- 1Centre for Medicine Use and Safety, Monash University, Parkville, VIC, Australia
- 2Section for Colorectal Cancer Screening, Cancer Registry of Norway, Oslo, Norway
- 3Research Department, Cancer Registry of Norway, Oslo, Norway
- 4Monash Institute of Pharmaceutical Sciences, Drug Discovery Biology Theme, Monash University, Parkville, VIC, Australia
- 5Division of Cancer Surgery, Peter MacCallum Cancer Centre, Melbourne, VIC, Australia
Introduction: Cancer registries and hospital electronic medical records are commonly used to investigate drug repurposing candidates for cancer. However, administrative data are often more accessible than data from cancer registries and medical records. Therefore, we evaluated if administrative data could be used to evaluate drug repurposing for cancer by conducting an example study on the association between beta-blocker use and breast cancer mortality.
Methods: A retrospective cohort study of women aged ≥50 years with incident breast cancer was conducted using a linked dataset with statewide hospital admission data and nationwide medication claims data. Women receiving beta blockers and first-line anti-hypertensives prior to and at diagnosis were compared. Breast cancer molecular subtypes and metastasis status were inferred by algorithms from commonly prescribed breast cancer antineoplastics and hospitalization diagnosis codes, respectively. Subdistribution hazard ratios (sHR) and corresponding 95% confidence intervals (CIs) for breast cancer mortality were estimated using Fine and Gray’s competing risk models adjusted for age, Charlson comorbidity index, congestive heart failure, myocardial infraction, molecular subtype, presence of metastasis at diagnosis, and breast cancer surgery.
Results: 2,758 women were hospitalized for incident breast cancer. 604 received beta-blockers and 1,387 received first-line antihypertensives. In total, 154 breast cancer deaths were identified over a median follow-up time of 2.7 years. We found no significant association between use of any beta-blocker and breast-cancer mortality (sHR 0.86, 95%CI 0.58–1.28), or when stratified by beta-blocker type (non-selective, sHR 0.42, 95%CI 0.14–1.25; selective, sHR 0.95, 95%CI 0.63–1.43). Results were not significant when stratified by molecular subtypes (e.g., triple negative breast cancer (TNBC), any beta blocker, sHR 0.16, 95%CI 0.02–1.51).
Discussion: It is possible to use administrative data to explore drug repurposing opportunities. Although non-significant, an indication of an association was found for the TNBC subtype, which aligns with previous studies using registry data. Future studies with larger sample size, longer follow-up are required to confirm the association, and linkage to clinical data sources are required to validate our methodologies.
Introduction
Drug repurposing is a promising supplement to de novo drug discovery and development due to time and cost savings (Parvathaneni et al., 2019; Pushpakom et al., 2019; Roy et al., 2021). Repurposed drugs have lower attrition rates across clinical trial and regulatory approval phases compared to their de novo counterparts (Parvathaneni et al., 2019; Pushpakom et al., 2019; Roy et al., 2021). This is particularly relevant to oncology, where on average only 3.4% of drugs successfully traverse from Phase I clinical trials to market approval (Wong et al., 2019). Real-world data, including administrative healthcare data, may be used to discover or validate drug repurposing candidates via pharmaco-epidemiological analyses (Pushpakom et al., 2019; Ozery-Flato et al., 2020; Xu et al., 2020; Chen et al., 2021). The benefits of administrative healthcare data are longitudinal individual-level records, large population-based sample sizes, and widespread availability (Katkade et al., 2018; Ilomaki et al., 2020; Franklin et al., 2021; Tan et al., 2023). These advantages are attributable to embedded data collection processes during routine provision of healthcare.
Beta-blockers have been identified as potential repurposing candidates for cancer due to emerging evidence from preclinical studies (Sloan et al., 2010; Cole and Sood, 2012; Magnon et al., 2013; Creed et al., 2015; Chang et al., 2016; Le et al., 2016), retrospective observational studies across various cancer types (e.g., melanoma (De Giorgi et al., 2013), ovarian cancer (Watkins et al., 2015; Couttenier et al., 2019), colorectal cancer (Jansen et al., 2014), prostate cancer (Lu et al., 2015; Malik et al., 2023), and breast cancer (Botteri et al., 2013; Lofling et al., 2022)), and recent prospective clinical trials (Shaashua et al., 2017; Hiller et al., 2020). Despite advances in breast cancer therapeutics, metastasis remains the key prognostic marker for poor survival and the main cause of death (Weigelt et al., 2005; Jung et al., 2011). Preclinical evidence suggests that beta-blockade reduces metastasis by limiting tumour cell invasion, inflammation in the tumor microenvironment, and lymphatic vasculature remodelling (Palm et al., 2006; Sloan et al., 2010; Creed et al., 2015; Chang et al., 2016; Le et al., 2016). Additionally, beta-blockers may interact with breast cancer treatments to exert their protective effects (Pasquier et al., 2011; Reeder et al., 2015; Chang et al., 2023). However, a meta-analysis of epidemiological and perioperative studies by Yap et al. (2018) raised the possibility that the effects of beta-blockers on cancer outcomes may be tumor type-specific, as significant benefits were observed only in melanoma and ovarian cancer (Yap et al., 2018). Several retrospective observational studies found that beta-blocker use is associated with improved cancer outcomes in breast cancer (Powe et al., 2010; Barron et al., 2011; Melhem-Bertrandt et al., 2011; Botteri et al., 2013; Sorensen et al., 2013; Choy et al., 2016; Gillis et al., 2021; Lofling et al., 2022), while other studies have reported no significant association (Cardwell et al., 2013; Ganz et al., 2011; Sakellakis et al., 2014; Modi et al., 2020-; Cardwell et al., 2016). Moreover, meta-analyses by Løfling et al. (2022), Caparica et al. (2021) and Spini et al. (2019) have demonstrated more consistent protective effects of beta-blockers amongst women with triple negative breast cancer (TNBC) (Spini et al., 2019; Caparica et al., 2021; Lofling et al., 2022). Previous observational studies predominantly used data from population-based cancer registries and hospital electronic medical records (Mohammadzadeh et al., 2017), which contain detailed histopathological and other clinical information about cancer. However, cancer registry data may be unavailable or unfit for research purposes, especially in some developing countries (Valsecchi and Steliarova-Foucher, 2008; Abdul-Sater et al., 2021).
There is currently a paucity of studies using administrative healthcare data alone to investigate oncology-related repurposing hypotheses. To address this, we aimed to evaluate a drug repurposing opportunity in cancer using administrative healthcare data by analysing the association between beta-blocker use and breast cancer survival. We used an active comparator study design that compared beta-blocker users to users of first-line anti-hypertensives to reduce confounding and healthy user bias (Lund et al., 2015). In the absence of detailed cancer-related clinical information in the administrative dataset, we used longitudinal hospital admission data and prescription medication claims to infer incident cancer cases, baseline medication use and comorbidities, as well as breast cancer molecular subtypes.
Materials and methods
Data sources
We conducted a cohort study using hospitalization data from the Victorian Admitted Episodes Dataset (VAED), linked to medication claims data from the Australian Pharmaceutical Benefits Scheme (PBS) and death data from the National Death Index. Probabilistic linkage was performed by the Australian Institute of Health and Welfare (Madden et al., 2022), based on first name, middle names, surname, date of birth, date of death, street address, suburb, postcode, and sex. This linked dataset consists of 450,958 individuals discharged from any public or private hospital in the state of Victoria, Australia (total population of 6.5 million) between 1 July 2012 and 30 June 2018, and had one of the following four conditions recorded at the hospital: diabetes complication, acute coronary syndrome, stroke, or hip fracture.
The VAED data contains hospital admission data for each person from 1 July 2006 to 30 June 2018, including admission and discharge dates, principal and up to 39 additional diagnostic codes based on International Statistical Classification of Diseases and Related Health Problems, 10th Revision, Australian Modification (ICD-10-AM) (The International Statistical Classification, 2019), procedural codes based on Australian Classification of Health Interventions (ACHI) (Australian Classification, 2019), demographic information including age in 5-year groups and sex. The PBS is a nationwide single-payer system that subsidizes prescription medications for Australian citizens, permanent residents, and people from countries with reciprocal healthcare arrangements with Australia. Linkage to the PBS allowed identification of prescription medicine claims from 1 January 2005 to 30 June 2018, including information on the medication name, strength, quantity supplied, and dispensing date (Mellish et al., 2015). The National Death Index provided the date and causes of death. Cause of death includes the underlying cause and other causes of death.
Study population
The study cohort was defined as women, aged ≥50 years discharged from any Victorian hospital between 1 November 2012 to 30 June 2018 with breast cancer as the principal diagnosis (ICD-10-AM code C50). This start date was necessary because medications costing less than the general co-payment amount were not recorded in the dataset for general beneficiaries prior to 1 July 2012 (Mellish et al., 2015), and we required a 120-day window for medication exposure ascertainment prior to hospital admission. The first hospitalization with breast cancer recorded as a principal diagnosis during the study period was defined as the index hospitalization. The discharge date of the index hospitalization was defined as the index date. The cohort was limited to women aged ≥50 years to exclude premenopausal women because of the use of different treatment strategies, poorer prognoses, and to reduce heterogeneity between beta-blocker users and comparators (Azim et al., 2016). People with prior hospital admissions with cancer recorded as any diagnoses since 1 July 2006 or dispensing records of any antineoplastics from 1 January 2005 until the index hospitalization were excluded to obtain an incident cancer cohort.
Exposure
We determined exposure to beta-blockers and comparator medications (first-line antihypertensives), using a 120-day lookback period from the admission date (inclusive) of index hospitalization (Figure 1). Pre-diagnostic use of beta-blocker was chosen as the exposure of interest to address the hypothesis that beta-blockers have synergistic effects with breast cancer treatment during the diagnostic period (Pasquier et al., 2011; Reeder et al., 2015). Beta-blocker exposure was defined by having at least one beta-blocker dispensing record within the lookback period (Supplementary Appendix S1). We classified people as having received either selective or nonselective beta blockers based on the most recent dispensing date. To reduce confounding by indication, the comparators were defined as people with at least one dispensing record of a first-line anti-hypertensive medication with no beta-blocker dispensing record during the look-back period. Comparator medications were selected based on first-line recommendations in the Australian Therapeutic Guidelines (Blood Pressure Reduction, 2021), including angiotensin-converting enzyme inhibitors, angiotensin-receptor blockers, thiazide-like diuretics and dihydropyridine calcium channel blockers. All medications were defined based on the Anatomical Therapeutic Chemical (ATC) classification of medications (Supplementary Appendix S1 and S2).
Covariates
Molecular subtypes of breast cancer diagnoses were inferred using an algorithm of breast cancer medications (Table 1), based on Australian clinical practice guidelines (eviQ Cancer Institute NSW, 2021; Immunomodulators and antineoplastics, 2021). This was completed using medication dispensing records from the 2 years during and after the index date, including medications that target the estrogen and/or progesterone receptor, namely, selective estrogen receptor modulators (tamoxifen, toremifene), aromatase inhibitors (letrozole, anastrozole, exemestane) and gonadotrophin-releasing hormone agonist (goserelin), and HER2 targeting monoclonal antibodies (trastuzumab and pertuzumab).
The Charlson comorbidity index by Quan et al. was used to identify 17 comorbidities based on ICD-10-AM codes from all hospitalizations on or before the index date (Supplementary Appendix S3) (Charlson et al., 1987; Quan et al., 2005). Weighted Charlson comorbidity scores were calculated, excluding cancer and metastasis because everyone in the cohort had one or both of these. Baseline metastasis was identified from the index hospitalization using ICD-10-AM codes C77 for regional lymph node metastasis, and C78, C79, and C80 for distant metastasis. Breast cancer surgery during index hospitalization was ascertained using recorded procedural codes. Breast cancer surgery codes include those for excision of lesions and mastectomy (Supplementary Appendix S4) (Victorian Cancer Quality, 2018).
Outcomes
The primary outcome in this study was breast cancer mortality and the secondary outcome was all-cause mortality. Follow-up started from the index date until the end of the study period 30 June 2018. People who died prior to hospital discharge were excluded. Breast cancer death was determined using ICD-10-AM code C50 recorded in the National Death Index using both underlying and other recorded causes of death. We included other recorded causes of death in the definition to improve sensitivity as it has been reported that only 77.5% of breast cancer-related deaths had breast cancer coded as the “underlying” cause of death in the National Death Index (Multiple causes of death, 2012).
Statistical analyses
Descriptive analyses were used to describe characteristics of the study cohort. Categorical variables are reported with frequencies and percentages; continuous variables with medians and interquartile ranges (IQR). Statistical significance in the cohort characteristics between the groups was determined using parametric or non-parametric tests as appropriate, with an alpha level of 0.05.
Multivariable analyses were conducted for all beta-blockers, and selective and nonselective beta-blockers separately. All models were adjusted for age group, weighted Charlson comorbidity index, congestive heart failure, myocardial infarction, baseline metastasis (no metastasis, regional or distant metastasis), breast cancer molecular subtypes and breast cancer surgery during the index hospitalization. Congestive heart failure and myocardial infarction were used as separate covariates due to both being the main indications for beta-blocker use. We carried out competing-risk analyses using Fine and Gray’s subdistribution hazards model to estimate the subdistribution hazards ratios (sHR) and 95% confidence intervals (CI) for breast cancer mortality (Fine and Gray, 1999). Deaths due to other causes were used as competing events in breast cancer mortality analyses. Cox proportional hazards model was used to estimate the hazard ratios (HR) and 95% CI for overall survival.
Stratified analyses were conducted for age groups (<65 years vs. ≥ 65 years), weighted Charlson comorbidity index (<3 and ≥3), hormone-receptor positive breast cancer, TNBC, and cancer baseline metastasis (no metastasis, regional or distant metastasis) to examine potential effect modification.
All analyses were conducted using SAS version 9.4 (SAS Institute Inc., Cary, NC, United States).
Results
Cohort description
From a cohort of 2,758 women ≥50 years with breast cancer, there was a total of 604 beta-blocker users in our study cohort, including 89 nonselective and 515 selective beta-blocker users (Figure 2; Table 2). There were in total 1,387 users of first-line antihypertensives (active comparators). The median age of our study population was 71 years.
Beta-blocker users were older than the comparators (≥80 years old: 29% vs. 18%, p < 0.001). (Table 2). Beta-blocker users tended to have more comorbidities compared to comparators (weighted Charlson comorbidity index of ≥4: 23% vs. 14%, p = 0.005). Beta-blockers users were more likely to have congestive heart failure and a history of myocardial infarction than comparators (15% vs. 5%, p < 0.001; 12% vs. 4%, p < 0.001). Beta-blocker users and comparators had similar distributions of baseline metastasis, breast cancer molecular subtypes, and breast cancer surgery during the index hospitalization period.
Breast cancer survival
There were in total 154 breast cancer deaths over the median follow-up time of 2.7 years (IQR 2.8 years, Table 3). After adjusting for all covariates, there was no difference in breast cancer survival between any beta-blocker users and the comparators (sHR 0.86, 95% CI 0.58–1.28). When stratified by beta-blocker type, there was no difference in breast cancer mortality between nonselective (sHR 0.42, 95% CI 0.14–1.25) or selective beta-blocker users (sHR 0.95, 95% CI 0.63–1.43) compared to the comparators.
Results were similar when stratified by age group, weighted Charlson comorbidity index, baseline metastasis and breast cancer molecular subtype (Figure 3). In particular, we did not find a significant association between beta blocker use and breast cancer survival in women with TNBC (any beta-blocker, sHR 0.16, 95% CI 0.02–1.51; selective beta-blocker, sHR 0.22, 95% CI 0.02–2.63). Results could not be determined for all HER2 positive subgroups and TNBC subgroup for non-selective beta-blocker users due to small number or lack of events.
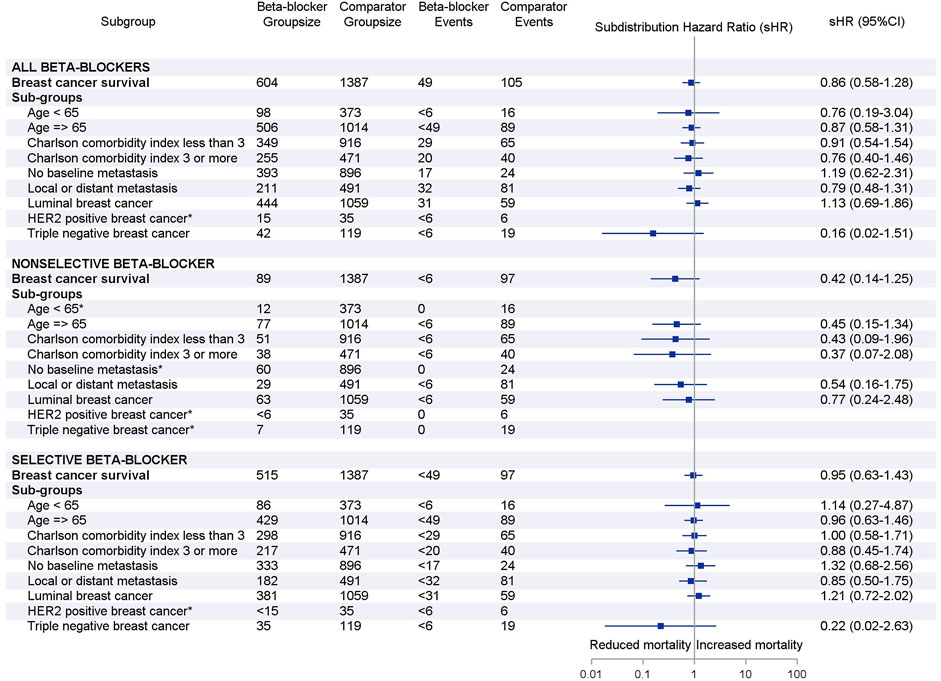
FIGURE 3. Forest plots of subgroup analyses by beta-blocker use. Adjusted subdistribution hazard ratios reported based on Fine and Gray’s subdistribution hazard model. Note: small cell suppression (<6) is required by Australian Institute of Health and Welfare. *Hazards ratio could not be estimated due to small number or lack of breast cancer deaths in subgroups.
All-cause survival
There were 265 all-cause deaths over the same follow-up period (Table 3). In the multivariable analyses, there was no significant difference in all-cause mortality between any beta-blocker use (HR 1.02, 95% CI 0.79–1.32) compared to the comparators. Results remained similar when stratified by beta-blocker type: nonselective beta-blocker (HR 0.80, 95% CI 0.45–1.42) and selective beta-blocker (HR 1.07, 95% CI 0.81–1.40).
Discussion
In this example using administrative healthcare data to investigate a drug repurposing candidate for cancer, we found no association between either use of any beta-blocker, nonselective beta-blocker use, or selective beta-blocker use, and short-term breast cancer mortality (median 2.7 years of follow-up). The results are aligned with a meta-analysis of 13 studies by Caparica et al. (2021) who reported no significant difference between beta blocker use and breast cancer mortality (Caparica et al., 2021). However, notably recent studies using cancer registry data or hospital electronic medical records with longer follow-up times and larger sample sizes have demonstrated that beta-blockers prolong breast cancer survival in TNBC patients. A recent meta-analysis of four independent studies by Løfling et al. (2022) reported a 26% reduced risk of breast cancer death in women with TNBC with the use of beta blockers compared to no use (Lofling et al., 2022). For example, Botteri et al. (2013) observed a 48% reduced risk of breast cancer death in women with TNBC who used any beta-blockers (n = 74) over 5 years (Botteri et al., 2013). Løfling et al. (2022) demonstrated a 34% improved breast cancer-survival with beta blocker use in women with TNBC (n = 312) over 5 years (Lofling et al., 2022). Our analyses indicated a possible reduction in breast cancer mortality for women with TNBC using beta-blockers compared to comparators (sHR 0.16, 95% CI 0.02–1.51), although results were not statistically significant. This may be due to short follow-up, small sample size, fundamental differences in study cohorts, and residual confounding (see limitations below).
The key advantage of administrative healthcare data over cancer registry data or electronic medical records is the presence of longitudinal and comprehensive records of medication use. In this study, we ascertained baseline use of beta-blockers by using longitudinal prescription medication claims. This allowed us to accurately identify the type of beta-blocker use (selective vs. non-selective) dispensed prior to breast cancer diagnosis. Studies using cancer registry data without linkage to medication claims often rely on patient self-reports or hospital electronic medical records to identify baseline use of medications (Pop et al., 2019). Self-reporting in cancer registry data is subject to recall bias. Moreover, hospital electronic medical records may not have exact information when the medication was initiated and may include initiation of exposure medication use after a breast cancer diagnosis. Ascertaining exposure of medications after the index date may lead to an immortal time bias, where people by design need to survive event-free until the exposure starts at a time after the index date (Suissa, 2008). Immortal time bias could distort results towards favourable drug-outcome associations (Weberpals et al., 2016). This was demonstrated in a meta-analysis by Zhong et al. (2016), which found that post- but not pre-diagnostic use of beta-blockers was associated with improved cancer survival (Zhong et al., 2016).
Cancer registries remain the gold standard real-world data source for evaluating drug repurposing opportunities for cancer due to rich clinical information on cancer diagnosis and management. However, administrative healthcare data are considered as alternative data sources if cancer registry data are unavailable or unfit for research purposes, especially in developing countries (Valsecchi and Steliarova-Foucher, 2008; Abdul-Sater et al., 2021). Several previous studies have used administrative data to evaluate drug repurposing opportunities for cancers other than breast cancer (Chen et al., 2019; Chen et al., 2020; Ng et al., 2021; Park et al., 2021). Some of these studies even have enriched administrative healthcare data (Ng et al., 2021), or linkage to external registry to provide or confirm cancer-related clinical information (Chen et al., 2019; Chen et al., 2020).
Due to the lack of cancer-specific histopathological clinical data in our dataset, we acquired information on incident breast cancer cases from longitudinal hospitalization data and prescription medication claims. We identified the first hospitalization record where breast cancer was recorded as a primary diagnosis and used this as a proxy for the date of breast cancer diagnosis. Cancer registries usually record incident cancer cases and provide accurate dates of diagnosis (Yuen et al., 2011; Okuyama et al., 2018). However, Yuen et al. have demonstrated good sensitivity (84.8%) and specificity (99.9%) between administrative data and actual recorded dates of diagnosis in cancer registry (Yuen et al., 2011).
We used diagnosis and procedural codes from hospitalization data and prescription medication claims data to infer breast cancer molecular subtypes and staging. We developed a novel algorithm to estimate breast cancer molecular subtypes from commonly prescribed antineoplastics used to treat each molecular subtype of breast cancer. Molecular subtype was used to stratify and adjust our analyses. Moreover, we used the presence of breast cancer surgery at index hospital admission and diagnosis codes for metastasis to infer early breast cancer cases. The meta-analysis by Caparica et al. (2021) evaluated the association between beta-blocker use and breast cancer survival in early breast cancer cases given their better prognoses (Caparica et al., 2021). Given that early breast cancer cases were likely to have localized operable tumors or no metastasis, we included both the presence of breast cancer surgery during index hospitalization and metastasis as independent covariates for adjustment in our analyses.
Strengths of our study include the use of active comparators and competing risk analyses. The use of active comparators minimizes confounding by indication (Lund et al., 2015), and other differences in baseline characteristics between the exposure groups. The active comparators also minimize healthy user bias as all women in the study were active users of preventive cardiovascular medications and the healthcare system (Lund et al., 2015). Despite this, a higher proportion of beta-blocker users had congestive heart failure and higher weighted Charlson comorbidity scores than the comparators, therefore, our model adjusted for both of these. We also considered competing risks in our time-to-event survival analyses using Fine and Gray’s subdistribution hazard model. Competing risk events can lead to overestimation of risk when using the conventional Cox proportional hazards regression model (Tan et al., 2018). This is especially true when analyzing a cohort with a substantial proportion of older people, as the incidence of diseases that are attributable to aging and frailty increases (Tan et al., 2018).
There are important limitations to this study. First, the limited sample size and short follow-up time (median 2.7 years) led to underpowered analyses. Second, the study cohort included people admitted to hospitals with diabetes, acute coronary syndrome, stroke, or hip fracture only. This limited the ability to generalize the results to a general population of women with breast cancer. To address these two limitations, future studies would benefit from using larger scale administrative data from a nationwide or general population-based cohort to both increase the power of study and generalizability of results. Third, there is the potential of confounding by severity as beta-blockers are not first-line anti-hypertensives and are prescribed for people with additional cardiovascular comorbidities, notably ischaemic heart disease and congestive heart failure (Blood Pressure Reduction, 2021). We considered first-line anti-hypertensives as the best possible active comparator medications as they have previously been used in other studies to reduce confounding (Gillis et al., 2021; Lofling et al., 2022), and included myocardial infarction and congestive heart failure as covariates in the models. Furthermore, there could be residual confounding due to undetermined histopathological characteristics of breast cancer tumors, for example, tumor size and Ki-67 expression, which are conventionally available from cancer registry data but not in our dataset. Fourth, we used dispensing records during follow-up to estimate breast cancer molecular subtype, which may lead to selection bias in the stratified analyses by molecular subtypes. This is because people need to survive during follow-up until receiving the breast cancer treatments (Acton et al., 2023). Furthermore, we were not able to validate the sensitivity and specificity of the algorithm used in inferring breast cancer molecular subtypes as we did not have information on the subtypes available. Future validation studies with data linkage to cancer registries are required to confirm the validity of the algorithm. Moreover, we were unable to classify breast cancer molecular subtype for 14% of women as they did not have dispensing records of either breast cancer antineoplastics or conventional chemotherapy. Therefore, the algorithm could not infer the breast cancer molecular subtypes for women who have undergone surgery or radiotherapy only. Lastly, given that this study only includes data from patients treated until mid-2018, our results do not include the effects of emerging immunotherapies used since 2019 (Barzaman et al., 2021). Preclinical studies have reported that beta-blockade enhances the response to immunotherapy, for example, anti-CTLA4 therapy (Fjaestad et al., 2022), raising the possibility that beta blocker use might enhance new immunotherapy regimens.
Conclusion
Use of administrative data as an alternative to registry data increases opportunities to identify novel drug repurposing candidates. In addition to being more widespread and accessible than registry data, administrative data often has longitudinal and comprehensive records of medication use that can be used to ascertain medication exposure. The current study emphasizes the need for use of large administrative datasets to provide sufficient power to identify associations. The current study describes the development of methodologies to infer cancer-related clinical information from variables available in administrative dataset. Future studies with linkage to clinical data sources are required to validate these methodologies.
Data availability statement
The data analyzed in this study is subject to the following licenses/restrictions; The data that support the findings of this study are available from the Australian Institute of Health and Welfare. Restrictions apply to the availability of these data, which were used under license for this study. Data are available from the authors only with the permission of the Australian Institute of Health and Welfare. Requests to access these datasets should be directed to the Australian Institute of Health and Welfare.
Ethics statement
The studies involving humans were approved by the Monash University Human Research Ethics Committee and the Australian Institute of Health and Welfare Ethics Committee. The studies were conducted in accordance with the local legislation and institutional requirements. Written informed consent for participation was not required from the participants or the participants’ legal guardians/next of kin in accordance with the national legislation and institutional requirements.
Author contributions
GT contributed to the design of the study, performed the statistical analysis and literature search, and wrote and revise the manuscript. JI contributed to the design of the study, acquisition of data and revision of the manuscript. EB, SW, and ES contributed to the design of the study and revision of the manuscript. All authors contributed to the article and approved the submitted version.
Funding
GT was supported by the Monash Graduate Scholarship. ES and JI were supported by funding from the National Breast Cancer Foundation (IIRS-20-025).
Acknowledgments
We would like to thank Ms. Catherine Downey from Peter MacCallum Cancer Centre and Dr. Belinda Yeo, Olivia Newton John Cancer Research Institute, Victorian Comprehensive Cancer Centre, for providing guidance in medication use by breast cancer patients, and Mr. Anthony Del Monaco from Monash Centre for Occupational and Environmental Health for advice on the use of the National Death Index. We are also grateful for the National Breast Cancer Foundation for supporting this work.
Conflict of interest
The authors declare that the research was conducted in the absence of any commercial or financial relationships that could be construed as a potential conflict of interest.
Publisher’s note
All claims expressed in this article are solely those of the authors and do not necessarily represent those of their affiliated organizations, or those of the publisher, the editors and the reviewers. Any product that may be evaluated in this article, or claim that may be made by its manufacturer, is not guaranteed or endorsed by the publisher.
Supplementary material
The Supplementary Material for this article can be found online at: https://www.frontiersin.org/articles/10.3389/fphar.2023.1227330/full#supplementary-material
References
Abdul-Sater, Z., Shamseddine, A., Taher, A., Fouad, F., Abu-Sitta, G., Fadhil, I., et al. (2021). Cancer registration in the Middle East, north africa, and Turkey: scope and challenges. JCO Glob. Oncol. 7, 1101–1109. doi:10.1200/GO.21.00065
Acton, E. K., Willis, A. W., and Hennessy, S. (2023). Core concepts in pharmacoepidemiology: key biases arising in pharmacoepidemiologic studies. Pharmacoepidemiol Drug Saf. Jan. 32 (1), 9–18. doi:10.1002/pds.5547
Australian Classification (2019). Australian classification of Health Interventions (ACHI). 11th ed. Australian: Australian Consortium for Classification Development.
Azim, H. A., Davidson, N. E., and Ruddy, K. J. (2016). Challenges in treating premenopausal women with endocrine-sensitive breast cancer. Am. Soc. Clin. Oncol. Educ. Book 35 (36), 23–32. doi:10.1200/EDBK_159069
Barron, T. I., Connolly, R. M., Sharp, L., Bennett, K., and Visvanathan, K. (2011). Beta blockers and breast cancer mortality: a population-based study. J. Clin. Oncol. 29 (19), 2635–2644. doi:10.1200/JCO.2010.33.5422
Barzaman, K., Moradi-Kalbolandi, S., Hosseinzadeh, A., Kazemi, M. H., Khorramdelazad, H., Safari, E., et al. (2021). Breast cancer immunotherapy: current and novel approaches. Int. Immunopharmacol. 98, 107886. doi:10.1016/j.intimp.2021.107886
Blood Pressure Reduction (2021). Therapeutic guidelines (eTG). Mar 2021. Therapeutic Guidelines Limited. Available at: https://www.tg.org.au/.
Botteri, E., Munzone, E., Rotmensz, N., Cipolla, C., De Giorgi, V., Santillo, B., et al. (2013). Therapeutic effect of beta-blockers in triple-negative breast cancer postmenopausal women. Breast Cancer Res. Treat. Aug 140 (3), 567–575. doi:10.1007/s10549-013-2654-3
Caparica, R., Bruzzone, M., Agostinetto, E., De Angelis, C., Fêde, Â., Ceppi, M., et al. (2021). Beta-blockers in early-stage breast cancer: a systematic review and meta-analysis. ESMO Open 6 (2), 100066. doi:10.1016/j.esmoop.2021.100066
Cardwell, C. R., Coleman, H. G., Murray, L. J., Entschladen, F., and Powe, D. G. (2013). Beta-blocker usage and breast cancer survival: a nested case-control study within a UK clinical practice research datalink cohort. Int. J. Epidemiol. Dec 42 (6), 1852–1861. doi:10.1093/ije/dyt196
Cardwell, C. R., Pottegard, A., Vaes, E., Garmo, H., Murray, L. J., Brown, C., et al. (2016). Propranolol and survival from breast cancer: a pooled analysis of European breast cancer cohorts. Breast Cancer Res. 18 (1), 119. doi:10.1186/s13058-016-0782-5
Chang, A., Botteri, E., Gillis, R. D., Löfling, L., Le, C. P., Ziegler, A. I., et al. (2023). Beta-blockade enhances anthracycline control of metastasis in triple-negative breast cancer. Sci. Transl. Med. 15 (693), eadf1147. doi:10.1126/scitranslmed.adf1147
Chang, A., Le, C. P., Walker, A. K., Creed, S. J., Pon, C. K., Albold, S., et al. (2016). β2-Adrenoceptors on tumor cells play a critical role in stress-enhanced metastasis in a mouse model of breast cancer. Brain Behav. Immun. 57, 106–115. doi:10.1016/j.bbi.2016.06.011
Charlson, M. E., Pompei, P., Ales, K. L., and MacKenzie, C. R. (1987). A new method of classifying prognostic comorbidity in longitudinal studies: development and validation. J. Chronic Dis. 40 (5), 373–383. doi:10.1016/0021-9681(87)90171-8
Chen, V. C., Chan, H. L., Hsu, T. C., Lu, M. L., Lee, Y. C., Lee, Y., et al. (2019). New use for old drugs: the protective effect of atypical antipsychotics on hepatocellular carcinoma. Int. J. Cancer 144 (10), 2428–2439. doi:10.1002/ijc.31980
Chen, V. C., Hsieh, Y. H., Lin, T. C., Lu, M. L., Liao, Y. T., Yang, Y. H., et al. (2020). New use for old drugs: the protective effect of risperidone on colorectal cancer. Cancers (Basel) 12 (6), 1560. doi:10.3390/cancers12061560
Chen, Z., Liu, X., Hogan, W., Shenkman, E., and Bian, J. (2021). Applications of artificial intelligence in drug development using real-world data. Drug Discov. Today 26 (5), 1256–1264. doi:10.1016/j.drudis.2020.12.013
Choy, C., Raytis, J. L., Smith, D. D., Duenas, M., Neman, J., Jandial, R., et al. (2016). Inhibition of β2-adrenergic receptor reduces triple-negative breast cancer brain metastases: the potential benefit of perioperative β-blockade. Oncol. Rep. Jun 35 (6), 3135–3142. doi:10.3892/or.2016.4710
Cole, S. W., and Sood, A. K. (2012). Molecular pathways: beta-adrenergic signaling in cancer. Clin. Cancer Res. Mar. 1 18 (5), 1201–1206. doi:10.1158/1078-0432.CCR-11-0641
Couttenier, A., Lacroix, O., Silversmit, G., Vaes, E., De Schutter, H., and Robert, A. (2019). Beta-blocker use and mortality following ovarian cancer diagnosis: a population-based study. Cancer Epidemiol. 62, 101579. doi:10.1016/j.canep.2019.101579
Creed, S. J., Le, C. P., Hassan, M., Pon, C. K., Albold, S., Chan, K. T., et al. (2015). β2-adrenoceptor signaling regulates invadopodia formation to enhance tumor cell invasion. Breast Cancer Res. Nov. 25 17 (1), 145. doi:10.1186/s13058-015-0655-3
De Giorgi, V., Gandini, S., Grazzini, M., Benemei, S., Marchionni, N., and Geppetti, P. (2013). Effect of beta-blockers and other antihypertensive drugs on the risk of melanoma recurrence and death. Mayo Clin. Proc. Nov. 88 (11), 1196–1203. doi:10.1016/j.mayocp.2013.09.001
eviQ (Cancer Institute NSW) eviQ (cancer Institute NSW). Cancer Institute NSW. Available at: https://www.eviq.org.au/ (Accessed October 20, 2021).
Fine, J. P., and Gray, R. J. (1999). A proportional hazards model for the subdistribution of a competing risk. J. Am. Stat. Assoc. Jun 94 (446), 496–509. doi:10.1080/01621459.1999.10474144
Fjaestad, K. Y., Romer, A. M. A., Goitea, V., Johansen, A. Z., Thorseth, M. L., Carretta, M., et al. (2022). Blockade of beta-adrenergic receptors reduces cancer growth and enhances the response to anti-CTLA4 therapy by modulating the tumor microenvironment. Oncogene. Feb 41 (9), 1364–1375. doi:10.1038/s41388-021-02170-0
Franklin, J. M., Lin, K. J., Gatto, N. M., Rassen, J. A., Glynn, R. J., and Schneeweiss, S. (2021). Real-world evidence for assessing pharmaceutical treatments in the context of COVID-19. Clin. Pharmacol. Ther. 109 (4), 816–828. doi:10.1002/cpt.2185
Ganz, P. A., Habel, L. A., Weltzien, E. K., Caan, B. J., and Cole, S. W. (2011). Examining the influence of beta blockers and ACE inhibitors on the risk for breast cancer recurrence: results from the LACE cohort. Breast Cancer Res. Treat. Sep. 129 (2), 549–556. doi:10.1007/s10549-011-1505-3
Gillis, R. D., Botteri, E., Chang, A., Ziegler, A. I., Chung, N. C., Pon, C. K., et al. (2021). Carvedilol blocks neural regulation of breast cancer progression in vivo and is associated with reduced breast cancer mortality in patients. Eur. J. Cancer 147, 106–116. doi:10.1016/j.ejca.2021.01.029
Hiller, J. G., Cole, S. W., Crone, E. M., Byrne, D. J., Shackleford, D. M., Pang, J. M. B., et al. (2020). Preoperative beta-blockade with propranolol reduces biomarkers of metastasis in breast cancer: a phase II randomized trial. Clin. Cancer Res. 26 (8), 1803–1811. doi:10.1158/1078-0432.CCR-19-2641
Ilomaki, J., Lai, E. C., and Bell, J. S. (2020). Using clinical registries, administrative data and electronic medical records to improve medication safety and effectiveness in dementia. Curr Opin Psychiatry. Mar 33 (2), 163–169. doi:10.1097/YCO.0000000000000579
Immunomodulators and antineoplastics (2021). Immunomodulators and antineoplastics. Australian medicines handbook (AMH). Australian: Australian Medicines Handbook Pty Ltd. Available at: https://amhonline.amh.net.au//chapters/immunomodulators-antineoplastics.
Jansen, L., Hoffmeister, M., Arndt, V., Chang-Claude, J., and Brenner, H. Stage-specific associations between beta blocker use and prognosis after colorectal cancer. 2014;120(8):1178–1186. doi:10.1002/cncr.28546
Jung, S. Y., Sereika, S. M., Linkov, F., Brufsky, A., Weissfeld, J. L., and Rosenzweig, M. (2011). The effect of delays in treatment for breast cancer metastasis on survival. Breast Cancer Res. Treat. Dec 130 (3), 953–964. doi:10.1007/s10549-011-1662-4
Katkade, V. B., Sanders, K. N., and Zou, K. H. (2018). Real world data: an opportunity to supplement existing evidence for the use of long-established medicines in health care decision making. J. Multidiscip. Healthc. 11, 295–304. doi:10.2147/JMDH.S160029
Le, C. P., Nowell, C. J., Kim-Fuchs, C., Botteri, E., Hiller, J. G., Ismail, H., et al. (2016). Chronic stress in mice remodels lymph vasculature to promote tumour cell dissemination. Nat. Commun. Mar. 1 7, 10634. doi:10.1038/ncomms10634
Lofling, L. L., Stoer, N. C., Sloan, E. K., Chang, A., Gandini, S., Ursin, G., et al. (2022). β-Blockers and breast cancer survival by molecular subtypes: a population-based cohort study and meta-analysis. Br. J. cancer 127 (6), 1086–1096. doi:10.1038/s41416-022-01891-7
Lu, H., Liu, X., Guo, F., Tan, S., Wang, G., et al. (2015). Impact of beta-blockers on prostate cancer mortality: a meta-analysis of 16,825 patients. Onco Targets Ther. 8, 985–990. doi:10.2147/OTT.S78836
Lund, J. L., Richardson, D. B., and Sturmer, T. (2015). The active comparator, new user study design in pharmacoepidemiology: historical foundations and contemporary application. Curr. Epidemiol. Rep. Dec 2 (4), 221–228. doi:10.1007/s40471-015-0053-5
Madden, R., Fortune, N., and Gordon, J. (2022). Health statistics in Australia: what we know and do not know. Int. J. Environ. Res. Public Health 19 (9), 4959. doi:10.3390/ijerph19094959
Magnon, C., Hall, S. J., Lin, J., Xue, X., Gerber, L., Freedland, S. J., et al. (2013). Autonomic nerve development contributes to prostate cancer progression. Science 341 (6142), 1236361. doi:10.1126/science.1236361
Malik, J. A., Ahmed, S., Momin, S. S., Shaikh, S., Alafnan, A., Alanazi, J., et al. (2023). Drug repurposing: a new hope in drug discovery for prostate cancer. ACS Omega. Jan. 8 (1), 56–73. doi:10.1021/acsomega.2c05821
Melhem-Bertrandt, A., Chavez-Macgregor, M., Lei, X., Brown, E. N., Lee, R. T., Meric-Bernstam, F., et al. (2011). Beta-blocker use is associated with improved relapse-free survival in patients with triple-negative breast cancer. J. Clin. Oncol. 29 (19), 2645–2652. doi:10.1200/JCO.2010.33.4441
Mellish, L., Karanges, E. A., Litchfield, M. J., Schaffer, A. L., Blanch, B., Daniels, B. J., et al. (2015). The Australian pharmaceutical benefits Scheme data collection: a practical guide for researchers. BMC Res. Notes. Nov. 2 8, 634. doi:10.1186/s13104-015-1616-8
Modi, N. D., Tan, J. Q. E., Rowland, A., Koczwara, B., Kichenadasse, G., McKinnon, R. A., et al. (20202020). The influence of pre-existing beta-blockers use on survival outcomes in HER2 positive advanced breast cancer: pooled analysis of clinical trial data. Front. Oncol. 10 (1130), 1130. doi:10.3389/fonc.2020.01130
Mohammadzadeh, Z., Ghazisaeedi, M., Nahvijou, A., Rostam Niakan Kalhori, S., Davoodi, S., and Zendehdel, K. (2017). Systematic review of hospital based cancer registries (HBCRs): necessary tool to improve quality of care in cancer patients. Asian Pac J. Cancer Prev. 18 (8), 2027–2033. doi:10.22034/APJCP.2017.18.8.2027
Multiple causes of death (2012). 105 Multiple causes of death: An analysis of all natural and selected chronic disease causes of death 1997-2007. Australia: Australian Institute of Health and Welfare.
Ng, L., Foo, D. C., Wong, C. K., Man, A. T., Lo, O. S., and Law, W. L. (2021). Repurposing DPP-4 inhibitors for colorectal cancer: a retrospective and single center study. Cancers (Basel) 13 (14), 3588. doi:10.3390/cancers13143588
Okuyama, A., Shibata, A., and Nishimoto, H. (2018). Critical points for interpreting patients' survival rate using cancer registries: a literature review. J. Epidemiol. Feb 5 28 (2), 61–66. doi:10.2188/jea.JE20160180
Ozery-Flato, M., Goldschmidt, Y., Shaham, O., Ravid, S., and Yanover, C. (2020). Framework for identifying drug repurposing candidates from observational healthcare data. JAMIA Open 3 (4), 536–544. doi:10.1093/jamiaopen/ooaa048
Palm, D., Lang, K., Niggemann, B., Drell, T. L., Masur, K., Zaenker, K. S., et al. (2006). The norepinephrine-driven metastasis development of PC-3 human prostate cancer cells in BALB/c nude mice is inhibited by beta-blockers. Int. J. Cancer 118 (11), 2744–2749. doi:10.1002/ijc.21723
Park, J. Y., Lim, M. C., Baek, M. H., Park, Y. H., and Kim, S. (2021). Impact of metformin on survival outcome in ovarian cancer: a nationwide population-based cohort study. J. Gynecol. Oncol. Jul 32 (4), e65. doi:10.3802/jgo.2021.32.e65
Parvathaneni, V., Kulkarni, N. S., Muth, A., and Gupta, V. (2019). Drug repurposing: a promising tool to accelerate the drug discovery process. Drug Discov. Today 24 (10), 2076–2085. doi:10.1016/j.drudis.2019.06.014
Pasquier, E., Ciccolini, J., Carre, M., Giacometti, S., Fanciullino, R., Pouchy, C., et al. (2011). Propranolol potentiates the anti-angiogenic effects and anti-tumor efficacy of chemotherapy agents: implication in breast cancer treatment. Oncotarget 2 (10), 797–809. doi:10.18632/oncotarget.343
Pop, B., Fetica, B., Blaga, M. L., Trifa, A. P., Achimas-Cadariu, P., Vlad, C. I., et al. (2019). The role of medical registries, potential applications and limitations. Med. Pharm. Rep. Jan. 92 (1), 7–14. doi:10.15386/cjmed-1015
Powe, D. G., Voss, M. J., Zanker, K. S., Habashy, H. O., Green, A. R., Ellis, I. O., et al. (2010). Beta-blocker drug therapy reduces secondary cancer formation in breast cancer and improves cancer specific survival. Oncotarget. Nov. 1 (7), 628–638. doi:10.18632/oncotarget.101009
Pushpakom, S., Iorio, F., Eyers, P. A., Escott, K. J., Hopper, S., Wells, A., et al. (2019). Drug repurposing: progress, challenges and recommendations. Nat. Rev. Drug Discov. Jan. 18 (1), 41–58. doi:10.1038/nrd.2018.168
Quan, H., Sundararajan, V., Halfon, P., Fong, A., Burnand, B., Luthi, J. C., et al. (2005). Coding algorithms for defining comorbidities in ICD-9-CM and ICD-10 administrative data. Med. Care. Nov. 43 (11), 1130–1139. doi:10.1097/01.mlr.0000182534.19832.83
Reeder, A., Attar, M., Nazario, L., Bathula, C., Zhang, A., Hochbaum, D., et al. (2015). Stress hormones reduce the efficacy of paclitaxel in triple negative breast cancer through induction of DNA damage. Br. J. cancer 112 (9), 1461–1470. doi:10.1038/bjc.2015.133
Roy, S., Dhaneshwar, S., and Bhasin, B. (2021). Drug repurposing: an emerging tool for drug reuse, recycling and discovery. Curr. Drug Res. Rev. 13 (2), 101–119. doi:10.2174/2589977513666210211163711
Sakellakis, M., Kostaki, A., Starakis, I., and Koutras, A. (2014). β-Blocker use and risk of recurrence in patients with early breast cancer. Chemotherapy 60 (5-6), 288–289. doi:10.1159/000371871
Shaashua, L., Shabat-Simon, M., Haldar, R., Matzner, P., Zmora, O., Shabtai, M., et al. (2017). Perioperative COX-2 and beta-adrenergic blockade improves metastatic biomarkers in breast cancer patients in a phase-II randomized trial. Clin. Cancer Res. 23 (16), 4651–4661. doi:10.1158/1078-0432.CCR-17-0152
Sloan, E. K., Priceman, S. J., Cox, B. F., Yu, S., Pimentel, M. A., Tangkanangnukul, V., et al. (2010). The sympathetic nervous system induces a metastatic switch in primary breast cancer. Cancer Res. 70 (18), 7042–7052. doi:10.1158/0008-5472.CAN-10-0522
Sorensen, G. V., Ganz, P. A., Cole, S. W., Pedersen, L. A., Sørensen, H. T., Cronin-Fenton, D. P., et al. (2013). Use of beta-blockers, angiotensin-converting enzyme inhibitors, angiotensin II receptor blockers, and risk of breast cancer recurrence: a Danish nationwide prospective cohort study. J. Clin. Oncol. 31 (18), 2265–2272. doi:10.1200/JCO.2012.43.9190
Spini, A., Roberto, G., Gini, R., Bartolini, C., Bazzani, L., Donnini, S., et al. (2019). Evidence of beta-blockers drug repurposing for the treatment of triple negative breast cancer: a systematic review. Neoplasma. Nov. 66 (6), 963–970. doi:10.4149/neo_2019_190110N34
Suissa, S. (2008). Immortal time bias in pharmaco-epidemiology. Am. J. Epidemiol. Feb 15 167 (4), 492–499. doi:10.1093/aje/kwm324
Tan, G. S. Q., Sloan, E. K., Lambert, P., Kirkpatrick, C. M. J., and Ilomaki, J. (2023). Drug repurposing using real-world data. Drug Discov. Today 28 (1), 103422. doi:10.1016/j.drudis.2022.103422
Tan, K. S., Eguchi, T., and Adusumilli, P. S. (2018). Competing risks and cancer-specific mortality: why it matters. Oncotarget. Jan. 26 9 (7), 7272–7273. doi:10.18632/oncotarget.23729
The International Statistical Classification (2019). The international statistical classification of diseases and related Health Problems, Tenth revision, Australian modification (ICD-10-AM). 11th ed. Australian: Australian Consortium for Classification Development.
Valsecchi, M. G., and Steliarova-Foucher, E. (2008). Cancer registration in developing countries: luxury or necessity? Lancet Oncol. Feb 9 (2), 159–167. doi:10.1016/S1470-2045(08)70028-7
Victorian Cancer Quality (2018). Victorian cancer quality index technical appendix. New York: Victorian Department of Health and Human Services.
Watkins, J. L., Thaker, P. H., Nick, A. M., Ramondetta, L. M., Kumar, S., Urbauer, D. L., et al. (2015). Clinical impact of selective and nonselective beta-blockers on survival in patients with ovarian cancer. Cancer 121 (19), 3444–3451. doi:10.1002/cncr.29392
Weberpals, J., Jansen, L., Carr, P. R., Hoffmeister, M., and Brenner, H. (2016). Beta blockers and cancer prognosis - the role of immortal time bias: a systematic review and meta-analysis. Cancer Treat. Rev. Jun 47, 1–11. doi:10.1016/j.ctrv.2016.04.004
Weigelt, B., Peterse, J. L., and van 't Veer, L. J. (2005). Breast cancer metastasis: markers and models. Nat. Rev. Cancer. Aug 5 (8), 591–602. doi:10.1038/nrc1670
Wong, C. H., Siah, K. W., and Lo, A. W. (2019). Estimation of clinical trial success rates and related parameters. Biostatistics 20 (2), 273–286. doi:10.1093/biostatistics/kxx069
Xu, H., Li, J., Jiang, X., and Chen, Q. Electronic health records for drug repurposing: current status, challenges, and future directions. Clin. Pharmacol. Ther. Apr 2020;107(4):712–714. doi:10.1002/cpt.1769
Yap, A., Lopez-Olivo, M. A., Dubowitz, J., Pratt, G., Hiller, J., Gottumukkala, V., et al. (2018). Effect of beta-blockers on cancer recurrence and survival: a meta-analysis of epidemiological and perioperative studies. Br. J. Anaesth. 121 (1), 45–57. doi:10.1016/j.bja.2018.03.024
Yuen, E., Louis, D., Cisbani, L., Rabinowitz, C., De Palma, R., Maio, V., et al. (2011). Using administrative data to identify and stage breast cancer cases: implications for assessing quality of care. Tumori 97 (4), 428–435. doi:10.1700/950.10393
Keywords: drug repurposing, real-world data, administrative healthcare data, breast cancer, beta-blocker, cancer mortality, cancer survival
Citation: Tan GSQ, Botteri E, Wood S, Sloan EK and Ilomäki J (2023) Using administrative healthcare data to evaluate drug repurposing opportunities for cancer: the possibility of using beta-blockers to treat breast cancer. Front. Pharmacol. 14:1227330. doi: 10.3389/fphar.2023.1227330
Received: 23 May 2023; Accepted: 28 July 2023;
Published: 10 August 2023.
Edited by:
Mohammed S. Salahudeen, University of Tasmania, AustraliaReviewed by:
Sirajudheen Anwar, University of Hail, Saudi ArabiaDavid Dora, Semmelweis University, Hungary
Copyright © 2023 Tan, Botteri, Wood, Sloan and Ilomäki. This is an open-access article distributed under the terms of the Creative Commons Attribution License (CC BY). The use, distribution or reproduction in other forums is permitted, provided the original author(s) and the copyright owner(s) are credited and that the original publication in this journal is cited, in accordance with accepted academic practice. No use, distribution or reproduction is permitted which does not comply with these terms.
*Correspondence: George S. Q. Tan, shao.tan@monash.edu; Jenni Ilomäki, jenni.ilomaki@monash.edu