priori” knowledge of disease mechanisms, their introduction into clinical practice may not be feasible, mainly because of coping with the volume of data and the cost per sample. On the other hand, targeted sequencing may facilitate the introduction of bulk sequencing into routine clinical practice where testing of multiple molecular biomarkers has become common practice, as has been the case with TMB and NTRK gene fusions. Again, the best choice between the two strategies must be balanced between cost and application.
Epigenomic sequencing has proliferated in recent years. Epigenetics is a branch of biology that studies the causal interactions between genes and their products. Basically, epigenetics studies all changes and phenotypes in gene expression that cannot be attributed to genetic causes. The most important changes can occur directly at the DNA, e.g., cytosine methylation, or at the chromatin proteins, e.g., acetylation, methylation, phosphorylation, and others (Kouzarides, 2007). The consequence of these changes is the modulation of the accessibility of the DNA sequence to enzymatic complexes, which determines the state of gene activation.
Sequencing of epigenetic modifications (epigenomics) identifies specific cancer signatures involved in tumorigenesis as well as cancer metastasis and recurrence (Huang et al., 2018; Malta et al., 2018; Sengupta et al., 2021). In particular, some histone modifications, such as reduced lysine acetylation and methylation, may act as prognostic biomarkers in breast cancer (Elsheikh et al., 2009; Zhou et al., 2022) or they may predict response to treatment, as in the case of immunotherapy (Peng et al., 2015; Hoffmann et al., 2023). In addition, dysregulation of genes involved in chromatin remodeling can also be a hallmark of a particular tumor. This is the case with mutations of histone deacetylase (HDAC) in multiple myeloma and lymphoma. HDAC inhibitors and DNA methylation inhibitors are anticancer drugs developed to target the aberrant activity of these molecules (Blumenschein et al., 2008; Galanis et al., 2009).
Proteomics and metabolomics are high-throughput screening of protein expression and metabolite abundance, respectively. Proteomics data can be considered a readout of the transcriptome, but it has been reported that only 40% of protein expression can be explained by a corresponding gene expression profile (Ideker et al., 2001; Vogel et al., 2010). Proteomic studies take pictures of the cellular protein repertoire that include protein abundance and turnover, post-translational modifications, subcellular localization, interactions with other proteins and structures, and finally protein involvement in metabolic pathways (Altelaar et al., 2013).
On the other hand, metabolomics studies investigate the presence of metabolites, their concentration and their interactions with biological systems. Unlike other “omics” approaches, metabolomics can reflect the actual biochemical activity and the state of cells and determine the true cellular phenotype (Patti et al., 2012; Tan et al., 2012).
In cancer research, proteomics and metabolomics strategies have been used not only to identify novel biomarkers involved in tumor resistance and signature that predicts treatment outcome (Dytfeld et al., 2016; Shrestha et al., 2021; Robles et al., 2022), but also to uncover cancer metabolic pathways and oncometabolites that may drive tumorigenesis and sustain tumor progression (Xu et al., 2011; Drusian et al., 2018).
Over the past 20 years, molecular assessment of tumors has entered routine clinical practice and has been incorporated into the WHO classification criteria for tumor diagnosis, grading and prognosis (Organisation mondiale de la santé and Centre international de recherche sur le cancer, 2020; Sbaraglia et al., 2020; Louis et al., 2021).
As a result, the treatment of patients shifted mainly towards tailored therapies, and the development of new classes of anticancer drugs increased, defining the beginning of the molecular era of targeted therapy. The so-called “targeted therapy” refers to drugs that are aimed at interfere with specific molecular target that is thought to play an important role in tumor development and progression. One of the first and most successful examples of targeted therapy is imatinib, a small molecule receptor tyrosine kinase (RTK) inhibitor that targets a variety of RTKs. The use of imatinib in tumors harboring activating mutations of RTKs (e.g., gastrointestinal stromal tumors, dermatofibrosarcoma protuberans) or oncogenic RTK fusion proteins (e.g., chronic myeloid leukemia positive for the BCR::ABL fusion, myelodysplastic/myeloproliferative disorders associated with PDGFR gene rearrangements), leads to increased life expectancy for patients whose prognosis was previously very poor (Druker et al., 1996; Druker et al., 2001; Demetri et al., 2002).
The specificity of targeted therapies usually gives these drugs particularly high efficacy while reducing off-target toxicity to normal cells, but it is also responsible for mechanisms of tumor resistance. It is noteworthy that not only the presence of a mutated drug-responsive gene, but also the type of mutation on the same gene can play a role in drug response. The EGFR gene in particular can serve as an example. Most glioma tumors are dependent on EGFR signaling, making approved drugs targeting this gene attractive for precision oncology of gliomas. However, most clinical trials have failed to demonstrate the benefit of EGFR-targeted therapies in gliomas, as approved EGFR therapies have mainly focused on NSCLC EGFR gene alterations that are, however, distinct from those driving gliomagenesis (Lin et al., 2022). On the other hand, secondary resistance occurs when a fraction of cancer cells develops new acquired mutations or alterations in antigen presentation, which can also drastically affect the efficacy of targeted therapies. Transcriptional deregulation and de-repression of alternative RTK are also common strategies to facilitate adaptive evasion signaling, which is likely promoted by epigenetic changes (Jun et al., 2012; Akhavan et al., 2013). The presence of resistant cells forces clinicians to dose escalate with the risk of increased toxicity or to switch molecular targets with the risk of no new therapeutic options being available.
In the scenario of personalized cancer therapy, the assessment of the individual status of the tumor immune system also plays an important role and has led to the development of nanoparticles, monoclonal antibodies, and chimeric antigen receptor T-cell therapies (CAR-T) as well as antibody-drug conjugates. Some examples include the generation of third-generation CAR-T cells against the oncoembryonic antigen ROR1 (Meng et al., 2023) and the study of the chemokine expression signature that correlates with the characteristics of T-cell inflammation and potential response to immune checkpoint inhibitors in various cancers (Romero et al., 2023).
Another goal of precision medicine is to identify new molecular biomarkers that can monitor both disease stage and the efficacy of selective therapies. In this context, tumor molecules secreted in the bloodstream, such as microRNAs, non-coding RNAs, exosomes, and circulating tumor DNAs (ctDNAs) are of particular interest and can be detected without invasive procedures thanks to liquid biopsy. For example, the expression of circulating miR-221/222 correlates with response to and resistance to tamoxifen in the luminal subtype of breast cancer patients (Patellongi et al., 2023), circRNA_047733 can be used as a biomarker for risk assessment of lymph node metastasis in patients with oral squamous cell carcinoma (Deng et al., 2023), and baseline ctDNA mutation frequency can be used as a prognostic marker in patients with metastatic colorectal cancer (Bachet et al., 2023). In addition, measurement of ctDNA at specific time points by liquid biopsy can also be used as a biomarker of efficacy and toxicity to guide the dose and schedule of radiotherapy in cancer patients (McLaren and Aitman, 2023).
Finally, several cancer hospitals have interdisciplinary teams of experts, called molecular tumor boards, that recommend a patient-specific therapeutic strategy based on data from NGS profiling. A retrospective study of biliary tract tumor patients showed that comprehensive genomic profiling along with molecular targeted therapy discussed by the molecular tumor board resulted in a higher response rate and better overall survival for patients who received the recommended treatment (Zhang D et al., 2023). In addition, these molecular tumor boards can bridge the gap between research and the clinic by recruiting patients early for clinical trials (Weiss et al., 2023).
As discussed in the following section, various genetic polymorphisms that can be studied using omics experiments and that are located in genes associated with drugs PK and/or PD, can influence efficacy and toxicity.
In addition to targeted therapy, NGS approaches can support the concept of personalized medicine by being included in PGx studies and linked to drug safety profiles (Figure 2A). Sequencing results can thus be associated with the identification of novel biomarkers related to drug efficacy and toxicity.
FIGURE 2. Personalized medicine and PGx studies. (A) Aims of personalized medicine. Patient data from multiple sources (health records, medical imaging and omics data) are combined to identify a patient-specific fingerprint that determines response to therapy, efficacy and toxicity. (B) PGx study design strategy. Three drug phenotypes will be identified (responders, non-responders and toxicity) and different populations will be studied to identify the genetic traits involved in particular drug phenotype. The strategies are divided into candidate genes, GWAS and NGS. The aim of PGx studies is to stratify the population based on genetic background to maximize drug efficacy and reduce toxicity. Abbreviations: GWAS, Genome-wide Association Study; NGS, Next-Generation Sequencing.
The three main research strategies for PGx biomarker discovery are candidate gene studies, Genome-Wide Associated Studies (GWAS), and NGS (Figure 2B). Candidate gene studies are based on genotyping or sequencing of genes known to be involved in PK and PD processes to uncover potential variants; this is the main approach taken so far. This approach is based on “a priori” knowledge, as genes are selected based on their membership in specific pathways (Malta et al., 2018; Kwok et al., 2022; Maeda et al., 2023). A limitation of this approach is that polymorphisms that are part of unexplored pathways and may alter the phenotype of the drug response are not detected. However, this approach may have higher statistical power than other approaches even with few samples (Chan et al., 2019).
On the contrary, GWAS discovers millions of SNPs across the genome and has the potential to find variants in unexplored genes and intergenic regions not previously thought to affect drug response (Uffelmann et al., 2021). One example is the germline variants in the PRUNE2 and BARD1 genes, which have prognostic potential in advanced colorectal cancer and ovarian cancer, respectively. In addition, the variants in the AGAP1 gene may affect patient response to bevacizumab (Quintanilha et al., 2022b). Moreover, most of the SNPs detected are not the causal variants responsible for the observed phenotype, but are instead associated with the presence of functional variants in high linkage disequilibrium in a given population (Bei et al., 2010). One of the major limitations of this technique is the low statistical power in detecting associated signals for rare polymorphisms and thus the inability to find variants with small effect sizes, especially when a drug effect trait is not directly associated with drug effect or is population/region specific (Wang et al., 2019).
Finally, NGS approaches offer the possibility of generating a large amount of information on novel, common, or rare variants potentially associated with drug response, such as GWAS, but suffer less from the requirement of a large number of samples to achieve statistical power, overcoming the drawbacks of the other two SNP approaches (Sharma et al., 2014; Auwerx et al., 2022). However, NGS is not a panacea for identifying all inheritance patterns in PGx. The lack of standardization of NGS techniques and limitations in sample quality or quantity are issues that should be addressed to achieve comprehensive and robust detection and association of somatic and germline variants involved in individual drug response (Mu et al., 2019).
Although candidate gene studies, GWAS, and NGS enable the identification of variants potentially involved in individual drug response, the results obtained in these studies require internal and external validation before they can be adopted in clinical practice. Validation strategies include case/control studies, cross-validation based methods, and an independent series of patients to confirm results. In addition, orthogonal technical validation using low-throughput methods is required, with real-time PCR with TaqMan assay and pyrosequencing often being the first choice (Arbitrio et al., 2021; Hertz et al., 2021).
The efficacy of a drug is related to its plasmatic concentration, which is a surrogate for measuring the percentage of the administered dose that can reach the molecular target. Genetic variants in genes involved in PK or/and PD can affect the efficacy of a drug, as discussed below. Although much of the variation is due to PK (Roden et al., 2019), PD variations may also be important for treatment efficacy.
Classic examples of PGx in drug response involving PK mechanisms include glutathione S-transferase, cytochrome P450 genes and MDR1 gene polymorphisms. Glutathione S-transferase (GST) is a class of metabolic enzymes that conjugates glutathione to xenobiotics for detoxification purposes. GST substrates include anthracyclines and cyclophosphamide, anticancer drugs used in breast cancer protocols. In particular, the GG genetic variant in the GSTP1 gene (c.313A>G) appears to be associated with a lower risk of chemoresistance in breast cancer patients treated with doxorubicin (Romero et al., 2012) and a lower risk of death in patients treated with cyclophosphamide compared to patients with proficient-GST (Sweeney et al., 2003). One possible mechanism to explain these observations is that decreased GST activity leads to an increase in the systemic dose of active metabolites, which in turn results in a better therapeutic effect, even if this increases the risk of toxicity.
Cytochrome P450 is one of the most important enzyme classes involved in the metabolism of xenobiotics. This group includes the enzyme CYP2D6, which is involved in the conversion of tamoxifen to its more active metabolites, 4-hydroxytamoxifen and endoxifen. Genetic variants in the CYP2D6 gene (*4, *5, *10 and *41) result in impaired enzyme activity, leading to lower production of active tamoxifen metabolites and shorter overall survival in cancer patients taking tamoxifen (Schroth et al., 2007).
Genetic variants affecting transporters may also play a role in altered drugs response. A silent polymorphism in the MDR1 gene, one of the best-known efflux pump proteins involved in drug resistance mechanisms, has been shown to affect the timing of MDR1 mRNA translation into folded protein, thereby reducing total protein levels (Kimchi-Sarfaty et al., 2007).
Novel PK-related genetic variants have also been discovered. Germline polymorphisms in the NT5C2 gene (e.g., rs72846714) were recently discovered in a GWAS study, and some of them have been linked to 6-mercaptopurine (6-MP) metabolism in patients with acute myeloid leukemia, as they are responsible for differential activation of 6-MP and thus its bioavailability (Jiang et al., 2021).
At PD, it can be speculated that any variant (germline or somatic) that affects the accessibility of the drug to its target or the affinity of the drug binding may result in altered drug efficacy. These scenarios include variants that alter the amino acid sequence at the core binding site between the drug and the target, thereby affecting binding affinity, variants that alter the spatial conformation of the protein and may lead to partial misfolding, and alterations in weakly bound bridges between individual nucleic bases. In NSCLC patients treated with the EGFR inhibitor gefitinib, patients with specific in-frame indel mutations in the EGFR gene were more sensitive to gefitinib, as these mutations increase the tumor’s dependence on growth factor signaling, compared to patients without such mutations. Therefore, patients with these mutations respond better to gefitinib than patients who have other mutations (Lynch et al., 2004).
In glioblastoma, EGFR mutations and amplifications account for at least 50% of molecular alterations (Brennan et al., 2013). The EGFR variant III (EGFRvIII) is the product of the most common deletion in GBM, resulting from the deletion of exon 2-7 of the extracellular domain (ECD). This alteration occurs predominantly in cancer cells and in approximately one-third of GBM, making this variant an ideal epitope for immunotherapy. Rindopepimut is a peptide-based cancer vaccine that targets EGFRvIII. Although EGFRvIII is an extremely attractive therapeutic target, tumor cells escape this immune-mediated therapy by losing the EGFRvIII expression as a resistance mechanism (Binder et al., 2018).
Epigenetic changes may also affect PGx. Hypermethylation of MLH1, which is involved in the mismatch repair system, may affect the response to cancer therapy targeting this pathway (Wu F et al., 2015; Bukowski et al., 2020; Loukovaara et al., 2021). In addition, altered histone modifications that may occur during tumorigenesis and other pathological conditions may lead to heterogeneous expression of drug efflux proteins and thus affect PK (Kondo and Issa, 2004; Baker et al., 2005; Wu L-X et al., 2015). In particular, demethylation of the ABCB1 gene in cancer cells can lead to a reduction in the accumulation of anticancer drugs in cancer cells, resulting in the acquisition of a resistant phenotype (Toth et al., 2012).
Drug toxicity refers to a variety of adverse effects associated with the use of a particular drug. The mechanisms of drug toxicity can vary widely and include four main aspects: on-target toxicity, off-target toxicity, hypersensitivity reactions and idiosyncratic reactions (Guengerich, 2011). Genetic polymorphisms may enhance or attenuate these reactions.
On-target toxicity refers to the adverse effect of a particular drug depending on its mechanism of action. This phenomenon is related to the binding of the drug to its therapeutic receptor, but in a different body compartment. Polymorphisms that enhance this type of response include rs9501929 of the TUBB2A gene, which encodes the β-tubulin protein. Although there are conflicting opinions about its clinical utility, rs9501929 may alter the toxicity profile of paclitaxel, an antimitotic drug that binds specifically to β-tubulin to arrest cell cycle progression. Patients carrying this variant have a higher risk of developing paclitaxel-induced neuropathy, a disease characterized by abnormal aggregation of microtubules in neurons (Abraham et al., 2014). This effect can be explained by the fact that β-tubulin is also targeted by paclitaxel in normal neurons, which is, by definition, an on-target toxicity. The lack of a selective and specific target for cancer cells is one of the major limitations of conventional chemotherapy such as paclitaxel.
Off-target toxicity refers to the adverse effects of a drug that binds to both its therapeutic and nontherapeutic receptors and is also related to mechanisms that are independent of the mechanisms of action (Rudmann, 2013). Some of these issues can be addressed in the preclinical stages of drug development by altering the structure of the drug to modulate its affinity to undesirable receptors. In addition, local administration, if applicable, may also partially help (Li M et al., 2022). Hypersensitivity reactions and idiosyncratic reactions depend on the activation of the immune system and the intrinsic characteristics of patients, respectively (Doña et al., 2014).
The best characterization of adverse reactions involves genes from PK processes. Individual variants in transporters and metabolic enzymes are responsible for most differences in drug response. In particular, polymorphisms in the gene SLCO1B, which encodes the OATP1B1 transporter responsible for cellular uptake of multiple substrates, can impair the availability of irinotecan and lead to drug toxicity (Nozawa et al., 2005; Di Martino et al., 2011). In addition, part of irinotecan is oxidized by CYP3A4, while another part of irinotecan is activated to SN-38 and its glucuronide conjugate SN-38G. These reactions are catalyzed by carboxylesterase and UGT1A1, respectively. The genetic variant UGT1A1*28 is associated with decreased glucuronidation activity, which in turn prolongs the mean half-life of the metabolite SN-38 and increases patient susceptibility to gastrointestinal and hematologic toxicity (Iyer et al., 2002; Innocenti et al., 2004; Peeters et al., 2023). Similar toxicities to irinotecan have been noted in patients carrying polymorphisms in the ETS1 and ABCG2 genes, which encode carboxylesterase and a membrane transporter, respectively (De With et al., 2023). The UGT1A1*28 polymorphism, together with the UGT1A1*60, UGT1A1*6, and UGT1A1*27 polymorphisms is associated with the metabolism of several anticancer drugs such as belinostat, an HDAC inhibitor, nilotinib and pazopanib, two RTK inhibitors. Thus, loss-of-function alleles are responsible for increased toxicities, such as neutropenia, thrombocytopenia and prolonged QTc intervals in patients treated with belinostat (Goey et al., 2016; Balasubramaniam et al., 2018).
Another example is 5-fluorouracil (5-FU), an antimetabolite that has long been used to treat tumors of the stomach, colon and rectum. Approximately 80% of 5-FU is converted to the inactive metabolite 5,6-dihydrofluorouracil by the rate-limiting enzyme DihydroPYrimidine Dehydrogenase (DPYD). Genetic mutations in the DPYD gene associated with lower DPYD activity, such as *2A, *13 and rs67376798, can lead to fluoropyrimidine toxicity (Caudle et al., 2013; Glewis et al., 2023; Lešnjaković et al., 2023).
Germline mutations in the TPMT gene, as well as in the NUDT15 gene, may otherwise affect the metabolism of the thiopurines 6-mercaptopurine (6-MP) and 6-thioguanine (6-TG). In thiopurine metabolism, TPMT is a key enzyme that converts 6-MP and 6-TG to their inactive metabolites. Patients with loss of function alleles have higher circulating thiopurines levels, which increases the risk for developing myelosuppression, a common adverse effect of these drugs. In these patients, a lower starting dose is recommended to minimize toxicities (Wang et al., 2010). The same precautions should be observed in patients with loss-of-function alleles of the gene NUDT15, which is also involved in the metabolism of thiopurines (Moriyama et al., 2016). Genetic testing for TPMT and NUDT15 genes has entered clinical practice and is strongly recommended for cancer patients who are to receive thiopurine therapeutics (Relling et al., 2019).
Some of the classic gene polymorphisms such as cytochrome P450, DPYD, UGT, TPMT, and HLA have already entered clinical practice. Panels of specific genes are routinely used to determine the optimal therapeutic window in cancer patients and their utility has been demonstrated. A recent multicenter implementation study evaluated a panel of 12 genes for pharmacogenetic testing in several European countries. The most important finding of this study, in addition to demonstrating that genotyping of this 12-gene panel leads to a reduction in the incidence of relevant adverse drug reactions, is the cross-national feasibility of these genetic tests, which paves the way for harmonization of genotyping (Swen et al., 2023).
In summary, PGx testing offers a number of benefits, including enhancing intended treatment benefits, reducing the likelihood of adverse effects and risk of dependence, reducing healthcare expenditures and the need for hospitalization in the event of severe adverse events, and shortening the time to achieve therapeutic effect. Although researchers and clinicians are increasingly aware of the importance of genetic testing for personalized oncology, global clinical implementation is still lacking, in part due to the need for standard procedures, cost reduction, but also support from healthcare systems, especially in less affluent countries.
Machine learning (ML) is a subfield of artificial intelligence that aims to make predictions and inferences within a certain range of accuracy by analyzing multiple variables in input data, such as clinical and/or molecular data (Mitchell, 2013). In addition, without explicitly programming, ML can find hidden patterns and identify relationships between multiple variables to correctly predict the outcome.
Machine learning has indeed proven to be a powerful tool in cancer research, as it has the potential to improve cancer diagnosis, classification and prognosis (Kourou et al., 2015; Cui et al., 2022a). An example of the use of ML in cancer research is the study of medical imaging, where large amounts of data are available that are difficult to analyze. In particular, the emerging field of radiomics uses images routinely produced in clinical settings to evaluate patients undergoing treatment to develop a ML approach to disease detection (Lambin et al., 2012). Another example is radiogenomics, where key features extracted from radiological images can be linked to the genetic profile of the tumor. Thanks to the linkage of image and genotype highlighted by ML, radiogenomics offers the possibility of becoming a noninvasive surrogate for genetic testing (Meißner et al., 2022).
Other important approaches of ML in cancer research focus on treatment. Predicting how a particular tumor will respond to therapy, or which patient characteristics better predict response to therapy, is a fundamental goal of modern oncology that should ultimately lead to tailored treatment. For example, genetic profiles and clinical information of breast cancer patients from a complete study dataset were used to train a ML algorithm to predict the 5-year survival rate of these patients who underwent a specific medication (Tabl et al., 2019). In this context, genomic profiling can provide information about the role of biological pathways in cancer cells and their relationship with a specific medication, thus helping clinicians to tailor treatment for patients based on their molecular background.
A detailed description of ML is beyond the scope of this review; however, we provide here an overview of the main features of the algorithms of ML.
Supervised learning, unsupervised learning and reinforcement learning are three main types of machine learning approaches (Van Der Lee and Swen, 2023). A more classical classification based on the model built using this approach is divided into supervised, unsupervised and semi-supervised models based on the type of input data, i.e., whether it is labeled, unlabeled or a combination of both (Koteluk et al., 2021; Naik et al., 2023). A graphical representation of the concept is shown in Figure 3A.
FIGURE 3. Machine learning algorithms. (A) ML main classification based on input data labels and possible outputs. (B) Trade-off between accuracy and interpretability for ML models grouped by supervised, unsupervised or both types of algorithms (C) ML fusion modeling. The light blue box represents the input data (health records, medical imaging and omics data), the green box represents the type of ML fusion: early fusion (top) computes a single ML model; intermediate ML fusion (middle) computes two or more ML models, with the final model using the output of previous model as input; late fusion (bottom) creates multiple ML models and then fuses the outputs of each model to produce the data output. The light red box represents examples of the outputs obtained through ML, from the top to the bottom: Classification, Decision Tree, Regression, Clustering. Abbreviation: LVM, latent variable model; SVM, support vector machine.
In supervised models, which account for the majority of published ML methods, each data point contains an associated label (correct/expected response) for which a ML model must be developed. Typically, the data is split into two subsets: the training data and the testing data. The training data is used to tune and train the model (Shahin et al., 2023). The testing data is used to evaluate the generality of the model. In addition, supervised learning can be used to solve regression and classification problems. In regression problems, the labels are continuous values, while in classification problems labels are discrete values. Finally, the metrics used to assess the quality of the model depend on the nature of the problem and the type of application (Boyd, 2010; Sra et al., 2012; Orozco-Arias et al., 2020; Woodman and Mangoni, 2023).
In unsupervised models, the output label measurement is usually not available. In this case, the algorithm learns relationships from data structures to provide latent patterns that need to be evaluated for utility. However, this process still requires human intervention to validate the output variable. In general, unsupervised methods deal with clustering and dimensionality reduction, leading to the identification of subgroups with common features, which is one of the main applications of unsupervised ML (Sajda, 2006; Handelman et al., 2018).
Reinforcement learning is a type of learning where an agent learns to make decisions by interacting with an environment. The agent receives feedback in the form of rewards or penalties for its actions and learns to maximize the cumulative reward over time (Woodman and Mangoni, 2023). Unlike supervised and unsupervised learning, reinforcement learning does not require labeled data or a training set. This type of learning is often compared to a scenario where an agent learns through trial and error (Shahin et al., 2023). Reinforcement learning can be used to solve problems that deal with complex dynamics that are influenced by changing stimuli and conditions, such as in the real clinical world (Eckardt et al., 2021; Ryan et al., 2023). In particular, reinforcement learning could help physicians to select the right therapeutic regimens for a patient, and it is able to correct its predictions based on the observation of the adverse reaction resulting from the interaction between the agent and the environment (Niraula et al., 2021; Ryan et al., 2023).
Semi-supervised approach is a method in which there is a mixture of labeled and unlabeled data (Ge et al., 2020; Shi et al., 2021; Roy et al., 2022). There have been significant developments in the field of semi-supervised learning, as researchers have proposed various techniques to make effective use of the combination of labeled and unlabeled data. These techniques aim to overcome the challenges posed by the limited amount of labeled data and the growing volume of unlabeled data (Eckardt et al., 2022; Dou et al., 2023).
In ML it is important to note the differences between prediction and inference. These two terms, often used as synonyms, are used differently in ML algorithms. Prediction is about estimating or predicting unknown outcomes, while inference is about understanding the factors and relationships that contribute to those predictions. Both aspects are crucial in ML, as prediction enables useful forecasts, while inference helps to gain insights into the underlying mechanisms and to learn from the trained models (James et al., 2013). Depending on the objective, ML algorithms have been developed to make predictions, inferences or a combination of both.
An important issue to address when discussing ML is the trade-off between model accuracy and interpretability. Some approaches are easier to interpret, but are more rigid and less accurate, because they may be based on linear functions such as linear regression. Conversely, other ML models are more flexible in estimating the functional form of the function but can be difficult to explain (Eckardt et al., 2022; Kang et al., 2023). Figure 3B illustrates the trade-off between flexibility and interpretability for some of the most commonly used ML approaches.
ML input data can be of different origins, e.g., clinical data, medical imaging, omics, time series. The use of a single type of input data is characteristic of unimodal ML, while the use of different types of input data is a feature of multimodal ML. Each type of data can be modeled in different ways, resulting in early, intermediate and late fusion (Figure 3C). In early fusion, the input data types are merged at the beginning to create a single ML model. In intermediate fusion, ML models are created interlocked, each refining the previous model. Late fusion creates separate unimodal models that are combined into a final model. The multimodal ML provides more comprehensive and accurate predictions than unimodal models. Moreover, within the multimodal approaches, the intermediate and late fusion strategies achieve better results because they take complementarity information into account when training the model (Steinberg et al., 1999; Kline et al., 2022). In cancer research these multimodal approaches are considered very useful, but their application is not so obvious. ML late fusion strategies can be used to improve the oldest diagnosis criteria, tumor classification and subtype identification, as in the case of NSCLC, where a study shows that fusion of 5 different sources of information achieves the better performance in classification compared to algorithms using only single source information (Carrillo-Perez et al., 2022). In addition, they can be used to develop software for cancer theranostics, a cancer control strategy that combines early diagnosis, accurate molecular imaging, and personalized radiation treatment (in terms of chosen agent, dose, and timing) based on the individual omics profile.
Numerous ML methods have been developed for medical research and recent applications of ML are summarized in Table 1. Here we give an overview of the main algorithms used in the field of oncology (Figure 4), namely, k-means clustering and hierarchical clustering, latent variable model, support vector machine, decision tree learning, and neural networks. For neural network algorithms, we focus on Deep Learning (DL), which is becoming increasingly important in cancer research.
TABLE 1. ML algorithms in cancer research in the last 2 year. In this table, we summarize the most important research topics in cancer research using ML. For each publication, we describe the type of the ML algorithm used and the research outcome of the selected study. Publication years: 2021–2023.
FIGURE 4. Main uses of ML algorithms in cancer research. Light yellow box represents preclinical studies where ML has been demonstrated to be effective. Light blue box represents the phases of drug utilization in target populations where ML has taken improvements. In the green light column, different ML algorithms that can be used for each research topic. Abbreviations: LVM, latent variable model; SVM, support vector machine.
In unsupervised learning, ML methods are not task-specific (i.e., they are not based on a specific predicted outcome, such as survival), provide general insights, and include methods such as k-means clustering and hierarchical clustering. These methods have been used in oncology to identify cancer subtypes, stratify patients, and create clusters from gene expression data to identify patterns and groupings (Eckardt et al., 2023). Another example of unsupervised learning is the latent variable model (LVM), which can capture unobserved variables that may affect the outcome. LVM can therefore be used to regress variables into one or more classes that would best explain the heterogeneity in the data (Miettunen et al., 2016).
Supervised methods include the support vector machine (SVM), which divides data into categories (two or more) to solve both regression and classification problems. It is based on kernel algorithms that can expand the feature space to make data more accessible. It is considered one of the most robust models to date (Tate et al., 2006; Pacurari et al., 2023).
Decision tree learning is a supervised model that is commonly used in clinical practice for decision-making processes. In this case, ML can help identify which variables have the highest separability to the desired categories and is used for both classification and regression (Podgorelec et al., 2002). Random Forest (RF) is an extension of decision tree learning that combines multiple randomly generated decision trees to improve decision making. Given the complexity of biology, data scientists usually prefer RF to decision tree (Breiman, 2001; Cui et al., 2022b).
Finally, neural networks (NNs) are models used in all three types of learning. NNs, which mimic the neural architecture of the human brain, can integrate multiple sources of information and process them in nodes and layers. Each node represents a specific feature with a specific weight, and nodes of the same level represent a layer. Moreover, nodes of different layers can be connected to each other. If the information stored in the node is valuable, the node weight exceeds a certain threshold, which means that the node is triggered and the network is active. During the training, the weight values and threshold are continuously adjusted to form the best combination of nodes and weights that results in the most informative NN (Kriegeskorte and Golan, 2019).
Deep Learning (DL) is one of the NN algorithms where the number of hidden layers and nodes is increased and the overall size of the network is very large, which allows better representation of complex relationships. The main advantage of DL is that it identifies hidden features as part of the learning process, making DL faster and more automated compared to ML (Erickson et al., 2017). These features also correlate with sensitivity and availability of cheaper computing power. Therefore, DL is now referred to as a specific subset of ML with its own algorithms and applications, and has become one of the most widely used approaches in cancer research. Thus, in the following part of this section, we discuss some applications of DL in cancer research.
The use of DL in oncology began with the analysis of medical images, because it is particularly good at identifying pathogenic features of the observed cells, and in certain cases the performance of DL is almost equal to human performance (LeCun et al., 2015; Jalloul et al., 2023). For example, the application MIA was developed to analyze images from microscopy and can be used for classification, object recognition, segmentation, and tracking (Körber, 2023). In addition, medical images of histopathological tumor sections were used to test whether DL can predict response to therapy in patients with adenocarcinoma of the gastroesophageal junction. In this work, researchers found that DL is able to distinguish patients who respond to neoadjuvant chemotherapy from those who do not by extracting certain features on the images before therapy initiation (Hörst et al., 2023). A Swedish study has developed a DL tool for detecting lymph node metastases in colorectal cancer that has excellent accuracy compared to human performance. This tool reduces the time required to assess lymph nodes, which in turn improves the diagnostic process and treatment decisions (Kindler et al., 2023). Another DL tool has been developed to assist clinicians in digital pathology by assessing the tumor cellularity of histopathologic hematoxylin and eosin sections (Altini et al., 2023). Apart from the importance that this algorithm may have in the clinical setting, it is important to point out that its use may also be useful in research, as it allows pathologists to share valuable information with researchers in an automated manner. A high percentage of tumor cells is an important requirement for researchers to perform NGS sequencing, as the biological material taken from the slice must be representative of the tumor in order to reduce the contribution of normal adjacent tissue, thereby reducing background noise and improving sequencing quality. In addition, DL has been successfully developed to predict optimal radiotherapy for patients with brain metastases using CT images and non-image clinical information (Cao et al., 2023). It has also been developed to predict pneumonitis risk in lung cancer patients treated with immune checkpoint inhibitors and to identify morphologic features that predict ERBB2 status and trastuzumab efficacy in breast cancer patients (Bychkov et al., 2021; Cheng et al., 2023). In a retrospective multicenter study, a DL algorithm was developed to help radiologists diagnose breast cancer lesions and differentiate axillary lymph node metastases based on radiological features (Zhou et al., 2023).
Although medical imaging remains the foremost application of DL, it is also used in the analysis of genomics and transcriptomics data, including data from single-cell experiments that can improve variant detection calling at cell-specific resolution. DL improvements in single-cell sequencing could enhance the ability of researchers to understand intratumoral heterogeneity and identify previously unknown cell subpopulations, making this a particularly attractive area for molecular oncology (Erfanian et al., 2023; Halawani et al., 2023; Shen et al., 2023). DeepTTA is a DL model that uses transcriptomic data to predict anticancer drug response, which can shorten the preclinical phases of drug development and drug screening. In addition, DL models which can predict cancer drug response can also be used to identify new potential clinical applications of known drugs based on target affinity and mechanism of action (Douglass et al., 2022; Jiang et al., 2022; Park et al., 2023). DeepTAP is another DL algorithm capable of predicting sequence peptides that bind to tumor neoantigens, which may be of interest to the field of cancer immunotherapy (Zhang X et al., 2023). With this in mind, DL algorithms are being used in precision medicine to predict anticancer drug response in patient-derived cancer cell lines, as in the case of the DeepDRK framework, which is freely available (Wang Y et al., 2021). A method based on DL was developed to study the uptake of targeted nanoparticles in triple-negative breast cancer, which could be useful for proper dosing in clinical practice (Ali et al., 2022). A nanodiamond biosensor platform using DL was developed to rapidly assess individual specific sensitivity to oxidative phosphorylation inhibitors in patients with hepatocellular carcinoma (Xu et al., 2023).
Finally, network pharmacology is a new approach in drug development that aims to understand the network interactions of multiple drug combinations. In this context, the network algorithms of DL may be useful to identify synergistic combinations of multiple drugs targeting a specific network that can be used to improve cancer treatment (Noor et al., 2023). DeepDTnet, for example, is a DL method for network-based target identification that reveals novel therapeutic effects of known molecules, which in turn can accelerate drug repurposing, a process aimed at finding new uses for drugs that are approved or in trials (Zeng et al., 2020). The strategy of drug repurposing offers numerous advantages over developing a completely new drug, such as a lower risk of failure because safety and risk assessment have already been tested, cost and time savings in the preclinical phases, and finally, phase I and II results are already available, thanks to sophisticated algorithms such as those used in DL and ML (Pushpakom et al., 2019). Based on this approach, many oncology and non-oncology drugs have been reviewed in recent years, and drug repurposing is particularly valuable in rare or late-stage diseases where the development of a new drug may be difficult in terms of patient recruitment and the time required for a complete clinical trial may be unreasonable.
In PGx studies, DL has also been used to predict the toxicity of specific medications. In recent works, DL methods were able to predict the toxicity of radiation-based therapy in four different cancer types (Tan et al., 2023) and identify SNP signatures associated with urinary symptoms and overall toxicity in prostate cancer patients treated with radiation therapy (Massi et al., 2020). An important implementation of DL in PGx studies may interest medical imaging with feature extraction that predicts drug response based on SNP signatures. However, this task is very difficult to accomplish because the DL algorithms used to scan medical images are designed to extract “abnormal” features, and PGx often refers to germline (i.e., “normal”) variants. However, in this way, it would be possible to reduce the number of diagnostic tests a patient has to undergo, also in the context of the most appropriate choice of therapy after diagnosis.
The use of DL, as well as ML in general, can improve healthcare and assist clinicians by shortening the time required for diagnosis and staging and facilitating decision-making in drug selection and administration of the correct dose, thus contributing to the clinical translation of precision medicine in cancer.
PGx studies have gradually shifted from reactive testing on a single gene to proactive testing on multiple genes to improve treatment outcomes. This move has been made possible by the implementation of high-throughput data generation and analysis.
With the advent of ML and computer science in cancer research, it is now possible to discover previously unknown cancer-related features and latent signatures that impact tumor development, progression and recurrence. In addition, ML offers the opportunity to gain insight into failed clinical trials to understand their limitations and potential benefits, and to prevent toxicity and other drug effects that can impact patient quality of life and treatment efficacy (Harrer et al., 2019).
One of the first constraints in screening new drugs is selecting candidate molecules from the initial bulk of drug libraries. By combining genomic features of cell lines and chemical information of molecular compounds, researchers have been able to create in silico ML multi-drug models to predict IC50 values, saving cost and time (Menden et al., 2013). Furthermore, these in silico approaches enabled the identification of genomic events associated with altered drug sensitivity, optimizing drug trial design (Huang et al., 2017).
In cancer, multitherapy is often used not only to reduce the toxicity of a single anticancer agent and achieve synergistic effects, but also to overcome drug resistance (Dear et al., 2013; Lee et al., 2019; Kim et al., 2020). Screening to predict synergistic drug combinations is a computational approach that has been explored using ML technology. For example, screening multiple administrations of over 40 different drugs in melanoma cancer cells led to the identification of 11 validated, previously untested drug combinations that lead to different outcomes (Gayvert et al., 2017).
As mentioned earlier, there is growing evidence that optimal prediction of drug response relies on individualized molecular profiling (Bode and Dong, 2017). Many ML approaches in PGx studies have been developed to predict the best match between genetic alterations involved in the pathogenesis or recurrence of a given cancer and drugs targeting these alterations (Chang et al., 2018). From this perspective, therapeutic drug monitoring (TDM) is an experimental procedure that measures the plasmatic concentration of a given drug in a specific time window after administration. Recent work has shown that ML methods applied to TDM were able to predict the appropriate dosing for various drugs, e.g., lapatinib dose for patients with metastatic breast cancer (Yu et al., 2022) and cisplatin dose in cohort of patients with head and neck cancer to avoid cisplatin-related toxicity (Cauvin et al., 2022).
Integration of different omics information better captures the complexity of cancer through different molecular layers and therefore improves diagnosis, prognosis and treatment compared to using a single “omic” alone (Heo et al., 2021).
There are many examples of successful integration of multi-omics approaches. Whole genome and transcriptome data have been used to quantify the extent of specific genetic alterations at the mRNA level and derive quantitative trait loci (eQTLs). In this context, polymorphisms associated with specific phenotypes are found to be associated with eQTLs, and genetic risk factors associated with eQTLs can bona fide predict the level of the corresponding gene product (Nicolae et al., 2010).
Epigenomics and transcriptomics/proteomics data can be aligned to explain how epigenetic changes can affect protein turnover (Wang X et al., 2021). In addition, transcriptome sequencing has often been combined with miRNome sequencing to determine which microRNAs and non-coding RNAs may alter gene expression and modulate response to chemotherapy (Fazi et al., 2015; Cuttano et al., 2022). Many consortia and catalogs have been developed to promote the understanding of tumor processes with high-throughput data, such as the Clinical Proteomic Tumor Analysis Consortium (CPTAC), which integrates genomic and proteomic data to create a proteogenomic portrait of cancer toxicity and resistance (Ellis et al., 2013), the International Cancer Genome Consortium (ICGC), which provides cancer genomic, DNA methylation and gene expression data (Zhang et al., 2011), and The Cancer Genome Atlas (TCGA) program, which provides a collection of genomic, epigenomic, transcriptomic and proteomic data on 33 different cancer types (The Cancer Genome Atlas Research Network et al., 2013). These data collections are the tip of the iceberg of the various omics data consortia that have emerged to date, and they serve as a valuable resource for omics and ML modeling studies of cancer.
The application of ML with the integration of multi-omics data has resulted in several scores for risk prediction and diagnostic/therapeutic potential, such as the polygenic risk score. The polygenic risk score considers all genetic inheritance variants known to be associated with a particular disease and measures the risk associated with the development of the disease under investigation, thereby improving risk stratification and screening (Akdeniz et al., 2023). Other examples include the BRECADA application, which uses genetic and nongenetic risk factors for early detection of breast cancer, and the OncoNPC signature, which classifies cancer of unknown primary and accordingly tailors initial palliative treatment intent, a strategy that often leads to better patient outcomes compared with cancer treated without querying the OncoNPC signature (Moon et al., 2023; Tao et al., 2023). In addition, some radiomics features have been extracted from images of brain metastases of extracranial primary tumors and correlated with the expression level of PD-L1, allowing stratification of patients according to their sensitivity to immune checkpoint inhibitors using a ML noninvasive classifier (Meißner et al., 2022).
The ability of ML to handle multiple data structures, namely, clinical, molecular and imaging data, allows to discover hidden correlations among different input data in PGx studies to make more accurate predictions and inferences. However, there are several crucial aspects to consider when managing and using multi-omics data that should be examined. First, collection of multi-omics data requires careful evaluation of the entire experimental workflow, from tissue collection and high-quality extraction of nucleic acids and proteins to sample preparation and sequencing. Second, data analysis pipelines need to be developed to integrate individual omics approaches. In this context, early, intermediate and late ML fusions help to address the management of multimodal approaches with positive impact on clinical cancer research. Third, the expertise required for analytical and bioinformatics analyses often requires the collaboration of multiple experts to properly mediate the integration of multi-omics data. Thus, building a multidisciplinary team is therefore challenging for the success of multimodal data integration, but the positive outcomes of multimodal approaches have already been demonstrated and adopted (Kwon et al., 2015; Jing et al., 2020).
Clinical trials are later phases of drug development and incur very high costs. Clinical trials in oncology have the highest overall failure rate, mainly due to poor trial design (Wong et al., 2019). Therefore, the use of ML in clinical trials could be an opportunity to increase success. However, most applications of ML have focused on preclinical studies rather than improving clinical trial design, possibly due to the significant regulatory challenges associated with the use of ML in a clinical context (Massella et al., 2022).
ML improvements in study design can be attributed to three main strategies: cohort composition to improve suitability by reducing cohort heterogeneity, patient recruitment to improve eligibility by maximizing patient-study match, and patient monitoring to improve adherence and endpoint detection to reduce dropout rates (Harrer et al., 2019; Van Der Lee and Swen, 2023).
As mentioned earlier, oncology clinical trials often fail to meet primary endpoints due to inadequate stratification criteria, poor recruitment and evidence of severe drug toxicity (Hwang et al., 2016; Kim et al., 2023). To address these issues, the RainForest algorithm was developed. The CAIRO2 clinical trial investigated the use of cetuximab in patients with metastatic colorectal cancer and concluded that there was no benefit to using this agent in the overall population. However, the RainForest algorithm was able to identify a small subset of patients who actually benefit from cetuximab treatment based on the SNP germline profile of patients (Ubels et al., 2020). The use of the RainForest algorithm in clinical trials can save enormous resources, as the cost of a single agent is estimated to be around 2.8 million US dollars in the final stages of approval (DiMasi et al., 2016).
Severe toxicity is another major issue in clinical trials, and changes or interruptions to treatment schedules account for at least 30% of failures in phases II and III (Harrison, 2016). ML has also supported the design of clinical trials in term of drug safety. A recent work has shown that SNPs signatures can serve as genetic predictors of toxicity in personalized medicine. The germline variant rs4864950 T>A in the KDR gene increased the risk of composite toxicity (occurrence of any of hypertension, diarrhea and dermatological reactions) in patients treated with the VEGFR TKIs sorafenib and regorafenib (Quintanilha et al., 2022a). In another work, the ABCB1 rs9282564 was the variant most strongly associated with hypertension and nonhematological toxicities in ovarian cancer patients treated with bevacizumab, and SNPs in genes related to the biological oxidation pathway (CYP3A4 rs28371763 and CYP1B1 rs9341266) were the most significant variants associated with hematological toxicity in the same cohort (Polano et al., 2023).
Clinically relevant predictors of toxicity have also been found in many GWAS studies, e.g., SNPs predicting severe skin toxicity in patients with colorectal carcinoma treated with cetuximab (Baas et al., 2018) or predicting dysphagia in patients with nasopharyngeal carcinoma treated with radiotherapy (Wang et al., 2022) or predicting neurotoxicity and leukoencephalopathy in patients with lymphoblastic leukemia treated with methotrexate (Bhojwani et al., 2014). Many other correlations between SNPs and toxicity can be found in the literature. Most importantly, prediction of cancer-related toxicities can prevent deterioration in patients’ quality of life and adherence to treatment and can be used to manage chemotherapy-related adverse effects in the clinical setting (On et al., 2022).
Incorporating assessment of somatic and germline variations into treatment decisions with FOLFIRI in elderly patients with metastatic colorectal cancer has been shown to be effective. This regimen requires assessment of RAS mutations as well as DPYD and UGT polymorphisms prior to treatment with the FOLFIRI protocol (Mathijssen et al., 2003; Morel et al., 2006; Sepulveda et al., 2017; Battaglin et al., 2018). Although PGx test guidelines have already been implemented in clinical practice, another important issue in this context is the implementation of ML in clinical practice. ML has demonstrated its usefulness in retrospectively classifying patients during clinical trials to assess drug safety and prognosis (Chang et al., 2018; Quintanilha et al., 2022a; Chen et al., 2022), but the incorporation of ML into clinical trials and clinical practice is still up for debate. Standard guidelines and protocols need to be thoroughly regulated to obtain comparable information and a precise methodological approach, not only for the algorithms of ML, but also for PGx studies. It is worth noting that different ML models can be applied to the same given subject, as there is no universal applicability of ML algorithms and this could lead to different results in the same dataset.
Moreover, NGS has only recently been introduced into clinical practice to assess diagnosis and prognosis and to evaluate therapeutic strategies, but it is still a niche and there is room for improvement. In addition, sequencing of some parts of the genome remains challenging, e.g., highly polygenic regions, pseudogenes, triplet expansions, low complexity regions, short repetitive sequences, regions of high-similarity, and complex structural rearrangements (Treangen and Salzberg, 2012; Rojahn et al., 2022). It is estimated that approximately 14% of clinically relevant genetic tests are located in these genomic regions (Lincoln et al., 2021), and correct variant identification can be difficult. On the one hand, short tandem repeats and complex structural variants known to play a role in the pathogenesis of certain diseases can now be sequenced using a targeted approach with long reads sequencing technology, as longer reads are expected to generate appropriate sequence length that overlaps better during assembly (Stevanovski et al., 2022). On the other hand, these technical difficulties can be at least partially overcome by adapting NGS analysis workflows accordingly (Rojahn et al., 2022). However, detecting variants in these regions remains challenging and difficult to validate. Detection of rare and very rare mutations with low allele frequency should also be considered. Inclusion of all probes in the ML learning datasets can be helpful, because some isoforms are more informative than others, and pooling them into averages may lead to dilution or loss of this information. NGS and ML can lead to great improvements in PGx studies, as multiple samples and multiple genes can be tested simultaneously, and clinically relevant hidden patterns can be uncovered from complex data structures.
However, ML and DL also have their own limitations. In general, ML algorithms are not error-free: one of the biggest challenges is the learning model itself. Indeed, many ML approaches have problems with underfitting or overfitting, where the data follows the trained data or noise signals too closely, resulting in poor curve estimation. An appropriate size of the training dataset is also important for ML models to learn properly and make accurate predictions. The reliance on large datasets for developing accurate models is also a challenge due to the lack of sample availability. In addition, the relationship between bias and variance also plays a role in obtaining the best performance from ML models. On the other hand, the accessibility of the code used for ML in PGx studies is low (Huang et al., 2017). To further improve knowledge and sharing among distant researchers around the world, platforms for sharing data and code should be established. To this end, computer scientists could have the opportunity to address underestimated problems and find common resources to overcome them. In addition, the development and improvement of multimodal ML methods, such as late ML fusions, may encourage a more holistic view of specific patient characteristics across different input data types.
As previously reported, PGx testing has demonstrated its utility in many situations, and new variants of uncertain significance have been reported thanks to GWAS and NGS PGx studies. On the one hand, the impact of these new variants on PGx testing is still being evaluated. On the other hand, the clinical implementation of genotyping of genes known to be involved in individual drug response needs to be monitored. In particular, the development of genotyping panels should also be improved to enable the translation of new relevant findings into the clinical settings. One of the most important and unanswered questions related to the use of these genotyping panels is how representative they are of individual variability in drug response. Moreover, PGx studies often suffer from a lack of homogeneous tumor samples, which is particularly true for rare tumors and inconsistent sample ancestry origins and incomplete data are also common complaints. Harmonization of samples and data collection, as well as free and easy access to sample datasets, could facilitate PGx studies with ML. Finally, new and standardized scores to track NGS quality, ML accuracy and significance of PGx variants could also be developed to address new tasks.
The molecular revolution in oncology continues to grow, with the paradigm flowing from pathological oncology based on morpho-histological assessment of tumor specimens to molecularly driven oncology, where precise individual molecular features are considered as part of diagnosis, grading and prognosis to tailor treatment to the individual. In this context, not only are targeted molecular therapy and patient genetic characteristics important factors in predicting therapeutic response, but drug repurposing can also be a valuable resource by using drugs approved for other diseases to treat cancer.
The therapeutic margin in cancer treatment is often small because the dose-toxicity curve is often close to the dose-response curve, so even small fluctuations in drug concentration can lead to severe side effects (Lowe and Lertora, 2012). Therapeutic drug monitoring (TDM) has already demonstrated its validity for assessing correct dosing, although its application is still limited to a small number of anticancer drugs (Knezevic and Clarke, 2020). Assessing genetic polymorphisms that may alter drug response can be beneficial for many reasons, including drug safety profile, patient adherence, and cost savings, not only in oncology, where PGx testing has been adopted more rapidly, but also in other areas (Roden et al., 2019). Therefore, proactive testing is becoming increasingly important for developing treatment strategies for patients based on individual genetic variability and needs, from the perspective of even more personalized medicine.
ML can improve understanding of data generated from PGx studies, increase understanding of clinical trial results, predict clinical outcomes, and discover new biomarkers even at very early stages of drug development to identify subgroups of patients who actually benefit from treatment, and subgroups of patients who do not benefit and may experience toxicity. Although the results of ML models derived from high-throughput data should be confirmed by classical functional studies, they offer researchers the opportunity to explore the extensive relationships that exist in biological processes.
Finally, ML can also be used in cancer theranostics, a combination of diagnostic and therapeutic procedures in which radioactive drugs are first used to identify the disease and then to deliver therapies. ML is a very innovative and versatile tool but the adoption of ML into routine clinical practice is still unsettled and does not yet seem to be truly welcome. On the one hand, this feeling can be explained by the lack of standardization and the fact that specific guidelines for the use of ML have not yet been established. On the other hand, the lack of understanding of the hidden algorithms driving ML decisions may be perceived as a barrier to clinicians’ skills and expertise. In addition, there is no single ML model that can solve a particular problem, so the use of a particular model is not tailored to the task at hand, which may increase the risk of complications in ML harmonization. Finally, patients should be informed and their data protected. Therefore, ethical aspects related to the security of individual data and the protection of privacy are a challenge and a mandatory requirement to gain patients’ trust and prevent them from feeling threatened by ML. The innovation that the use of ML could bring to clinical practice is unquestionable and could help to improve cancer treatment towards a more personalized medicine.
AM: Formal Analysis, Writing–original draft, Writing–review and editing. MDB: Visualization, Writing–original draft, Writing–review and editing. GT: Visualization, Writing–original draft, Writing–review and editing, Funding acquisition. MP: Visualization, Writing–original draft, Writing–review and editing, Conceptualization, Supervision.
The author(s) declare financial support was received for the research, authorship, and/or publication of this article. This work is founded by the Progetto di Ricerca Finalizzata Regione Friuli Venezia Giulia Anno 2021 LR 13/2021, art. 8, c. 28-30 to GT (CUP J35F21002710002 of Centro di Riferimento Oncologico di Aviano, IRCCS), and by the Italian Ministry of Health (Ricerca Corrente).
The authors declare that the research was conducted in the absence of any commercial or financial relationships that could be construed as a potential conflict of interest.
All claims expressed in this article are solely those of the authors and do not necessarily represent those of their affiliated organizations, or those of the publisher, the editors and the reviewers. Any product that may be evaluated in this article, or claim that may be made by its manufacturer, is not guaranteed or endorsed by the publisher.
Abraham, J. E., Guo, Q., Dorling, L., Tyrer, J., Ingle, S., Hardy, R., et al. (2014). Replication of genetic polymorphisms reported to Be associated with taxane-related sensory neuropathy in patients with early breast cancer treated with paclitaxel. Clin. Cancer Res. 20, 2466–2475. doi:10.1158/1078-0432.CCR-13-3232
Abraham, J. P., Magee, D., Cremolini, C., Antoniotti, C., Halbert, D. D., Xiu, J., et al. (2021). Clinical validation of a machine-learning–derived signature predictive of outcomes from first-line oxaliplatin-based chemotherapy in advanced colorectal cancer. Clin. Cancer Res. 27, 1174–1183. doi:10.1158/1078-0432.CCR-20-3286
Akdeniz, B. C., Mattingsdal, M., Dominguez-Valentin, M., Frei, O., Shadrin, A., Puustusmaa, M., et al. (2023). A breast cancer polygenic risk score is feasible for risk stratification in the Norwegian population. Cancers 15, 4124. doi:10.3390/cancers15164124
Akhavan, D., Pourzia, A. L., Nourian, A. A., Williams, K. J., Nathanson, D., Babic, I., et al. (2013). De-repression of PDGFRβ transcription promotes acquired resistance to EGFR tyrosine kinase inhibitors in glioblastoma patients. Cancer Discov. 3, 534–547. doi:10.1158/2159-8290.CD-12-0502
Ali, R., Balamurali, M., and Varamini, P. (2022). Deep learning-based artificial intelligence to investigate targeted nanoparticles’ uptake in TNBC cells. IJMS 23, 16070. doi:10.3390/ijms232416070
Altelaar, A. F. M., Munoz, J., and Heck, A. J. R. (2013). Next-generation proteomics: towards an integrative view of proteome dynamics. Nat. Rev. Genet. 14, 35–48. doi:10.1038/nrg3356
Altini, N., Puro, E., Taccogna, M. G., Marino, F., De Summa, S., Saponaro, C., et al. (2023). Tumor cellularity assessment of breast histopathological slides via instance segmentation and pathomic features explainability. Bioengineering 10, 396. doi:10.3390/bioengineering10040396
Arbitrio, M., Scionti, F., Di Martino, M. T., Caracciolo, D., Pensabene, L., Tassone, P., et al. (2021). Pharmacogenomics biomarker discovery and validation for translation in clinical practice. Clin. Transl. Sci. 14, 113–119. doi:10.1111/cts.12869
Auwerx, C., Sadler, M. C., Reymond, A., and Kutalik, Z. (2022). From pharmacogenetics to pharmaco-omics: milestones and future directions. Hum. Genet. Genomics Adv. 3, 100100. doi:10.1016/j.xhgg.2022.100100
Baas, J., Krens, L., Bohringer, S., Mol, L., Punt, C., Guchelaar, H.-J., et al. (2018). Genome wide association study to identify predictors for severe skin toxicity in colorectal cancer patients treated with cetuximab. PLoS ONE 13, e0208080. doi:10.1371/journal.pone.0208080
Bachet, J.-B., Laurent-Puig, P., Meurisse, A., Bouché, O., Mas, L., Taly, V., et al. (2023). Circulating tumour DNA at baseline for individualised prognostication in patients with chemotherapy-naïve metastatic colorectal cancer. An AGEO prospective study. Eur. J. Cancer 189, 112934. doi:10.1016/j.ejca.2023.05.022
Baker, E. K., Johnstone, R. W., Zalcberg, J. R., and El-Osta, A. (2005). Epigenetic changes to the MDR1 locus in response to chemotherapeutic drugs. Oncogene 24, 8061–8075. doi:10.1038/sj.onc.1208955
Balasubramaniam, S., Redon, C. E., Peer, C. J., Bryla, C., Lee, M.-J., Trepel, J. B., et al. (2018). Phase I trial of belinostat with cisplatin and etoposide in advanced solid tumors, with a focus on neuroendocrine and small cell cancers of the lung. Anti-Cancer Drugs 29, 457–465. doi:10.1097/CAD.0000000000000596
Barnell, E. K., Newcomer, K. F., Skidmore, Z. L., Krysiak, K., Anderson, S. R., Wartman, L. D., et al. (2021). Impact of a 40-gene targeted panel test on physician decision making for patients with acute myeloid leukemia. JCO Precis. Oncol. 5, 191–203. doi:10.1200/PO.20.00182
Battaglin, F., Puccini, A., Naseem, M., Schirripa, M., Berger, M. D., Tokunaga, R., et al. (2018). Pharmacogenomics in colorectal cancer: current role in clinical practice and future perspectives. JCMT 4, 12. doi:10.20517/2394-4722.2018.04
Bedon, L., Cecchin, E., Fabbiani, E., Dal Bo, M., Buonadonna, A., Polano, M., et al. (2022). Machine learning application in a phase I clinical trial allows for the identification of clinical-biomolecular markers significantly associated with toxicity. Clin. Pharmacol. Ther. 111, 686–696. doi:10.1002/cpt.2511
Bei, J.-X., Li, Y., Jia, W.-H., Feng, B.-J., Zhou, G., Chen, L.-Z., et al. (2010). A genome-wide association study of nasopharyngeal carcinoma identifies three new susceptibility loci. Nat. Genet. 42, 599–603. doi:10.1038/ng.601
Belkadi, A., Bolze, A., Itan, Y., Cobat, A., Vincent, Q. B., Antipenko, A., et al. (2015). Whole-genome sequencing is more powerful than whole-exome sequencing for detecting exome variants. Proc. Natl. Acad. Sci. U.S.A. 112, 5473–5478. doi:10.1073/pnas.1418631112
Bewicke-Copley, F., Arjun Kumar, E., Palladino, G., Korfi, K., and Wang, J. (2019). Applications and analysis of targeted genomic sequencing in cancer studies. Comput. Struct. Biotechnol. J. 17, 1348–1359. doi:10.1016/j.csbj.2019.10.004
Bhojwani, D., Sabin, N. D., Pei, D., Yang, J. J., Khan, R. B., Panetta, J. C., et al. (2014). Methotrexate-induced neurotoxicity and leukoencephalopathy in childhood acute lymphoblastic leukemia. JCO 32, 949–959. doi:10.1200/JCO.2013.53.0808
Binder, D. C., Ladomersky, E., Lenzen, A., Zhai, L., Lauing, K. L., Otto-Meyer, S. D., et al. (2018). Lessons learned from rindopepimut treatment in patients with EGFRvIII-expressing glioblastoma. Transl. Cancer Res. 7, S510–S513. doi:10.21037/tcr.2018.03.36
Blumenschein, G. R., Kies, M. S., Papadimitrakopoulou, V. A., Lu, C., Kumar, A. J., Ricker, J. L., et al. (2008). Phase II trial of the histone deacetylase inhibitor vorinostat (ZolinzaTM, suberoylanilide hydroxamic acid, SAHA) in patients with recurrent and/or metastatic head and neck cancer. Invest. New Drugs 26, 81–87. doi:10.1007/s10637-007-9075-2
Bode, A. M., and Dong, Z. (2017). Precision oncology-the future of personalized cancer medicine? npj Precis. Onc 1 (2), 2. doi:10.1038/s41698-017-0010-5
Boyd, S. (2010). Distributed optimization and statistical learning via the alternating direction method of multipliers. FNT Mach. Learn. 3, 1–122. doi:10.1561/2200000016
Bozic, I., Antal, T., Ohtsuki, H., Carter, H., Kim, D., Chen, S., et al. (2010). Accumulation of driver and passenger mutations during tumor progression. Proc. Natl. Acad. Sci. U.S.A. 107, 18545–18550. doi:10.1073/pnas.1010978107
Brennan, C. W., Verhaak, R. G. W., McKenna, A., Campos, B., Noushmehr, H., Salama, S. R., et al. (2013). The somatic genomic landscape of glioblastoma. Cell 155, 462–477. doi:10.1016/j.cell.2013.09.034
Brindha, G. R., Rishiikeshwer, B. S., Santhi, B., Nakendraprasath, K., Manikandan, R., and Gandomi, A. H. (2022). Precise prediction of multiple anticancer drug efficacy using multi target regression and support vector regression analysis. Comput. Methods Programs Biomed. 224, 107027. doi:10.1016/j.cmpb.2022.107027
Bukowski, K., Kciuk, M., and Kontek, R. (2020). Mechanisms of multidrug resistance in cancer chemotherapy. IJMS 21, 3233. doi:10.3390/ijms21093233
Bychkov, D., Linder, N., Tiulpin, A., Kücükel, H., Lundin, M., Nordling, S., et al. (2021). Deep learning identifies morphological features in breast cancer predictive of cancer ERBB2 status and trastuzumab treatment efficacy. Sci. Rep. 11, 4037. doi:10.1038/s41598-021-83102-6
Cao, Y., Kunaprayoon, D., and Ren, L. (2023). Interpretable AI-assisted Clinical Decision Making (CDM) for dose prescription in radiosurgery of brain metastases. Radiotherapy Oncol. 187, 109842. doi:10.1016/j.radonc.2023.109842
Carrillo-Perez, F., Morales, J. C., Castillo-Secilla, D., Gevaert, O., Rojas, I., and Herrera, L. J. (2022). Machine-learning-based late fusion on multi-omics and multi-scale data for non-small-cell lung cancer diagnosis. JPM 12, 601. doi:10.3390/jpm12040601
Caudle, K. E., Thorn, C. F., Klein, T. E., Swen, J. J., McLeod, H. L., Diasio, R. B., et al. (2013). Clinical pharmacogenetics implementation Consortium guidelines for dihydropyrimidine dehydrogenase genotype and fluoropyrimidine dosing. Clin. Pharmacol. Ther. 94, 640–645. doi:10.1038/clpt.2013.172
Cauvin, C., Bourguignon, L., Carriat, L., Mence, A., Ghipponi, P., Salas, S., et al. (2022). Machine-learning exploration of exposure-effect relationships of cisplatin in head and neck cancer patients. Pharmaceutics 14, 2509. doi:10.3390/pharmaceutics14112509
Chan, H. T., Chin, Y. M., and Low, S.-K. (2019). The roles of common variation and somatic mutation in cancer pharmacogenomics. Oncol. Ther. 7, 1–32. doi:10.1007/s40487-018-0090-6
Chang, Y., Park, H., Yang, H.-J., Lee, S., Lee, K.-Y., Kim, T. S., et al. (2018). Cancer drug response profile scan (CDRscan): a deep learning model that predicts drug effectiveness from cancer genomic signature. Sci. Rep. 8, 8857. doi:10.1038/s41598-018-27214-6
Chen, D., Liu, J., Zang, L., Xiao, T., Zhang, X., Li, Z., et al. (2022). Integrated machine learning and bioinformatic analyses constructed a novel stemness-related classifier to predict prognosis and immunotherapy responses for hepatocellular carcinoma patients. Int. J. Biol. Sci. 18, 360–373. doi:10.7150/ijbs.66913
Chen, T.-Y., You, L., Hardillo, J. A. U., and Chien, M.-P. (2023). Spatial transcriptomic technologies. Cells 12, 2042. doi:10.3390/cells12162042
Chen, X., Qian, X., Xiao, M., and Zhang, P. (2023). Survival outcomes and efficacy of platinum in early breast cancer patients with germline BRCA1 or BRCA2 mutation: a multicenter retrospective cohort study. BCTT 15, 671–682. doi:10.2147/BCTT.S423330
Cheng, M., Lin, R., Bai, N., Zhang, Y., Wang, H., Guo, M., et al. (2023). Deep learning for predicting the risk of immune checkpoint inhibitor-related pneumonitis in lung cancer. Clin. Radiol. 78, e377–e385. doi:10.1016/j.crad.2022.12.013
Cui, Y., Li, Z., Xiang, M., Han, D., Yin, Y., and Ma, C. (2022a). Machine learning models predict overall survival and progression free survival of non-surgical esophageal cancer patients with chemoradiotherapy based on CT image radiomics signatures. Radiat. Oncol. 17, 212. doi:10.1186/s13014-022-02186-0
Cui, Y., Wang, Q., Shi, X., Ye, Q., Lei, M., and Wang, B. (2022b). Development of a web-based calculator to predict three-month mortality among patients with bone metastases from cancer of unknown primary: an internally and externally validated study using machine-learning techniques. Front. Oncol. 12, 1095059. doi:10.3389/fonc.2022.1095059
Cuttano, R., Colangelo, T., Guarize, J., Dama, E., Cocomazzi, M. P., Mazzarelli, F., et al. (2022). miRNome profiling of lung cancer metastases revealed a key role for miRNA-PD-L1 axis in the modulation of chemotherapy response. J. Hematol. Oncol. 15, 178. doi:10.1186/s13045-022-01394-1
Dear, R. F., McGeechan, K., Jenkins, M. C., Barratt, A., Tattersall, M. H., and Wilcken, N. (2013). Combination versus sequential single agent chemotherapy for metastatic breast cancer. Cochrane Database Syst. Rev. 2013, CD008792. doi:10.1002/14651858.CD008792.pub2
Demetri, G. D., von Mehren, M., Blanke, C. D., Van den Abbeele, A. D., Eisenberg, B., Roberts, P. J., et al. (2002). Efficacy and safety of imatinib mesylate in advanced gastrointestinal stromal tumors. N. Engl. J. Med. 347, 472–480. doi:10.1056/NEJMoa020461
Deng, Q., Chen, Y., Lin, L., Lin, J., Wang, H., Qiu, Y., et al. (2023). Exosomal hsa_circRNA_047733 integrated with clinical features for preoperative prediction of lymph node metastasis risk in oral squamous cell carcinoma. J. Oral Pathol. Med. 52, 37–46. doi:10.1111/jop.13379
De With, M., Van Doorn, L., Kloet, E., Van Veggel, A., Matic, M., De Neijs, M. J., et al. (2023). Irinotecan-induced toxicity: a pharmacogenetic study beyond UGT1A1. Clin. Pharmacokinet. 62, 1589–1597. doi:10.1007/s40262-023-01279-7
Di Martino, M. T., Arbitrio, M., Leone, E., Guzzi, P. H., Saveria Rotundo, M., Ciliberto, D., et al. (2011). Single nucleotide polymorphisms of ABCC5 and ABCG1 transporter genes correlate to irinotecan-associated gastrointestinal toxicity in colorectal cancer patients: a DMET microarray profiling study. Cancer Biol. Ther. 12, 780–787. doi:10.4161/cbt.12.9.17781
DiMasi, J. A., Grabowski, H. G., and Hansen, R. W. (2016). Innovation in the pharmaceutical industry: new estimates of R&D costs. J. Health Econ. 47, 20–33. doi:10.1016/j.jhealeco.2016.01.012
Doña, I., Barrionuevo, E., Blanca-Lopez, N., Torres, M. J., Fernandez, T. D., Mayorga, C., et al. (2014). Trends in hypersensitivity drug reactions: more drugs, more response patterns, more heterogeneity. J. Investig. Allergol. Clin. Immunol. 24, 143–153. quiz 1 p following 153.
Dou, B., Zhu, Z., Merkurjev, E., Ke, L., Chen, L., Jiang, J., et al. (2023). Machine learning methods for small data challenges in molecular science. Chem. Rev. 123, 8736–8780. doi:10.1021/acs.chemrev.3c00189
Douglass, E. F., Allaway, R. J., Szalai, B., Wang, W., Tian, T., Fernández-Torras, A., et al. (2022). A community challenge for a pancancer drug mechanism of action inference from perturbational profile data. Cell Rep. Med. 3, 100492. doi:10.1016/j.xcrm.2021.100492
Drilon, A., Laetsch, T. W., Kummar, S., DuBois, S. G., Lassen, U. N., Demetri, G. D., et al. (2018). Efficacy of larotrectinib in TRK fusion–positive cancers in adults and children. N. Engl. J. Med. 378, 731–739. doi:10.1056/NEJMoa1714448
Druker, B. J., Sawyers, C. L., Kantarjian, H., Resta, D. J., Reese, S. F., Ford, J. M., et al. (2001). Activity of a specific inhibitor of the BCR-ABL tyrosine kinase in the blast crisis of chronic myeloid leukemia and acute lymphoblastic leukemia with the Philadelphia chromosome. N. Engl. J. Med. 344, 1038–1042. doi:10.1056/NEJM200104053441402
Druker, B. J., Tamura, S., Buchdunger, E., Ohno, S., Segal, G. M., Fanning, S., et al. (1996). Effects of a selective inhibitor of the Abl tyrosine kinase on the growth of Bcr–Abl positive cells. Nat. Med. 2, 561–566. doi:10.1038/nm0596-561
Drusian, L., Nigro, E. A., Mannella, V., Pagliarini, R., Pema, M., Costa, A. S. H., et al. (2018). mTORC1 upregulation leads to accumulation of the oncometabolite fumarate in a mouse model of renal cell carcinoma. Cell Rep. 24, 1093–1104. doi:10.1016/j.celrep.2018.06.106
Dytfeld, D., Luczak, M., Wrobel, T., Usnarska-Zubkiewicz, L., Brzezniakiewicz, K., Jamroziak, K., et al. (2016). Comparative proteomic profiling of refractory/relapsed multiple myeloma reveals biomarkers involved in resistance to bortezomib-based therapy. Oncotarget 7, 56726–56736. doi:10.18632/oncotarget.11059
Eckardt, J.-N., Bornhäuser, M., Wendt, K., and Middeke, J. M. (2022). Semi-supervised learning in cancer diagnostics. Front. Oncol. 12, 960984. doi:10.3389/fonc.2022.960984
Eckardt, J.-N., Röllig, C., Metzeler, K., Heisig, P., Stasik, S., Georgi, J.-A., et al. (2023). Unsupervised meta-clustering identifies risk clusters in acute myeloid leukemia based on clinical and genetic profiles. Commun. Med. 3, 68. doi:10.1038/s43856-023-00298-6
Eckardt, J.-N., Wendt, K., Bornhäuser, M., and Middeke, J. M. (2021). Reinforcement learning for precision oncology. Cancers 13, 4624. doi:10.3390/cancers13184624
Ellis, M. J., Gillette, M., Carr, S. A., Paulovich, A. G., Smith, R. D., Rodland, K. K., et al. (2013). Connecting genomic alterations to cancer biology with proteomics: the NCI clinical proteomic tumor analysis Consortium. Cancer Discov. 3, 1108–1112. doi:10.1158/2159-8290.CD-13-0219
Elsheikh, S. E., Green, A. R., Rakha, E. A., Powe, D. G., Ahmed, R. A., Collins, H. M., et al. (2009). Global histone modifications in breast cancer correlate with tumor phenotypes, prognostic factors, and patient outcome. Cancer Res. 69, 3802–3809. doi:10.1158/0008-5472.CAN-08-3907
Erfanian, N., Heydari, A. A., Feriz, A. M., Iañez, P., Derakhshani, A., Ghasemigol, M., et al. (2023). Deep learning applications in single-cell genomics and transcriptomics data analysis. Biomed. Pharmacother. 165, 115077. doi:10.1016/j.biopha.2023.115077
Erickson, B. J., Korfiatis, P., Akkus, Z., and Kline, T. L. (2017). Machine learning for medical imaging. RadioGraphics 37, 505–515. doi:10.1148/rg.2017160130
Fazi, B., Felsani, A., Grassi, L., Moles, A., D’Andrea, D., Toschi, N., et al. (2015). The transcriptome and miRNome profiling of glioblastoma tissues and peritumoral regions highlights molecular pathways shared by tumors and surrounding areas and reveals differences between short-term and long-term survivors. Oncotarget 6, 22526–22552. doi:10.18632/oncotarget.4151
Fogel, D. B. (2018). Factors associated with clinical trials that fail and opportunities for improving the likelihood of success: a review. Contemp. Clin. Trials Commun. 11, 156–164. doi:10.1016/j.conctc.2018.08.001
Galanis, E., Jaeckle, K. A., Maurer, M. J., Reid, J. M., Ames, M. M., Hardwick, J. S., et al. (2009). Phase II trial of vorinostat in recurrent glioblastoma multiforme: a north central cancer treatment group study. JCO 27, 2052–2058. doi:10.1200/JCO.2008.19.0694
Garon, E. B., Reck, M., Nishio, K., Heymach, J. V., Nishio, M., Novello, S., et al. (2023). Ramucirumab plus erlotinib versus placebo plus erlotinib in previously untreated EGFR-mutated metastatic non-small-cell lung cancer (RELAY): exploratory analysis of next-generation sequencing results. ESMO Open 8, 101580. doi:10.1016/j.esmoop.2023.101580
Gayvert, K. M., Aly, O., Platt, J., Bosenberg, M. W., Stern, D. F., and Elemento, O. (2017). A computational approach for identifying synergistic drug combinations. PLoS Comput. Biol. 13, e1005308. doi:10.1371/journal.pcbi.1005308
Ge, C., Gu, I.Y.-H., Jakola, A. S., and Yang, J. (2020). Deep semi-supervised learning for brain tumor classification. BMC Med. Imaging 20, 87. doi:10.1186/s12880-020-00485-0
Glewis, S., Lingaratnam, S., Krishnasamy, M., H Martin, J., Tie, J., Alexander, M., et al. (2023). Pharmacogenetics testing (DPYD and UGT1A1) for fluoropyrimidine and irinotecan in routine clinical care: perspectives of medical oncologists and oncology pharmacists. J. Oncol. Pharm. Pract. 2023, 107815522311675. doi:10.1177/10781552231167554
Goey, A. K. L., Sissung, T. M., Peer, C. J., Trepel, J. B., Lee, M., Tomita, Y., et al. (2016). Effects of UGT1A1 genotype on the pharmacokinetics, pharmacodynamics, and toxicities of belinostat administered by 48-hour continuous infusion in patients with cancer. J. Clin. Pharma 56, 461–473. doi:10.1002/jcph.625
Guengerich, F. P. (2011). Mechanisms of drug toxicity and relevance to pharmaceutical development. Drug Metabolism Pharmacokinet. 26, 3–14. doi:10.2133/dmpk.DMPK-10-RV-062
Guo, L., Wang, W., Xie, X., Wang, S., and Zhang, Y. (2023). Machine learning for genetic prediction of chemotherapy toxicity in cervical cancer. Biomed. Pharmacother. 161, 114518. doi:10.1016/j.biopha.2023.114518
Halawani, R., Buchert, M., and Chen, Y.-P. P. (2023). Deep learning exploration of single-cell and spatially resolved cancer transcriptomics to unravel tumour heterogeneity. Comput. Biol. Med. 164, 107274. doi:10.1016/j.compbiomed.2023.107274
Handelman, G. S., Kok, H. K., Chandra, R. V., Razavi, A. H., Lee, M. J., and Asadi, H. (2018). eDoctor: machine learning and the future of medicine. J. Intern Med. 284, 603–619. doi:10.1111/joim.12822
Harrer, S., Shah, P., Antony, B., and Hu, J. (2019). Artificial intelligence for clinical trial design. Trends Pharmacol. Sci. 40, 577–591. doi:10.1016/j.tips.2019.05.005
Harrison, R. K. (2016). Phase II and phase III failures: 2013–2015. Nat. Rev. Drug Discov. 15, 817–818. doi:10.1038/nrd.2016.184
Heo, Y. J., Hwa, C., Lee, G.-H., Park, J.-M., and An, J.-Y. (2021). Integrative multi-omics approaches in cancer research: from biological networks to clinical subtypes. Mol. Cells 44, 433–443. doi:10.14348/molcells.2021.0042
Hertz, D. L., Arwood, M. J., Stocco, G., Singh, S., Karnes, J. H., and Ramsey, L. B. (2021). Planning and conducting a pharmacogenetics association study. Clin Pharma Ther. 110, 688–701. doi:10.1002/cpt.2270
Hoffmann, F., Franzen, A., De Vos, L., Wuest, L., Kulcsár, Z., Fietz, S., et al. (2023). CTLA4 DNA methylation is associated with CTLA-4 expression and predicts response to immunotherapy in head and neck squamous cell carcinoma. Clin. Epigenet 15, 112. doi:10.1186/s13148-023-01525-6
Hörst, F., Ting, S., Liffers, S.-T., Pomykala, K. L., Steiger, K., Albertsmeier, M., et al. (2023). Histology-based prediction of therapy response to neoadjuvant chemotherapy for esophageal and esophagogastric junction adenocarcinomas using deep learning. JCO Clin. Cancer Inf. 7, e2300038. doi:10.1200/CCI.23.00038
Hou, Y. C., Neidich, J. A., Duncavage, E. J., Spencer, D. H., and Schroeder, M. C. (2022). Clinical whole-genome sequencing in cancer diagnosis. Hum. Mutat. 43, 1519–1530. doi:10.1002/humu.24381
Huang, C., Mezencev, R., McDonald, J. F., and Vannberg, F. (2017). Open source machine-learning algorithms for the prediction of optimal cancer drug therapies. PLoS ONE 12, e0186906. doi:10.1371/journal.pone.0186906
Huang, X., Yan, J., Zhang, M., Wang, Y., Chen, Y., Fu, X., et al. (2018). Targeting epigenetic crosstalk as a therapeutic strategy for EZH2-aberrant solid tumors. Cell 175, 186–199. doi:10.1016/j.cell.2018.08.058
Hussen, B. M., Abdullah, S. T., Salihi, A., Sabir, D. K., Sidiq, K. R., Rasul, M. F., et al. (2022). The emerging roles of NGS in clinical oncology and personalized medicine. Pathology - Res. Pract. 230, 153760. doi:10.1016/j.prp.2022.153760
Hwang, T. J., Carpenter, D., Lauffenburger, J. C., Wang, B., Franklin, J. M., and Kesselheim, A. S. (2016). Failure of investigational drugs in late-stage clinical development and publication of trial results. JAMA Intern Med. 176, 1826–1833. doi:10.1001/jamainternmed.2016.6008
Ideker, T., Thorsson, V., Ranish, J. A., Christmas, R., Buhler, J., Eng, J. K., et al. (2001). Integrated genomic and proteomic analyses of a systematically perturbed metabolic network. Science 292, 929–934. doi:10.1126/science.292.5518.929
Innocenti, F., Undevia, S. D., Iyer, L., Xian Chen, P., Das, S., Kocherginsky, M., et al. (2004). Genetic variants in the UDP-glucuronosyltransferase 1A1 gene predict the risk of severe neutropenia of irinotecan. JCO 22, 1382–1388. doi:10.1200/JCO.2004.07.173
Ismail, S., and Essawi, M. (2012). Genetic polymorphism studies in humans: Middle East. J. Med. Genet. 1, 57–63. doi:10.1097/01.MXE.0000415225.85003.47
Iyer, L., Das, S., Janisch, L., Wen, M., Ramírez, J., Karrison, T., et al. (2002). UGT1A1*28 polymorphism as a determinant of irinotecan disposition and toxicity. Pharmacogenomics J. 2, 43–47. doi:10.1038/sj.tpj.6500072
Jalloul, R., Chethan, H. K., and Alkhatib, R. (2023). A review of machine learning techniques for the classification and detection of breast cancer from medical images. Diagnostics 13, 2460. doi:10.3390/diagnostics13142460
James, G., Witten, D., Hastie, T., and Tibshirani, R. (2013). An introduction to statistical learning: with applications in R (New York: Springer). Springer texts in statistics.
Jiang, C., Yang, W., Moriyama, T., Liu, C., Smith, C., Yang, W., et al. (2021). Effects of NT5C2 germline variants on 6-mecaptopurine metabolism in children with acute lymphoblastic leukemia. Clin. Pharmacol. Ther. 109, 1538–1545. doi:10.1002/cpt.2095
Jiang, L., Jiang, C., Yu, X., Fu, R., Jin, S., and Liu, X. (2022). DeepTTA: a transformer-based model for predicting cancer drug response. Briefings Bioinforma. 23, bbac100. doi:10.1093/bib/bbac100
Jing, Y., Liu, J., Ye, Y., Pan, L., Deng, H., Wang, Y., et al. (2020). Multi-omics prediction of immune-related adverse events during checkpoint immunotherapy. Nat. Commun. 11, 4946. doi:10.1038/s41467-020-18742-9
Jun, H. J., Acquaviva, J., Chi, D., Lessard, J., Zhu, H., Woolfenden, S., et al. (2012). Acquired MET expression confers resistance to EGFR inhibition in a mouse model of glioblastoma multiforme. Oncogene 31, 3039–3050. doi:10.1038/onc.2011.474
Kang, J., Chowdhry, A. K., Pugh, S. L., and Park, J. H. (2023). Integrating artificial intelligence and machine learning into cancer clinical trials. Seminars Radiat. Oncol. 33, 386–394. doi:10.1016/j.semradonc.2023.06.004
Kim, H., Lee, S. J., Lee, I. K., Min, S. C., Sung, H. H., Jeong, B. C., et al. (2020). Synergistic effects of combination therapy with AKT and mTOR inhibitors on bladder cancer cells. IJMS 21, 2825. doi:10.3390/ijms21082825
Kim, Y., Armstrong, T. S., Gilbert, M. R., and Celiku, O. (2023). A critical analysis of neuro-oncology clinical trials. Neuro Oncol. 25, 1658–1671. doi:10.1093/neuonc/noad036
Kimchi-Sarfaty, C., Oh, J. M., Kim, I.-W., Sauna, Z. E., Calcagno, A. M., Ambudkar, S. V., et al. (2007). A “silent” polymorphism in the MDR 1 gene changes substrate specificity. Science 315, 525–528. doi:10.1126/science.1135308
Kindler, C., Elfwing, S., Öhrvik, J., and Nikberg, M. (2023). A deep neural network–based decision support tool for the detection of lymph node metastases in colorectal cancer specimens. Mod. Pathol. 36, 100015. doi:10.1016/j.modpat.2022.100015
Kline, A., Wang, H., Li, Y., Dennis, S., Hutch, M., Xu, Z., et al. (2022). Multimodal machine learning in precision health: a scoping review. npj Digit. Med. 5, 171. doi:10.1038/s41746-022-00712-8
Knezevic, C. E., and Clarke, W. (2020). Cancer chemotherapy: the case for therapeutic drug monitoring. Ther. Drug Monit. 42, 6–19. doi:10.1097/FTD.0000000000000701
Kondo, Y., and Issa, J.-P. J. (2004). Epigenetic changes in colorectal cancer. Cancer Metastasis Rev. 23, 29–39. doi:10.1023/A:1025806911782
Körber, N. (2023). MIA is an open-source standalone deep learning application for microscopic image analysis. Cell Rep. Methods 3, 100517. doi:10.1016/j.crmeth.2023.100517
Koteluk, O., Wartecki, A., Mazurek, S., Kołodziejczak, I., and Mackiewicz, A. (2021). How do machines learn? Artificial intelligence as a new era in medicine. JPM 11, 32. doi:10.3390/jpm11010032
Kourou, K., Exarchos, T. P., Exarchos, K. P., Karamouzis, M. V., and Fotiadis, D. I. (2015). Machine learning applications in cancer prognosis and prediction. Comput. Struct. Biotechnol. J. 13, 8–17. doi:10.1016/j.csbj.2014.11.005
Kouzarides, T. (2007). Chromatin modifications and their function. Cell 128, 693–705. doi:10.1016/j.cell.2007.02.005
Kriegeskorte, N., and Golan, T. (2019). Neural network models and deep learning. Curr. Biol. 29, R231–R236. doi:10.1016/j.cub.2019.02.034
Kwok, W. C., Lam, D. C. L., Ip, M. S. M., Tam, T. C. C., and Ho, J. C. M. (2022). Association of genetic polymorphisms of CYP3A4 and CYP2D6 with gefitinib-induced toxicities. Anti-Cancer Drugs 33, 1139–1144. doi:10.1097/CAD.0000000000001360
Kwon, M.-S., Kim, Y., Lee, S., Namkung, J., Yun, T., Yi, S. G., et al. (2015). Integrative analysis of multi-omics data for identifying multi-markers for diagnosing pancreatic cancer. BMC Genomics 16, S4. doi:10.1186/1471-2164-16-S9-S4
Lakhotia, S. C., Mallick, B., and Roy, J. (2020). Non-coding RNAs: ever-expanding diversity of types and functions. Rna-Based Regul. Hum. Health Dis. 19, 5–57. doi:10.1016/B978-0-12-817193-6.00002-9
Lambin, P., Rios-Velazquez, E., Leijenaar, R., Carvalho, S., Van Stiphout, R. G. P. M., Granton, P., et al. (2012). Radiomics: extracting more information from medical images using advanced feature analysis. Eur. J. Cancer 48, 441–446. doi:10.1016/j.ejca.2011.11.036
Lauschke, V. M., Milani, L., and Ingelman-Sundberg, M. (2018). Pharmacogenomic biomarkers for improved drug therapy—recent progress and future developments. AAPS J. 20, 4. doi:10.1208/s12248-017-0161-x
LeCun, Y., Bengio, Y., and Hinton, G. (2015). Deep learning. Nature 521, 436–444. doi:10.1038/nature14539
Lee, J. O., Kang, M. J., Byun, W. S., Kim, S. A., Seo, I. H., Han, J.Ah., et al. (2019). Metformin overcomes resistance to cisplatin in triple-negative breast cancer (TNBC) cells by targeting RAD51. Breast Cancer Res. 21, 115. doi:10.1186/s13058-019-1204-2
Lenahan, A. L., Squire, A. E., and Miller, D. E. (2023). Panels, exomes, genomes, and more—finding the best path through the diagnostic odyssey. Pediatr. Clin. N. Am. 70, 905–916. doi:10.1016/j.pcl.2023.06.001
Lešnjaković, L., Ganoci, L., Bilić, I., Šimičević, L., Mucalo, I., Pleština, S., et al. (2023). DPYD genotyping and predicting fluoropyrimidine toxicity: where do we stand? Pharmacogenomics 24, 93–106. doi:10.2217/pgs-2022-0135
Lheureux, S., Prokopec, S. D., Oldfield, L. E., Gonzalez-Ochoa, E., Bruce, J. P., Wong, D., et al. (2023). Identifying mechanisms of resistance by circulating tumor DNA in EVOLVE, a phase II trial of cediranib plus olaparib for ovarian cancer at time of PARP inhibitor progression. Clin. Cancer Res. 29, 3706–3716. doi:10.1158/1078-0432.CCR-23-0797
Li, M., Mei, S., Yang, Y., Shen, Y., and Chen, L. (2022). Strategies to mitigate the on- and off-target toxicities of recombinant immunotoxins: an antibody engineering perspective. Antib. Ther. 5, 164–176. doi:10.1093/abt/tbac014
Li, R., Tian, Y., Yang, Z., Ji, Y., Ding, J., and Yan, A. (2023). Classification models and SAR analysis on HDAC1 inhibitors using machine learning methods. Mol. Divers 27, 1037–1051. doi:10.1007/s11030-022-10466-w
Li, X., and Wang, C.-Y. (2021). From bulk, single-cell to spatial RNA sequencing. Int. J. Oral Sci. 13, 36. doi:10.1038/s41368-021-00146-0
Lin, B., Ziebro, J., Smithberger, E., Skinner, K. R., Zhao, E., Cloughesy, T. F., et al. (2022). EGFR, the Lazarus target for precision oncology in glioblastoma. Neuro-Oncology 24, 2035–2062. doi:10.1093/neuonc/noac204
Lincoln, S. E., Hambuch, T., Zook, J. M., Bristow, S. L., Hatchell, K., Truty, R., et al. (2021). One in seven pathogenic variants can be challenging to detect by NGS: an analysis of 450,000 patients with implications for clinical sensitivity and genetic test implementation. Genet. Med. 23, 1673–1680. doi:10.1038/s41436-021-01187-w
Lionel, A. C., Costain, G., Monfared, N., Walker, S., Reuter, M. S., Hosseini, S. M., et al. (2018). Improved diagnostic yield compared with targeted gene sequencing panels suggests a role for whole-genome sequencing as a first-tier genetic test. Genet. Med. 20, 435–443. doi:10.1038/gim.2017.119
Li S, S., Yang, M., Ji, L., and Fan, H. (2022). A multi-omics machine learning framework in predicting the recurrence and metastasis of patients with pancreatic adenocarcinoma. Front. Microbiol. 13, 1032623. doi:10.3389/fmicb.2022.1032623
Liu, Y., Sun, J., Sun, H., and Chang, Y. (2022). Identification of key somatic oncogenic mutation based on a confounder-free causal inference model. PLoS Comput. Biol. 18, e1010529. doi:10.1371/journal.pcbi.1010529
Liu, Z., Lin, G., Yan, Z., Li, L., Wu, X., Shi, J., et al. (2022). Predictive mutation signature of immunotherapy benefits in NSCLC based on machine learning algorithms. Front. Immunol. 13, 989275. doi:10.3389/fimmu.2022.989275
Louis, D. N., Perry, A., Wesseling, P., Brat, D. J., Cree, I. A., Figarella-Branger, D., et al. (2021). The 2021 WHO classification of tumors of the central nervous system: a summary. Neuro-Oncology 23, 1231–1251. doi:10.1093/neuonc/noab106
Loukovaara, M., Pasanen, A., and Bützow, R. (2021). Mismatch repair protein and MLH1 methylation status as predictors of response to adjuvant therapy in endometrial cancer. Cancer Med. 10, 1034–1042. doi:10.1002/cam4.3691
Lowe, E. S., and Lertora, J. J. L. (2012). “Dose–effect and concentration–effect analysis,” in Principles of clinical pharmacology (Elsevier), 343–356. doi:10.1016/B978-0-12-385471-1.00020-9
Lv, B., Xu, R., Xing, X., Liao, C., Zhang, Z., Zhang, P., et al. (2022). Discovery of synergistic drug combinations for colorectal cancer driven by tumor barcode derived from metabolomics “big data.”. Metabolites 12, 494. doi:10.3390/metabo12060494
Lynch, T. J., Bell, D. W., Sordella, R., Gurubhagavatula, S., Okimoto, R. A., Brannigan, B. W., et al. (2004). Activating mutations in the epidermal growth factor receptor underlying responsiveness of non–small-cell lung cancer to gefitinib. N. Engl. J. Med. 350, 2129–2139. doi:10.1056/NEJMoa040938
Ma, T., Liu, Q., Li, H., Zhou, M., Jiang, R., and Zhang, X. (2022). DualGCN: a dual graph convolutional network model to predict cancer drug response. BMC Bioinforma. 23, 129. doi:10.1186/s12859-022-04664-4
Maeda, A., Ando, H., Irie, K., Hashimoto, N., Morishige, J.-I., Fukushima, S., et al. (2023). Effects of ABCB1 and ABCG2 polymorphisms on the pharmacokinetics of abemaciclib metabolites (M2, M20, M18). Anticancer Res. 43, 1283–1289. doi:10.21873/anticanres.16275
Malta, T. M., Sokolov, A., Gentles, A. J., Burzykowski, T., Poisson, L., Weinstein, J. N., et al. (2018). Machine learning identifies stemness features associated with oncogenic dedifferentiation. Cell 173, 338–354.e15. doi:10.1016/j.cell.2018.03.034
Mandelker, D., and Ceyhan-Birsoy, O. (2020). Evolving significance of tumor-normal sequencing in cancer care. Trends Cancer 6, 31–39. doi:10.1016/j.trecan.2019.11.006
Marcus, L., Fashoyin-Aje, L. A., Donoghue, M., Yuan, M., Rodriguez, L., Gallagher, P. S., et al. (2021). FDA approval summary: pembrolizumab for the treatment of tumor mutational burden–high solid tumors. Clin. Cancer Res. 27, 4685–4689. doi:10.1158/1078-0432.CCR-21-0327
Martin-Broto, J., Valverde, C., Hindi, N., Vincenzi, B., Martinez-Trufero, J., Grignani, G., et al. (2023). REGISTRI: regorafenib in first-line of KIT/PDGFRA wild type metastatic GIST: a collaborative Spanish (GEIS), Italian (ISG) and French Sarcoma Group (FSG) phase II trial. Mol. Cancer 22, 127. doi:10.1186/s12943-023-01832-9
Massella, M., Dri, D. A., and Gramaglia, D. (2022). Regulatory considerations on the use of machine learning based tools in clinical trials. Health Technol. 12, 1085–1096. doi:10.1007/s12553-022-00708-0
Massi, M. C., Gasperoni, F., Ieva, F., Paganoni, A. M., Zunino, P., Manzoni, A., et al. (2020). A deep learning approach validates genetic risk factors for late toxicity after prostate cancer radiotherapy in a REQUITE multi-national cohort. Front. Oncol. 10, 541281. doi:10.3389/fonc.2020.541281
Mathijssen, R. H. J., Marsh, S., Karlsson, M. O., Xie, R., Baker, S. D., Verweij, J., et al. (2003). Irinotecan pathway genotype analysis to predict pharmacokinetics. Clin. Cancer Res. 9, 3246–3253.
McGrail, D. J., Pilié, P. G., Rashid, N. U., Voorwerk, L., Slagter, M., Kok, M., et al. (2021). High tumor mutation burden fails to predict immune checkpoint blockade response across all cancer types. Ann. Oncol. 32, 661–672. doi:10.1016/j.annonc.2021.02.006
McLaren, D. B., and Aitman, T. J. (2023). Redefining precision radiotherapy through liquid biopsy. Br. J. Cancer 129, 900–903. doi:10.1038/s41416-023-02398-5
Meienberg, J., Bruggmann, R., Oexle, K., and Matyas, G. (2016). Clinical sequencing: is WGS the better WES? Hum. Genet. 135, 359–362. doi:10.1007/s00439-015-1631-9
Meißner, A.-K., Gutsche, R., Galldiks, N., Kocher, M., Jünger, S. T., Eich, M.-L., et al. (2022). Radiomics for the noninvasive prediction of the BRAF mutation status in patients with melanoma brain metastases. Neuro-Oncology 24, 1331–1340. doi:10.1093/neuonc/noab294
Menden, M. P., Iorio, F., Garnett, M., McDermott, U., Benes, C. H., Ballester, P. J., et al. (2013). Machine learning prediction of cancer cell sensitivity to drugs based on genomic and chemical properties. PLoS ONE 8, e61318. doi:10.1371/journal.pone.0061318
Meng, S., Li, M., Qin, L., Lv, J., Wu, D., Zheng, D., et al. (2023). The onco-embryonic antigen ROR1 is a target of chimeric antigen T cells for colorectal cancer. Int. Immunopharmacol. 121, 110402. doi:10.1016/j.intimp.2023.110402
Merino, D. M., McShane, L. M., Fabrizio, D., Funari, V., Chen, S.-J., White, J. R., et al. (2020). Establishing guidelines to harmonize tumor mutational burden (TMB): in silico assessment of variation in TMB quantification across diagnostic platforms: phase I of the Friends of Cancer Research TMB Harmonization Project. J. Immunother. Cancer 8, e000147. doi:10.1136/jitc-2019-000147
Miettunen, J., Nordström, T., Kaakinen, M., and Ahmed, A. O. (2016). Latent variable mixture modeling in psychiatric research – a review and application. Psychol. Med. 46, 457–467. doi:10.1017/S0033291715002305
Mitchell, T. M. (2013). “Machine learning, nachdr,” in McGraw-hill series in computer science (New York: McGraw-Hill).
Moon, I., LoPiccolo, J., Baca, S. C., Sholl, L. M., Kehl, K. L., Hassett, M. J., et al. (2023). Machine learning for genetics-based classification and treatment response prediction in cancer of unknown primary. Nat. Med. 29, 2057–2067. doi:10.1038/s41591-023-02482-6
Morel, A., Boisdron-Celle, M., Fey, L., Soulie, P., Craipeau, M. C., Traore, S., et al. (2006). Clinical relevance of different dihydropyrimidine dehydrogenase gene single nucleotide polymorphisms on 5-fluorouracil tolerance. Mol. Cancer Ther. 5, 2895–2904. doi:10.1158/1535-7163.MCT-06-0327
Moriyama, T., Nishii, R., Perez-Andreu, V., Yang, W., Klussmann, F. A., Zhao, X., et al. (2016). NUDT15 polymorphisms alter thiopurine metabolism and hematopoietic toxicity. Nat. Genet. 48, 367–373. doi:10.1038/ng.3508
Mu, W., Li, B., Wu, S., Chen, J., Sain, D., Xu, D., et al. (2019). Detection of structural variation using target captured next-generation sequencing data for genetic diagnostic testing. Genet. Med. 21, 1603–1610. doi:10.1038/s41436-018-0397-6
Naik, K., Goyal, R. K., Foschini, L., Chak, C. W., Thielscher, C., Zhu, H., et al. (2023). Current status and future directions: the application of artificial intelligence/machine learning (AI/ML) for precision medicine. Clin Pharma Ther. cpt 3152. doi:10.1002/cpt.3152
Nebert, D. W. (1999). Pharmacogenetics and pharmacogenomics: why is this relevant to the clinical geneticist? pharmacogenetics and pharmacogenomics. Clin. Genet. 56, 247–258. doi:10.1034/j.1399-0004.1999.560401.x
Nicolae, D. L., Gamazon, E., Zhang, W., Duan, S., Dolan, M. E., and Cox, N. J. (2010). Trait-associated SNPs are more likely to Be eQTLs: annotation to enhance discovery from GWAS. PLoS Genet. 6, e1000888. doi:10.1371/journal.pgen.1000888
Nindra, U., Pal, A., Bray, V., Yip, P. Y., Tognela, A., Roberts, T. L., et al. (2023). Utility of multigene panel next-generation sequencing in routine clinical practice for identifying genomic alterations in newly diagnosed metastatic nonsmall cell lung cancer. Intern. Med. J. imj 2023, 16224. doi:10.1111/imj.16224
Niraula, D., Jamaluddin, J., Matuszak, M. M., Haken, R. K. T., and Naqa, I. E. (2021). Quantum deep reinforcement learning for clinical decision support in oncology: application to adaptive radiotherapy. Sci. Rep. 11, 23545. doi:10.1038/s41598-021-02910-y
Noor, F., Asif, M., Ashfaq, U. A., Qasim, M., and Tahir Ul Qamar, M. (2023). Machine learning for synergistic network pharmacology: a comprehensive overview. Briefings Bioinforma. 24, bbad120. doi:10.1093/bib/bbad120
Nozawa, T., Minami, H., Sugiura, S., Tsuji, A., and Tamai, I. (2005). Role of organic anion transporter Oatp1b1 (oatp-C) in hepatic uptake of irinotecan and its active metabolite, 7-ethyl-10-hydroxycamptothecin: in vitro evidence and effect of single nucleotide polymorphisms. Drug Metab. Dispos. 33, 434–439. doi:10.1124/dmd.104.001909
On, J., Park, H.-A., and Yoo, S. (2022). Development of a prediction models for chemotherapy-induced adverse drug reactions: a retrospective observational study using electronic health records. Eur. J. Oncol. Nurs. 56, 102066. doi:10.1016/j.ejon.2021.102066
Organ isation mondiale de la santé, Centre international de recherche sur le cancer (2020). “Soft tissue and bone tumours,” in World health organization classification of tumours. 5th ed (Geneva: OMS).
Orozco-Arias, S., Piña, J. S., Tabares-Soto, R., Castillo-Ossa, L. F., Guyot, R., and Isaza, G. (2020). Measuring performance metrics of machine learning algorithms for detecting and classifying transposable elements. Transposable Elem. Process. 8, 638. doi:10.3390/pr8060638
Pacurari, A. C., Bhattarai, S., Muhammad, A., Avram, C., Mederle, A. O., Rosca, O., et al. (2023). Diagnostic accuracy of machine learning ai architectures in detection and classification of lung cancer: a systematic review. Diagnostics 13, 2145. doi:10.3390/diagnostics13132145
Park, A., Lee, Y., and Nam, S. (2023). A performance evaluation of drug response prediction models for individual drugs. Sci. Rep. 13, 11911. doi:10.1038/s41598-023-39179-2
Parsons, D. W., Janeway, K. A., Patton, D. R., Winter, C. L., Coffey, B., Williams, P. M., et al. (2022). Actionable tumor alterations and treatment protocol enrollment of pediatric and young adult patients with refractory cancers in the national cancer institute–children’s oncology group pediatric MATCH trial. JCO 40, 2224–2234. doi:10.1200/JCO.21.02838
Patellongi, I., Amiruddin, A., Massi, M. N., Islam, A. A., Pratama, M. Y., Sutandyo, N., et al. (2023). Circulating miR-221/222 expression as microRNA biomarker predicting tamoxifen treatment outcome: a case–control study. Ann. Med. Surg. 85, 3806–3815. doi:10.1097/MS9.0000000000001061
Patti, G. J., Yanes, O., and Siuzdak, G. (2012). Innovation: metabolomics: the apogee of the omics trilogy. Nat. Rev. Mol. Cell Biol. 13, 263–269. doi:10.1038/nrm3314
Peeters, S. L., Deenen, M. J., Thijs, A. M., Hulshof, E. C., Mathijssen, R. H., Gelderblom, H., et al. (2023). UGT1A1 genotype-guided dosing of irinotecan: time to prioritize patient safety. Pharmacogenomics 24, 435–439. doi:10.2217/pgs-2023-0096
Peng, D., Kryczek, I., Nagarsheth, N., Zhao, L., Wei, S., Wang, W., et al. (2015). Epigenetic silencing of TH1-type chemokines shapes tumour immunity and immunotherapy. Nature 527, 249–253. doi:10.1038/nature15520
Pixberg, C., Zapatka, M., Hlevnjak, M., Benedetto, S., Suppelna, J. P., Heil, J., et al. (2022). COGNITION: a prospective precision oncology trial for patients with early breast cancer at high risk following neoadjuvant chemotherapy. ESMO Open 7, 100637. doi:10.1016/j.esmoop.2022.100637
Podgorelec, V., Kokol, P., Stiglic, B., and Rozman, I. (2002). Decision trees: an overview and their use in medicine. J. Med. Syst. 26, 445–463. doi:10.1023/A:1016409317640
Polano, M., Bedon, L., Bo, M. D., Sorio, R., Bartoletti, M., De Mattia, E., et al. (2023). Machine learning application identifies germline markers of hypertension in ovarian cancer patients treated with carboplatin, taxane and bevacizumab. Clin. Pharmacol. Ther. 2023, 2960. doi:10.1002/cpt.2960
Pushpakom, S., Iorio, F., Eyers, P. A., Escott, K. J., Hopper, S., Wells, A., et al. (2019). Drug repurposing: progress, challenges and recommendations. Nat. Rev. Drug Discov. 18, 41–58. doi:10.1038/nrd.2018.168
Quintanilha, J. C. F., Geyer, S., Etheridge, A. S., Racioppi, A., Hammond, K., Crona, D. J., et al. (2022a). KDR genetic predictor of toxicities induced by sorafenib and regorafenib. Pharmacogenomics J. 22, 251–257. doi:10.1038/s41397-022-00279-3
Quintanilha, J. C. F., Wang, J., Sibley, A. B., Xu, W., Espin-Garcia, O., Jiang, C., et al. (2022b). Genome-wide association studies of survival in 1520 cancer patients treated with bevacizumab-containing regimens. Intl J. Cancer 150, 279–289. doi:10.1002/ijc.33810
Ramesh, P., Karuppasamy, R., and Veerappapillai, S. (2022). Machine learning driven drug repurposing strategy for identification of potential RET inhibitors against non-small cell lung cancer. Med. Oncol. 40, 56. doi:10.1007/s12032-022-01924-4
Relling, M. V., Schwab, M., Whirl-Carrillo, M., Suarez-Kurtz, G., Pui, C., Stein, C. M., et al. (2019). Clinical pharmacogenetics implementation Consortium guideline for thiopurine dosing based on TPMT and NUDT 15 genotypes: 2018 update. Clin Pharma Ther. 105, 1095–1105. doi:10.1002/cpt.1304
Robles, J., Pintado-Berninches, L., Boukich, I., Escudero, B., De Los Rios, V., Bartolomé, R. A., et al. (2022). A prognostic six-gene expression risk-score derived from proteomic profiling of the metastatic colorectal cancer secretome. J. Pathology CR 8, 495–508. doi:10.1002/cjp2.294
Roden, D. M., McLeod, H. L., Relling, M. V., Williams, M. S., Mensah, G. A., Peterson, J. F., et al. (2019). Pharmacogenomics. Lancet 394, 521–532. doi:10.1016/S0140-6736(19)31276-0
Rojahn, S., Hambuch, T., Adrian, J., Gafni, E., Gileta, A., Hatchell, H., et al. (2022). Scalable detection of technically challenging variants through modified next-generation sequencing. Molec Gen Gen Med 10, e2072. doi:10.1002/mgg3.2072
Romero, A., Martín, M., Oliva, B., De La Torre, J., Furio, V., De La Hoya, M., et al. (2012). Glutathione S-transferase P1 c.313A > G polymorphism could be useful in the prediction of doxorubicin response in breast cancer patients. Ann. Oncol. 23, 1750–1756. doi:10.1093/annonc/mdr483
Romero, J. M., Titmuss, E., Wang, Y., Vafiadis, J., Pacis, A., Jang, G. H., et al. (2023). Chemokine expression predicts T cell-inflammation and improved survival with checkpoint inhibition across solid cancers. npj Precis. Onc. 7, 73. doi:10.1038/s41698-023-00428-2
Roy, B., Stepišnik, T., Vens, C., Džeroski, S., and Džeroski, S. (2022). Survival analysis with semi-supervised predictive clustering trees. Comput. Biol. Med. 141, 105001. doi:10.1016/j.compbiomed.2021.105001
Rudmann, D. G. (2013). On-target and off-target-based toxicologic effects. Toxicol. Pathol. 41, 310–314. doi:10.1177/0192623312464311
Ryan, D. K., Maclean, R. H., Balston, A., Scourfield, A., Shah, A. D., and Ross, J. (2023). Artificial intelligence and machine learning for clinical pharmacology. Brit J. Clin. Pharma bcp 2023, 15930. doi:10.1111/bcp.15930
Sajda, P. (2006). Machine learning for detection and diagnosis of disease. Annu. Rev. Biomed. Eng. 8, 537–565. doi:10.1146/annurev.bioeng.8.061505.095802
Sbaraglia, M., Bellan, E., and Dei Tos, A. P. (2020). The 2020 WHO classification of soft tissue tumours: news and perspectives. Pathologica 113, 70–84. doi:10.32074/1591-951X-213
Schoot, V. V. D., Viellevoije, S. J., Tammer, F., Brunner, H. G., Arens, Y., Yntema, H. G., et al. (2021). The impact of unsolicited findings in clinical exome sequencing, a qualitative interview study. Eur. J. Hum. Genet. 29, 930–939. doi:10.1038/s41431-021-00834-9
Schroth, W., Antoniadou, L., Fritz, P., Schwab, M., Muerdter, T., Zanger, U. M., et al. (2007). Breast cancer treatment outcome with adjuvant tamoxifen relative to patient CYP2D6 and CYP2C19 genotypes. JCO 25, 5187–5193. doi:10.1200/JCO.2007.12.2705
Sengupta, D., Zeng, L., Li, Y., Hausmann, S., Ghosh, D., Yuan, G., et al. (2021). NSD2 dimethylation at H3K36 promotes lung adenocarcinoma pathogenesis. Mol. Cell 81, 4481–4492.e9. doi:10.1016/j.molcel.2021.08.034
Sepulveda, A. R., Hamilton, S. R., Allegra, C. J., Grody, W., Cushman-Vokoun, A. M., Funkhouser, W. K., et al. (2017). Molecular biomarkers for the evaluation of colorectal cancer: guideline from the American society for clinical pathology, college of American pathologists, association for molecular pathology, and American society of clinical oncology. J. Mol. Diagnostics 19, 187–225. doi:10.1016/j.jmoldx.2016.11.001
Shahin, M. H., Barth, A., Podichetty, J. T., Liu, Q., Goyal, N., Jin, J. Y., et al. (2023). Artificial intelligence: from buzzword to useful tool in clinical pharmacology. Clin Pharma Ther. cpt 2023, 3083. doi:10.1002/cpt.3083
Sharma, M., Krüger, R., and Gasser, T. (2014). From genome-wide association studies to next-generation sequencing: lessons from the past and planning for the future. JAMA Neurol. 71, 5–6. doi:10.1001/jamaneurol.2013.3682
Shen, Y., Kim, I., and Tang, Y. (2023). Uncovering the heterogeneity of cardiac lin –KIT + cells: a scRNA-seq study on the identification of subpopulations. Stem Cells 41, 958–970. doi:10.1093/stmcls/sxad057
Shi, M., Sheng, Z., and Tang, H. (2021). Prognostic outcome prediction by semi-supervised least squares classification. Briefings Bioinforma. 22, bbaa249. doi:10.1093/bib/bbaa249
Shrestha, R., Llaurado Fernandez, M., Dawson, A., Hoenisch, J., Volik, S., Lin, Y.-Y., et al. (2021). Multiomics characterization of low-grade serous ovarian carcinoma identifies potential biomarkers of MEK inhibitor sensitivity and therapeutic vulnerability. Cancer Res. 81, 1681–1694. doi:10.1158/0008-5472.CAN-20-2222
Sims, D., Sudbery, I., Ilott, N. E., Heger, A., and Ponting, C. P. (2014). Sequencing depth and coverage: key considerations in genomic analyses. Nat. Rev. Genet. 15, 121–132. doi:10.1038/nrg3642
Sra, S., Nowozin, S., and Wright, S. J. (2012). Optimization for machine learning, Neural information processing series. Cambridge: MIT press.
Steinberg, A. N., Bowman, C. L., and White, F. E. (1999). “Revisions to the JDL data fusion model,” in Presented at the AeroSense ’99. Editor B. V. Dasarathy (Orlando, FL: SPIE Digital Library), 430. doi:10.1117/12.341367
Stevanovski, I., Chintalaphani, S. R., Gamaarachchi, H., Ferguson, J. M., Pineda, S. S., Scriba, C. K., et al. (2022). Comprehensive genetic diagnosis of tandem repeat expansion disorders with programmable targeted nanopore sequencing. Sci. Adv. 8, eabm5386. doi:10.1126/sciadv.abm5386
Stockley, T. L., Lo, B., Box, A., Corredor, A. G., DeCoteau, J., Desmeules, P., et al. (2023). CANTRK: a Canadian ring study to optimize detection of NTRK gene fusions by next-generation RNA sequencing. J. Mol. Diagnostics 25, 168–174. doi:10.1016/j.jmoldx.2022.12.004
Sweeney, C., Ambrosone, C. B., Joseph, L., Stone, A., Hutchins, L. F., Kadlubar, F. F., et al. (2003). Association between a glutathioneS-transferase A1 promoter polymorphism and survival after breast cancer treatment. Int. J. Cancer 103, 810–814. doi:10.1002/ijc.10896
Swen, J. J., Van Der Wouden, C. H., Manson, L. E., Abdullah-Koolmees, H., Blagec, K., Blagus, T., et al. (2023). A 12-gene pharmacogenetic panel to prevent adverse drug reactions: an open-label, multicentre, controlled, cluster-randomised crossover implementation study. Lancet 401, 347–356. doi:10.1016/S0140-6736(22)01841-4
Tabl, A. A., Alkhateeb, A., ElMaraghy, W., Rueda, L., and Ngom, A. (2019). A machine learning approach for identifying gene biomarkers guiding the treatment of breast cancer. Front. Genet. 10, 256. doi:10.3389/fgene.2019.00256
Tan, D., Mohd Nasir, N. F., Abdul Manan, H., and Yahya, N. (2023). Prediction of toxicity outcomes following radiotherapy using deep learning-based models: a systematic review. Cancer/Radiothérapie 27, 398–406. doi:10.1016/j.canrad.2023.05.001
Tan, H. T., Lee, Y. H., and Chung, M. C. M. (2012). Cancer proteomics: CANCER PROTEOMICS. Mass Spectrom. Rev. 31, 583–605. doi:10.1002/mas.20356
Tao, L. R., Ye, Y., and Zhao, H. (2023). Early breast cancer risk detection: a novel framework leveraging polygenic risk scores and machine learning. J. Med. Genet. jmedgenet- 60, 960–964. doi:10.1136/jmg-2022-108582
Tate, A. R., Underwood, J., Acosta, D. M., Julià-Sapé, M., Majós, C., Moreno-Torres, À., et al. (2006). Development of a decision support system for diagnosis and grading of brain tumours usingin vivo magnetic resonance single voxel spectra. NMR Biomed. 19, 411–434. doi:10.1002/nbm.1016
The Cancer Genome Atlas Research Network Weinstein, J. N., Collisson, E. A., Mills, G. B., Shaw, K. R. M., Ozenberger, B. A., et al. (2013). The cancer genome Atlas pan-cancer analysis project. Nat. Genet. 45, 1113–1120. doi:10.1038/ng.2764
Toth, M., Boros, I. M., and Balint, E. (2012). Elevated level of lysine 9-acetylated histone H3 at the MDR1 promoter in multidrug-resistant cells. Cancer Sci. 103, 659–669. doi:10.1111/j.1349-7006.2012.02215.x
Treangen, T. J., and Salzberg, S. L. (2012). Repetitive DNA and next-generation sequencing: computational challenges and solutions. Nat. Rev. Genet. 13, 36–46. doi:10.1038/nrg3117
Ubels, J., Schaefers, T., Punt, C., Guchelaar, H.-J., and De Ridder, J. (2020). RAINFOREST: a random forest approach to predict treatment benefit in data from (failed) clinical drug trials. Bioinformatics 36, i601–i609. doi:10.1093/bioinformatics/btaa799
Uffelmann, E., Huang, Q. Q., Munung, N. S., De Vries, J., Okada, Y., Martin, A. R., et al. (2021). Genome-wide association studies. Nat. Rev. Methods Prim. 1, 59. doi:10.1038/s43586-021-00056-9
Van Der Lee, M., and Swen, J. J. (2023). Artificial intelligence in pharmacology research and practice. Clin. Transl. Sci. 16, 31–36. doi:10.1111/cts.13431
Vogel, C., De Sousa Abreu, R., Ko, D., Le, S., Shapiro, B. A., Burns, S. C., et al. (2010). Sequence signatures and mRNA concentration can explain two-thirds of protein abundance variation in a human cell line. Mol. Syst. Biol. 6, 400. doi:10.1038/msb.2010.59
Wang, L., Pelleymounter, L., Weinshilboum, R., Johnson, J. A., Hebert, J. M., Altman, R. B., et al. (2010). Very important pharmacogene summary: thiopurine S-methyltransferase. Pharmacogenetics Genomics 20, 401–405. doi:10.1097/FPC.0b013e3283352860
Wang, M. H., Cordell, H. J., and Van Steen, K. (2019). Statistical methods for genome-wide association studies. Seminars Cancer Biol. 55, 53–60. doi:10.1016/j.semcancer.2018.04.008
Wang, W., Li, X., Qin, X., Miao, Y., Zhang, Y., Li, S., et al. (2023). Germline Neurofibromin 1 mutation enhances the anti-tumour immune response and decreases juvenile myelomonocytic leukaemia tumourigenicity. Br. J. Haematol. 202, 328–343. doi:10.1111/bjh.18851
Wang, X., Tokheim, C., Gu, S. S., Wang, B., Tang, Q., Li, Y., et al. (2021). In vivo CRISPR screens identify the E3 ligase Cop1 as a modulator of macrophage infiltration and cancer immunotherapy target. Cell 184, 5357–5374. doi:10.1016/j.cell.2021.09.006
Wang, Y., Xiao, F., Zhao, Y., Mao, C.-X., Yu, L.-L., Wang, L.-Y., et al. (2022). A two-stage genome-wide association study to identify novel genetic loci associated with acute radiotherapy toxicity in nasopharyngeal carcinoma. Mol. Cancer 21, 169. doi:10.1186/s12943-022-01631-8
Wang, Y., Yang, Y., Chen, S., and Wang, J. (2021). DeepDRK: a deep learning framework for drug repurposing through kernel-based multi-omics integration. Briefings Bioinforma. 22, bbab048. doi:10.1093/bib/bbab048
Weiss, L., Dorman, K., Boukovala, M., Schwinghammer, F., Jordan, P., Fey, T., et al. (2023). Early clinical trial unit tumor board: a real-world experience in a national cancer network. J. Cancer Res. Clin. Oncol. 149, 13383–13390. doi:10.1007/s00432-023-05196-x
Werner, R., Connolly, A., Bennett, M., Hand, C. K., and Burke, L. (2022). Implementation of an ISO15189 accredited next-generation sequencing service with the fully automated Ion Torrent Genexus: the experience of a clinical diagnostic laboratory. J. Clin. Pathol. jcp 2022, 208625. doi:10.1136/jcp-2022-208625
Wong, C. H., Siah, K. W., and Lo, A. W. (2019). Corrigendum: estimation of clinical trial success rates and related parameters. Biostatistics 20, 366. doi:10.1093/biostatistics/kxy072
Woodman, R. J., and Mangoni, A. A. (2023). A comprehensive review of machine learning algorithms and their application in geriatric medicine: present and future. Aging Clin. Exp. Res. 35, 2363–2397. doi:10.1007/s40520-023-02552-2
Wu, F., Lu, M., Qu, L., Li, D.-Q., and Hu, C.-H. (2015). DNA methylation of hMLH1 correlates with the clinical response to cisplatin after a surgical resection in Non-small cell lung cancer. Int. J. Clin. Exp. Pathol. 8, 5457–5463.
Wu, L.-X., Wen, C.-J., Li, Y., Zhang, X., Shao, Y.-Y., Yang, Z., et al. (2015). Interindividual epigenetic variation in ABCB1 promoter and its relationship with ABCB1 expression and function in healthy Chinese subjects: interindividual epigenetic variation in ABCB1 in healthy Chinese subjects. Br. J. Clin. Pharmacol. 80, 1109–1121. doi:10.1111/bcp.12675
Xu, J., Zheng, M., Thng, D. K. H., Toh, T. B., Zhou, L., Bonney, G. K., et al. (2023). NanoBeacon.AI: AI-enhanced nanodiamond biosensor for automated sensitivity prediction to oxidative phosphorylation inhibitors. ACS Sens. 8, 1989–1999. doi:10.1021/acssensors.3c00126
Xu, W., Yang, H., Liu, Y., Yang, Y., Wang, P., Kim, S.-H., et al. (2011). Oncometabolite 2-hydroxyglutarate is a competitive inhibitor of α-ketoglutarate-dependent dioxygenases. Cancer Cell 19, 17–30. doi:10.1016/j.ccr.2010.12.014
Yu, Y., Werdyani, S., Carey, M., Parfrey, P., Yilmaz, Y. E., and Savas, S. (2021). A comprehensive analysis of SNPs and CNVs identifies novel markers associated with disease outcomes in colorectal cancer. Mol. Oncol. 15, 3329–3347. doi:10.1002/1878-0261.13067
Yu, Z., Ye, X., Liu, H., Li, H., Hao, X., Zhang, J., et al. (2022). Predicting lapatinib dose regimen using machine learning and deep learning techniques based on a real-world study. Front. Oncol. 12, 893966. doi:10.3389/fonc.2022.893966
Zeng, X., Zhu, S., Lu, W., Liu, Z., Huang, J., Zhou, Y., et al. (2020). Target identification among known drugs by deep learning from heterogeneous networks. Chem. Sci. 11, 1775–1797. doi:10.1039/C9SC04336E
Zhang, D., Dorman, K., Heinrich, K., Weiss, L., Boukovala, M., Haas, M., et al. (2023). A retrospective analysis of biliary tract cancer patients presented to the molecular tumor board at the comprehensive cancer center munich. Targ. Oncol. 18, 767–776. doi:10.1007/s11523-023-00985-3
Zhang, H., Huang, J., Chen, R., Cai, H., Chen, Y., He, S., et al. (2022). Ligand- and structure-based identification of novel CDK9 inhibitors for the potential treatment of leukemia. Bioorg. Med. Chem. 72, 116994. doi:10.1016/j.bmc.2022.116994
Zhang, J., Baran, J., Cros, A., Guberman, J. M., Haider, S., Hsu, J., et al. (2011). International cancer genome Consortium data portal--a one-stop shop for cancer genomics data. Database 2011, bar026. doi:10.1093/database/bar026
Zhang, X., Wu, J., Baeza, J., Gu, K., Zheng, Y., Chen, S., et al. (2023). DeepTAP: an RNN-based method of TAP-binding peptide prediction in the selection of tumor neoantigens. Comput. Biol. Med. 164, 107247. doi:10.1016/j.compbiomed.2023.107247
Zhou, H., Hua, Z., Gao, J., Lin, F., Chen, Y., Zhang, S., et al. (2023). Multitask deep learning-based whole-process system for automatic diagnosis of breast lesions and axillary lymph node metastasis discrimination from dynamic contrast-enhanced- mri: a multicenter study. Magn. Reson. Imaging jmri 2023, 28913. doi:10.1002/jmri.28913
Zhou, M., Yan, J., Chen, Q., Yang, Y., Li, Y., Ren, Y., et al. (2022). Association of H3K9me3 with breast cancer prognosis by estrogen receptor status. Clin. Epigenet 14, 135. doi:10.1186/s13148-022-01363-y
Keywords: pharmacogenomics, machine learning, omics, targeted therapy, drug toxicity, drug efficacy, drug repurposing
Citation: Mondello A, Dal Bo M, Toffoli G and Polano M (2024) Machine learning in onco-pharmacogenomics: a path to precision medicine with many challenges. Front. Pharmacol. 14:1260276. doi: 10.3389/fphar.2023.1260276
Received: 17 July 2023; Accepted: 26 December 2023;
Published: 09 January 2024.
Edited by:
Shaoqiu Chen, University of Hawaii at Mānoa, United StatesReviewed by:
Luca Cardone, National Research Council (CNR), ItalyCopyright © 2024 Mondello, Dal Bo, Toffoli and Polano. This is an open-access article distributed under the terms of the Creative Commons Attribution License (CC BY). The use, distribution or reproduction in other forums is permitted, provided the original author(s) and the copyright owner(s) are credited and that the original publication in this journal is cited, in accordance with accepted academic practice. No use, distribution or reproduction is permitted which does not comply with these terms.
*Correspondence: Maurizio Polano, bXBvbGFub0Bjcm8uaXQ=
Disclaimer: All claims expressed in this article are solely those of the authors and do not necessarily represent those of their affiliated organizations, or those of the publisher, the editors and the reviewers. Any product that may be evaluated in this article or claim that may be made by its manufacturer is not guaranteed or endorsed by the publisher.
Research integrity at Frontiers
Learn more about the work of our research integrity team to safeguard the quality of each article we publish.